r psm倾向性匹配
Retail managers have been facing an extraordinary time with the COVID-19 pandemic. But the typical plans to prepare for seasonal sales will be a new challenge. More seasonal products have been introduced over the years, making August the best point to conduct a seasonal analysis. So this means you, as a marketer, can get to understand seasonal shopping
零售经理面对COVID-19大流行正处于非同寻常的时期。 但是为季节性销售做准备的典型计划将是一个新的挑战。 多年来引入了更多的季节性产品,使8月成为进行季节性分析的最佳时机。 因此,这意味着您作为营销人员可以了解季节性购物
I have a nickname for the online metrics associated with seasonal retail behavior. I call them Pumpkin Spice Metrics or PSMs, given that so many products with a pumpkin theme arrive in stores during the fall.
我有一个与季节性零售行为相关联的在线指标的昵称。 鉴于秋季有许多以南瓜为主题的产品到货,因此我将它们称为“南瓜香料”或“ PSM”。
Pumpkin Spice Metrics aid overall marketing activity by defining what typically happens during a seasonal sales period. The metrics themselves are not technologically different than the ones chosen for digital marketing campaigns or ones related to KPI (key performance indicators — metrics related to business objectives). The key value in establishing PSMs is to determine segments and activity based on the seasonal influences and campaign details that are known.
南瓜香料度量标准通过定义季节性销售期间通常发生的情况来辅助总体营销活动。 指标本身在技术上与为数字营销活动选择的指标或与KPI相关的指标(技术指标-与业务目标相关的指标)没有技术差异。 建立PSM的关键价值是根据已知的季节性影响和活动详细信息来确定细分和活动。
So where should a business start to plan effective measurement against the seasons, especially as shopping behavior has shifted?
那么,企业应该从哪里开始计划针对季节的有效衡量标准,尤其是在购物行为发生变化时?
One clear starting point is just looking at what regional traffic has been typical. Different regions celebrate seasonal events in which businesses participate with promotions. This means more activity related to those events can appear in the analytics as increases from a local region.
一个明确的起点只是看区域交通的典型情况。 不同地区庆祝企业参加促销活动的季节性活动。 这意味着与这些事件相关的更多活动可以随着本地区域的增加而出现在分析中。
So imagine website visits associated with Los Angeles or Houston experiencing a rising count near the time of Cinco De Mayo, a celebration of Mexican culture and heritage. Both regions are home to the largest Hispanic populations in the United States, while a Wikipedia post on Cinco De Mayo notes that the celebration, originated in the southwestern region of the United States, is celebrated in large cities with large Mexican-American populations. Companies with products normally purchased near the May 5th date would review visits to see if they map to those locations, then decide where to best deploy advertising.
因此,想象一下在临近Cinco De Mayo(庆祝墨西哥文化和遗产)的时期,与洛杉矶或休斯顿相关的网站访问量正在上升。 这两个地区都是美国最大的西班牙裔人口的家园,而维基百科上Cinco De Mayo的帖子则指出,这项庆祝活动起源于美国西南部地区,是在墨西哥裔美国人人口众多的大城市中进行庆祝的。 购买产品通常在5月5日前后购买的公司会审核访问,以查看它们是否映射到这些位置,然后决定在哪里最好地部署广告。
There are several ways to do this in an analytics solution. The easiest is to set up a geolocation report in Google Analytics or Adobe Analytics. Chart the number of visits from a region in which the population is understood. Analysts usually examine the reports for a consistent number of visits, with signs of engagement, either by time spent on-site or by conversion rate.
