均线交易策略的回测 r
R Programming language is an open-source software developed by statisticians and it is widely used among Data Miners for developing Data Analysis. R can be best programmed and developed in RStudio which is an IDE (Integrated Development Environment) for R. The advantages of R programming language include quality plotting and eye catching reports, vast source of packages used for various functions, exemplary source for Data Wrangling, performance of various Machine Learning operations and flashy for Statistics. In my experience, R is the best language for self-learning and also it is very flexible for Financial Analysis and Graphing.
R编程语言是由统计学家开发的开源软件,在数据挖掘人员中广泛用于开发数据分析。 R可以在RStudio(R的一个IDE(集成开发环境))中最好地进行编程和开发。R编程语言的优点包括质量绘图和醒目报告,用于各种功能的大量软件包,用于数据整理的示例性源,各种机器学习操作的性能,并且对统计信息不满意。 以我的经验,R是用于自学的最佳语言,并且对于财务分析和图形处理非常灵活。
In this article, we are going to create six trading strategies and backtest its results using R and its powerful libraries.
在本文中,我们将创建六种交易策略,并使用R及其强大的库对其结果进行回测。
此过程涉及的步骤 (Steps Involved in this process)
1. Importing required libraries
1.导入所需的库
2. Extracting stock data (Apple, Tesla, Netflix) from Yahoo and Basic Plotting
2.从Yahoo和Basic Plotting提取股票数据(Apple,Tesla,Netflix)
3. Creating technical indicators
3.创建技术指标
4. Creating trading signals
4.创建交易信号
5. Creating trading strategies
5.制定交易策略
6. Backtesting and Comparing the results
6.回测和比较结果
步骤1:导入所需的库 (Step-1 : Importing required libraries)
Firstly, to perform our process of creating trading strategies, we have to import the required libraries to our environment. Throughout this process, we will be using some of the most popular finance libraries in R namely Quantmod, TTR and Performance Analytics. Using the library function in R, we can import our required packages. Let’s do it!
首先,要执行创建交易策略的过程,我们必须将所需的库导入到我们的环境中。 在整个过程中,我们将使用R中一些最受欢迎的财务库,即Quantmod,TTR和Performance Analytics。 使用R中的库函数,我们可以导入所需的包。 我们开始做吧!
Yes! We successfully completed our first step!
是! 我们成功地完成了第一步!
步骤2:从Yahoo和基本绘图中提取数据 (Step-2 : Extracting Data from Yahoo and Basic Plotting)
Throughout our process, we will be working with stock price data of Apple, Tesla and Netflix. Let’s extract the data of these companies from Yahoo in R.
在整个过程中,我们将使用Apple,Tesla和Netflix的股价数据。 让我们从R中的Yahoo中提取这些公司的数据。
Now let’s do some visualization of our extracted data! The following code produces a financial bar chart of stock prices along with volume.
现在,让我们对提取的数据进行一些可视化! 以下代码生成股票价格和数量的财务条形图。
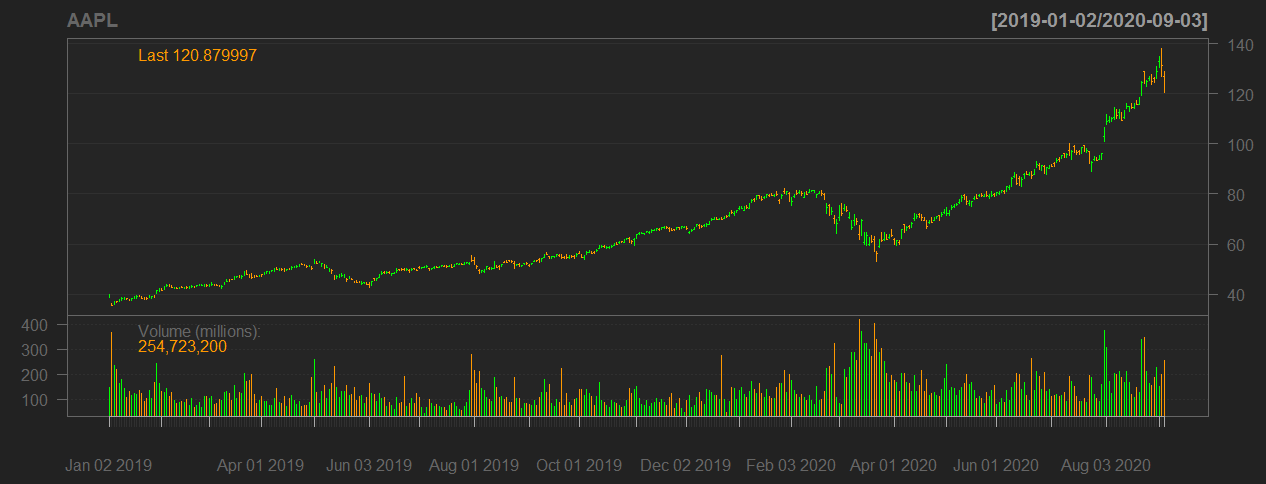
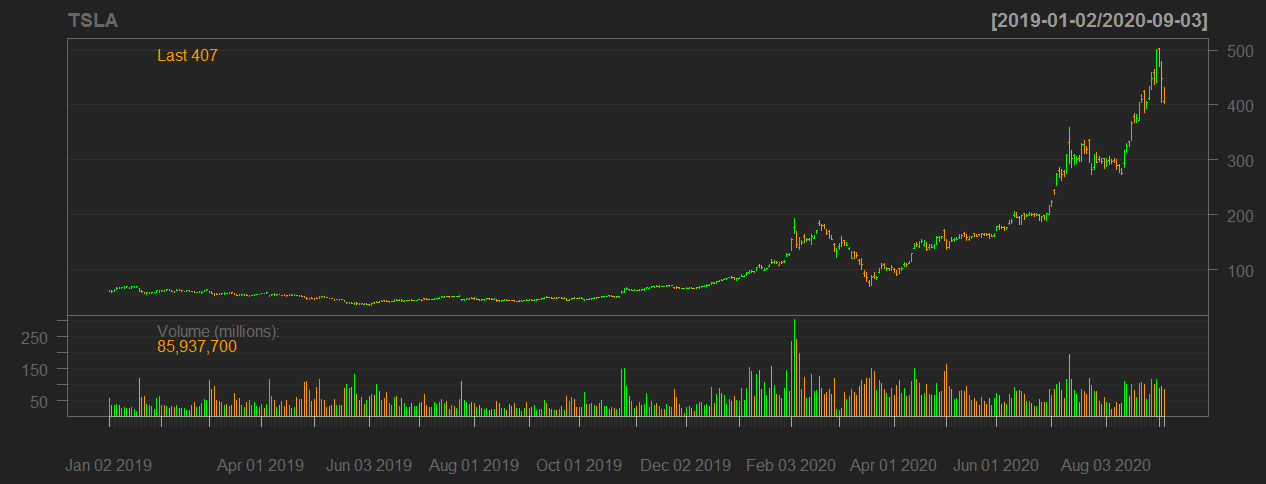

步骤3:创建技术指标 (Step-3: Creating Technical Indicators)
There are many technical indicators used for financial analysis but, for our analysis we are going to use six of the most famous technical indicators namely : Simple Moving Average (SMA), Parabolic Stop And Reverse (SAR), Commodity Channel Index (CCI), Rate Of Change (ROC), Stochastic Momentum Index (SMI) and finally Williams %R
有许多技术指标可用于财务分析,但在我们的分析中,我们将使用六种最著名的技术指标:简单移动平均线(SMA),抛物线止损和反向(SAR),商品通道指数(CCI),变化率(ROC),随机动量指数(SMI),最后是威廉姆斯%R
Simple Moving Average (SMA) :
简单移动平均线(SMA):
The standard interval of time we are going to use is 20 days SMA and 50 days SMA. But, there no restrictions to use any interval of time.
我们将使用的标准时间间隔是20天SMA和50天SMA。 但是,使用任何时间间隔都没有限制。
The following code will calculate three companies’ 20 days SMA and 50 days SMA along with a plot:
以下代码将计算三个公司的20天SMA和50天SMA以及图表:
# 1. AAPL
sma20_aapl <- SMA(AAPL$AAPL.Close, n = 20)
sma50_aapl <- SMA(AAPL$AAPL.Close, n = 50)
lineChart(AAPL, theme = chartTheme('black'))
addSMA(n = 20, col = 'blue')
addSMA(n = 50, col = 'orange')
legend('left', col = c('green','blue','orange'),legend = c('AAPL','SMA20','SMA50'), lty = 1, bty = 'n',text.col = 'white', cex = 0.8)# 2. TSLA
sma20_tsla <- SMA(TSLA$TSLA.Close, n = 20)
sma50_tsla <- SMA(TSLA$TSLA.Close, n = 50)
lineChart(TSLA, theme = 'black')
addSMA(n = 20, col = 'blue')
addSMA(n = 50, col = 'orange')
legend('left', col = c('green','blue','orange'),legend = c('AAPL','SMA20','SMA50'), lty = 1, bty = 'n',text.col = 'white', cex = 0.8)# 3. NFLX
sma20_nflx <- SMA(NFLX$NFLX.Close, n = 20)
sma50_nflx <- SMA(NFLX$NFLX.Close, n = 50)
lineChart(NFLX, theme = 'black')
addSMA(n = 20, col = 'blue')
addSMA(n = 50, col = 'orange')
legend('left', col = c('green','blue','orange'),legend = c('AAPL','SMA20','SMA50'), lty = 1, bty = 'n',text.col = 'white', cex = 0.8)
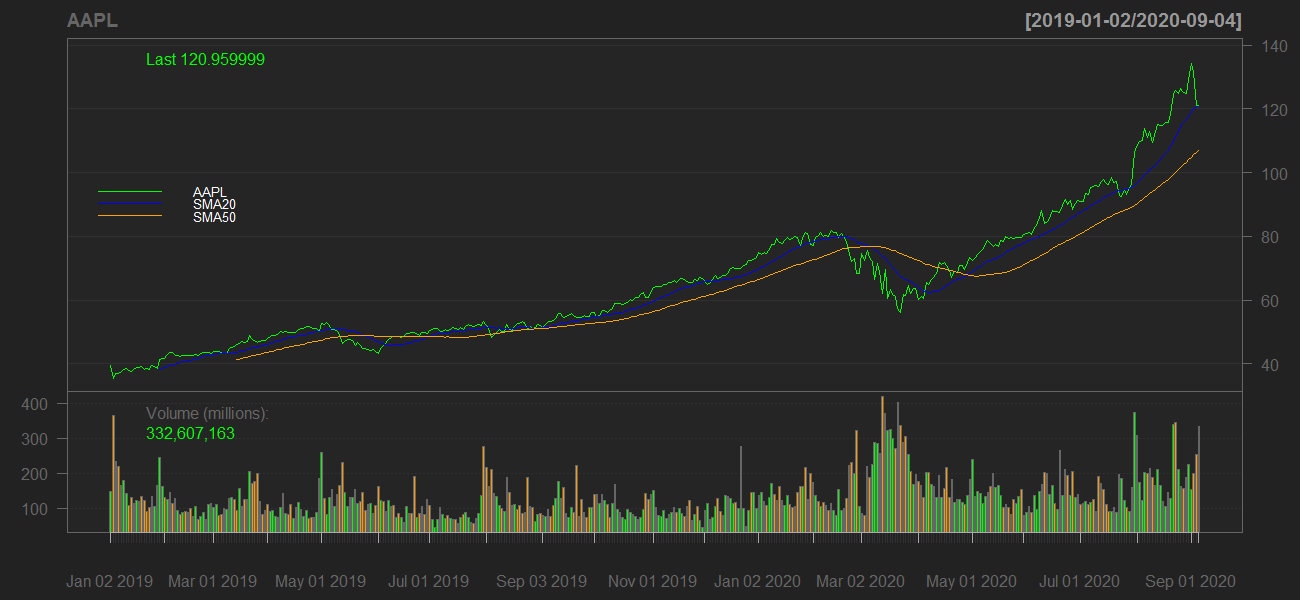

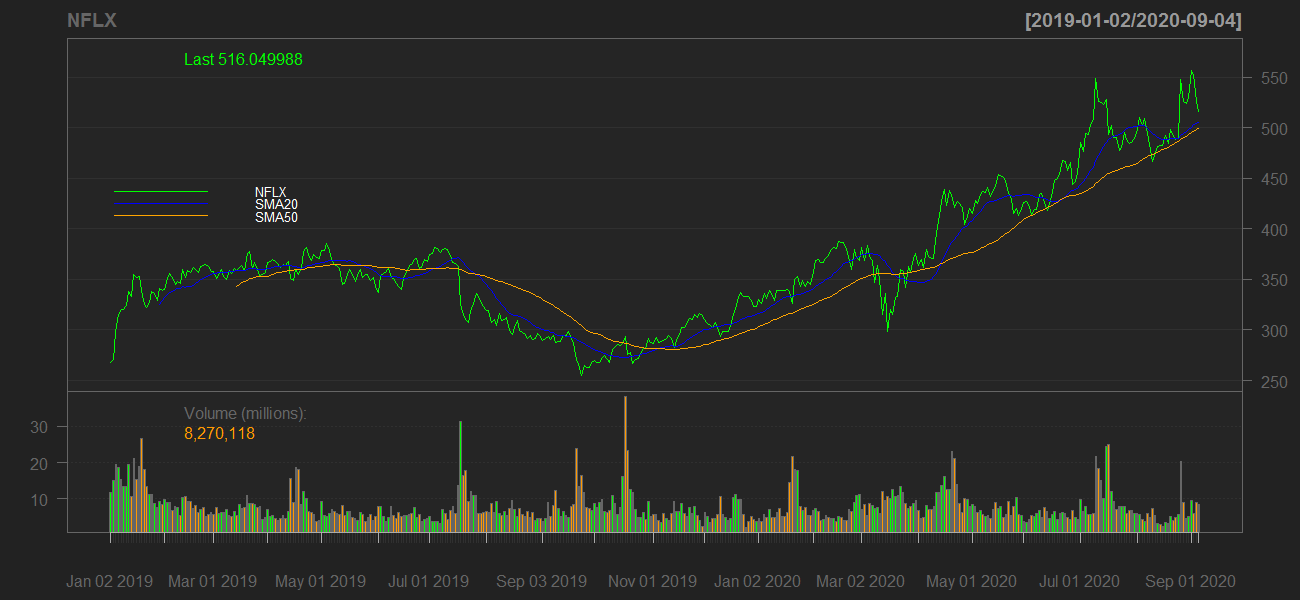
Parabolic Stop And Reverse (SAR) :
抛物线反转(SAR):
To calculate Parabolic SAR, we have to pass on daily High and Low prices of the companies along with a given acceleration value.
要计算抛物线比吸收率,我们必须传递公司的每日最高价和最低价以及给定的加速值。
The following code will calculate the companies’ Parabolic SAR along with a plot:
以下代码将计算公司的抛物线比吸收率以及图:
# 1. AAPL
sar_aapl <- SAR(cbind(Hi(AAPL),Lo(AAPL)), accel = c(0.02, 0.2))
barChart(AAPL, theme = 'black')
addSAR(accel = c(0.02, 0.2), col = 'lightblue')# 2. TSLA
sar_tsla <- SAR(cbind(Hi(TSLA),Lo(TSLA)), accel = c(0.02, 0.2))
barChart(TSLA, theme = 'black')
addSAR(accel = c(0.02, 0.2), col = 'lightblue')# 3. NFLX
sar_nflx <- SAR(cbind(Hi(NFLX),Lo(NFLX)), accel = c(0.02, 0.2))
barChart(NFLX, theme = 'black')
addSAR(accel = c(0.02, 0.2), col = 'lightblue')
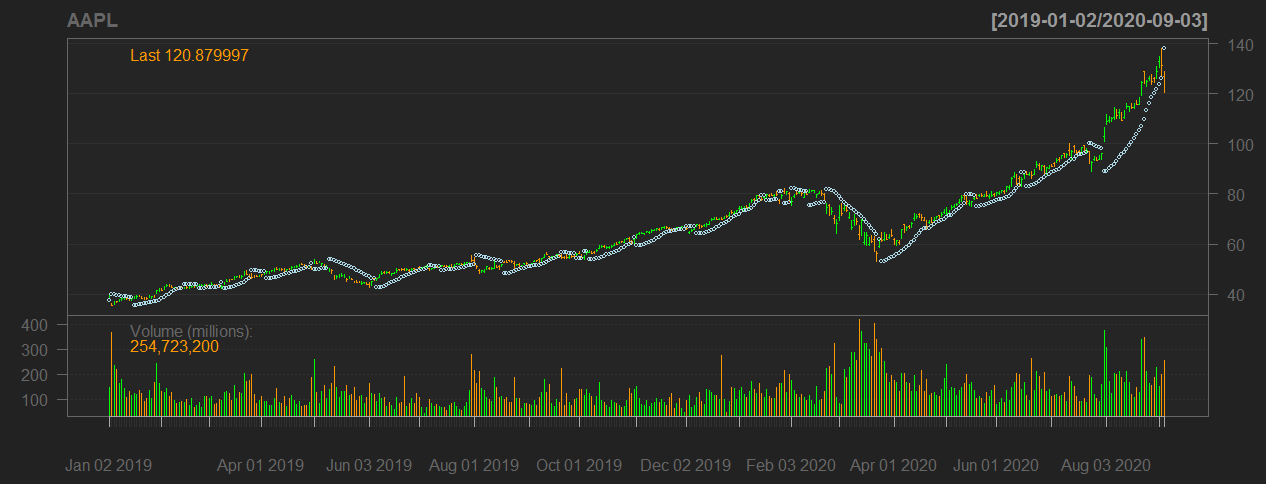


Commodity Channel Index (CCI) :
商品渠道指数(CCI):
To calculate CCI, we have to pass on daily High, Low and Close prices of companies along with a specified time period and a constant value. In this step, we are going to take 20 days as time period and 0.015 as the constant value.
