熊猫烧香分析报告
目录 (Table of Contents)
- Introduction 介绍
- Overview 总览
- Variables 变数
- Interactions 互动互动
- Correlations 相关性
- Missing Values 缺失值
- Sample 样品
- Summary 摘要
介绍 (Introduction)
There are countless ways to perform exploratory data analysis (EDA) in Python (and in R). I do most of mine in the popular Jupyter Notebook. Once I realized there was a library that could summarize my dataset with just one line of code, I made sure to utilize it for every project, reaping countless benefits from the ease of this EDA tool. The EDA step should be performed first before executing any Machine Learning models for all Data Scientists, therefore, the kind and intelligent developers from Pandas Profiling [2] have made it easy to view your dataset in a beautiful format, while also describing the information well in your dataset.
在Python( 和R )中执行探索性数据分析(EDA)的方法有无数种。 我在流行的Jupyter笔记本电脑上做大多数事情。 一旦意识到有一个库可以用一行代码来总结我的数据集,我便确保将其用于每个项目,并从此EDA工具的易用性中获得了无数的收益。 在为所有数据科学家执行任何机器学习模型之前,应首先执行EDA步骤,因此, Pandas Profiling [2]的友善而又聪明的开发人员已轻松以美观的格式查看数据集,同时也很好地描述了信息在您的数据集中。
The Pandas Profiling report serves as this excellent EDA tool that can offer the following benefits: overview, variables, interactions, correlations, missing values, and a sample of your data. I will be using randomly generated data to serve as an example of this useful tool.
熊猫分析报告是一种出色的EDA工具,可提供以下好处:概述,变量,交互作用,相关性,缺失值和数据样本。 我将使用随机生成的数据作为此有用工具的示例。
总览 (Overview)

The overview tab in the report provides a quick glance at how many variables and observations you have or the number of rows and columns. It will also perform a calculation to see how many of your missing cells there are compared to the whole dataframe column. Additionally, it will point out duplicate rows as well and calculate that percentage. This tab is most similar to part of the describe function from Pandas, while providing a better user-interface (UI) experience.
报告中的“概述”选项卡可让您快速浏览一下您拥有多少变量和观测值,或者行和列的数量。 它还将执行计算,以查看与整个数据框列相比有多少个丢失的单元格。 此外,它还将指出重复的行并计算该百分比。 此选项卡与Pandas的describe函数的一部分最为相似,同时提供了更好的用户界面 ( UI )体验。
The overview is broken into dataset statistics and variable types. You can also refer to warnings and reproduction for more specific information on your data.
概述分为数据集统计信息和变量类型。 您还可以参考警告和复制以获取有关数据的更多特定信息。
I will be discussing variables, which are also referred to as columns or features of your dataframe
我将讨论变量,这些变量也称为数据框的列或特征
变数 (Variables)

To achieve more granularity in your descriptive statistics, the variables tab is the way to go. You can look at distinct, missing, aggregations or calculations like mean, min, and max of your dataframe features or variables. You can also see the type of data you are working with (i.e., NUM). Not pictured is when you click on ‘Toggle details’. This toggle prompts a whole plethora of more usable statistics. The details include:
为了使描述性统计信息更加精确,可以使用“变量”选项卡。 您可以查看数据框特征或变量的不同,缺失,聚合或计算,例如均值,最小值和最大值。 您还可以查看正在使用的数据类型( 即NUM )。 当您单击“ 切换详细信息 ”时,未显示图片。 此切换提示大量更多可用统计信息。 详细信息包括:
Statistics — quantile and descriptive
统计-分位数和描述性
quantile
分位数
Minimum
5th percentile
Q1
Median
Q3
95th percentile
Maximum
Range
Interquartile range (IQR)
descriptive
描述性的
Standard deviation
Coefficient of variation (CV)
Kurtosis
Mean
Median Absolute Deviation (MAD)
Skewness
Sum
Variance
Monotonicity
These statistics also provide similar information from the describe function I see most Data Scientists using today, however, there are a few more and it presents them in an easy-to-view format.
这些统计信息还提供了我今天看到的大多数数据科学家使用的describe函数的类似信息,但是,还有更多信息,并且以易于查看的格式显示。
Histograms
直方图
The histograms provide for an easily digestible visual of your variables. You can expect to see the frequency of your variable on the y-axis and fixed-size bins (bins=15 is the default) on the x-axis.
直方图为您的变量提供了易于理解的视觉效果。 你可以期望看到的在y轴变量的在x轴的频率和固定大小的块( 仓= 15是默认值 )。
Common Values
共同价值观
The common values will provide the value, count, and frequency that are most common for your variable.
公用值将提供最常用于变量的值,计数和频率。
Extreme Values
极端值
The extreme values will provide the value, count, and frequency that are in the minimum and maximum values of your dataframe.
极值将提供数据框的最小值和最大值中的值,计数和频率。
互动互动 (Interactions)

