匿名内部类和匿名类
Everybody loves a fad. You can pinpoint someone’s generation better than carbon dating by asking them what their favorite toys and gadgets were as a kid. Tamagotchi and pogs? You were born around 1988, weren’t you? Coleco Electronic Quarterback and Garanimals? Well well, an early X-er. A fad is cultural currency and social lubricant at the same time: even if you don’t have the thing itself, it’s a shared reference point that helps locate you as part of a particular time and place. Paradoxically, fads also help identify when a concept has gone stale, depending on who does it.
每个人都喜欢时尚。 通过询问某人小时候最喜欢的玩具和小玩意,可以比碳测年更好地确定某人的年龄。 他妈哥池和猪? 您出生于1988年左右,不是吗? Coleco电子四分卫和Garanimals? 好吧,早期的X-er。 一时流行是文化货币和社会润滑剂:即使您本身没有东西,它也是一个共享的参考点,可以帮助您在特定的时间和地点定位自己。 矛盾的是,时尚还有助于确定概念何时过时,具体取决于谁。
Fads happen in business, too. From corporate retreats to themed attire days (back in the olden times when we went to retreats, offices or, you know, anywhere) or the more recent mandatory fun on Zoom, enterprises are no less susceptible to fads, especially when they involve technology. Part of it is a desire to seem cutting edge, but a large part of it, we think, is simple misunderstanding. Without a good grasp of new systems and tools or the concepts that underlie them, it’s hard to tell the difference between a fad and a future.
时尚也在商业中发生。 从公司务虚会到主题化的装束日子(从前我们到务虚会,办公室或任何地方都可以参观)或最近在Zoom上享受的强制性娱乐,企业都同样容易受到时尚的影响, 特别是当涉及技术时。 它的一部分是希望看起来很前沿,但我们认为,很大一部分是简单的误解。 如果不能很好地掌握新系统和工具或它们背后的概念,就很难说出时尚与未来之间的区别。
Guess Who?!
猜猜是谁?!
Case in point: anonymization. Although the concept of masking identity or erasing identifiable features has long been a component of data science, it was not a widespread topic of discussion in industry in the US until the late 2000s and, really, just before GDPR came into effect and fears of 4% penalties kicked in. Hundreds of vendors promise services that allow you to “anonymize” user data in an effort to find safe harbors or avoid liability, but most businesses have only a vague understanding of what the concept of anonymized data really is and how to do it.
例子:匿名化。 尽管掩盖身份或擦除可识别特征的概念长期以来一直是数据科学的组成部分,但直到2000年代后期,而且直到GDPR生效和人们担心4时,它才成为美国工业界广泛讨论的话题。罚款率开始上升。成百上千的供应商承诺提供服务,使您可以“匿名”用户数据,以寻找安全港或避免承担责任,但大多数企业对匿名数据的真正含义以及如何使用这些概念只有模糊的了解。做吧。
To unpack anonymous data, it’s important to clear up a few terms so that we don’t run into confusion. First, what is anonymized? Anonymous data is data that does not relate to an identified or identifiable natural person, or data modified such that the data subject is not or no longer identifiable.
要解包匿名数据,重要的是要清理一些术语,以免引起混乱。 首先,匿名是什么? 匿名数据是与已识别或可识别的自然人无关的数据,或者经过修改使得数据主体不再或不再可识别的数据。
That is an extremely vague definition for a concept that is so important, and so let’s dive into that a little more, because this is a game of definitions (every lawyer’s favorite game). If data, on its own or with other data, can identify you, it’s personal data. We don’t talk about personally identifiable information, any more; that fad has passed. These days, you only talk about personal data.
对于一个非常重要的概念来说,这是一个非常模糊的定义,因此让我们再深入一点,因为这是一个定义游戏(每个律师最喜欢的游戏)。 如果数据本身或与其他数据一起可以识别您的身份 ,那就是个人数据 。 我们不再谈论个人身份信息; 这种时尚已经过去。 这些天,您只谈论个人数据。
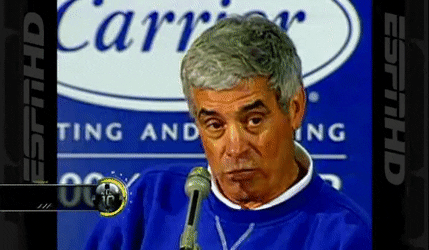
There are ways to make data less useful in identifying a person, but that does not mean that it is anonymous. Instead, there are varying degrees of data obfuscation — means hiding attributes to make reidentification more difficult — on the way to actual anonymization. Here are the two most important kinds.