要计算CCI,我们必须传递公司的每日最高价,最低价和收盘价以及指定的时间段和恒定值。 在此步骤中,我们将时间段设为20天,将常数值设为0.015。
The following code will calculate the companies’ CCI along with a plot:
以下代码将计算公司的CCI以及图:
# 1. AAPL
cci_aapl <- CCI(HLC(AAPL), n = 20, c = 0.015)
barChart(AAPL, theme = 'black')
addCCI(n = 20, c = 0.015)# 2. TSLA
cci_tsla <- CCI(HLC(TSLA), n = 20, c = 0.015)
barChart(TSLA, theme = 'black')
addCCI(n = 20, c = 0.015)# 3. NFLX
cci_nflx <- CCI(HLC(NFLX), n = 20, c = 0.015)
barChart(NFLX, theme = 'black')
addCCI(n = 20, c = 0.015)

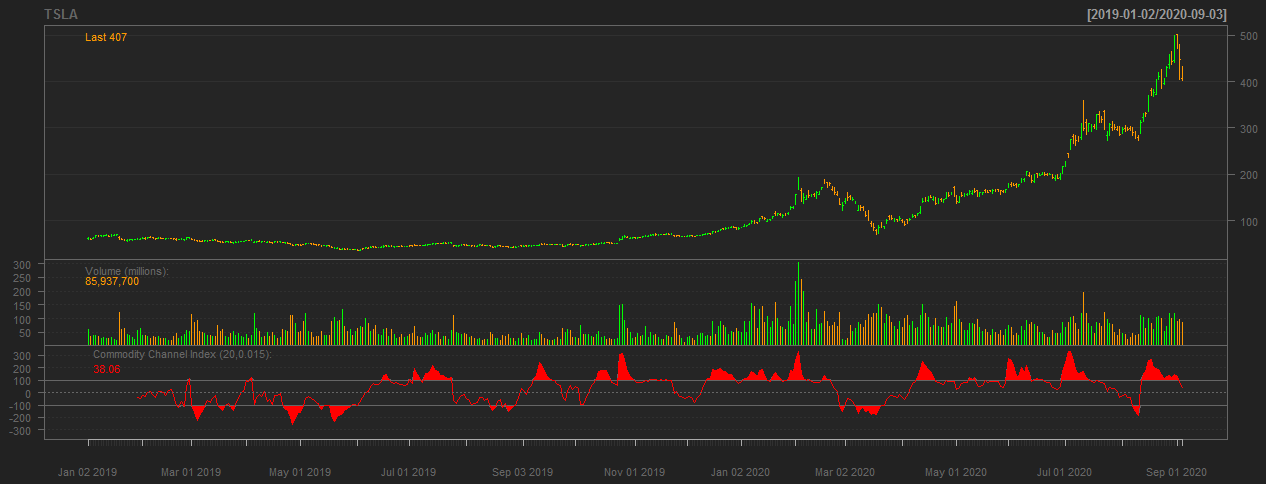
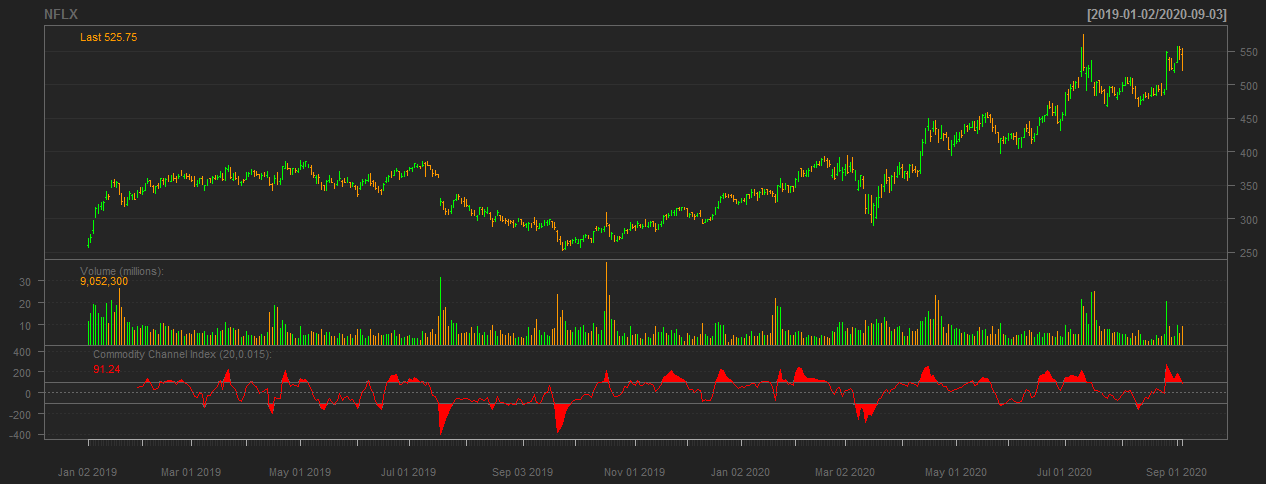
Rate Of Change (ROC)
变化率(ROC)
To calculate ROC, we have to pass on a specified interval of time and there is no restrictions in using any number of period. In this step, we are going to use 25 days as the period of time.
要计算ROC,我们必须经过指定的时间间隔,并且使用任何数量的周期都没有限制。 在此步骤中,我们将使用25天作为时间段。
The following code will calculate the companies’ ROC along with a plot:
以下代码将计算公司的ROC以及图表:
# 1. AAPL
roc_aapl <- ROC(AAPL$AAPL.Close, n = 25)
barChart(AAPL, theme = 'black')
addROC(n = 25)
legend('left', col = 'red', legend = 'ROC(25)', lty = 1, bty = 'n',text.col = 'white', cex = 0.8)# 1. TSLA
roc_tsla <- ROC(TSLA$TSLA.Close, n = 25)
barChart(TSLA, theme = 'black')
addROC(n = 25)
legend('left', col = 'red', legend = 'ROC(25)', lty = 1, bty = 'n',text.col = 'white', cex = 0.8)# 1. NFLX
roc_nflx <- ROC(NFLX$NFLX.Close, n = 25)
barChart(NFLX, theme = 'black')
addROC(n = 25)
legend('right', col = 'red', legend = 'ROC(25)', lty = 1, bty = 'n',text.col = 'white', cex = 0.8)
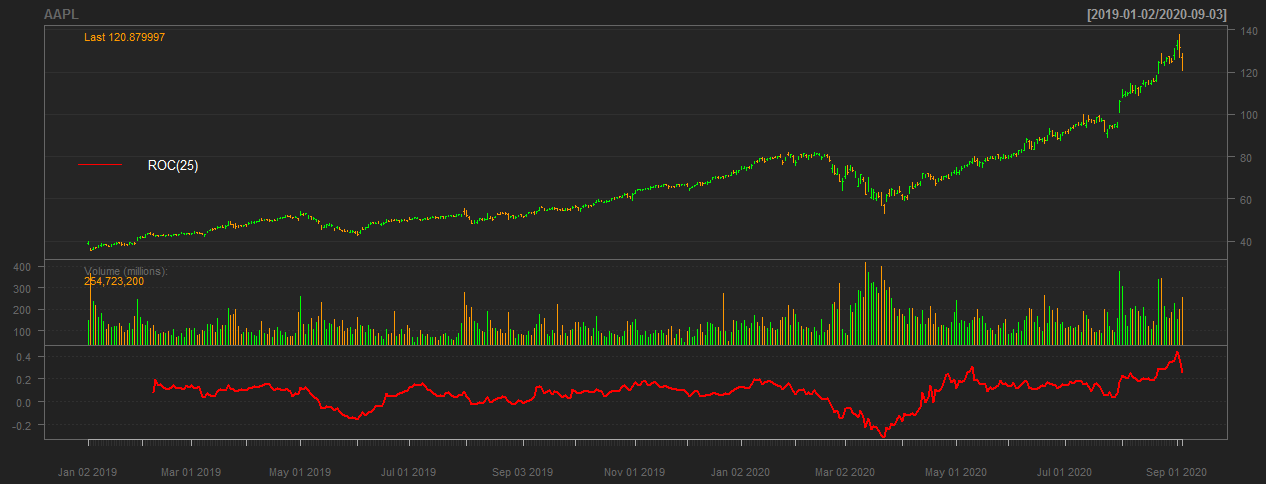
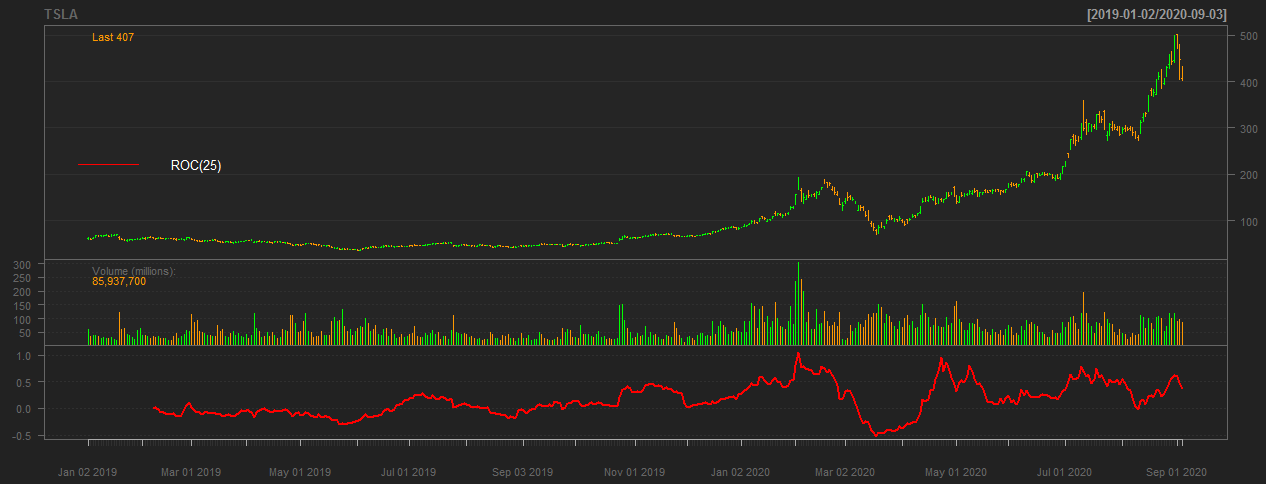
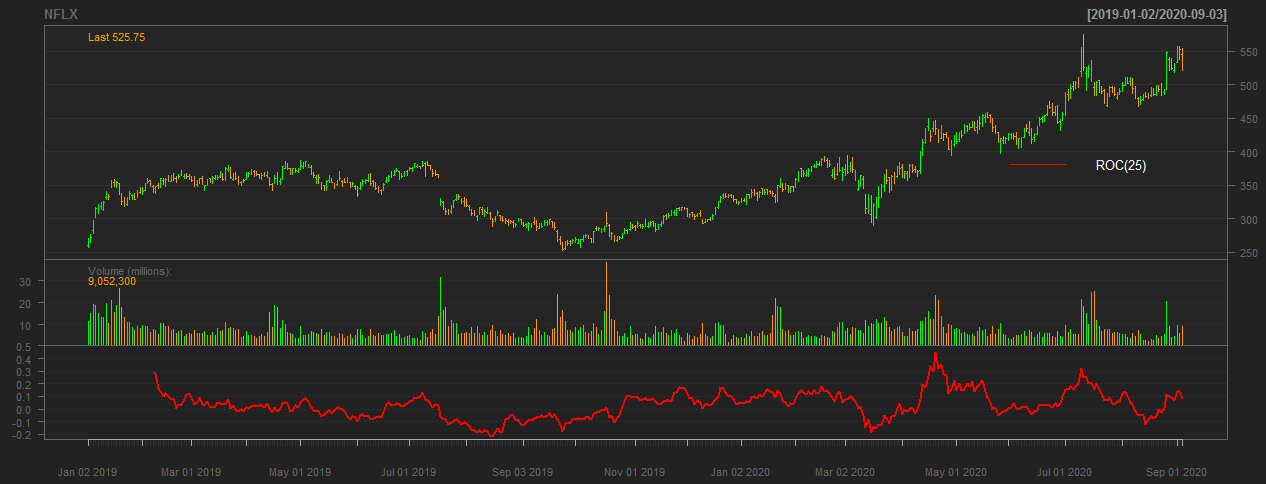
Stochastic Momentum Index (SMI) :
随机动量指数(SMI):
To calculate SMI, we have to pass on daily High, Low and Close prices of companies, a specified interval of time, two smoothing parameters and a signal value.
要计算SMI,我们必须传递公司的每日最高价,最低价和收盘价,指定的时间间隔,两个平滑参数和一个信号值。
The following code will calculate companies’ SMI along with a plot:
以下代码将计算公司的SMI以及图表:
# 1. AAPL
smi_aapl <- SMI(HLC(AAPL),n = 13, nFast = 2, nSlow = 25, nSig = 9)
barChart(AAPL, theme = 'black')
addSMI(n = 13, fast = 2, slow = 2, signal = 9)# 2. TSLA
smi_tsla <- SMI(HLC(TSLA),n = 13, nFast = 2, nSlow = 25, nSig = 9)
barChart(TSLA, theme = 'black')
addSMI(n = 13, fast = 2, slow = 2, signal = 9)# 3. NFLX
smi_nflx <- SMI(HLC(NFLX),n = 13, nFast = 2, nSlow = 25, nSig = 9)
barChart(NFLX, theme = 'black')
addSMI(n = 13, fast = 2, slow = 2, signal = 9)

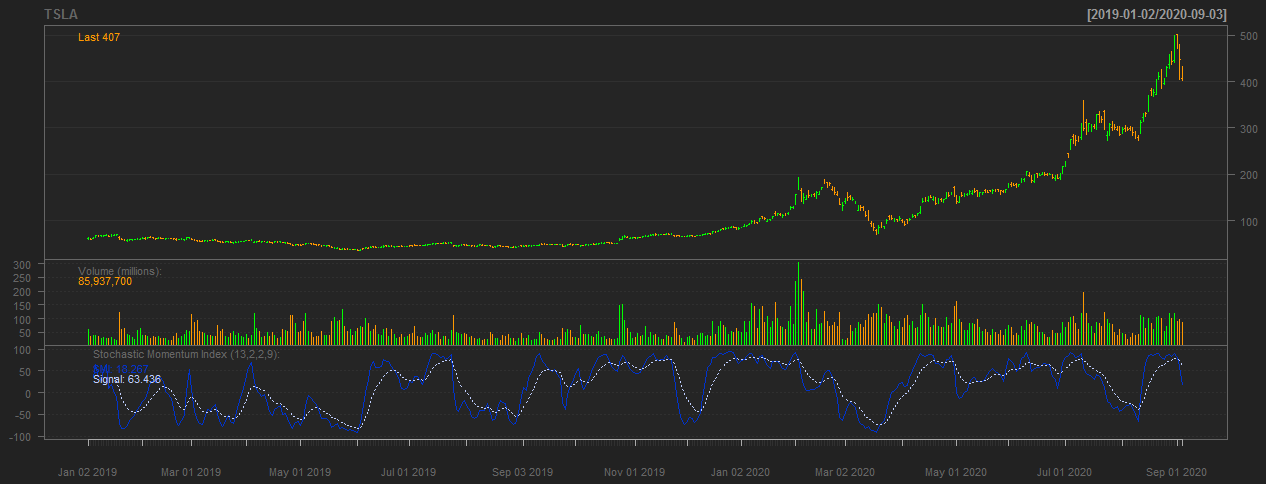

Williams %R
威廉姆斯%R
To calculate Williams %R, we have to pass on the daily High, Low and Close prices of companies along with a specified number of periods.
要计算Williams%R,我们必须传递公司的每日最高价,最低价和收盘价以及指定的期间数。
The following code will calculate the companies’ Williams %R along with a plot:
以下代码将计算公司的Williams%R以及图表:
# 1. AAPL
wpr_aapl <- WPR(HLC(AAPL), n = 14)
colnames(wpr_aapl) <- 'wpr'
barChart(AAPL, theme = 'black')
addWPR(n = 14)# 1. TSLA
wpr_tsla <- WPR(HLC(TSLA), n = 14)
colnames(wpr_tsla) <- 'wpr'
barChart(TSLA, theme = 'black')
addWPR(n = 14)# 1. NFLX
wpr_nflx <- WPR(HLC(NFLX), n = 14)
colnames(wpr_nflx) <- 'wpr'
barChart(NFLX, theme = 'black')
addWPR(n = 14)



步骤4:创建交易信号 (Step-4 : Creating Trading Signals)
In this step, we are going to use the previously created indicators to build ‘BUY’ or ‘SELL’ trading signals. Basically, we will pass on specific conditions and if the condition satisfies a ‘BUY’ signal our created trading signal will turn to 1 which means a buy. If the condition satisfies a ‘SELL’ signal our created trading signal will turn to -1 which means a sell. If it doesn’t satisfies any of the mentioned conditions, then it will turn to 0 which means nothing. It might sounds like a whole bunch of fuzz but, it will be clear once we dive into the coding section. After coding your trading signals, you can use the ‘which’ command in R to see how may ‘BUY’ signals and how many ‘SELL’ signals.