在分析解决方案中,有几种方法可以做到这一点。 最简单的方法是在Google Analytics(分析)或Adobe Analytics中设置地理位置报告。 绘制来自了解人口的区域的访问次数。 分析师通常根据在现场花费的时间或转化率来检查报告中是否有一致的访问次数,并带有参与的迹象。
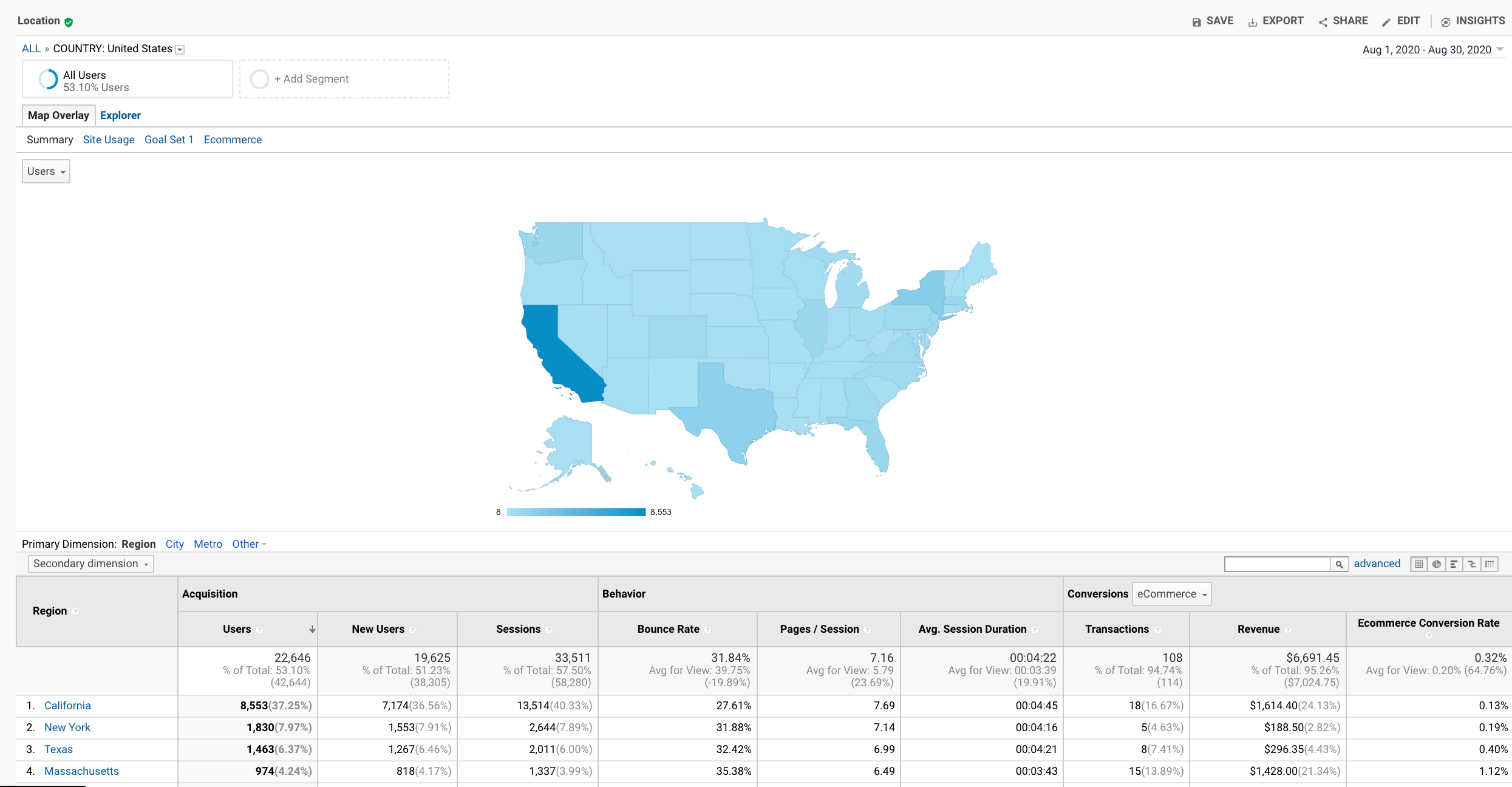