有一些方法可以使数据在识别个人时不那么有用,但这并不意味着它是匿名的。 取而代之的是,在进行实际匿名处理的过程中,存在各种程度的数据混淆 -意味着隐藏属性以使重新识别更加困难。 这是两个最重要的种类。
Masked Data
屏蔽数据
Masked Data is information modified to hide (or “mask”) the underlying, true data. This is a common practice in business, and it is most effective against unauthorized internal review (and pilfering) of valuable business/customer data and against external actors learning important details about clients and vendors. A simplified explanation of masked data is a customer list that details first and last name, age, address, and amount spent with surnames changed to dummy names, ages shifted, and amounts spent reallocated randomly. Much of the derivative analytic data remains the same (amounts spent, total number of customers, locations of accounts, etc) but it is difficult to reidentify any individual user.
屏蔽数据是经过修改以隐藏(或“屏蔽”)基础真实数据的信息。 这是业务中的常见做法,对于防止对有价值的业务/客户数据进行未经授权的内部审阅(和窃取)以及对了解有关客户和供应商重要细节的外部参与者而言,这是最有效的。 屏蔽数据的简化说明是一个客户列表,其中详细列出了姓氏和名字,年龄,地址和花费的金额,其中姓氏更改为虚拟名称,年龄变化和花费的费用随机分配。 许多派生分析数据保持不变(花费金额,客户总数,帐户位置等),但是很难重新识别任何单个用户。
What it Isn’t
不是什么
Having a list where the names and identifiers are shifted is a great business approach, but it usually falls short of anonymous in the real world. Why? Because usable data is accurate data, and being able to run the kind of analytics you want means being able to easily mix and match the true underlying information. As such, having the master list (the non-masked data) available means that you will always hold onto the original information, which means you’re still holding personal data, which means you’re not protected by the anonymity safe harbor. Thanks for playing.
列出名称和标识符在其中进行了移位的列表是一种很好的业务方法,但是在现实世界中通常缺少匿名性。 为什么? 因为可用数据是准确的数据,并且能够运行您想要的那种分析,则意味着能够轻松地混合和匹配真实的基础信息。 因此,拥有主列表(未屏蔽的数据)意味着您将始终保留原始信息,这意味着您仍在保留个人数据,这意味着您不受匿名安全港的保护。 感谢参与。
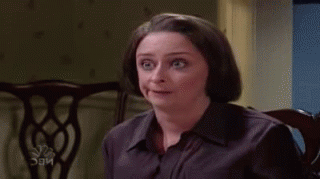
Pseudonymized Data
假名数据
Pseudonymous data is data that has the most important identifiers removed: names, email addresses, social security numbers, etc. Pseudonymous data still identifies a person, but it isn’t obvious on its face who that person is. Think back to school when they would post grades outside of a classroom but only use student numbers on the chart. In the Mad-Max rush to the sheet of paper to see your grades, it wasn’t possible to see anyone else’s name, and so you only were able to know what your outcome was. This is a good example of pseudonymization and a good example of why it’s used: to protect the rights of individuals from unnecessary exposure of their personal details, including a devastatingly embarrassing failed geometry test in ninth grade.
假名数据是除去了最重要的标识符的数据:姓名,电子邮件地址,社会保险号等。假名数据仍可以识别一个人,但从表面上看不出该人是谁。 当他们想在教室外发布成绩但只在图表上使用学生人数时,请回想学校。 在疯狂的麦克斯(Mad-Max)急于浏览纸质成绩的过程中,不可能看到别人的名字,因此您只能知道结果是什么。 这是假名的一个很好的例子,也是一个为什么使用假名的很好的例子:保护个人的权利免于不必要地暴露其个人详细信息,包括在九年级时令人尴尬的几何测试失败。
The more attributes you remove from a dataset, the thinking goes, the more pseudonymized the data becomes, and the closer it gets to full anonymization, at which point you’re in the clear.
从数据集中删除的属性越多,人们的想法就越多,数据变得越假名化,就越接近完全匿名化,这时您就很清楚了。
What it Isn’t
不是什么
A panacea, or, honestly, nearly as useful as it might sound. Pseudonymization in practice is often something like this:
灵丹妙药,或者说,听起来几乎一样有用。 在实践中,化名通常是这样的:
- We have an excel spreadsheet with names, addresses, account numbers, customer spend, and profile data. 我们有一个Excel电子表格,其中包含名称,地址,帐号,客户支出和个人资料数据。
- We delete the customer name. 我们删除客户名称。
- Presto, pseudonymized data! 预先加密的数据!