在这一步中,我们将使用先前创建的指标来建立“买入”或“卖出”交易信号。 基本上,我们将传递特定的条件,如果条件满足“买入”信号,我们创建的交易信号将变为1,表示买入。 如果条件满足“卖出”信号,我们创建的交易信号将变为-1,这意味着卖出。 如果不满足上述任何条件,则它将变为0,这意味着没有任何意义。 听起来似乎很模糊,但是一旦我们深入到编码部分,这一点就很清楚了。 在对交易信号进行编码之后,您可以使用R中的“哪个”命令来查看“买入”信号的数量和“卖出”信号的数量。
Simple Moving Average
简单移动平均线
The following code will create SMA trading signals for companies if it satisfies our given condition:
如果满足我们的条件,以下代码将为公司创建SMA交易信号:
# SMA # a. AAPL
# SMA 20 Crossover Signal
sma20_aapl_ts <- Lag(ifelse(Lag(Cl(AAPL)) < Lag(sma20_aapl) & Cl(AAPL) > sma20_aapl,1,ifelse(Lag(Cl(AAPL)) > Lag(sma20_aapl) & Cl(AAPL) < sma20_aapl,-1,0)))
sma20_aapl_ts[is.na(sma20_aapl_ts)] <- 0
# SMA 50 Crossover Signal
sma50_aapl_ts <- Lag(ifelse(Lag(Cl(AAPL)) < Lag(sma50_aapl) & Cl(AAPL) > sma50_aapl,1,ifelse(Lag(Cl(AAPL)) > Lag(sma50_aapl) & Cl(AAPL) < sma50_aapl,-1,0)))
sma50_aapl_ts[is.na(sma50_aapl_ts)] <- 0
# SMA 20 and SMA 50 Crossover Signal
sma_aapl_ts <- Lag(ifelse(Lag(sma20_aapl) < Lag(sma50_aapl) & sma20_aapl > sma50_aapl,1,ifelse(Lag(sma20_aapl) > Lag(sma50_aapl) & sma20_aapl < sma50_aapl,-1,0)))
sma_aapl_ts[is.na(sma_aapl_ts)] <- 0# b. TSLA
# SMA 20 Crossover Signal
sma20_tsla_ts <- Lag(ifelse(Lag(Cl(TSLA)) < Lag(sma20_tsla) & Cl(TSLA) > sma20_tsla,1,ifelse(Lag(Cl(TSLA)) > Lag(sma20_tsla) & Cl(TSLA) < sma20_tsla,-1,0)))
sma20_tsla_ts[is.na(sma20_tsla_ts)] <- 0
# SMA 50 Crossover Signal
sma50_tsla_ts <- Lag(ifelse(Lag(Cl(TSLA)) < Lag(sma50_tsla) & Cl(TSLA) > sma50_tsla,1,ifelse(Lag(Cl(TSLA)) > Lag(sma50_tsla) & Cl(TSLA) < sma50_tsla,-1,0)))
sma50_tsla_ts[is.na(sma50_tsla_ts)] <- 0
# SMA 20 and SMA 50 Crossover Signal
sma_tsla_ts <- Lag(ifelse(Lag(sma20_tsla) < Lag(sma50_tsla) & sma20_tsla > sma50_tsla,1,ifelse(Lag(sma20_tsla) > Lag(sma50_tsla) & sma20_tsla < sma50_tsla,-1,0)))
sma_tsla_ts[is.na(sma_tsla_ts)] <- 0# c. NFLX
# SMA 20 Crossover Signal
sma20_nflx_ts <- Lag(ifelse(Lag(Cl(NFLX)) < Lag(sma20_nflx) & Cl(NFLX) > sma20_nflx,1,ifelse(Lag(Cl(NFLX)) > Lag(sma20_nflx) & Cl(NFLX) < sma20_nflx,-1,0)))
sma20_nflx_ts[is.na(sma20_nflx_ts)] <- 0
# SMA 50 Crossover Signal
sma50_nflx_ts <- Lag(ifelse(Lag(Cl(NFLX)) < Lag(sma50_nflx) & Cl(NFLX) > sma50_nflx,1,ifelse(Lag(Cl(NFLX)) > Lag(sma50_nflx) & Cl(NFLX) < sma50_nflx,-1,0)))
sma50_nflx_ts[is.na(sma50_nflx_ts)] <- 0
# SMA 20 and SMA 50 Crossover Signal
sma_nflx_ts <- Lag(ifelse(Lag(sma20_nflx) < Lag(sma50_nflx) & sma20_nflx > sma50_nflx,1,ifelse(Lag(sma20_nflx) > Lag(sma50_nflx) & sma20_nflx < sma50_nflx,-1,0)))
sma_nflx_ts[is.na(sma_nflx_ts)] <- 0
Parabolic Stop and Reverse (SAR)
抛物线停止和反向(SAR)
The following code will create Parabolic SAR trading signal for companies if it satisfies our conditions:
如果满足我们的条件,以下代码将为公司创建抛物线SAR交易信号:
# Parabolic Stop And Reverse (SAR) # a. AAPL
sar_aapl_ts <- Lag(ifelse(Lag(Cl(AAPL)) < Lag(sar_aapl) & Cl(AAPL) > sar_aapl,1,ifelse(Lag(Cl(AAPL)) > Lag(sar_aapl) & Cl(AAPL) < sar_aapl,-1,0)))
sar_aapl_ts[is.na(sar_aapl_ts)] <- 0# b. TSLA
sar_tsla_ts <- Lag(ifelse(Lag(Cl(TSLA)) < Lag(sar_tsla) & Cl(TSLA) > sar_tsla,1,ifelse(Lag(Cl(TSLA)) > Lag(sar_tsla) & Cl(TSLA) < sar_tsla,-1,0)))
sar_tsla_ts[is.na(sar_tsla_ts)] <- 0# c. NFLX
sar_nflx_ts <- Lag(ifelse(Lag(Cl(NFLX)) < Lag(sar_nflx) & Cl(NFLX) > sar_nflx,1,ifelse(Lag(Cl(NFLX)) > Lag(sar_nflx) & Cl(NFLX) < sar_nflx,-1,0)))
sar_nflx_ts[is.na(sar_nflx_ts)] <- 0
Commodity Channel Index (CCI)
商品渠道指数(CCI)
The following code will create CCI trading signals for companies if it satisfies our conditions:
如果满足我们的条件,以下代码将为公司创建CCI交易信号:
# Commodity Channel Index (CCI)# a. AAPL
cci_aapl_ts <- Lag(ifelse(Lag(cci_aapl) < (-100) & cci_aapl > (-100),1,ifelse(Lag(cci_aapl) < (100) & cci_aapl > (100),-1,0)))
cci_aapl_ts[is.na(cci_aapl_ts)] <- 0# b. TSLA
cci_tsla_ts <- Lag(ifelse(Lag(cci_tsla) < (-100) & cci_tsla > (-100),1,ifelse(Lag(cci_tsla) < (100) & cci_tsla > (100),-1,0)))
cci_tsla_ts[is.na(cci_tsla_ts)] <- 0# c. NFLX
cci_nflx_ts <- Lag(ifelse(Lag(cci_nflx) < (-100) & cci_nflx > (-100),1,ifelse(Lag(cci_nflx) < (100) & cci_nflx > (100),-1,0)))
cci_nflx_ts[is.na(cci_nflx_ts)] <- 0
Rate of Change (ROC)
变化率(ROC)
The following code will create Rate Of Change (ROC) trading signals for companies if it satisfies our conditions:
如果满足我们的条件,以下代码将为公司创建更改率(ROC)交易信号:
# Rate of Change (ROC)# a. AAPL
roc_aapl_ts <- Lag(ifelse(Lag(roc_aapl) < (-0.05) & roc_aapl > (-0.05),1,ifelse(Lag(roc_aapl) < (0.05) & roc_aapl > (0.05),-1,0)))
roc_aapl_ts[is.na(roc_aapl_ts)] <- 0# b. TSLA
roc_tsla_ts <- Lag(ifelse(Lag(roc_tsla) < (-0.05) & roc_tsla > (-0.05),1,ifelse(Lag(roc_tsla) < (0.05) & roc_tsla > (0.05),-1,0)))
roc_tsla_ts[is.na(roc_tsla_ts)] <- 0# c. NFLX
roc_nflx_ts <- Lag(ifelse(Lag(roc_nflx) < (-0.05) & roc_nflx > (-0.05),1,ifelse(Lag(roc_nflx) < (0.05) & roc_nflx > (0.05),-1,0)))
roc_nflx_ts[is.na(roc_nflx_ts)] <- 0
Stochastic Momentum Index (SMI)
随机动量指数(SMI)
The following code will create Stochastic Momentum Index (SMI) trading signals for companies if it satisfies our conditions:
如果满足我们的条件,以下代码将为公司创建随机动量指数(SMI)交易信号:
# Stochastic Momentum Index (SMI)# a. AAPL
smi_aapl_ts <- Lag(ifelse(Lag(smi_aapl[,1]) < Lag(smi_aapl[,2]) & smi_aapl[,1] > smi_aapl[,2],1, ifelse(Lag(smi_aapl[,1]) > Lag(smi_aapl[,2]) & smi_aapl[,1] < smi_aapl[,2],-1,0)))
smi_aapl_ts[is.na(smi_aapl_ts)] <- 0# b. TSLA
smi_tsla_ts <- Lag(ifelse(Lag(smi_tsla[,1]) < Lag(smi_tsla[,2]) & smi_tsla[,1] > smi_tsla[,2],1, ifelse(Lag(smi_tsla[,1]) > Lag(smi_tsla[,2]) & smi_tsla[,1] < smi_tsla[,2],-1,0)))
smi_tsla_ts[is.na(smi_tsla_ts)] <- 0# a. NFLX
smi_nflx_ts <- Lag(ifelse(Lag(smi_nflx[,1]) < Lag(smi_nflx[,2]) & smi_nflx[,1] > smi_nflx[,2],1, ifelse(Lag(smi_nflx[,1]) > Lag(smi_nflx[,2]) & smi_nflx[,1] < smi_nflx[,2],-1,0)))
smi_nflx_ts[is.na(smi_nflx_ts)] <- 0
Williams %R
威廉姆斯%R
The following code will create Williams %R trading signals for companies if it satisfies our conditions:
如果满足我们的条件,以下代码将为公司创建Williams%R交易信号:
# Williams %R# a. AAPL
wpr_aapl_ts <- Lag(ifelse(Lag(wpr_aapl) > 0.8 & wpr_aapl < 0.8,1,ifelse(Lag(wpr_aapl) > 0.2 & wpr_aapl < 0.2,-1,0)))
wpr_aapl_ts[is.na(wpr_aapl_ts)] <- 0# b. TSLA
wpr_tsla_ts <- Lag(ifelse(Lag(wpr_tsla) > 0.8 & wpr_tsla < 0.8,1,ifelse(Lag(wpr_tsla) > 0.2 & wpr_tsla < 0.2,-1,0)))
wpr_tsla_ts[is.na(wpr_tsla_ts)] <- 0# c. NFLX
wpr_nflx_ts <- Lag(ifelse(Lag(wpr_nflx) > 0.8 & wpr_nflx < 0.8,1,ifelse(Lag(wpr_nflx) > 0.2 & wpr_nflx < 0.2,-1,0)))
wpr_nflx_ts[is.na(wpr_nflx_ts)] <- 0
步骤5:制定交易策略 (Step-5 : Creating Trading Strategies)
This is the most interesting step in our process as we are going to create Trading Strategies using our previously created Trading Signals. In our previous step, we created signals whether to buy or sell and in this step we are going to create conditions to check our holding position in that stock (i.e., whether we hold, bought or sold the stock). The trading strategy we are about to code will return 1 if we hold the stock or returns 0 if we don’t own the stock.
这是我们流程中最有趣的步骤,因为我们将使用先前创建的交易信号来创建交易策略。 在上一步中,我们创建了买入或卖出信号,在这一步中,我们将创建条件来检查我们在该股票中的持仓状况(即我们是否持有,买入或卖出该股票)。 如果我们持有股票,我们将要编码的交易策略将返回1;如果我们不拥有股票,则将返回0。
Simple Moving Average
简单移动平均线
The following code will create a SMA trading strategy if satisfies our given conditions:
如果满足我们的条件,以下代码将创建SMA交易策略:
# SMA 20 and SMA 50 Crossover Strategy# a. AAPLsma_aapl_strat <- ifelse(sma_aapl_ts > 1,0,1)
for (i in 1 : length(Cl(AAPL))) {sma_aapl_strat[i] <- ifelse(sma_aapl_ts[i] == 1,1,ifelse(sma_aapl_ts[i] == -1,0,sma_aapl_strat[i-1]))
}
sma_aapl_strat[is.na(sma_aapl_strat)] <- 1
sma_aapl_stratcomp <- cbind(sma20_aapl, sma50_aapl, sma_aapl_ts, sma_aapl_strat)
colnames(sma_aapl_stratcomp) <- c('SMA(20)','SMA(50)','SMA SIGNAL','SMA POSITION')# b. TSLA
sma_tsla_strat <- ifelse(sma_tsla_ts > 1,0,1)
for (i in 1 : length(Cl(TSLA))) {sma_tsla_strat[i] <- ifelse(sma_tsla_ts[i] == 1,1,ifelse(sma_tsla_ts[i] == -1,0,sma_tsla_strat[i-1]))
}
sma_tsla_strat[is.na(sma_tsla_strat)] <- 1
sma_tsla_stratcomp <- cbind(sma20_tsla, sma50_tsla, sma_tsla_ts, sma_tsla_strat)
colnames(sma_tsla_stratcomp) <- c('SMA(20)','SMA(50)','SMA SIGNAL','SMA POSITION')# c. NFLX
sma_nflx_strat <- ifelse(sma_nflx_ts > 1,0,1)
for (i in 1 : length(Cl(NFLX))) {sma_nflx_strat[i] <- ifelse(sma_nflx_ts[i] == 1,1,ifelse(sma_nflx_ts[i] == 'SEL',0,sma_nflx_strat[i-1]))
}
sma_nflx_strat[is.na(sma_nflx_strat)] <- 1
sma_nflx_stratcomp <- cbind(sma20_nflx, sma50_nflx, sma_nflx_ts, sma_nflx_strat)
colnames(sma_nflx_stratcomp) <- c('SMA(20)','SMA(50)','SMA SIGNAL','SMA POSITION')
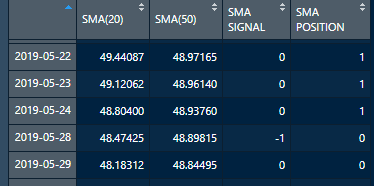
This is an example table of our created SMA Apple trading strategy. In this table we can observe that, on 2019–05–24 SMA 20 went below SMA 50 so our created signal returns -1 (‘SELL’) the next day also our position changes to 0 which means we don’t hold the stock. From this, we can say that our created strategy works as we expected. Now, let’s proceed to the remaining Trading Strategies.