The interactions feature of the profiling report is unique in that you can choose from your list of columns to either be on the x-axis or y-xis provided. For example, pictured above is variable A against variable A, which is why you see overlapping. You can easily switch to other variables or columns to achieve a different plot and an excellent representation of your data points.
分析报告的交互功能是独特的,因为您可以从列列表中选择在提供的x轴还是y-xis上 。 例如,如上图所示, 变量A相对于变量A ,这就是为什么看到重叠的原因。 您可以轻松地切换到其他变量或列,以实现不同的图并很好地表示数据点。
相关性 (Correlations)

Sometimes making fancier or colorful correlation plots can be time-consuming if you make them from line-by-line Python code. However, with this correlation plot, you can easily visualize the relationships between variables in your data, which are also nicely color-coded. There are four main plots that you can display:
如果使用逐行的 Python代码进行绘制,有时制作更精美的彩色关联图可能会很耗时。 但是,使用此相关图,您可以轻松地可视化数据中变量之间的关系,这些变量也已进行了很好的颜色编码 。 您可以显示四个主要图表:
Pearson’s r
皮尔逊河
Spearman’s ρ
斯皮尔曼的ρ
Kendall’s τ
肯德尔的τ
Phik (φk)
皮克(φk)
You may only be used to one of these correlation methods, so the other ones may sound confusing or not usable. Therefore, the correlation plot also comes provided with a toggle for details onto the meaning of each correlation you can visualize — this feature really helps when you need a refresher on correlation, as well as when you are deciding between which plot(s) to use for your analysis
您可能只习惯了这些相关方法之一,因此其他方法可能听起来令人困惑或无法使用。 因此,相关情节还附带提供了一个切换为细节上可以直观的每个相关的含义-这个功能真的帮助,当你需要在相关复习,以及当你决定为与该阴谋( 县 )使用供您分析
缺失值 (Missing Values)

As you can see from the plot above, the report tool also includes missing values. You can see how much of each variable is missing, including the count, and matrix. It is a nice way to visualize your data before you perform any models with it. You would preferably want to see a plot like the above, meaning you have no missing values.
从上图可以看到,报告工具还包含缺失值。 您可以看到缺少每个变量的多少,包括计数和矩阵。 这是在执行任何模型之前可视化数据的好方法。 您最好希望看到上面的图,这意味着您没有缺失的值。
样品 (Sample)

