Being a sneakerhead is a culture on its own and has its own industry. Every month Biggest brands introduce few select Limited Edition Sneakers which are sold in the markets according to Lottery System called ‘Raffle’. Which have created a new market of its own, where people who able to win the Sneakers from the Lottery system want to Sell at higher prices to the people who wished for the shoes more. One can find many websites like stockx.com, goat.com to resell untouched Limited Edition sneakers.
成为运动鞋者本身就是一种文化,并且拥有自己的行业。 每个月,最大的品牌都会推出极少数的限量版运动鞋,根据抽奖系统“ Raffle”的规定,这些运动鞋会在市场上出售。 这就创造了一个自己的新市场,那些能够从彩票系统中赢得运动鞋的人们希望以更高的价格卖给那些希望获得更多鞋子的人们。 您可以找到许多网站,例如stockx.com ,山羊网站(comat.com)来转售未经修饰的限量版运动鞋。
But the problem with reselling the Sneakers is, that every Limited edition sneaker is not a success, and cannot return big profits. One has to study the “hype”, “popularity” which model is a hot topic and is in discussion more than others, and if one can find that well, can gain even up to 300% profits.
但是转售Sneakers的问题在于,每一款限量版运动鞋都不会成功,也无法获得丰厚的利润。 人们必须研究“炒作”,“受欢迎程度”,该模型是一个热门话题,并且比其他人更受讨论,如果能找到一个很好的例子,则可以获得多达300%的利润。
I found a way to discover that “hype” or popularity of certain models by doing Instagram Analysis, and studying the hashtags related to the Sneakers, and find out which Sneaker is a unicorn.
我找到了一种方法,可以通过进行Instagram分析并研究与运动鞋相关的标签来发现某些模型的“炒作”或受欢迎程度,并找出哪个运动鞋是独角兽。
数据抓取和准备数据 (Data Scraping and Preparing the data)
Instagram Api doesn't let you study about likes and comments on other profiles,so instead of using Instagram Api, I used Data scraping. To scrape data from Instagram you will need a hash query like this.
Instagram Api不允许您研究其他个人资料上的喜欢和评论,因此我没有使用Instagram Api,而是使用数据抓取。 要从Instagram抓取数据,您将需要像这样的哈希查询。
url='https://www.instagram.com/graphql/query/?query_hash=c769cb6c71b24c8a86590b22402fda50&variables=%7B%22tag_name%22%3A%22azareth%22%2C%22first%22%3A2%2C%22after%22%3A%22QVFCVDVxVUdMLWlnTlBaQjNtcUktUkR4M2dSUS1lSzkzdGVkSkUyMFB1aXRadkE1RzFINHdzTmprY1Yxd0ZnemZQSFJ5Q1hXMm9KZGdLeXJuLWRScXlqMA%3D%3D%22%7D'
As you can see keyword azareth , that is my Hashtag. You can simply change that keyword to any hashtag you want to get the data from.
如您所见,关键字azareth就是我的标签 。 您可以简单地将该关键字更改为要从中获取数据的任何主题标签。
Let us select some hashtags for Air Jordan 1 “Fearless” Sneakers #airjordanfearless,#fearless,#jordanbluefearless,#fearlessjordan,#aj1fearless,#ajonefearless,#airjordanonefearless
让我们为Air Jordan 1“ Fearless”运动鞋选择一些标签,#airjordanfearless,#fearless,#jordanbluefearless,#fearlessjordan,#aj1fearless,#ajonefearless,#airjordanonefearless
#Creating a dataframe with columns hashtags
airjordanfearless = ["airjordanfearless","fearless","jordanbluefearless","fearlessjordan","aj1fearless","ajonefearless","airjordanonefearless"]
airjordanfearless=pd.DataFrame(airjordanfearless)
airjordanfearless.columns=["hashtag"]
#Creating a Coloumn of URL in order to place respective urls of the hashtags
url='https://www.instagram.com/graphql/query/?query_hash=c769cb6c71b24c8a86590b22402fda50&variables=%7B%22tag_name%22%3A%22azareth%22%2C%22first%22%3A2%2C%22after%22%3A%22QVFCVDVxVUdMLWlnTlBaQjNtcUktUkR4M2dSUS1lSzkzdGVkSkUyMFB1aXRadkE1RzFINHdzTmprY1Yxd0ZnemZQSFJ5Q1hXMm9KZGdLeXJuLWRScXlqMA%3D%3D%22%7D'
airjordanfearless["url"]= url
#code to replace the hashtag in the query URL
airjordanfearless['url'] = airjordanfearless['hashtag'].apply(lambda x : url.replace('azareth',x.lower()))
After we have a Dataframe, Its time to see what we can do with the Instagram hash query. We can find Total Likes, Total Comments, Total posts related to a certain hashtag and these parameters can help us predict the “hype” and “popularity” of the sneakers.
有了Dataframe之后,该该看看该如何处理Instagram哈希查询了。 我们可以找到总喜欢,总评论,与某个标签相关的总帖子 ,而这些参数可以帮助我们预测运动鞋的“炒作”和“受欢迎程度”。
We will need urlib and requests libraries to open the URL and retrieve certain values we require like Total Likes, Total Comments, or even images themselves.