这是我们创建的SMA Apple交易策略的示例表。 在此表中,我们可以观察到,在2019–05–24 SMA 20低于SMA 50,因此我们创建的信号在第二天返回-1(“卖出”),我们的头寸也变为0,这意味着我们不持有股票。 由此,我们可以说我们创建的策略可以按预期工作。 现在,让我们继续其余的交易策略。
Parabolic Stop And Reverse (SAR)
抛物线停止和反转(SAR)
The following code will create a Parabolic SAR trading strategy if satisfies our given conditions:
如果满足我们的条件,以下代码将创建抛物线形SAR交易策略:
# Parabolic SAR Strategy # a. AAPL
sar_aapl_strat <- ifelse(sar_aapl_ts > 1,0,1)
for (i in 1 : length(Cl(AAPL))) {sar_aapl_strat[i] <- ifelse(sar_aapl_ts[i] == 1,1,ifelse(sar_aapl_ts[i] == -1,0,sar_aapl_strat[i-1]))
}
sar_aapl_strat[is.na(sar_aapl_strat)] <- 1
sar_aapl_stratcomp <- cbind(Cl(AAPL), sar_aapl, sar_aapl_ts, sar_aapl_strat)
colnames(sar_aapl_stratcomp) <- c('Close','SAR','SAR SIGNAL','SAR POSITION')# b. TSLA
sar_tsla_strat <- ifelse(sar_tsla_ts > 1,0,1)
for (i in 1 : length(Cl(TSLA))) {sar_tsla_strat[i] <- ifelse(sar_tsla_ts[i] == 1,1,ifelse(sar_tsla_ts[i] == -1,0,sar_tsla_strat[i-1]))
}
sar_tsla_strat[is.na(sar_tsla_strat)] <- 1
sar_tsla_stratcomp <- cbind(Cl(TSLA), sar_tsla, sar_tsla_ts, sar_tsla_strat)
colnames(sar_tsla_stratcomp) <- c('Close','SAR','SAR SIGNAL','SAR POSITION')# c. NFLX
sar_nflx_strat <- ifelse(sar_nflx_ts > 1,0,1)
for (i in 1 : length(Cl(NFLX))) {sar_nflx_strat[i] <- ifelse(sar_nflx_ts[i] == 1,1,ifelse(sar_nflx_ts[i] == -1,0,sar_nflx_strat[i-1]))
}
sar_nflx_strat[is.na(sar_nflx_strat)] <- 1
sar_nflx_stratcomp <- cbind(Cl(NFLX), sar_nflx, sar_nflx_ts, sar_nflx_strat)
colnames(sar_nflx_stratcomp) <- c('Close','SAR','SAR SIGNAL','SAR POSITION')
Commodity Channel Index (CCI) :
商品渠道指数(CCI):
The following code will create a CCI trading strategy if satisfies our given conditions:
如果满足我们的条件,以下代码将创建CCI交易策略:
# CCI# a. AAPL
cci_aapl_strat <- ifelse(cci_aapl_ts > 1,0,1)
for (i in 1 : length(Cl(AAPL))) {cci_aapl_strat[i] <- ifelse(cci_aapl_ts[i] == 1,1,ifelse(cci_aapl_ts[i] == -1,0,cci_aapl_strat[i-1]))
}
cci_aapl_strat[is.na(cci_aapl_strat)] <- 1
cci_aapl_stratcomp <- cbind(cci_aapl, cci_aapl_ts, cci_aapl_strat)
colnames(cci_aapl_stratcomp) <- c('CCI','CCI SIGNAL','CCI POSITION')# b. TSLA
cci_tsla_strat <- ifelse(cci_tsla_ts > 1,0,1)
for (i in 1 : length(Cl(TSLA))) {cci_tsla_strat[i] <- ifelse(cci_tsla_ts[i] == 1,1,ifelse(cci_tsla_ts[i] == -1,0,cci_tsla_strat[i-1]))
}
cci_tsla_strat[is.na(cci_tsla_strat)] <- 1
cci_tsla_stratcomp <- cbind(cci_tsla, cci_tsla_ts, cci_tsla_strat)
colnames(cci_tsla_stratcomp) <- c('CCI','CCI SIGNAL','CCI POSITION')# c. NFLX
cci_nflx_strat <- ifelse(cci_nflx_ts > 1,0,1)
for (i in 1 : length(Cl(NFLX))) {cci_nflx_strat[i] <- ifelse(cci_nflx_ts[i] == 1,1,ifelse(cci_nflx_ts[i] == -1,0,cci_nflx_strat[i-1]))
}
cci_nflx_strat[is.na(cci_nflx_strat)] <- 1
cci_nflx_stratcomp <- cbind(cci_nflx, cci_nflx_ts, cci_nflx_strat)
colnames(cci_nflx_stratcomp) <- c('CCI','CCI SIGNAL','CCI POSITION')
Rate Of Change (ROC)
变化率(ROC)
The following code will create a Rate Of Change (ROC) trading strategy if satisfies our given conditions:
如果满足我们的给定条件,以下代码将创建一个变化率(ROC)交易策略:
# ROC# a. AAPL
roc_aapl_strat <- ifelse(roc_aapl_ts > 1,0,1)
for (i in 1 : length(Cl(AAPL))) {roc_aapl_strat[i] <- ifelse(roc_aapl_ts[i] == 1,1,ifelse(roc_aapl_ts[i] == -1,0,roc_aapl_strat[i-1]))
}
roc_aapl_strat[is.na(roc_aapl_strat)] <- 1
roc_aapl_stratcomp <- cbind(roc_aapl, roc_aapl_ts, roc_aapl_strat)
colnames(roc_aapl_stratcomp) <- c('ROC(25)','ROC SIGNAL','ROC POSITION')# b. TSLA
roc_tsla_strat <- ifelse(roc_tsla_ts > 1,0,1)
for (i in 1 : length(Cl(TSLA))) {roc_tsla_strat[i] <- ifelse(roc_tsla_ts[i] == 1,1,ifelse(roc_tsla_ts[i] == -1,0,roc_tsla_strat[i-1]))
}
roc_tsla_strat[is.na(roc_tsla_strat)] <- 1
roc_tsla_stratcomp <- cbind(roc_tsla, roc_tsla_ts, roc_tsla_strat)
colnames(roc_tsla_stratcomp) <- c('ROC(25)','ROC SIGNAL','ROC POSITION')# c. NFLX
roc_nflx_strat <- ifelse(roc_nflx_ts > 1,0,1)
for (i in 1 : length(Cl(NFLX))) {roc_nflx_strat[i] <- ifelse(roc_nflx_ts[i] == 1,1,ifelse(roc_nflx_ts[i] == -1,0,roc_nflx_strat[i-1]))
}
roc_nflx_strat[is.na(roc_nflx_strat)] <- 1
roc_nflx_stratcomp <- cbind(roc_nflx, roc_nflx_ts, roc_nflx_strat)
colnames(roc_nflx_stratcomp) <- c('ROC(25)','ROC SIGNAL','ROC POSITION')
Stochastic Momentum Index (SMI)
随机动量指数(SMI)
The following code will create a SMI trading strategy if it satisfies our given conditions:
如果满足我们的条件,以下代码将创建一个SMI交易策略:
# SMI# a. AAPL
smi_aapl_strat <- ifelse(smi_aapl_ts > 1,0,1)
for (i in 1 : length(Cl(AAPL))) {smi_aapl_strat[i] <- ifelse(smi_aapl_ts[i] == 1,1,ifelse(smi_aapl_ts[i] == -1,0,smi_aapl_strat[i-1]))
}
smi_aapl_strat[is.na(smi_aapl_strat)] <- 1
smi_aapl_stratcomp <- cbind(smi_aapl[,1],smi_aapl[,2],smi_aapl_ts,smi_aapl_strat)
colnames(smi_aapl_stratcomp) <- c('SMI','SMI(S)','SMI SIGNAL','SMI POSITION')# b. TSLA
smi_tsla_strat <- ifelse(smi_tsla_ts > 1,0,1)
for (i in 1 : length(Cl(TSLA))) {smi_tsla_strat[i] <- ifelse(smi_tsla_ts[i] == 1,1,ifelse(smi_tsla_ts[i] == -1,0,smi_tsla_strat[i-1]))
}
smi_tsla_strat[is.na(smi_tsla_strat)] <- 1
smi_tsla_stratcomp <- cbind(smi_tsla[,1],smi_tsla[,2],smi_tsla_ts,smi_tsla_strat)
colnames(smi_tsla_stratcomp) <- c('SMI','SMI(S)','SMI SIGNAL','SMI POSITION')# c. NFLX
smi_nflx_strat <- ifelse(smi_nflx_ts > 1,0,1)
for (i in 1 : length(Cl(NFLX))) {smi_nflx_strat[i] <- ifelse(smi_nflx_ts[i] == 1,1,ifelse(smi_nflx_ts[i] == -1,0,smi_nflx_strat[i-1]))
}
smi_nflx_strat[is.na(smi_nflx_strat)] <- 1
smi_nflx_stratcomp <- cbind(smi_nflx[,1],smi_nflx[,2],smi_nflx_ts,smi_nflx_strat)
colnames(smi_nflx_stratcomp) <- c('SMI','SMI(S)','SMI SIGNAL','SMI POSITION')
Williams %R
威廉姆斯%R
The following code will create a Williams %R trading strategy if it satisfies our given conditions:
如果满足我们给定的条件,以下代码将创建Williams%R交易策略:
# WPR# a. AAPL
wpr_aapl_strat <- ifelse(wpr_aapl_ts > 1,0,1)
for (i in 1 : length(Cl(AAPL))) {wpr_aapl_strat[i] <- ifelse(wpr_aapl_ts[i] == 1,1,ifelse(wpr_aapl_ts[i] == -1,0,wpr_aapl_strat[i-1]))
}
wpr_aapl_strat[is.na(wpr_aapl_strat)] <- 1
wpr_aapl_stratcomp <- cbind(wpr_aapl, wpr_aapl_ts, wpr_aapl_strat)
colnames(wpr_aapl_stratcomp) <- c('WPR(14)','WPR SIGNAL','WPR POSITION')# b. TSLA
wpr_tsla_strat <- ifelse(wpr_tsla_ts > 1,0,1)
for (i in 1 : length(Cl(TSLA))) {wpr_tsla_strat[i] <- ifelse(wpr_tsla_ts[i] == 1,1,ifelse(wpr_tsla_ts[i] == -1,0,wpr_tsla_strat[i-1]))
}
wpr_tsla_strat[is.na(wpr_tsla_strat)] <- 1
wpr_tsla_stratcomp <- cbind(wpr_tsla, wpr_tsla_ts, wpr_tsla_strat)
colnames(wpr_tsla_stratcomp) <- c('WPR(14)','WPR SIGNAL','WPR POSITION')# c. NFLX
wpr_nflx_strat <- ifelse(wpr_nflx_ts > 1,0,1)
for (i in 1 : length(Cl(NFLX))) {wpr_nflx_strat[i] <- ifelse(wpr_nflx_ts[i] == 1,1,ifelse(wpr_nflx_ts[i] == -1,0,wpr_nflx_strat[i-1]))
}
wpr_nflx_strat[is.na(wpr_nflx_strat)] <- 1
wpr_nflx_stratcomp <- cbind(wpr_nflx, wpr_nflx_ts, wpr_nflx_strat)
colnames(wpr_nflx_stratcomp) <- c('WPR(14)','WPR SIGNAL','WPR POSITION')
步骤6:回测和比较结果 (Step-6 : Backtesting and Comparing the Results)
In this step we are going to conduct backtests on our created trading strategies vs our created trading strategies commission adjusted (0.5%) vs the companies’ benchmark returns. Before conducting our backtests, we have calculate our daily benchmark returns i.e., daily returns of Apple, Tesla and Netflix. Let’s do it!
在 此步骤中,我们将对我们创建的交易策略与调整后的交易策略佣金(0.5%)与公司的基准收益进行回测。 在进行回测之前,我们已经计算了每日基准回报,即Apple,Tesla和Netflix的每日回报。 我们开始做吧!
# Calculating Returns & setting Benchmark for companiesret_aapl <- diff(log(Cl(AAPL)))
ret_tsla <- diff(log(Cl(TSLA)))
ret_nflx <- diff(log(Cl(NFLX)))benchmark_aapl <- ret_aapl
benchmark_tsla <- ret_tsla
benchmark_nflx <- ret_nflx
Now, we are set to conduct our backtests.
现在,我们准备进行回测。
Simple Moving Average (SMA)
简单移动平均线(SMA)
The following code will first calculate SMA strategy daily returns, commission adjusted SMA daily returns and finally runs the backtest (Comparison chart and an Annualized returns table) :
以下代码将首先计算SMA策略的每日收益,佣金调整后的SMA日收益,最后运行回溯测试(比较图和年化收益表):
# SMA # 1. AAPL
sma_aapl_ret <- ret_aapl*sma_aapl_strat
sma_aapl_ret_commission_adj <- ifelse((sma_aapl_ts == 1|sma_aapl_ts == -1) & sma_aapl_strat != Lag(sma_aapl_ts), (ret_aapl-0.05)*sma_aapl_strat, ret_aapl*sma_aapl_strat)
sma_aapl_comp <- cbind(sma_aapl_ret, sma_aapl_ret_commission_adj, benchmark_aapl)
colnames(sma_aapl_comp) <- c('SMA','SMA Commission Adj','Apple Benchmark')
charts.PerformanceSummary(sma_aapl_comp, main = 'Apple SMA Performance')
sma_aapl_comp_table <- table.AnnualizedReturns(sma_aapl_comp)# 2. TSLA
sma_tsla_ret <- ret_tsla*sma_tsla_strat
sma_tsla_ret_commission_adj <- ifelse((sma_tsla_ts == 1|sma_tsla_ts == -1) & sma_tsla_strat != Lag(sma_tsla_ts), (ret_tsla-0.05)*sma_tsla_strat, ret_tsla*sma_tsla_strat)
sma_tsla_comp <- cbind(sma_tsla_ret, sma_tsla_ret_commission_adj, benchmark_tsla)
colnames(sma_tsla_comp) <- c('SMA','SMA Commission Adj','Tesla Benchmark')
charts.PerformanceSummary(sma_tsla_comp, main = 'Tesla SMA Performance')
sma_tsla_comp_table <- table.AnnualizedReturns(sma_tsla_comp)# 3. NFLX
sma_nflx_ret <- ret_nflx*sma_nflx_strat
sma_nflx_ret_commission_adj <- ifelse((sma_nflx_ts == 1|sma_nflx_ts == -1) & sma_nflx_strat != Lag(sma_nflx_ts), (ret_nflx-0.05)*sma_nflx_strat, ret_nflx*sma_nflx_strat)
sma_nflx_comp <- cbind(sma_nflx_ret, sma_nflx_ret_commission_adj, benchmark_nflx)