Sample acts similarly to the head and tail function where it returns your dataframe’s first few rows or last rows. In this example, you can see the first rows and last rows as well. I use this tab when I want a sense of where my data started and where it ended — I recommend ranking or ordering to see more benefit out of this tab, as you can see the range of your data, with a visual respective representation.
Sample的行为类似于head和tail函数,它返回数据框的前几行或最后几行。 在此示例中,您还可以看到第一行和最后一行。 当我想了解我的数据的开始和结束位置时,可以使用此选项卡-我建议进行排序或排序,以便从该选项卡中获得更多好处,因为您可以看到数据的范围,并具有直观的外观。
摘要 (Summary)
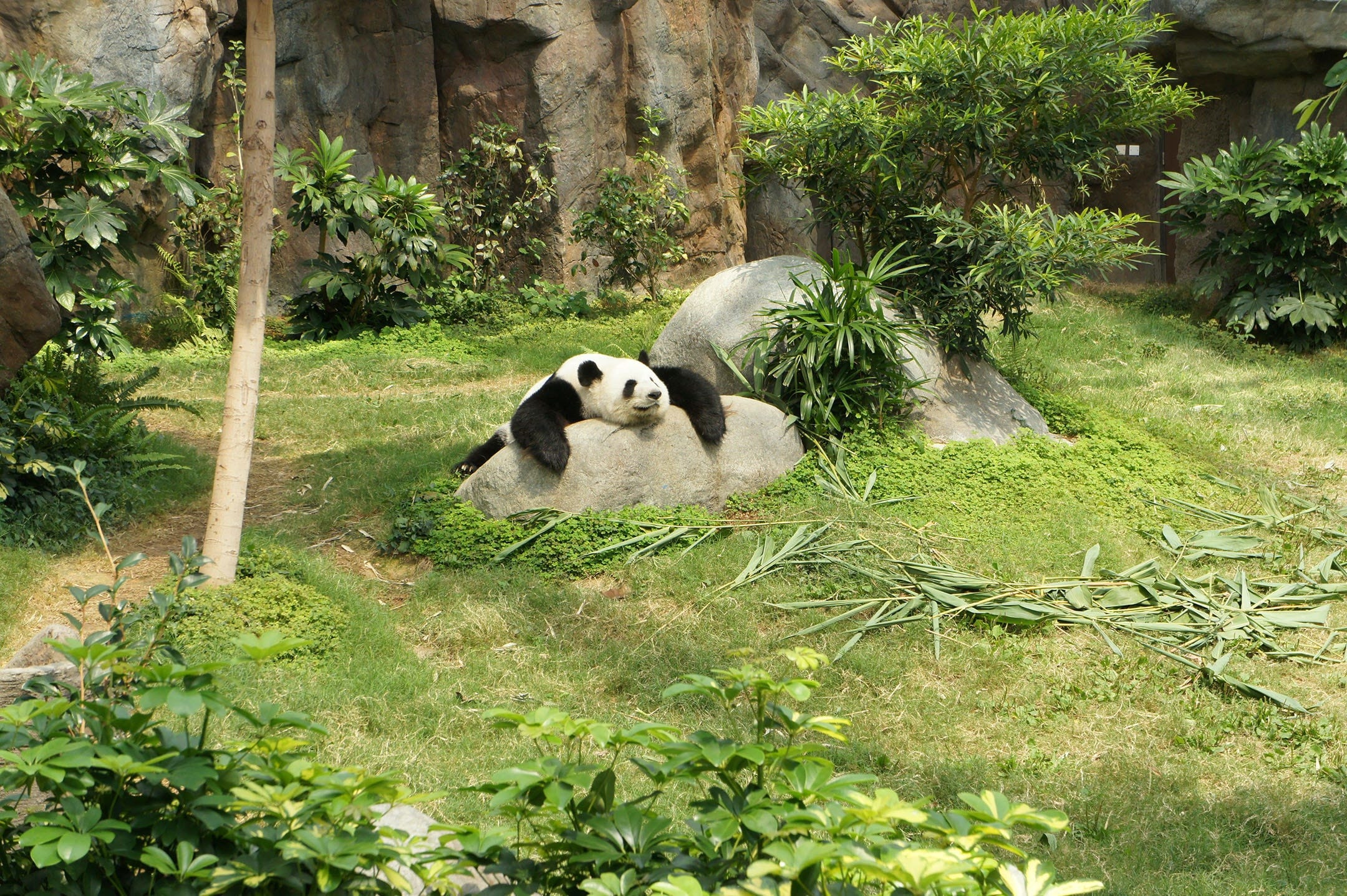
I hope this article provided you with some inspiration for your next exploratory data analysis. Being a Data Scientist can be overwhelming and EDA is often forgotten or not practiced as much as model-building. With the Pandas Profiling report, you can perform EDA with minimal code, providing useful statistics and visualizes as well. That way, you can focus on the fun part of Data Science and Machine Learning, the model process.
我希望本文能为您的下一个探索性数据分析提供一些启发。 身为数据科学家可能会令人不知所措,而EDA常常像建立模型一样被遗忘或未得到实践。 使用Pandas Profiling报告,您可以用最少的代码执行EDA,同时提供有用的统计信息并进行可视化。 这样,您就可以专注于数据科学和机器学习的有趣部分,即模型过程。
To summarize, the main features of Pandas Profiling report include overview, variables, interactions, correlations, missing values, and a sample of your data.
总之,Pandas Profiling报告的主要功能包括概述,变量,交互作用,相关性,缺失值以及数据样本。
Here is the code I used to install and import libraries, as well as to generate some dummy data for the example, and finally, the one line of code used to generate the Pandas Profile report based on your Pandas dataframe [10].
这是我用于安装和导入库以及为示例生成一些虚拟数据的代码,最后是用于基于您的Pandas数据框[10]生成Pandas Profile报告的一行代码。
# install library
#!pip install pandas_profilingimport pandas_profiling
import pandas as pd
import numpy as np# create data
df = pd.DataFrame(np.random.randint(0,200,size=(15, 6)), columns=list('ABCDEF'))# run your report!
df.profile_report()# I did get an error and had to reinstall matplotlib to fix
Please feel free to comment down below if you have any questions or have used this feature before. There is still some information I did not describe, but you can find more of that information on the link I provided from above.
如果您有任何疑问或以前使用过此功能,请在下面随意评论。 仍然有一些我没有描述的信息,但是您可以从上面提供的链接中找到更多的信息。
Thank you for reading, I hope you enjoyed!
谢谢您的阅读,希望您喜欢!
翻译自: https://towardsdatascience.com/the-best-exploratory-data-analysis-with-pandas-profiling-e85b4d514583
熊猫烧香分析报告
本文来自互联网用户投稿,该文观点仅代表作者本人,不代表本站立场。本站仅提供信息存储空间服务,不拥有所有权,不承担相关法律责任。如若转载,请注明出处:http://www.mzph.cn/news/389829.shtml
如若内容造成侵权/违法违规/事实不符,请联系多彩编程网进行投诉反馈email:809451989@qq.com,一经查实,立即删除!