我们将需要urlib并请求库来打开URL并检索我们需要的某些值,例如“总喜欢”,“总评论”,甚至是图像本身。
import urllib.request
import requests#opening the url and reading it to decode and search for parametrs edge_media_preview_like,edge_media_to_comment,edge_hashtag_to_mediaairjordanfearless['totalikes'] = airjordanfearless['url'].apply(lambda x :(urllib.request.urlopen(x).read().decode('UTF-8').rfind("edge_media_preview_like")))
airjordanfearless['totalcomments'] = airjordanfearless['url'].apply(lambda x :(urllib.request.urlopen(x).read().decode('UTF-8').rfind("edge_media_to_comment")))
airjordanfearless['totalposts'] = airjordanfearless['url'].apply(lambda x :(urllib.request.urlopen(x).read().decode('UTF-8').rfind("edge_hashtag_to_media")))
airjordanfearless['releaseprice'] = 160
airjordanfearless
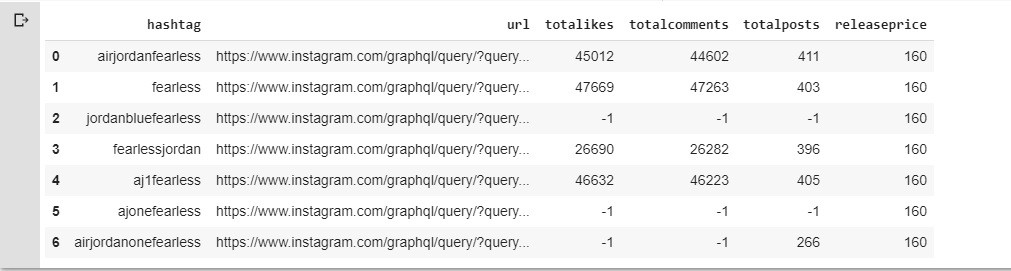
In order to create train data, I made similar data frames of some selected sneakers -Yeezy700 Azareth,Nike X Sacai Blazar,Puma Ralph Sampson OG,Nike SB Dunk X Civilist , Nike Space Hippie Collection.
为了创建火车数据,我对一些选定的运动鞋(Yeezy700 Azareth,Nike X Sacai Blazar,Puma Ralph Sampson OG,Nike SB Dunk X Civilist和Nike Space Hippie Collection)进行了类似的数据制作。
I took mean Values of Total Likes , comments and posts of all hashtags of each Sneakers to create Training Data.Max Resale prices of the following Sneakers were taken from goat.com.
我以平均总喜欢值,评论和每个运动鞋的所有标签的帖子来创建培训数据。以下运动鞋的最高转售价来自山羊网站。
traindata = {'name': ['yeezyazareth','airjordanfearless','sacainikeblazar' ,'pumaralphsamson' ,'nikedunkcivilist' ,'nikespacehippie'],'likes': [yeezyazareth.totalikes.mean(),airjordanfearless.totalikes.mean(),sacainikeblazar.totalikes.mean(),pumaralphsamson.totalikes.mean(),nikedunkcivilist.totalikes.mean(),nikespacehippie.totalikes.mean()],'comment': [yeezyazareth.totalcomments.mean(),airjordanfearless.totalcomments.mean(),sacainikeblazar.totalcomments.mean(),pumaralphsamson.totalcomments.mean(),nikedunkcivilist.totalcomments.mean(),nikespacehippie.totalcomments.mean()],'post': [yeezyazareth.totalposts.mean(),airjordanfearless.totalposts.mean(),sacainikeblazar.totalposts.mean(),pumaralphsamson.totalposts.mean(),nikedunkcivilist.totalposts.mean(),nikespacehippie.totalposts.mean()],'releaseprice': [yeezyazareth.releaseprice[1],airjordanfearless.releaseprice[1],sacainikeblazar.releaseprice[1],pumaralphsamson.releaseprice[1],nikedunkcivilist.releaseprice[1],nikespacehippie.releaseprice[1]],'maxresaleprice': [361,333,298,115,1000,330], #maxresaleprice data taken from goat.com'popular':[1,1,1,0,2,1]}df = pd.DataFrame (traindata, columns = ['name','likes','comment','post','releaseprice','maxresaleprice','popular'])
df

数据培训和ANN模型构建 (Data Training and ANN Model Building)
DATA TRAINING
资料训练
1- The hash query gives most recent photos from Instagram for certain hashtags, so it reduces the possibility of having any old-model sneakers photos into the data, this validates, as “HYPE” or “popularity” of a certain sneaker is possibly estimated from the most recent photos, so we can know which sneakers are in Talk and hot right now and have could more resale values.
1-哈希查询可提供来自Instagram最新照片中的特定标签,因此可以减少将任何旧款运动鞋照片纳入数据的可能性,这可以验证,因为可能会估计某个运动鞋的“ HYPE”或“人气”从最近的照片中,我们可以知道哪些运动鞋现在处于Talk和热门状态,并且可能具有更多的转售价值。
2- For any possibility of Hashtags overlaps over photos,(which is quite possible) I talk mean counts of TOTAL LIKES/COMMENTS and POSTS to train data and to predict resale prices.