colnames(sma_nflx_comp) <- c('SMA','SMA Commission Adj','Netflix Benchmark')
charts.PerformanceSummary(sma_nflx_comp, main = 'Netflix SMA Performance')
sma_nflx_comp_table <- table.AnnualizedReturns(sma_nflx_comp)

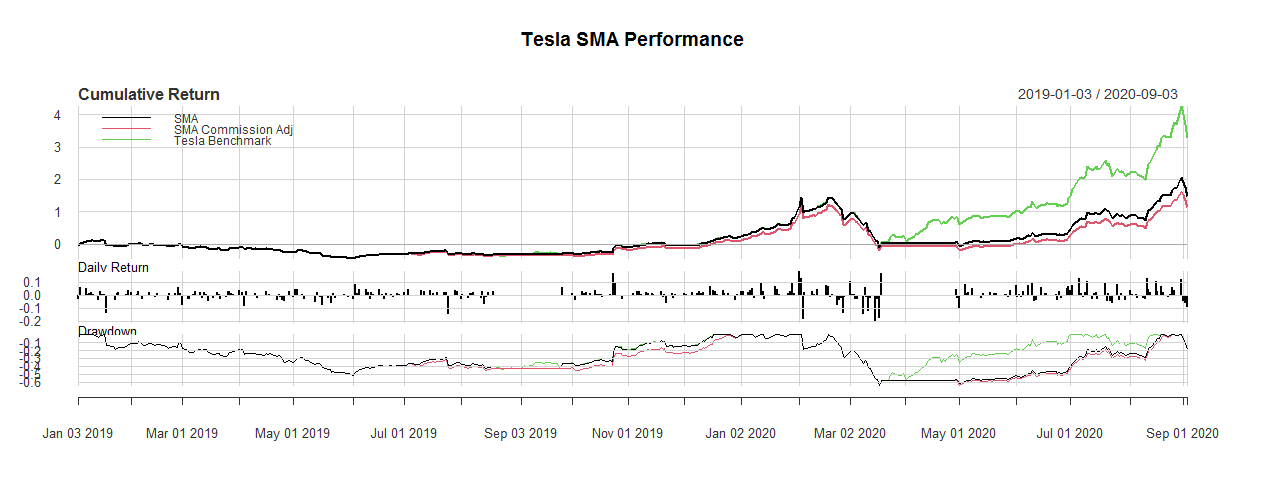
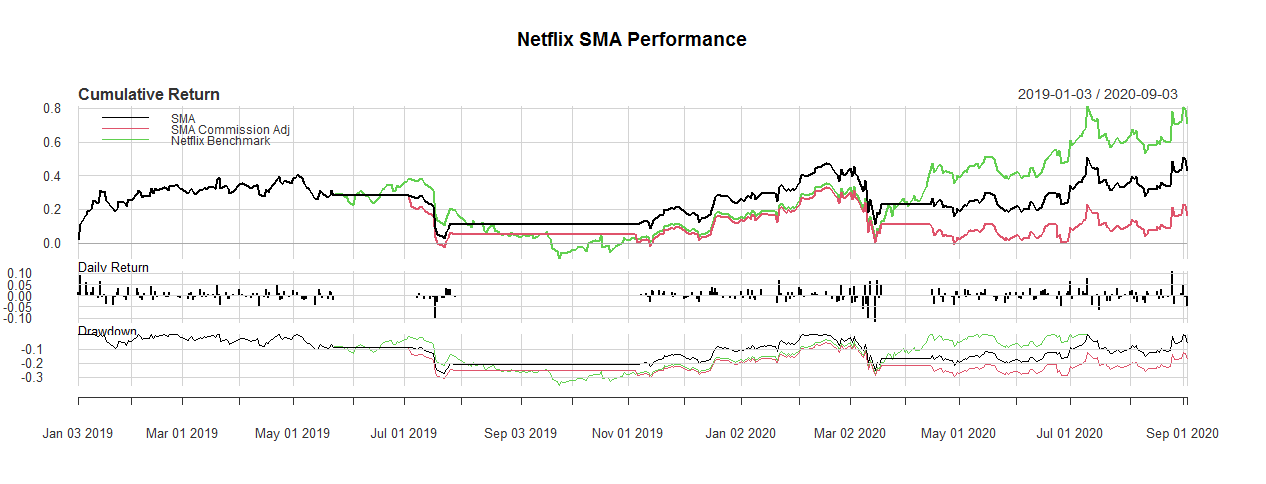
Parabolic SAR
抛物线SAR
The following code will first calculate Parabolic SAR strategy daily returns, commission adjusted Parabolic SAR daily returns and finally runs the backtest (Comparison chart and an Annualized returns table) :
以下代码将首先计算抛物线SAR策略的每日收益,佣金调整后的抛物线SAR每日收益,最后运行回测(比较图和年度收益表):
# Parabolic SAR # 1. AAPL
sar_aapl_ret <- ret_aapl*sar_aapl_strat
sar_aapl_ret_commission_adj <- ifelse((sar_aapl_ts == 1|sar_aapl_ts == -1) & sar_aapl_strat != Lag(sar_aapl_ts), (ret_aapl-0.05)*sar_aapl_strat, ret_aapl*sar_aapl_strat)
sar_aapl_comp <- cbind(sar_aapl_ret, sar_aapl_ret_commission_adj, benchmark_aapl)
colnames(sar_aapl_comp) <- c('SAR','SAR Commission Adj','Apple Benchmark')
charts.PerformanceSummary(sar_aapl_comp, main = 'Apple Parabolic SAR Performance')
sar_aapl_comp_table <- table.AnnualizedReturns(sar_aapl_comp)# 2. TSLA
sar_tsla_ret <- ret_tsla*sar_tsla_strat
sar_tsla_ret_commission_adj <- ifelse((sar_tsla_ts == 1|sar_tsla_ts == -1) & sar_tsla_strat != Lag(sar_tsla_ts), (ret_tsla-0.05)*sar_tsla_strat, ret_tsla*sar_tsla_strat)
sar_tsla_comp <- cbind(sar_tsla_ret, sar_tsla_ret_commission_adj, benchmark_tsla)
colnames(sar_tsla_comp) <- c('SAR','SAR Commission Adj','Tesla Benchmark')
charts.PerformanceSummary(sar_tsla_comp, main = 'Tesla Parabolic SAR Performance')
sar_tsla_comp_table <- table.AnnualizedReturns(sar_tsla_comp)# 3. NFLX
sar_nflx_ret <- ret_nflx*sar_nflx_strat
sar_nflx_ret_commission_adj <- ifelse((sar_nflx_ts == 1|sar_nflx_ts == -1) & sar_nflx_strat != Lag(sar_nflx_ts), (ret_nflx-0.05)*sar_nflx_strat, ret_nflx*sar_nflx_strat)
sar_nflx_comp <- cbind(sar_nflx_ret, sar_nflx_ret_commission_adj, benchmark_nflx)
colnames(sar_nflx_comp) <- c('SAR','SAR Commission Adj','Netflix Benchmark')
charts.PerformanceSummary(sar_nflx_comp, main = 'Netflix Parabolic SAR Performance')
sar_nflx_comp_table <- table.AnnualizedReturns(sar_nflx_comp)
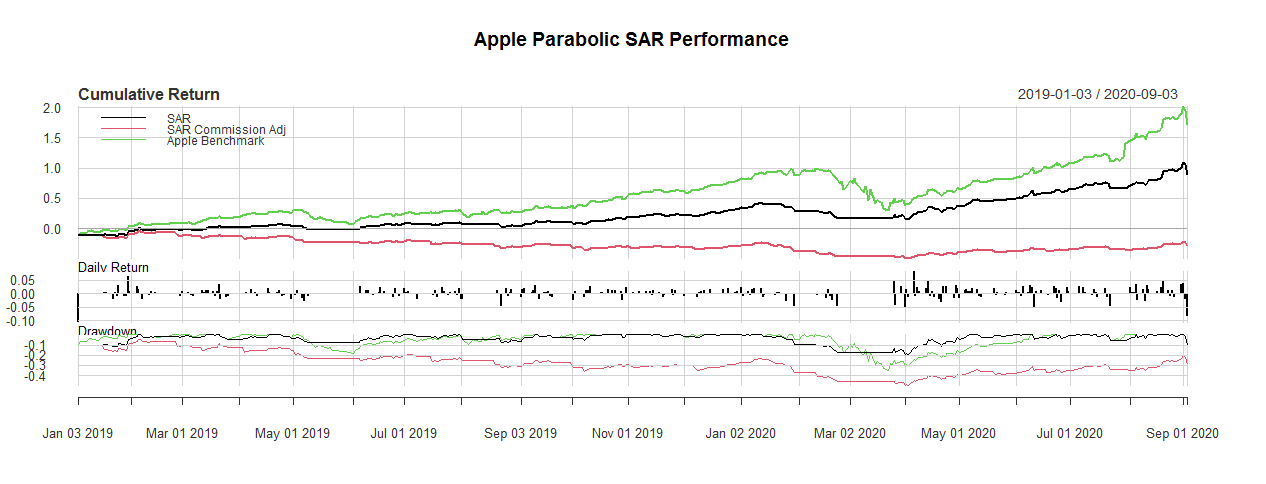
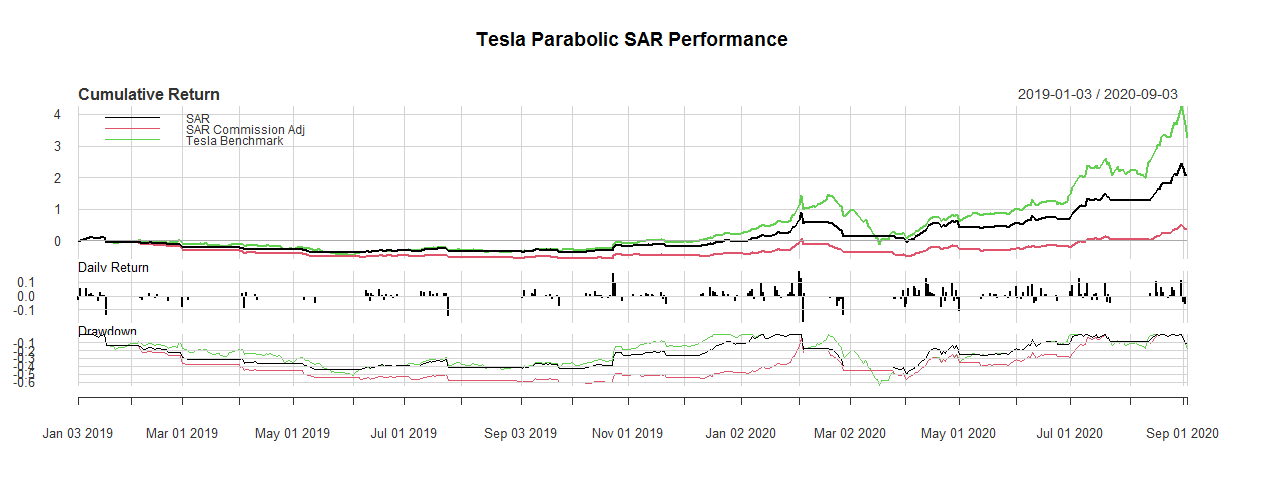

Commodity Channel Index (CCI)
商品渠道指数(CCI)
The following code will first calculate CCI strategy daily returns, commission adjusted CCI daily returns and finally runs the backtest (Comparison chart and an Annualized returns table) :
以下代码将首先计算CCI战略每日收益,佣金调整后的CCI日收益,最后运行回溯测试(比较图和年化收益表):
# CCI # 1. AAPL
cci_aapl_ret <- ret_aapl*cci_aapl_strat
cci_aapl_ret_commission_adj <- ifelse((cci_aapl_ts == 1|cci_aapl_ts == -1) & cci_aapl_strat != Lag(cci_aapl_ts), (ret_aapl-0.05)*cci_aapl_strat, ret_aapl*cci_aapl_strat)
cci_aapl_comp <- cbind(cci_aapl_ret, cci_aapl_ret_commission_adj, benchmark_aapl)
colnames(cci_aapl_comp) <- c('CCI','CCI Commission Adj','Apple Benchmark')
charts.PerformanceSummary(cci_aapl_comp, main = 'Apple CCI Performance')
cci_aapl_comp_table <- table.AnnualizedReturns(cci_aapl_comp)# 2. TSLA
cci_tsla_ret <- ret_tsla*cci_tsla_strat
cci_tsla_ret_commission_adj <- ifelse((cci_tsla_ts == 1|cci_tsla_ts == -1) & cci_tsla_strat != Lag(cci_tsla_ts), (ret_tsla-0.05)*cci_tsla_strat, ret_tsla*cci_tsla_strat)
cci_tsla_comp <- cbind(cci_tsla_ret, cci_tsla_ret_commission_adj, benchmark_tsla)
colnames(cci_tsla_comp) <- c('CCI','CCI Commission Adj','Tesla Benchmark')
charts.PerformanceSummary(cci_tsla_comp, main = 'Tesla CCI Performance')
cci_tsla_comp_table <- table.AnnualizedReturns(cci_tsla_comp)# 3. NFLX
cci_nflx_ret <- ret_nflx*cci_nflx_strat
cci_nflx_ret_commission_adj <- ifelse((cci_nflx_ts == 1|cci_nflx_ts == -1) & cci_nflx_strat != Lag(cci_nflx_ts), (ret_nflx-0.05)*cci_nflx_strat, ret_nflx*cci_nflx_strat)
cci_nflx_comp <- cbind(cci_nflx_ret, cci_nflx_ret_commission_adj, benchmark_nflx)
colnames(cci_nflx_comp) <- c('CCI','CCI Commission Adj','Netflix Benchmark')
charts.PerformanceSummary(cci_nflx_comp, main = 'Netflix CCI Performance')
cci_nflx_comp_table <- table.AnnualizedReturns(cci_nflx_comp)
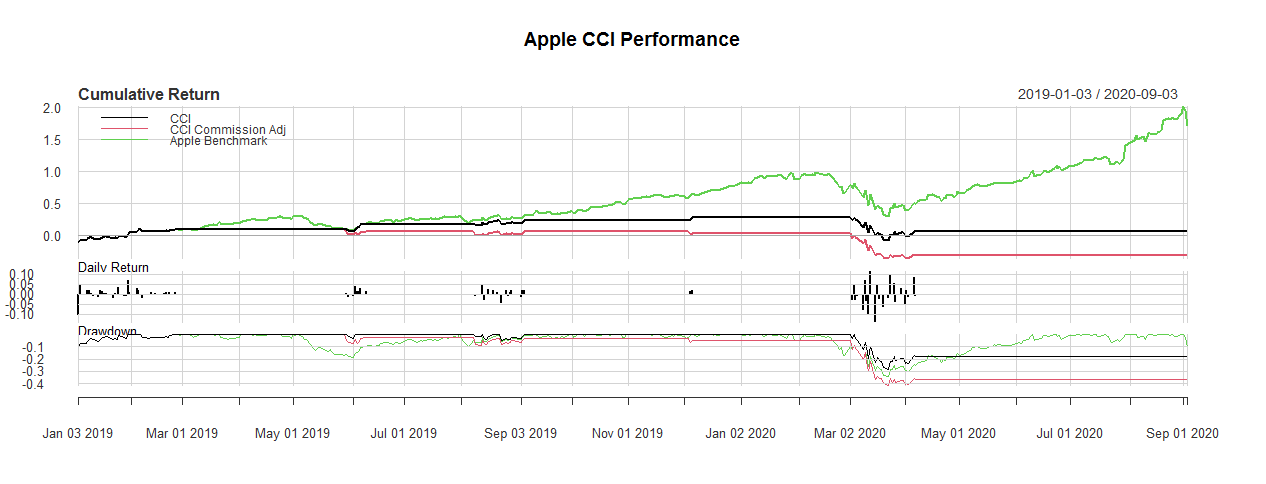

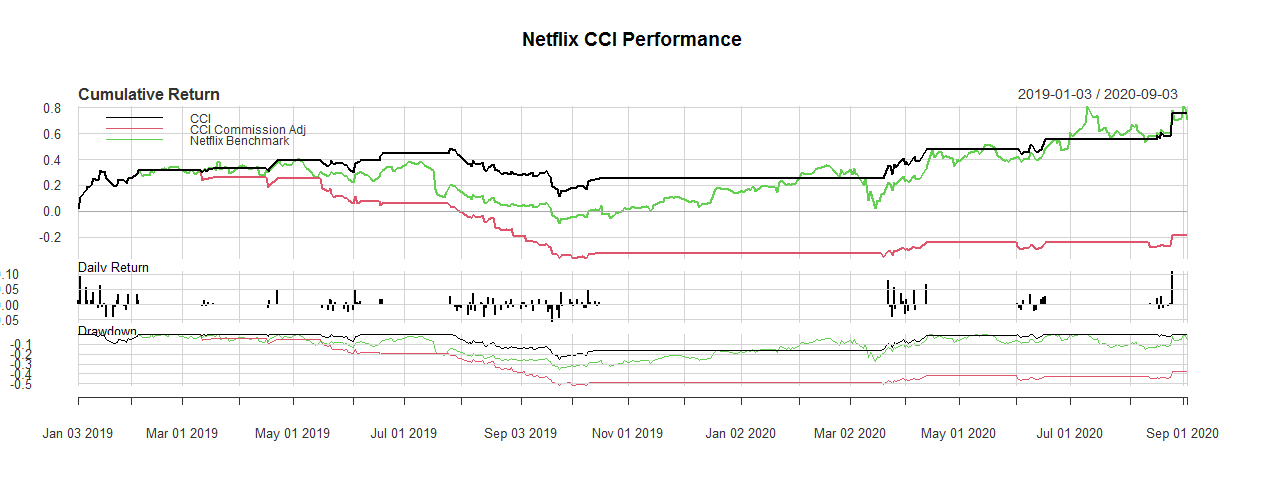
Rate Of Change (ROC)
变化率(ROC)
The following code will first calculate ROC strategy daily returns, commission adjusted ROC daily returns and finally runs the backtest (Comparison chart and an Annualized returns table) :
以下代码将首先计算ROC策略的每日收益,佣金调整后的ROC每日收益,最后运行回溯测试(比较图和年化收益表):
# ROC # 1. AAPL
roc_aapl_ret <- ret_aapl*roc_aapl_strat
roc_aapl_ret_commission_adj <- ifelse((roc_aapl_ts == 1|roc_aapl_ts == -1) & roc_aapl_strat != Lag(roc_aapl_ts), (ret_aapl-0.05)*roc_aapl_strat, ret_aapl*roc_aapl_strat)
roc_aapl_comp <- cbind(roc_aapl_ret, roc_aapl_ret_commission_adj, benchmark_aapl)
colnames(roc_aapl_comp) <- c('ROC','ROC Commission Adj','Apple Benchmark')
charts.PerformanceSummary(roc_aapl_comp, main = 'Apple ROC Performance')
roc_aapl_comp_table <- table.AnnualizedReturns(roc_aapl_comp)# 2. TSLA
roc_tsla_ret <- ret_tsla*roc_tsla_strat
roc_tsla_ret_commission_adj <- ifelse((roc_tsla_ts == 1|roc_tsla_ts == -1) & roc_tsla_strat != Lag(roc_tsla_ts), (ret_tsla-0.05)*roc_tsla_strat, ret_tsla*roc_tsla_strat)
roc_tsla_comp <- cbind(roc_tsla_ret, roc_tsla_ret_commission_adj, benchmark_tsla)
colnames(roc_tsla_comp) <- c('ROC','ROC Commission Adj','Tesla Benchmark')
charts.PerformanceSummary(roc_tsla_comp, main = 'Tesla ROC Performance')
roc_tsla_comp_table <- table.AnnualizedReturns(roc_tsla_comp)# 3. NFLX
roc_nflx_ret <- ret_nflx*roc_nflx_strat
roc_nflx_ret_commission_adj <- ifelse((roc_nflx_ts == 1|roc_nflx_ts == -1) & roc_nflx_strat != Lag(roc_nflx_ts), (ret_nflx-0.05)*roc_nflx_strat, ret_nflx*roc_nflx_strat)
roc_nflx_comp <- cbind(roc_nflx_ret, roc_nflx_ret_commission_adj, benchmark_nflx)
colnames(roc_nflx_comp) <- c('ROC','ROC Commission Adj','Netflix Benchmark')
charts.PerformanceSummary(roc_nflx_comp, main = 'Netflix ROC Performance')
roc_nflx_comp_table <- table.AnnualizedReturns(roc_nflx_comp)

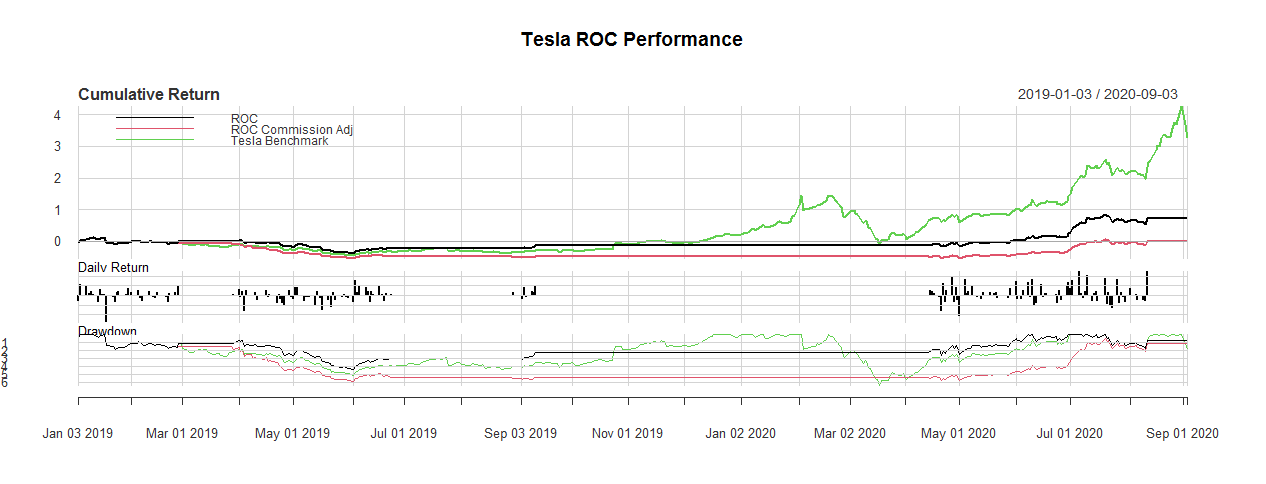
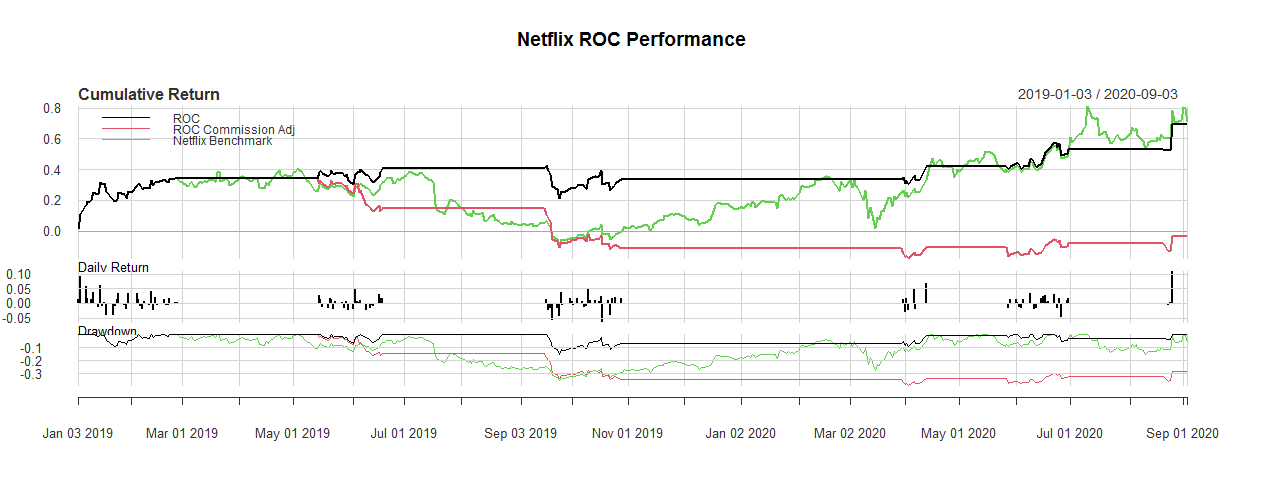
Stochastic Momentum Index (SMI)
随机动量指数(SMI)
The following code will first calculate SMI strategy daily returns, commission adjusted SMI daily returns and finally runs the backtest (Comparison chart and an Annualized returns table) :
以下代码将首先计算SMI策略的每日收益,佣金调整后的SMI每日收益,最后运行回溯测试(比较图和年化收益表):
# SMI # 1. AAPL
smi_aapl_ret <- ret_aapl*smi_aapl_strat
smi_aapl_ret_commission_adj <- ifelse((smi_aapl_ts == 1|smi_aapl_ts == -1) & smi_aapl_strat != Lag(smi_aapl_ts), (ret_aapl-0.05)*smi_aapl_strat, ret_aapl*smi_aapl_strat)
smi_aapl_comp <- cbind(smi_aapl_ret, smi_aapl_ret_commission_adj, benchmark_aapl)
colnames(smi_aapl_comp) <- c('SMI','SMI Commission Adj','Apple Benchmark')
charts.PerformanceSummary(smi_aapl_comp, main = 'Apple SMI Performance')
smi_aapl_comp_table <- table.AnnualizedReturns(smi_aapl_comp)# 2. TSLA
smi_tsla_ret <- ret_tsla*smi_tsla_strat
smi_tsla_ret_commission_adj <- ifelse((smi_tsla_ts == 1|smi_tsla_ts == -1) & smi_tsla_strat != Lag(smi_tsla_ts), (ret_tsla-0.05)*smi_tsla_strat, ret_tsla*smi_tsla_strat)
smi_tsla_comp <- cbind(smi_tsla_ret, smi_tsla_ret_commission_adj, benchmark_tsla)
colnames(smi_tsla_comp) <- c('SMI','SMI Commission Adj','Tesla Benchmark')
charts.PerformanceSummary(smi_tsla_comp, main = 'Tesla SMI Performance')
smi_tsla_comp_table <- table.AnnualizedReturns(smi_tsla_comp)# 3. NFLX
smi_nflx_ret <- ret_nflx*smi_nflx_strat
smi_nflx_ret_commission_adj <- ifelse((smi_nflx_ts == 1|smi_nflx_ts == -1) & smi_nflx_strat != Lag(smi_nflx_ts), (ret_nflx-0.05)*smi_nflx_strat, ret_nflx*smi_nflx_strat)
smi_nflx_comp <- cbind(smi_nflx_ret, smi_nflx_ret_commission_adj, benchmark_nflx)
colnames(smi_nflx_comp) <- c('SMI','SMI Commission Adj','Netflix Benchmark')
charts.PerformanceSummary(smi_nflx_comp, main = 'Netflix SMI Performance')
smi_nflx_comp_table <- table.AnnualizedReturns(smi_nflx_comp)

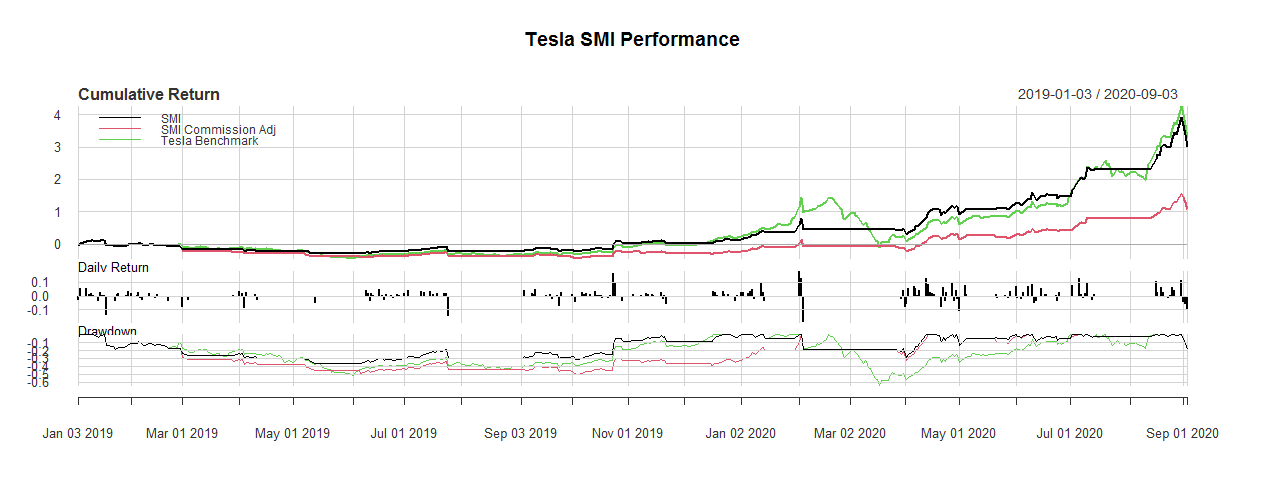
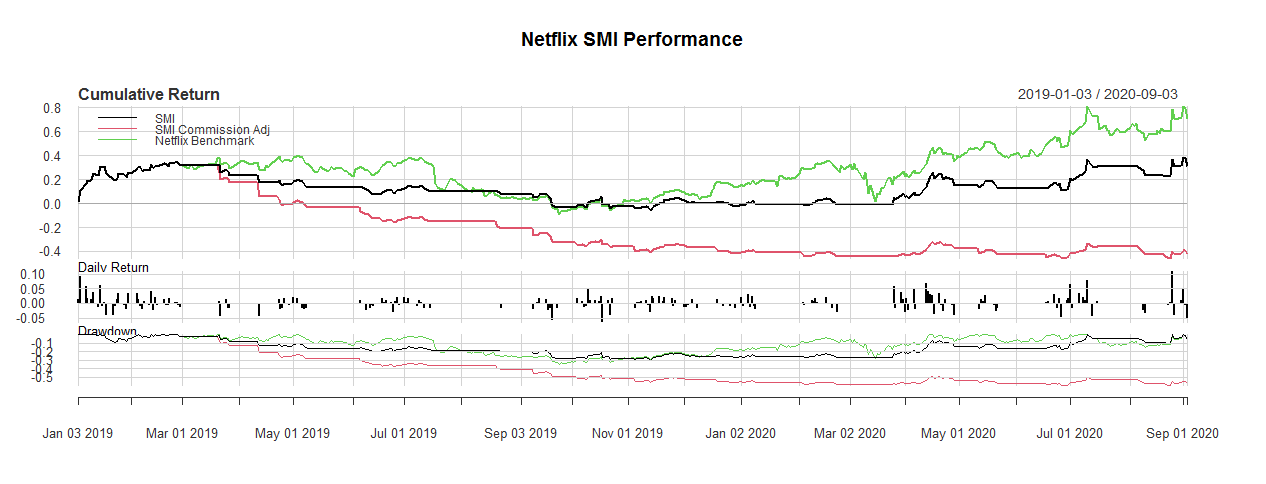
Williams %R
威廉姆斯%R
The following code will first calculate Williams %R strategy daily returns, commission adjusted Williams %R daily returns and finally runs the backtest (Comparison chart and an Annualized returns table) :
以下代码将首先计算Williams%R策略的每日收益,佣金调整后的Williams%R日收益,最后运行回溯测试(比较图和年化收益表):
# WPR # 1. AAPL
wpr_aapl_ret <- ret_aapl*wpr_aapl_strat
wpr_aapl_ret_commission_adj <- ifelse((wpr_aapl_ts == 1|wpr_aapl_ts == -1) & wpr_aapl_strat != Lag(wpr_aapl_ts), (ret_aapl-0.05)*wpr_aapl_strat, ret_aapl*wpr_aapl_strat)
wpr_aapl_comp <- cbind(wpr_aapl_ret, wpr_aapl_ret_commission_adj, benchmark_aapl)
colnames(wpr_aapl_comp) <- c('WPR','WPR Commission Adj','Apple Benchmark')
charts.PerformanceSummary(wpr_aapl_comp, main = 'Apple WPR Performance')
wpr_aapl_comp_table <- table.AnnualizedReturns(wpr_aapl_comp)# 2. TSLA
wpr_tsla_ret <- ret_tsla*wpr_tsla_strat
wpr_tsla_ret_commission_adj <- ifelse((wpr_tsla_ts == 1|wpr_tsla_ts == -1) & wpr_tsla_strat != Lag(wpr_tsla_ts), (ret_tsla-0.05)*wpr_tsla_strat, ret_tsla*wpr_tsla_strat)
wpr_tsla_comp <- cbind(wpr_tsla_ret, wpr_tsla_ret_commission_adj, benchmark_tsla)
colnames(wpr_tsla_comp) <- c('WPR','WPR Commission Adj','Tesla Benchmark')