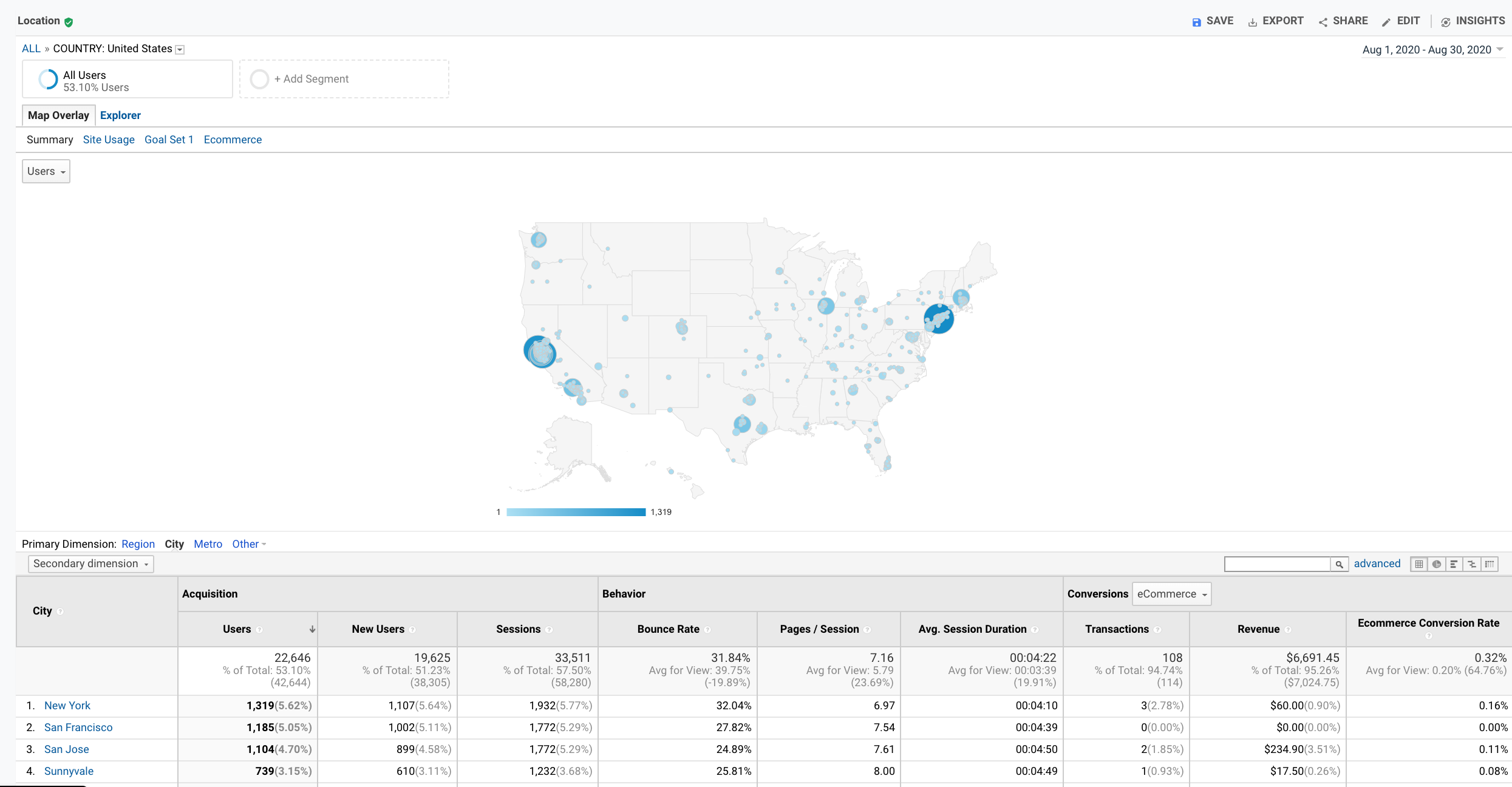
A quick aside: Conversion rate is the percentage of visitors who complete a given activity on your site. This metric is set in the analytics manually in the goals setting.