2-对于标签在照片上重叠的任何可能性(这很有可能),我说的是“总数” /“评论”和“帖子”的均值,以训练数据并预测转售价格。
3- To validate the model instead of splitting data to Train or test we can simply put hashtags into x_test of the recent release of a sneaker and compare our predictions with actual ongoing resale price.
3-为了验证模型,而不是将数据分割以进行训练或测试,我们可以简单地将标签添加到运动鞋最新版本的x_test中,然后将我们的预测与实际的持续转售价格进行比较。
Artificial Neural Network
人工神经网络
For X , I took variable “likes”, “comment”, “post”, “releaseprice” and for Y/Labels I used the “maxretailprices” in order to make the model learn itself to place weight differently in neurons from getting data from the x variables and reach to “maxretailprices” /y data, and find a pattern between Likes and comments and number of posts on Instagram to Max retail prices.
对于X,我采用了变量“喜欢”,“评论”,“发布”,“发行价格”,对于Y / Label,我使用了“最大零售价格”,以使模型从从获取数据中学习到如何在神经元中放置不同的权重x变量并到达“ maxretailprices” / y数据,并在“顶”和“评论”之间以及在Instagram上的帖子数量到最大零售价格之间找到一种模式。
The reason being, more likes, comments, and Posts on Instagram related to a particular Sneakers will reflect its hype, popularity among Instagram users, and Model can find accurate weights to determine the relation between
原因是,与某个特定运动鞋相关的更多喜欢,评论和Instagram上的帖子将反映其炒作,在Instagram用户中的流行度,并且Model可以找到准确的权重来确定两者之间的关系。
x = df[["likes","comment","post","releaseprice"]]
x=np.asarray(x)
y=np.asarray(df.maxresaleprice)
y
Model Tuning
模型调整
Learning Rate — I selected Low learning rate of 0.001 , in order to let model find weights and make gradients without overshooting the minima.
学习率 —我选择了0.001的低学习率,以使模型找到权重并进行渐变而不会超出最小值。
Loss method- I selected MSE as a loss method, as I am trying to find relations between the variable so it is a type of regression
损失法 -我选择MSE作为损失法,因为我试图查找变量之间的关系,因此它是一种回归类型
Activation Method -Relu was the best option, as it turns all negative values to 0 (being Instagram showing -1 values for 0) and places the exact value if higher then 0)
激活方法 -Relu是最好的选择,因为它将所有负值都变为0(Instagram表示0的值为-1),如果大于0则放置确切的值)
Layers and Neurons- I played with Neurons and layers to find the best combination where gradients do not blow up , and minimizes loss at best, and able to find patterns and weights better with 50 epochs.
图层和神经元 -我与神经元和图层一起使用,以找到梯度不爆破的最佳组合,并最大程度地减少了损失,并能够在50个历元时更好地找到样式和权重。
from keras.models import Model
from keras.layers import Input
from keras.layers import Dense
from keras.models import Sequential
import tensorflow as tf
model= Sequential()model.add(Dense(10,input_shape=[4,], activation='relu'))
model.add(Dense(30, activation='relu'))
model.add(Dense(1, activation='relu'))
mse = tf.keras.losses.MeanSquaredError()model.compile('Adam',loss=mse)
model.optimizer.lr =0.001model.fit(x,y,epochs=50, batch_size=10, verbose=1)
结果 (RESULTS)
I did not create big enough train data in order to split data between train and test. So in order to Verify results, I simply created an x_test data frame of some recent releases like the way I shown you and compared the predictions of my model with Resale prices with goat.com.Here is an example with Nike dunk LOW sb Black with resale price on goat.com 326 Euros
我没有创建足够大的火车数据来在火车和测试之间分配数据。 因此,为了验证结果,我只是创建了一些最新版本的x_test数据框(如我向您展示的方式),然后将其模型的预测与山羊皮.com的转售价格进行了比较。以下是Nike dunk LOW sb Black与出售价格goat.com 326欧元


For, complete jupyter notebook and code, you can view my repository over github.com — https://github.com/Alexamannn/Instagram-analysis-to-predict-Sneaker-resale-prices-with-ANN
对于完整的Jupyter笔记本和代码,您可以在github.com上查看我的存储库— https://github.com/Alexamannn/Instagram-analysis-to-predict-Sneaker-resale-prices-with-ANN
翻译自: https://medium.com/analytics-vidhya/instagram-analysis-to-predict-limited-edition-sneakers-resale-price-with-ann-5838cbecfab3
本文来自互联网用户投稿,该文观点仅代表作者本人,不代表本站立场。本站仅提供信息存储空间服务,不拥有所有权,不承担相关法律责任。如若转载,请注明出处:http://www.mzph.cn/news/389560.shtml
如若内容造成侵权/违法违规/事实不符,请联系多彩编程网进行投诉反馈email:809451989@qq.com,一经查实,立即删除!