charts.PerformanceSummary(wpr_tsla_comp, main = 'Tesla WPR Performance')
wpr_tsla_comp_table <- table.AnnualizedReturns(wpr_tsla_comp)# 3. NFLX
wpr_nflx_ret <- ret_nflx*wpr_nflx_strat
wpr_nflx_ret_commission_adj <- ifelse((wpr_nflx_ts == 1|wpr_nflx_ts == -1) & wpr_nflx_strat != Lag(wpr_nflx_ts), (ret_nflx-0.05)*wpr_nflx_strat, ret_nflx*wpr_nflx_strat)
wpr_nflx_comp <- cbind(wpr_nflx_ret, wpr_nflx_ret_commission_adj, benchmark_nflx)
colnames(wpr_nflx_comp) <- c('WPR','WPR Commission Adj','Netflix Benchmark')
charts.PerformanceSummary(wpr_nflx_comp, main = 'Netflix WPR Performance')
wpr_nflx_comp_table <- table.AnnualizedReturns(wpr_nflx_comp)
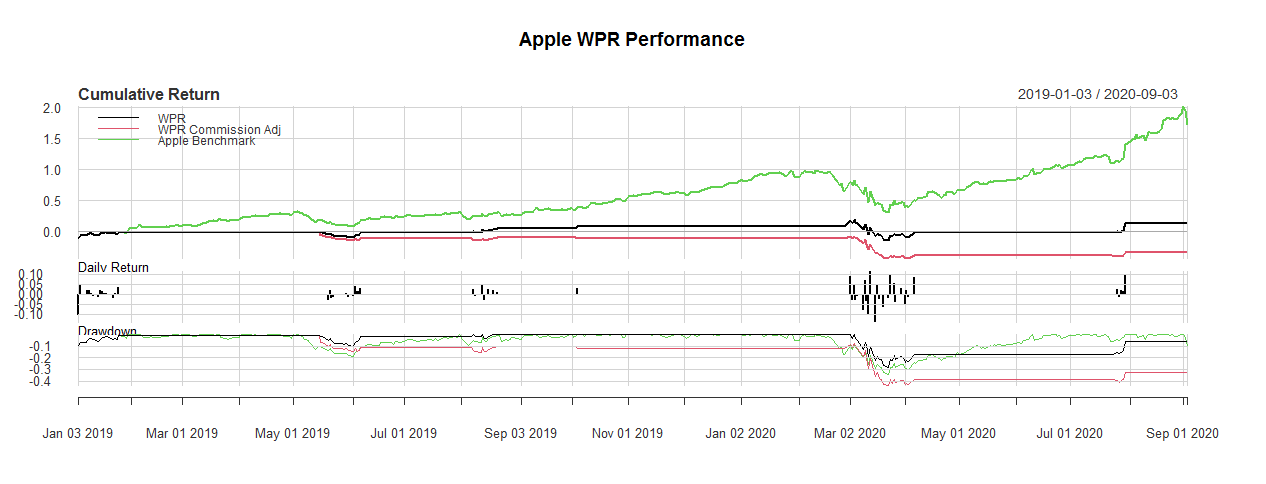


最后的想法! (Final Thoughts!)
Hurrah! We successfully finished our process of creating trading strategies and backtesting the results with R. I want to highlight that, every strategies and backtesting results are only for educational purpose and should not be taken as an investment advice. Apart from our created strategies, you can do your coding and frame your own trading strategies based on our needs and flexibility. If you’ve missed any of the coding sections, no worries. I’ve added the full code file at the end. In this article, we’ve covered only six major technical indicators but, there are more to discover. So, never stop learning and never stop coding!
欢呼! 我们成功地完成了创建交易策略并使用R对结果进行回测的过程。我想强调一点,每一种策略和回测结果仅用于教育目的,不应被视为投资建议。 除了我们创建的策略之外,您还可以根据我们的需求和灵活性进行编码并制定自己的交易策略。 如果您错过了任何编码部分,请不要担心。 我在末尾添加了完整的代码文件。 在本文中,我们仅介绍了六个主要技术指标,但还有更多发现之处。 因此,永远不要停止学习,永远不要停止编码!
Happy Coding and Analyzing!
快乐的编码和分析!
Full Code :
完整代码:
library(quantmod)
library(TTR)
library(PerformanceAnalytics)# Getting stock prices of AAPL, TSLA and NFLX
getSymbols('AAPL', src = 'yahoo', from = '2019-01-01')
getSymbols('TSLA', src = 'yahoo', from = '2019-01-01')
getSymbols('NFLX', src = 'yahoo', from = '2019-01-01')# Basic plot of the three stocks
barChart(AAPL, theme = chartTheme('black'))
barChart(TSLA, theme = chartTheme('black'))
barChart(NFLX, theme = chartTheme('black'))# Creating Leading and Lagging Technical Indicators# a. Simple Moving Average (SMA)# 1. AAPL
sma20_aapl <- SMA(AAPL$AAPL.Close, n = 20)
sma50_aapl <- SMA(AAPL$AAPL.Close, n = 50)
lineChart(AAPL, theme = chartTheme('black'))
addSMA(n = 20, col = 'blue')
addSMA(n = 50, col = 'orange')
legend('left', col = c('green','blue','orange'),legend = c('AAPL','SMA20','SMA50'), lty = 1, bty = 'n',text.col = 'white', cex = 0.8)
# 2. TSLA
sma20_tsla <- SMA(TSLA$TSLA.Close, n = 20)
sma50_tsla <- SMA(TSLA$TSLA.Close, n = 50)
lineChart(TSLA, theme = 'black')
addSMA(n = 20, col = 'blue')
addSMA(n = 50, col = 'orange')
legend('left', col = c('green','blue','orange'),legend = c('AAPL','SMA20','SMA50'), lty = 1, bty = 'n',text.col = 'white', cex = 0.8)
# 3. NFLX
sma20_nflx <- SMA(NFLX$NFLX.Close, n = 20)
sma50_nflx <- SMA(NFLX$NFLX.Close, n = 50)
lineChart(NFLX, theme = 'black')
addSMA(n = 20, col = 'blue')
addSMA(n = 50, col = 'orange')
legend('left', col = c('green','blue','orange'),legend = c('AAPL','SMA20','SMA50'), lty = 1, bty = 'n',text.col = 'white', cex = 0.8)# b. Parabolic Stop And Reverse (SAR)# 1. AAPL
sar_aapl <- SAR(cbind(Hi(AAPL),Lo(AAPL)), accel = c(0.02, 0.2))
barChart(AAPL, theme = 'black')
addSAR(accel = c(0.02, 0.2), col = 'lightblue')
# 2. TSLA
sar_tsla <- SAR(cbind(Hi(TSLA),Lo(TSLA)), accel = c(0.02, 0.2))
barChart(TSLA, theme = 'black')
addSAR(accel = c(0.02, 0.2), col = 'lightblue')
# 3. NFLX
sar_nflx <- SAR(cbind(Hi(NFLX),Lo(NFLX)), accel = c(0.02, 0.2))
barChart(NFLX, theme = 'black')
addSAR(accel = c(0.02, 0.2), col = 'lightblue')# c. Commodity Channel Index (CCI)# 1. AAPL
cci_aapl <- CCI(HLC(AAPL), n = 20, c = 0.015)
barChart(AAPL, theme = 'black')
addCCI(n = 20, c = 0.015)
# 2. TSLA
cci_tsla <- CCI(HLC(TSLA), n = 20, c = 0.015)
barChart(TSLA, theme = 'black')
addCCI(n = 20, c = 0.015)
# 3. NFLX
cci_nflx <- CCI(HLC(NFLX), n = 20, c = 0.015)
barChart(NFLX, theme = 'black')
addCCI(n = 20, c = 0.015)# d. Rate of Change (ROC)# 1. AAPL
roc_aapl <- ROC(AAPL$AAPL.Close, n = 25)
barChart(AAPL, theme = 'black')
addROC(n = 25)
legend('left', col = 'red', legend = 'ROC(25)', lty = 1, bty = 'n',text.col = 'white', cex = 0.8)
# 1. TSLA
roc_tsla <- ROC(TSLA$TSLA.Close, n = 25)
barChart(TSLA, theme = 'black')
addROC(n = 25)
legend('left', col = 'red', legend = 'ROC(25)', lty = 1, bty = 'n',text.col = 'white', cex = 0.8)
# 1. NFLX
roc_nflx <- ROC(NFLX$NFLX.Close, n = 25)
barChart(NFLX, theme = 'black')
addROC(n = 25)
legend('right', col = 'red', legend = 'ROC(25)', lty = 1, bty = 'n',text.col = 'white', cex = 0.8)# e. Stochastic Momentum Index (SMI)# 1. AAPL
smi_aapl <- SMI(HLC(AAPL),n = 13, nFast = 2, nSlow = 25, nSig = 9)
barChart(AAPL, theme = 'black')
addSMI(n = 13, fast = 2, slow = 2, signal = 9)
# 2. TSLA
smi_tsla <- SMI(HLC(TSLA),n = 13, nFast = 2, nSlow = 25, nSig = 9)
barChart(TSLA, theme = 'black')
addSMI(n = 13, fast = 2, slow = 2, signal = 9)
# 3. NFLX
smi_nflx <- SMI(HLC(NFLX),n = 13, nFast = 2, nSlow = 25, nSig = 9)
barChart(NFLX, theme = 'black')
addSMI(n = 13, fast = 2, slow = 2, signal = 9)# f. Williams %R# 1. AAPL
wpr_aapl <- WPR(HLC(AAPL), n = 14)
colnames(wpr_aapl) <- 'wpr'
barChart(AAPL, theme = 'black')
addWPR(n = 14)
# 1. TSLA
wpr_tsla <- WPR(HLC(TSLA), n = 14)
colnames(wpr_tsla) <- 'wpr'
barChart(TSLA, theme = 'black')
addWPR(n = 14)
# 1. NFLX
wpr_nflx <- WPR(HLC(NFLX), n = 14)
colnames(wpr_nflx) <- 'wpr'
barChart(NFLX, theme = 'black')
addWPR(n = 14)# Creating Trading signal with Indicators# SMA # a. AAPL
# SMA 20 Crossover Signal
sma20_aapl_ts <- Lag(ifelse(Lag(Cl(AAPL)) < Lag(sma20_aapl) & Cl(AAPL) > sma20_aapl,1,ifelse(Lag(Cl(AAPL)) > Lag(sma20_aapl) & Cl(AAPL) < sma20_aapl,-1,0)))
sma20_aapl_ts[is.na(sma20_aapl_ts)] <- 0
# SMA 50 Crossover Signal
sma50_aapl_ts <- Lag(ifelse(Lag(Cl(AAPL)) < Lag(sma50_aapl) & Cl(AAPL) > sma50_aapl,1,ifelse(Lag(Cl(AAPL)) > Lag(sma50_aapl) & Cl(AAPL) < sma50_aapl,-1,0)))
sma50_aapl_ts[is.na(sma50_aapl_ts)] <- 0
# SMA 20 and SMA 50 Crossover Signal
sma_aapl_ts <- Lag(ifelse(Lag(sma20_aapl) < Lag(sma50_aapl) & sma20_aapl > sma50_aapl,1,ifelse(Lag(sma20_aapl) > Lag(sma50_aapl) & sma20_aapl < sma50_aapl,-1,0)))
sma_aapl_ts[is.na(sma_aapl_ts)] <- 0# b. TSLA
# SMA 20 Crossover Signal
sma20_tsla_ts <- Lag(ifelse(Lag(Cl(TSLA)) < Lag(sma20_tsla) & Cl(TSLA) > sma20_tsla,1,ifelse(Lag(Cl(TSLA)) > Lag(sma20_tsla) & Cl(TSLA) < sma20_tsla,-1,0)))
sma20_tsla_ts[is.na(sma20_tsla_ts)] <- 0
# SMA 50 Crossover Signal
sma50_tsla_ts <- Lag(ifelse(Lag(Cl(TSLA)) < Lag(sma50_tsla) & Cl(TSLA) > sma50_tsla,1,ifelse(Lag(Cl(TSLA)) > Lag(sma50_tsla) & Cl(TSLA) < sma50_tsla,-1,0)))
sma50_tsla_ts[is.na(sma50_tsla_ts)] <- 0
# SMA 20 and SMA 50 Crossover Signal
sma_tsla_ts <- Lag(ifelse(Lag(sma20_tsla) < Lag(sma50_tsla) & sma20_tsla > sma50_tsla,1,ifelse(Lag(sma20_tsla) > Lag(sma50_tsla) & sma20_tsla < sma50_tsla,-1,0)))
sma_tsla_ts[is.na(sma_tsla_ts)] <- 0# c. NFLX
# SMA 20 Crossover Signal
sma20_nflx_ts <- Lag(ifelse(Lag(Cl(NFLX)) < Lag(sma20_nflx) & Cl(NFLX) > sma20_nflx,1,ifelse(Lag(Cl(NFLX)) > Lag(sma20_nflx) & Cl(NFLX) < sma20_nflx,-1,0)))
sma20_nflx_ts[is.na(sma20_nflx_ts)] <- 0
# SMA 50 Crossover Signal
sma50_nflx_ts <- Lag(ifelse(Lag(Cl(NFLX)) < Lag(sma50_nflx) & Cl(NFLX) > sma50_nflx,1,ifelse(Lag(Cl(NFLX)) > Lag(sma50_nflx) & Cl(NFLX) < sma50_nflx,-1,0)))
sma50_nflx_ts[is.na(sma50_nflx_ts)] <- 0
# SMA 20 and SMA 50 Crossover Signal
sma_nflx_ts <- Lag(ifelse(Lag(sma20_nflx) < Lag(sma50_nflx) & sma20_nflx > sma50_nflx,1,ifelse(Lag(sma20_nflx) > Lag(sma50_nflx) & sma20_nflx < sma50_nflx,-1,0)))
sma_nflx_ts[is.na(sma_nflx_ts)] <- 0# 2. Parabolic Stop And Reverse (SAR) # a. AAPL
sar_aapl_ts <- Lag(ifelse(Lag(Cl(AAPL)) < Lag(sar_aapl) & Cl(AAPL) > sar_aapl,1,ifelse(Lag(Cl(AAPL)) > Lag(sar_aapl) & Cl(AAPL) < sar_aapl,-1,0)))
sar_aapl_ts[is.na(sar_aapl_ts)] <- 0
# b. TSLA
sar_tsla_ts <- Lag(ifelse(Lag(Cl(TSLA)) < Lag(sar_tsla) & Cl(TSLA) > sar_tsla,1,ifelse(Lag(Cl(TSLA)) > Lag(sar_tsla) & Cl(TSLA) < sar_tsla,-1,0)))
sar_tsla_ts[is.na(sar_tsla_ts)] <- 0
# c. NFLX
sar_nflx_ts <- Lag(ifelse(Lag(Cl(NFLX)) < Lag(sar_nflx) & Cl(NFLX) > sar_nflx,1,ifelse(Lag(Cl(NFLX)) > Lag(sar_nflx) & Cl(NFLX) < sar_nflx,-1,0)))
sar_nflx_ts[is.na(sar_nflx_ts)] <- 0# 3. Commodity Channel Index (CCI)# a. AAPL
cci_aapl_ts <- Lag(ifelse(Lag(cci_aapl) < (-100) & cci_aapl > (-100),1,ifelse(Lag(cci_aapl) < (100) & cci_aapl > (100),-1,0)))
cci_aapl_ts[is.na(cci_aapl_ts)] <- 0
# b. TSLA
cci_tsla_ts <- Lag(ifelse(Lag(cci_tsla) < (-100) & cci_tsla > (-100),1,ifelse(Lag(cci_tsla) < (100) & cci_tsla > (100),-1,0)))
cci_tsla_ts[is.na(cci_tsla_ts)] <- 0
# c. NFLX
cci_nflx_ts <- Lag(ifelse(Lag(cci_nflx) < (-100) & cci_nflx > (-100),1,ifelse(Lag(cci_nflx) < (100) & cci_nflx > (100),-1,0)))
cci_nflx_ts[is.na(cci_nflx_ts)] <- 0# 4. Rate of Change (ROC)# a. AAPL
roc_aapl_ts <- Lag(ifelse(Lag(roc_aapl) < (-0.05) & roc_aapl > (-0.05),1,ifelse(Lag(roc_aapl) < (0.05) & roc_aapl > (0.05),-1,0)))
roc_aapl_ts[is.na(roc_aapl_ts)] <- 0
# b. TSLA
roc_tsla_ts <- Lag(ifelse(Lag(roc_tsla) < (-0.05) & roc_tsla > (-0.05),1,ifelse(Lag(roc_tsla) < (0.05) & roc_tsla > (0.05),-1,0)))
roc_tsla_ts[is.na(roc_tsla_ts)] <- 0