Of course, that might technically count as pseudonymization, but it’s virtually useless: you still have every other identifier for an individual, which means that not only is it not difficult to re-identify the person at issue, you haven’t even de-identified them to begin with. Think about it from a data perspective, rather than a human perspective: Column A contains alphanumeric characters used to identify an individual account, so does Column B. If they both do the same thing, what difference does it make if you delete Column A (where the alphanumeric characters are organized into what humans recognize as names) and keep Column B (where the alphanumeric characters are organized into what humans think of as an “account ID number.”)? Under the law, it’s all the same, and the database/algorithm analyzing the data won’t have any problem continuing on as before the deletion.
当然,从技术上讲 ,这可以算作假名,但这实际上是没有用的:您仍然拥有一个人的所有其他标识符,这意味着不仅不难重新识别出该人,而且甚至没有取消身份验证,确定了它们的开始。 从数据角度而不是从人类角度考虑:A列包含用于标识个人帐户的字母数字字符,B列也是如此。如果它们都执行相同的操作,则删除A列会产生什么不同(将字母数字字符组织成人类可以识别的名字)并保留B列(其中字母数字字符组织成人类认为的“帐户ID号”)? 根据法律,都是一样的,并且分析数据的数据库/算法不会像删除之前那样继续存在任何问题。
“Fine!” you shout, annoyed, “why don’t we just delete names, addresses, account numbers, and credit card information and only keep the more vague data attributes!” A great idea, and it’s the thought process behind GDPR’s approach to anonymization: if you delete enough data and remove enough identifiers, eventually you’ll get to a place where you don’t have personal data any more and the rights of natural persons are protected.
“精细!” 您大喊大叫,“为什么我们不删除姓名,地址,帐号和信用卡信息,而只保留更模糊的数据属性!” 一个好主意,这是GDPR匿名化方法的思想过程:如果删除足够的数据并删除足够的标识符,最终您将到达一个地方,不再拥有个人数据,自然人的权利得到保护。受保护的。
Except not really.
除了不是真的。
If you’re keeping any data at all, and especially if you’re keeping multiple data points and attributes, the likelihood is that you’re going to wind up capable of reidentifying an individual. A very important study in Nature Communications reviewed a variety of “anonymized” datasets and came to a pretty striking conclusion:
如果您要保留所有数据, 尤其是要保留多个数据点和属性,则很有可能您将能够重新识别个人。 自然通讯中一项非常重要的研究 回顾了各种“匿名”数据集,得出了一个非常惊人的结论:
Using our model, we find that 99.98% of Americans would be correctly re-identified in any dataset using 15 demographic attributes. Our results suggest that even heavily sampled anonymized datasets are unlikely to satisfy the modern standards for anonymization set forth by GDPR and seriously challenge the technical and legal adequacy of the de-identification release-and-forget model
使用我们的模型,我们发现使用15个人口统计属性的任何数据集都可以正确地重新识别99.98%的美国人。 我们的结果表明,即使采样大量的匿名数据集也不太可能满足GDPR设定的现代匿名标准,并严重挑战去身份化“遗忘释放”模型的技术和法律适用性
In other words, if you have enough data attributes, even “anonymous” data is nothing of the sort, which means that GDPR’s approach to anonymization (followed around the world) has a fatal flaw in the underlying thought process, and the Get-Out-Of-Brussels-Free Card that data companies thought would protect them is actually fairly useless.
换句话说,如果您有足够的数据属性,那么即使“匿名”数据也算不上什么,这意味着GDPR的匿名化方法(遍及全球)在潜在的思维过程和“走出去”中具有致命的缺陷。数据公司认为可以保护他们的无布鲁塞尔卡实际上是毫无用处的。
A Newer, Better Fad
更新,更好的时尚
This is usually the point in our blogs where we say “the good news is that there is another option” and lay out how to approach things differently. But today, we’re actually going to suggest following an older strategy to avoid some of this anonymization difficulty.
通常,在我们的博客中,我们说“好消息是还有另一种选择”,并阐明了如何以不同的方式处理事情。 但是今天,我们实际上将建议采用一种较旧的策略来避免某些匿名化难题。
Step 1: Get rid of all the data you don’t need to fulfill your core purposes tied to the data.