简单来说:转化率是完成您网站上给定活动的访问者的百分比。 该指标是在目标设置中的分析中手动设置的。
Another key analysis is using time as a demarcation between a seasonal period and time leading up to the season. Search patterns can change between a season and lead up period to the season. Marking the time periods can reveal search patterns allowing you to ask “Are there any keywords or phrases that are appearing regularly before the season?” Those words may be opportunities to adjust paid search ads or content ideas for a blog associated with a site. While hashtags are meant for social media, tweets and Pinterest pins can appear in search results. Thus the ideas from a search report can spark research ideas for hashtags as well. The end result for this exercise is to develop a list of content ideas that fits the seasons, the phrases that customers are collectively speaking.
另一个关键分析是将时间用作季节与季节之间的分界。 搜索模式可以在一个季节之间以及该季节的准备期之间变化。 标记时间段可以显示搜索模式,使您可以问:“在该季节之前是否有规律出现的关键字或词组?” 这些词可能是调整与网站关联的博客的付费搜索广告或内容创意的机会。 尽管标签是用于社交媒体的,但推文和Pinterest固定图钉可能会出现在搜索结果中。 因此,搜索报告中的构想也可以激发主题标签的研究构想。 此练习的最终结果是开发出适合季节的内容创意列表,即客户共同说出的短语。
In the instance of the fall retail seasons (Halloween, Thanksgiving, Christmas) you can look at search patterns from the time the pandemic began to the present. Comparing the search pattern from last year to this year can reveal if a past strategy will not be as effective for this year. That can make a difference in where to spend a digital ad budget or marketing resources.
在秋季零售季节(万圣节,感恩节,圣诞节)的情况下,您可以查看从大流行开始到现在的搜索模式。 比较去年和今年的搜索模式,可以发现过去的策略今年是否会不那么有效。 这可能会影响数字广告预算或营销资源的支出位置。
Businesses can consider PSMs as a factor in modeling advanced analytics, such as forecast modeling. But the behavioral differences people are exhibiting while social distancing means that a model may not be work as effectively as it would have given a standard retail year. It may be better to see if advanced modeling can be used for the next season.
企业可以将PSM作为建模高级分析(例如预测建模)的一个因素。 但是,人们在社交疏远时表现出的行为差异意味着该模型可能无法像给定的标准零售年份那样有效。 最好看看下一个赛季是否可以使用高级建模。

One advanced technique that can be used is a basket analysis. This analysis identifies the likelihood that one or more items are purchased alongside another item. A toothbrush alongside a toothpaste or mouthwash may make sense, but there could be other products that trigger the sales. The result from an analysis are suggestions for how products are mentioned on website pages, app pages, or in value coupons. Even having them appear alongside in a video would be helpful.
可以使用的一种先进技术是篮分析。 该分析确定了与另一个项目一起购买一个或多个项目的可能性。 牙刷与牙膏或漱口水一起使用可能很有意义,但可能还有其他产品触发了销售。 分析的结果是有关如何在网站页面,应用程序页面或价值优惠券中提及产品的建议。 即使让它们出现在视频中也会很有帮助。
The time period discovered for seasonal sales can be a starting point for this analysis.
发现季节性销售的时间段可以作为此分析的起点。
Overall, you just can’t be prepared for a seasonal event without the right strategy. Identifying the pumpkin spice metrics in your business is the right strategy of creating sweet campaign ideas from seasonal customer interest.
总体而言,如果没有正确的策略,您只是无法为季节性活动做好准备。 在您的业务中确定南瓜香料的度量标准是根据季节性客户兴趣创建甜美广告活动想法的正确策略。
翻译自: https://medium.com/@ZimanaAnalytics/pumpkin-spice-metrics-psm-how-to-plan-seasonal-advertising-8745bdbfe192
r psm倾向性匹配
本文来自互联网用户投稿,该文观点仅代表作者本人,不代表本站立场。本站仅提供信息存储空间服务,不拥有所有权,不承担相关法律责任。如若转载,请注明出处:http://www.mzph.cn/news/389549.shtml
如若内容造成侵权/违法违规/事实不符,请联系多彩编程网进行投诉反馈email:809451989@qq.com,一经查实,立即删除!