# c. NFLX
roc_nflx_ts <- Lag(ifelse(Lag(roc_nflx) < (-0.05) & roc_nflx > (-0.05),1,ifelse(Lag(roc_nflx) < (0.05) & roc_nflx > (0.05),-1,0)))
roc_nflx_ts[is.na(roc_nflx_ts)] <- 0# 5. Stochastic Momentum Index (SMI)# a. AAPL
smi_aapl_ts <- Lag(ifelse(Lag(smi_aapl[,1]) < Lag(smi_aapl[,2]) & smi_aapl[,1] > smi_aapl[,2],1, ifelse(Lag(smi_aapl[,1]) > Lag(smi_aapl[,2]) & smi_aapl[,1] < smi_aapl[,2],-1,0)))
smi_aapl_ts[is.na(smi_aapl_ts)] <- 0
# b. TSLA
smi_tsla_ts <- Lag(ifelse(Lag(smi_tsla[,1]) < Lag(smi_tsla[,2]) & smi_tsla[,1] > smi_tsla[,2],1, ifelse(Lag(smi_tsla[,1]) > Lag(smi_tsla[,2]) & smi_tsla[,1] < smi_tsla[,2],-1,0)))
smi_tsla_ts[is.na(smi_tsla_ts)] <- 0
# a. NFLX
smi_nflx_ts <- Lag(ifelse(Lag(smi_nflx[,1]) < Lag(smi_nflx[,2]) & smi_nflx[,1] > smi_nflx[,2],1, ifelse(Lag(smi_nflx[,1]) > Lag(smi_nflx[,2]) & smi_nflx[,1] < smi_nflx[,2],-1,0)))
smi_nflx_ts[is.na(smi_nflx_ts)] <- 0# 6. williams %R# a. AAPL
wpr_aapl_ts <- Lag(ifelse(Lag(wpr_aapl) > 0.8 & wpr_aapl < 0.8,1,ifelse(Lag(wpr_aapl) > 0.2 & wpr_aapl < 0.2,-1,0)))
wpr_aapl_ts[is.na(wpr_aapl_ts)] <- 0
# b. TSLA
wpr_tsla_ts <- Lag(ifelse(Lag(wpr_tsla) > 0.8 & wpr_tsla < 0.8,1,ifelse(Lag(wpr_tsla) > 0.2 & wpr_tsla < 0.2,-1,0)))
wpr_tsla_ts[is.na(wpr_tsla_ts)] <- 0
# c. NFLX
wpr_nflx_ts <- Lag(ifelse(Lag(wpr_nflx) > 0.8 & wpr_nflx < 0.8,1,ifelse(Lag(wpr_nflx) > 0.2 & wpr_nflx < 0.2,-1,0)))
wpr_nflx_ts[is.na(wpr_nflx_ts)] <- 0# Creating Trading Strategies using Signals# 1. SMA 20 and SMA 50 Crossover Strategy# a. AAPL
sma_aapl_strat <- ifelse(sma_aapl_ts > 1,0,1)
for (i in 1 : length(Cl(AAPL))) {sma_aapl_strat[i] <- ifelse(sma_aapl_ts[i] == 1,1,ifelse(sma_aapl_ts[i] == -1,0,sma_aapl_strat[i-1]))
}
sma_aapl_strat[is.na(sma_aapl_strat)] <- 1
sma_aapl_stratcomp <- cbind(sma20_aapl, sma50_aapl, sma_aapl_ts, sma_aapl_strat)
colnames(sma_aapl_stratcomp) <- c('SMA(20)','SMA(50)','SMA SIGNAL','SMA POSITION')
# b. TSLA
sma_tsla_strat <- ifelse(sma_tsla_ts > 1,0,1)
for (i in 1 : length(Cl(TSLA))) {sma_tsla_strat[i] <- ifelse(sma_tsla_ts[i] == 1,1,ifelse(sma_tsla_ts[i] == -1,0,sma_tsla_strat[i-1]))
}
sma_tsla_strat[is.na(sma_tsla_strat)] <- 1
sma_tsla_stratcomp <- cbind(sma20_tsla, sma50_tsla, sma_tsla_ts, sma_tsla_strat)
colnames(sma_tsla_stratcomp) <- c('SMA(20)','SMA(50)','SMA SIGNAL','SMA POSITION')
# c. NFLX
sma_nflx_strat <- ifelse(sma_nflx_ts > 1,0,1)
for (i in 1 : length(Cl(NFLX))) {sma_nflx_strat[i] <- ifelse(sma_nflx_ts[i] == 1,1,ifelse(sma_nflx_ts[i] == 'SEL',0,sma_nflx_strat[i-1]))
}
sma_nflx_strat[is.na(sma_nflx_strat)] <- 1
sma_nflx_stratcomp <- cbind(sma20_nflx, sma50_nflx, sma_nflx_ts, sma_nflx_strat)
colnames(sma_nflx_stratcomp) <- c('SMA(20)','SMA(50)','SMA SIGNAL','SMA POSITION')# Parabolic SAR Strategy # a. AAPL
sar_aapl_strat <- ifelse(sar_aapl_ts > 1,0,1)
for (i in 1 : length(Cl(AAPL))) {sar_aapl_strat[i] <- ifelse(sar_aapl_ts[i] == 1,1,ifelse(sar_aapl_ts[i] == -1,0,sar_aapl_strat[i-1]))
}
sar_aapl_strat[is.na(sar_aapl_strat)] <- 1
sar_aapl_stratcomp <- cbind(Cl(AAPL), sar_aapl, sar_aapl_ts, sar_aapl_strat)
colnames(sar_aapl_stratcomp) <- c('Close','SAR','SAR SIGNAL','SAR POSITION')
# b. TSLA
sar_tsla_strat <- ifelse(sar_tsla_ts > 1,0,1)
for (i in 1 : length(Cl(TSLA))) {sar_tsla_strat[i] <- ifelse(sar_tsla_ts[i] == 1,1,ifelse(sar_tsla_ts[i] == -1,0,sar_tsla_strat[i-1]))
}
sar_tsla_strat[is.na(sar_tsla_strat)] <- 1
sar_tsla_stratcomp <- cbind(Cl(TSLA), sar_tsla, sar_tsla_ts, sar_tsla_strat)
colnames(sar_tsla_stratcomp) <- c('Close','SAR','SAR SIGNAL','SAR POSITION')
# c. NFLX
sar_nflx_strat <- ifelse(sar_nflx_ts > 1,0,1)
for (i in 1 : length(Cl(NFLX))) {sar_nflx_strat[i] <- ifelse(sar_nflx_ts[i] == 1,1,ifelse(sar_nflx_ts[i] == -1,0,sar_nflx_strat[i-1]))
}
sar_nflx_strat[is.na(sar_nflx_strat)] <- 1
sar_nflx_stratcomp <- cbind(Cl(NFLX), sar_nflx, sar_nflx_ts, sar_nflx_strat)
colnames(sar_nflx_stratcomp) <- c('Close','SAR','SAR SIGNAL','SAR POSITION')# CCI# a. AAPL
cci_aapl_strat <- ifelse(cci_aapl_ts > 1,0,1)
for (i in 1 : length(Cl(AAPL))) {cci_aapl_strat[i] <- ifelse(cci_aapl_ts[i] == 1,1,ifelse(cci_aapl_ts[i] == -1,0,cci_aapl_strat[i-1]))
}
cci_aapl_strat[is.na(cci_aapl_strat)] <- 1
cci_aapl_stratcomp <- cbind(cci_aapl, cci_aapl_ts, cci_aapl_strat)
colnames(cci_aapl_stratcomp) <- c('CCI','CCI SIGNAL','CCI POSITION')
# b. TSLA
cci_tsla_strat <- ifelse(cci_tsla_ts > 1,0,1)
for (i in 1 : length(Cl(TSLA))) {cci_tsla_strat[i] <- ifelse(cci_tsla_ts[i] == 1,1,ifelse(cci_tsla_ts[i] == -1,0,cci_tsla_strat[i-1]))
}
cci_tsla_strat[is.na(cci_tsla_strat)] <- 1
cci_tsla_stratcomp <- cbind(cci_tsla, cci_tsla_ts, cci_tsla_strat)
colnames(cci_tsla_stratcomp) <- c('CCI','CCI SIGNAL','CCI POSITION')
# c. NFLX
cci_nflx_strat <- ifelse(cci_nflx_ts > 1,0,1)
for (i in 1 : length(Cl(NFLX))) {cci_nflx_strat[i] <- ifelse(cci_nflx_ts[i] == 1,1,ifelse(cci_nflx_ts[i] == -1,0,cci_nflx_strat[i-1]))
}
cci_nflx_strat[is.na(cci_nflx_strat)] <- 1
cci_nflx_stratcomp <- cbind(cci_nflx, cci_nflx_ts, cci_nflx_strat)
colnames(cci_nflx_stratcomp) <- c('CCI','CCI SIGNAL','CCI POSITION')# ROC# a. AAPL
roc_aapl_strat <- ifelse(roc_aapl_ts > 1,0,1)
for (i in 1 : length(Cl(AAPL))) {roc_aapl_strat[i] <- ifelse(roc_aapl_ts[i] == 1,1,ifelse(roc_aapl_ts[i] == -1,0,roc_aapl_strat[i-1]))
}
roc_aapl_strat[is.na(roc_aapl_strat)] <- 1
roc_aapl_stratcomp <- cbind(roc_aapl, roc_aapl_ts, roc_aapl_strat)
colnames(roc_aapl_stratcomp) <- c('ROC(25)','ROC SIGNAL','ROC POSITION')
# b. TSLA
roc_tsla_strat <- ifelse(roc_tsla_ts > 1,0,1)
for (i in 1 : length(Cl(TSLA))) {roc_tsla_strat[i] <- ifelse(roc_tsla_ts[i] == 1,1,ifelse(roc_tsla_ts[i] == -1,0,roc_tsla_strat[i-1]))
}
roc_tsla_strat[is.na(roc_tsla_strat)] <- 1
roc_tsla_stratcomp <- cbind(roc_tsla, roc_tsla_ts, roc_tsla_strat)
colnames(roc_tsla_stratcomp) <- c('ROC(25)','ROC SIGNAL','ROC POSITION')
# c. NFLX
roc_nflx_strat <- ifelse(roc_nflx_ts > 1,0,1)
for (i in 1 : length(Cl(NFLX))) {roc_nflx_strat[i] <- ifelse(roc_nflx_ts[i] == 1,1,ifelse(roc_nflx_ts[i] == -1,0,roc_nflx_strat[i-1]))
}
roc_nflx_strat[is.na(roc_nflx_strat)] <- 1
roc_nflx_stratcomp <- cbind(roc_nflx, roc_nflx_ts, roc_nflx_strat)
colnames(roc_nflx_stratcomp) <- c('ROC(25)','ROC SIGNAL','ROC POSITION')# SMI# a. AAPL
smi_aapl_strat <- ifelse(smi_aapl_ts > 1,0,1)
for (i in 1 : length(Cl(AAPL))) {smi_aapl_strat[i] <- ifelse(smi_aapl_ts[i] == 1,1,ifelse(smi_aapl_ts[i] == -1,0,smi_aapl_strat[i-1]))
}
smi_aapl_strat[is.na(smi_aapl_strat)] <- 1
smi_aapl_stratcomp <- cbind(smi_aapl[,1],smi_aapl[,2],smi_aapl_ts,smi_aapl_strat)
colnames(smi_aapl_stratcomp) <- c('SMI','SMI(S)','SMI SIGNAL','SMI POSITION')
# b. TSLA
smi_tsla_strat <- ifelse(smi_tsla_ts > 1,0,1)
for (i in 1 : length(Cl(TSLA))) {smi_tsla_strat[i] <- ifelse(smi_tsla_ts[i] == 1,1,ifelse(smi_tsla_ts[i] == -1,0,smi_tsla_strat[i-1]))
}
smi_tsla_strat[is.na(smi_tsla_strat)] <- 1
smi_tsla_stratcomp <- cbind(smi_tsla[,1],smi_tsla[,2],smi_tsla_ts,smi_tsla_strat)
colnames(smi_tsla_stratcomp) <- c('SMI','SMI(S)','SMI SIGNAL','SMI POSITION')
# c. NFLX
smi_nflx_strat <- ifelse(smi_nflx_ts > 1,0,1)
for (i in 1 : length(Cl(NFLX))) {smi_nflx_strat[i] <- ifelse(smi_nflx_ts[i] == 1,1,ifelse(smi_nflx_ts[i] == -1,0,smi_nflx_strat[i-1]))
}
smi_nflx_strat[is.na(smi_nflx_strat)] <- 1
smi_nflx_stratcomp <- cbind(smi_nflx[,1],smi_nflx[,2],smi_nflx_ts,smi_nflx_strat)
colnames(smi_nflx_stratcomp) <- c('SMI','SMI(S)','SMI SIGNAL','SMI POSITION')# WPR# a. AAPL
wpr_aapl_strat <- ifelse(wpr_aapl_ts > 1,0,1)
for (i in 1 : length(Cl(AAPL))) {wpr_aapl_strat[i] <- ifelse(wpr_aapl_ts[i] == 1,1,ifelse(wpr_aapl_ts[i] == -1,0,wpr_aapl_strat[i-1]))
}
wpr_aapl_strat[is.na(wpr_aapl_strat)] <- 1
wpr_aapl_stratcomp <- cbind(wpr_aapl, wpr_aapl_ts, wpr_aapl_strat)
colnames(wpr_aapl_stratcomp) <- c('WPR(14)','WPR SIGNAL','WPR POSITION')
# b. TSLA
wpr_tsla_strat <- ifelse(wpr_tsla_ts > 1,0,1)
for (i in 1 : length(Cl(TSLA))) {wpr_tsla_strat[i] <- ifelse(wpr_tsla_ts[i] == 1,1,ifelse(wpr_tsla_ts[i] == -1,0,wpr_tsla_strat[i-1]))
}
wpr_tsla_strat[is.na(wpr_tsla_strat)] <- 1
wpr_tsla_stratcomp <- cbind(wpr_tsla, wpr_tsla_ts, wpr_tsla_strat)
colnames(wpr_tsla_stratcomp) <- c('WPR(14)','WPR SIGNAL','WPR POSITION')
# c. NFLX
wpr_nflx_strat <- ifelse(wpr_nflx_ts > 1,0,1)
for (i in 1 : length(Cl(NFLX))) {wpr_nflx_strat[i] <- ifelse(wpr_nflx_ts[i] == 1,1,ifelse(wpr_nflx_ts[i] == -1,0,wpr_nflx_strat[i-1]))
}
wpr_nflx_strat[is.na(wpr_nflx_strat)] <- 1
wpr_nflx_stratcomp <- cbind(wpr_nflx, wpr_nflx_ts, wpr_nflx_strat)
colnames(wpr_nflx_stratcomp) <- c('WPR(14)','WPR SIGNAL','WPR POSITION')# Trading Strategy Performance # Calculating Returns & setting Benchmark for companies
ret_aapl <- diff(log(Cl(AAPL)))
ret_tsla <- diff(log(Cl(TSLA)))
ret_nflx <- diff(log(Cl(NFLX)))
benchmark_aapl <- ret_aapl
benchmark_tsla <- ret_tsla
benchmark_nflx <- ret_nflx# SMA # 1. AAPL
sma_aapl_ret <- ret_aapl*sma_aapl_strat
sma_aapl_ret_commission_adj <- ifelse((sma_aapl_ts == 1|sma_aapl_ts == -1) & sma_aapl_strat != Lag(sma_aapl_ts), (ret_aapl-0.05)*sma_aapl_strat, ret_aapl*sma_aapl_strat)
sma_aapl_comp <- cbind(sma_aapl_ret, sma_aapl_ret_commission_adj, benchmark_aapl)
colnames(sma_aapl_comp) <- c('SMA','SMA Commission Adj','Apple Benchmark')
charts.PerformanceSummary(sma_aapl_comp, main = 'Apple SMA Performance')