第1步:摆脱所有不需要的数据,即可满足与数据相关的核心目的。
Step 2: Then, once the core purpose is fulfilled, aggregate all of the data you need to run your analytics.
步骤2:然后,一旦实现了核心目的,就可以汇总运行分析所需的所有数据。
Step 3: Now delete the rest of the underlying data. Yes, all of it.
步骤3:现在删除其余的基础数据。 是的,全部。
You may be thinking that you’ve just deleted all of the data and you’d be right. That’s often the best answer: you can’t be held liable or responsible for data you no longer own. Get rid of it! Aggregated data is, in our view, the only truly anonymous data out there, because it’s not possible to walk the process back and reidentify an individual from aggregated statistics.
您可能会认为您刚刚删除了所有数据,这是对的。 通常,这是最好的答案:您不再对不再拥有的数据承担责任或承担责任。 摆脱它! 在我们看来,汇总数据是那里唯一的真正匿名数据,因为无法回退流程并从汇总统计信息中重新识别个人。
Now, will this work for everyone and for every dataset? Of course not. Sometimes you need the data for business purposes or for regulatory reasons. But in those cases, anonymization wasn’t appropriate anyway, because you have ongoing duties to protect data based on usage. Put another way, the problem with the anonymization fad is that it encourages shortcut thinking about data: “If we pseudonymize well enough, we can just do whatever we want with the data!” Except no, you can’t, and the data protection authorities are very touchy about what qualifies as properly pseudonymous or anonymized.
现在,这对所有人和每个数据集都适用吗? 当然不是。 有时您出于业务目的或出于法规原因需要数据。 但是在那种情况下,匿名化还是不合适的 ,因为您有持续的职责要根据使用情况保护数据。 换句话说,匿名化时尚的问题在于,它鼓励人们对数据进行捷径思考:“如果我们对假名足够好,我们就可以对数据做任何想做的事!” 除非否,否则您不能这样做,并且数据保护机构对于什么是适当的假名或匿名资格非常敏感 。
Is it possible to truly anonymize data? Yes. Is it the answer to all of your data concerns? Probably not, because the most important aspect to your data is how you use it, how you learn from it, and how you leverage it to grow. Anonymized data is stripped of much of its usefulness in favor of a flimsy sense of getting out of regulatory oversight. In the end, it’s a far better plan to protect the data you want, delete the data you don’t, create anonymous data only if it fits certain limited parameters, and leave the fads to the other folks. This approach gives you more time, resources, and money — and they never go out of fashion.
是否可以真正匿名化数据? 是。 是您所有数据问题的答案吗? 可能不是,因为数据最重要的方面是如何使用数据,如何学习数据以及如何利用数据进行增长。 匿名数据被剥夺了大部分有用性,转而摆脱了监管监督的脆弱感。 最后,这是一个更好的计划,可以保护所需的数据,删除不需要的数据,仅在满足某些有限参数的情况下创建匿名数据,然后再将风尚交给其他人。 这种方法为您提供了更多的时间,资源和金钱-而且它们永远不会过时。
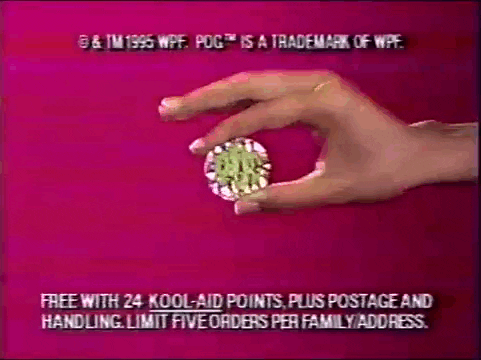
Originally published at https://wardpllc.com on September 1, 2020.
最初于 2020年9月1日 发布在 https://wardpllc.com 上。
翻译自: https://medium.com/swlh/anonymous-schanonymous-b6f6db9156bb
匿名内部类和匿名类
本文来自互联网用户投稿,该文观点仅代表作者本人,不代表本站立场。本站仅提供信息存储空间服务,不拥有所有权,不承担相关法律责任。如若转载,请注明出处:http://www.mzph.cn/news/389575.shtml
如若内容造成侵权/违法违规/事实不符,请联系多彩编程网进行投诉反馈email:809451989@qq.com,一经查实,立即删除!