sma_aapl_comp_table <- table.AnnualizedReturns(sma_aapl_comp)
# 2. TSLA
sma_tsla_ret <- ret_tsla*sma_tsla_strat
sma_tsla_ret_commission_adj <- ifelse((sma_tsla_ts == 1|sma_tsla_ts == -1) & sma_tsla_strat != Lag(sma_tsla_ts), (ret_tsla-0.05)*sma_tsla_strat, ret_tsla*sma_tsla_strat)
sma_tsla_comp <- cbind(sma_tsla_ret, sma_tsla_ret_commission_adj, benchmark_tsla)
colnames(sma_tsla_comp) <- c('SMA','SMA Commission Adj','Tesla Benchmark')
charts.PerformanceSummary(sma_tsla_comp, main = 'Tesla SMA Performance')
sma_tsla_comp_table <- table.AnnualizedReturns(sma_tsla_comp)
# 3. NFLX
sma_nflx_ret <- ret_nflx*sma_nflx_strat
sma_nflx_ret_commission_adj <- ifelse((sma_nflx_ts == 1|sma_nflx_ts == -1) & sma_nflx_strat != Lag(sma_nflx_ts), (ret_nflx-0.05)*sma_nflx_strat, ret_nflx*sma_nflx_strat)
sma_nflx_comp <- cbind(sma_nflx_ret, sma_nflx_ret_commission_adj, benchmark_nflx)
colnames(sma_nflx_comp) <- c('SMA','SMA Commission Adj','Netflix Benchmark')
charts.PerformanceSummary(sma_nflx_comp, main = 'Netflix SMA Performance')
sma_nflx_comp_table <- table.AnnualizedReturns(sma_nflx_comp)# Parabolic SAR # 1. AAPL
sar_aapl_ret <- ret_aapl*sar_aapl_strat
sar_aapl_ret_commission_adj <- ifelse((sar_aapl_ts == 1|sar_aapl_ts == -1) & sar_aapl_strat != Lag(sar_aapl_ts), (ret_aapl-0.05)*sar_aapl_strat, ret_aapl*sar_aapl_strat)
sar_aapl_comp <- cbind(sar_aapl_ret, sar_aapl_ret_commission_adj, benchmark_aapl)
colnames(sar_aapl_comp) <- c('SAR','SAR Commission Adj','Apple Benchmark')
charts.PerformanceSummary(sar_aapl_comp, main = 'Apple Parabolic SAR Performance')
sar_aapl_comp_table <- table.AnnualizedReturns(sar_aapl_comp)
# 2. TSLA
sar_tsla_ret <- ret_tsla*sar_tsla_strat
sar_tsla_ret_commission_adj <- ifelse((sar_tsla_ts == 1|sar_tsla_ts == -1) & sar_tsla_strat != Lag(sar_tsla_ts), (ret_tsla-0.05)*sar_tsla_strat, ret_tsla*sar_tsla_strat)
sar_tsla_comp <- cbind(sar_tsla_ret, sar_tsla_ret_commission_adj, benchmark_tsla)
colnames(sar_tsla_comp) <- c('SAR','SAR Commission Adj','Tesla Benchmark')
charts.PerformanceSummary(sar_tsla_comp, main = 'Tesla Parabolic SAR Performance')
sar_tsla_comp_table <- table.AnnualizedReturns(sar_tsla_comp)
# 3. NFLX
sar_nflx_ret <- ret_nflx*sar_nflx_strat
sar_nflx_ret_commission_adj <- ifelse((sar_nflx_ts == 1|sar_nflx_ts == -1) & sar_nflx_strat != Lag(sar_nflx_ts), (ret_nflx-0.05)*sar_nflx_strat, ret_nflx*sar_nflx_strat)
sar_nflx_comp <- cbind(sar_nflx_ret, sar_nflx_ret_commission_adj, benchmark_nflx)
colnames(sar_nflx_comp) <- c('SAR','SAR Commission Adj','Netflix Benchmark')
charts.PerformanceSummary(sar_nflx_comp, main = 'Netflix Parabolic SAR Performance')
sar_nflx_comp_table <- table.AnnualizedReturns(sar_nflx_comp)# CCI # 1. AAPL
cci_aapl_ret <- ret_aapl*cci_aapl_strat
cci_aapl_ret_commission_adj <- ifelse((cci_aapl_ts == 1|cci_aapl_ts == -1) & cci_aapl_strat != Lag(cci_aapl_ts), (ret_aapl-0.05)*cci_aapl_strat, ret_aapl*cci_aapl_strat)
cci_aapl_comp <- cbind(cci_aapl_ret, cci_aapl_ret_commission_adj, benchmark_aapl)
colnames(cci_aapl_comp) <- c('CCI','CCI Commission Adj','Apple Benchmark')
charts.PerformanceSummary(cci_aapl_comp, main = 'Apple CCI Performance')
cci_aapl_comp_table <- table.AnnualizedReturns(cci_aapl_comp)
# 2. TSLA
cci_tsla_ret <- ret_tsla*cci_tsla_strat
cci_tsla_ret_commission_adj <- ifelse((cci_tsla_ts == 1|cci_tsla_ts == -1) & cci_tsla_strat != Lag(cci_tsla_ts), (ret_tsla-0.05)*cci_tsla_strat, ret_tsla*cci_tsla_strat)
cci_tsla_comp <- cbind(cci_tsla_ret, cci_tsla_ret_commission_adj, benchmark_tsla)
colnames(cci_tsla_comp) <- c('CCI','CCI Commission Adj','Tesla Benchmark')
charts.PerformanceSummary(cci_tsla_comp, main = 'Tesla CCI Performance')
cci_tsla_comp_table <- table.AnnualizedReturns(cci_tsla_comp)
# 3. NFLX
cci_nflx_ret <- ret_nflx*cci_nflx_strat
cci_nflx_ret_commission_adj <- ifelse((cci_nflx_ts == 1|cci_nflx_ts == -1) & cci_nflx_strat != Lag(cci_nflx_ts), (ret_nflx-0.05)*cci_nflx_strat, ret_nflx*cci_nflx_strat)
cci_nflx_comp <- cbind(cci_nflx_ret, cci_nflx_ret_commission_adj, benchmark_nflx)
colnames(cci_nflx_comp) <- c('CCI','CCI Commission Adj','Netflix Benchmark')
charts.PerformanceSummary(cci_nflx_comp, main = 'Netflix CCI Performance')
cci_nflx_comp_table <- table.AnnualizedReturns(cci_nflx_comp)# ROC # 1. AAPL
roc_aapl_ret <- ret_aapl*roc_aapl_strat
roc_aapl_ret_commission_adj <- ifelse((roc_aapl_ts == 1|roc_aapl_ts == -1) & roc_aapl_strat != Lag(roc_aapl_ts), (ret_aapl-0.05)*roc_aapl_strat, ret_aapl*roc_aapl_strat)
roc_aapl_comp <- cbind(roc_aapl_ret, roc_aapl_ret_commission_adj, benchmark_aapl)
colnames(roc_aapl_comp) <- c('ROC','ROC Commission Adj','Apple Benchmark')
charts.PerformanceSummary(roc_aapl_comp, main = 'Apple ROC Performance')
roc_aapl_comp_table <- table.AnnualizedReturns(roc_aapl_comp)
# 2. TSLA
roc_tsla_ret <- ret_tsla*roc_tsla_strat
roc_tsla_ret_commission_adj <- ifelse((roc_tsla_ts == 1|roc_tsla_ts == -1) & roc_tsla_strat != Lag(roc_tsla_ts), (ret_tsla-0.05)*roc_tsla_strat, ret_tsla*roc_tsla_strat)
roc_tsla_comp <- cbind(roc_tsla_ret, roc_tsla_ret_commission_adj, benchmark_tsla)
colnames(roc_tsla_comp) <- c('ROC','ROC Commission Adj','Tesla Benchmark')
charts.PerformanceSummary(roc_tsla_comp, main = 'Tesla ROC Performance')
roc_tsla_comp_table <- table.AnnualizedReturns(roc_tsla_comp)
# 3. NFLX
roc_nflx_ret <- ret_nflx*roc_nflx_strat
roc_nflx_ret_commission_adj <- ifelse((roc_nflx_ts == 1|roc_nflx_ts == -1) & roc_nflx_strat != Lag(roc_nflx_ts), (ret_nflx-0.05)*roc_nflx_strat, ret_nflx*roc_nflx_strat)
roc_nflx_comp <- cbind(roc_nflx_ret, roc_nflx_ret_commission_adj, benchmark_nflx)
colnames(roc_nflx_comp) <- c('ROC','ROC Commission Adj','Netflix Benchmark')
charts.PerformanceSummary(roc_nflx_comp, main = 'Netflix ROC Performance')
roc_nflx_comp_table <- table.AnnualizedReturns(roc_nflx_comp)# SMI # 1. AAPL
smi_aapl_ret <- ret_aapl*smi_aapl_strat
smi_aapl_ret_commission_adj <- ifelse((smi_aapl_ts == 1|smi_aapl_ts == -1) & smi_aapl_strat != Lag(smi_aapl_ts), (ret_aapl-0.05)*smi_aapl_strat, ret_aapl*smi_aapl_strat)
smi_aapl_comp <- cbind(smi_aapl_ret, smi_aapl_ret_commission_adj, benchmark_aapl)
colnames(smi_aapl_comp) <- c('SMI','SMI Commission Adj','Apple Benchmark')
charts.PerformanceSummary(smi_aapl_comp, main = 'Apple SMI Performance')
smi_aapl_comp_table <- table.AnnualizedReturns(smi_aapl_comp)
# 2. TSLA
smi_tsla_ret <- ret_tsla*smi_tsla_strat
smi_tsla_ret_commission_adj <- ifelse((smi_tsla_ts == 1|smi_tsla_ts == -1) & smi_tsla_strat != Lag(smi_tsla_ts), (ret_tsla-0.05)*smi_tsla_strat, ret_tsla*smi_tsla_strat)
smi_tsla_comp <- cbind(smi_tsla_ret, smi_tsla_ret_commission_adj, benchmark_tsla)
colnames(smi_tsla_comp) <- c('SMI','SMI Commission Adj','Tesla Benchmark')
charts.PerformanceSummary(smi_tsla_comp, main = 'Tesla SMI Performance')
smi_tsla_comp_table <- table.AnnualizedReturns(smi_tsla_comp)
# 3. NFLX
smi_nflx_ret <- ret_nflx*smi_nflx_strat
smi_nflx_ret_commission_adj <- ifelse((smi_nflx_ts == 1|smi_nflx_ts == -1) & smi_nflx_strat != Lag(smi_nflx_ts), (ret_nflx-0.05)*smi_nflx_strat, ret_nflx*smi_nflx_strat)
smi_nflx_comp <- cbind(smi_nflx_ret, smi_nflx_ret_commission_adj, benchmark_nflx)
colnames(smi_nflx_comp) <- c('SMI','SMI Commission Adj','Netflix Benchmark')
charts.PerformanceSummary(smi_nflx_comp, main = 'Netflix SMI Performance')
smi_nflx_comp_table <- table.AnnualizedReturns(smi_nflx_comp)# WPR # 1. AAPL
wpr_aapl_ret <- ret_aapl*wpr_aapl_strat
wpr_aapl_ret_commission_adj <- ifelse((wpr_aapl_ts == 1|wpr_aapl_ts == -1) & wpr_aapl_strat != Lag(wpr_aapl_ts), (ret_aapl-0.05)*wpr_aapl_strat, ret_aapl*wpr_aapl_strat)
wpr_aapl_comp <- cbind(wpr_aapl_ret, wpr_aapl_ret_commission_adj, benchmark_aapl)
colnames(wpr_aapl_comp) <- c('WPR','WPR Commission Adj','Apple Benchmark')
charts.PerformanceSummary(wpr_aapl_comp, main = 'Apple WPR Performance')
wpr_aapl_comp_table <- table.AnnualizedReturns(wpr_aapl_comp)
# 2. TSLA
wpr_tsla_ret <- ret_tsla*wpr_tsla_strat
wpr_tsla_ret_commission_adj <- ifelse((wpr_tsla_ts == 1|wpr_tsla_ts == -1) & wpr_tsla_strat != Lag(wpr_tsla_ts), (ret_tsla-0.05)*wpr_tsla_strat, ret_tsla*wpr_tsla_strat)
wpr_tsla_comp <- cbind(wpr_tsla_ret, wpr_tsla_ret_commission_adj, benchmark_tsla)
colnames(wpr_tsla_comp) <- c('WPR','WPR Commission Adj','Tesla Benchmark')
charts.PerformanceSummary(wpr_tsla_comp, main = 'Tesla WPR Performance')
wpr_tsla_comp_table <- table.AnnualizedReturns(wpr_tsla_comp)
# 3. NFLX
wpr_nflx_ret <- ret_nflx*wpr_nflx_strat
wpr_nflx_ret_commission_adj <- ifelse((wpr_nflx_ts == 1|wpr_nflx_ts == -1) & wpr_nflx_strat != Lag(wpr_nflx_ts), (ret_nflx-0.05)*wpr_nflx_strat, ret_nflx*wpr_nflx_strat)
wpr_nflx_comp <- cbind(wpr_nflx_ret, wpr_nflx_ret_commission_adj, benchmark_nflx)
colnames(wpr_nflx_comp) <- c('WPR','WPR Commission Adj','Netflix Benchmark')
charts.PerformanceSummary(wpr_nflx_comp, main = 'Netflix WPR Performance')
wpr_nflx_comp_table <- table.AnnualizedReturns(wpr_nflx_comp)
翻译自: https://medium.com/swlh/creating-trading-strategies-and-backtesting-with-r-bc206a95da00
均线交易策略的回测 r
本文来自互联网用户投稿,该文观点仅代表作者本人,不代表本站立场。本站仅提供信息存储空间服务,不拥有所有权,不承担相关法律责任。如若转载,请注明出处:http://www.mzph.cn/news/389564.shtml
如若内容造成侵权/违法违规/事实不符,请联系多彩编程网进行投诉反馈email:809451989@qq.com,一经查实,立即删除!