青年报告
Youth-led media is any effort created, planned, implemented, and reflected upon by young people in the form of media, including websites, newspapers, television shows, and publications. Such platforms connect writers, artists, and photographers in the age range of 13–24 all around the globe and promote and defend a free youth press. Members of these platforms not only have the freedom to express their own opinions on various issues and topics but also represent various communities and let their voices be heard.
青年领导的媒体是年轻人以媒体的形式(包括网站,报纸,电视节目和出版物)创建,计划,实施和反思的任何努力。 这样的平台将全球13至24岁的作家,艺术家和摄影师联系起来,并促进和捍卫自由的青年报刊。 这些平台的成员不仅可以自由表达自己在各种问题和主题上的意见,而且可以代表各种社区并发表自己的声音。
Hence, such platforms prove to be a good source of data to understand and analyze youth aspirations across various parts of the globe. In the remaining sections, we will explain our methodology of data collection and will list down our results and insights derived from the analysis of various topics.
因此,这些平台被证明是理解和分析全球各地青年志向的良好数据来源。 在其余各节中,我们将解释我们的数据收集方法,并将列出我们的结果和从各种主题分析中得出的见解。
本节谈论什么? (What does the section talk about?)
This Section is overall given insights about the data was distributed over newspapers and articles, the insights and visualizations tell us about how youths are going on and how their sentiments change overtime period (Ranges from 2015–2020)
本节总体上给出了关于数据分布在报纸和文章上的见解,这些见解和可视化告诉我们有关青年的发展状况以及他们的情绪随时间变化的情况(2015-2020年的范围)
我们为什么选择这个主题? (Why did we choose this topic?)
This topic aims to analyze data from a different perspective i.e Outside Social media. This is the reason we choose this topic to scrape and analyze the data i.e present over there outside social media and we present our insights accordingly.
本主题旨在从不同角度(即外部社交媒体)分析数据。 这就是我们选择该主题来抓取和分析数据(即存在于外部社交媒体上的数据)的原因,我们相应地提出了自己的见解。
目标 (Objectives)
- To scrape and process News articles from different resources, to prepare it for sentiment analysis and topic modeling, in order to draw useful insights about the sentiment of the youth from it. 从不同的资源中抓取和处理新闻文章,以准备进行情感分析和主题建模,以便从中获得有关青年情感的有用见解。
- To conduct sentiment analysis, for understanding the youth sentiment better. 进行情绪分析,以更好地了解青年情绪。
- To collect the insights from all of these points and to visualize the results in a cogent manner for the audience. 从所有这些方面收集见解,并以令人信服的方式为观众呈现结果。
方法 (Methodology)
数据采集 (Data Collection)
To collect articles, we scraped data from various media platforms (ref. Table 1) using a scraper we made using BeautifulSoup and requests a library in Python. Lots of articles were scraped ranging from the year 1994 to 2020 and merged to a final dataset that we used for analysis. We also focused on extracting articles for certain categories, viz:
为了收集文章,我们使用了使用BeautifulSoup制作的抓取工具,从各种媒体平台(参见表1)抓取了数据,并请求使用Python库。 从1994年到2020年,我们刮掉了许多文章,并将其合并为我们用于分析的最终数据集。 我们还专注于提取某些类别的文章,即:
- Education 教育
- Environment & Climate 环境与气候
- Human Rights 人权
- COVID-19 新冠肺炎
- Politics 政治
- Health and Leisure 健康休闲
使用的工具: (Tools Used:)
For scraping data:
对于抓取数据:
- Beautiful soup 美丽的汤
- Requests 要求
- Selenium Selenium
For visualizing data:
为了可视化数据:
- Matplotlib Matplotlib
- Seaborn Seaborn
- Python-Plotly Python皮
- Matplotlib-Animations Matplotlib动画
- Tableau 画面
- Python Word Clouds Python文字云
For sentiment analysis:
对于情绪分析:
- Text Blob 文字斑点
- Empath Analysis 移情分析
- Region-Based Analysis 基于区域的分析
- Knowledge Graph 知识图
- Network Analysis 网络分析
数据预处理 (Data Preprocessing)
With all the articles scraped, next, we focused on preprocessing the articles. While preprocessing, one of our major challenges was to identify and remove promotional content from the articles. To start with, we removed all the URLs from the articles. Next, we identified the templates that each of the platforms used for advertisements or for promoting other articles and used regular expressions to identify and remove them from the articles. We then sent our articles through a basic preprocessing pipeline to change the case, stem, lemmatize and remove special characters and regular stopwords, etc. We also identified certain redundant words like journalism, etc. that didn’t add to the analysis and removed them from our dataset.
在抓取所有文章之后,接下来,我们将重点放在预处理文章上。 在进行预处理时,我们面临的主要挑战之一是从文章中识别并删除促销内容。 首先,我们从文章中删除了所有URL。 接下来,我们确定了每个平台用于广告或促销其他文章的模板,并使用正则表达式来标识它们并将其从文章中删除。 然后,我们通过基本的预处理流程发送文章,以更改大小写,词干,词形化并删除特殊字符和常规停用词等。我们还发现了某些未添加到分析中的多余词(例如新闻等),并将其删除从我们的数据集中。
Additionally, we also did a keyword analysis as a preprocessing step so as to ensure that we have everything ready before we start with our analysis. Next, we used Stanford’s NER and Python’s geopy library to identify locations with respect to the articles. Then, we used LDA and Empath based analysis for topic modeling and recognized 9 following topics:
此外,我们还将关键字分析作为预处理步骤,以确保在开始分析之前已准备就绪。 接下来,我们使用Stanford的NER和Python的geopy库来确定关于文章的位置。 然后,我们使用基于LDA和Empath的分析进行主题建模,并识别出以下9个主题:
Environment (Climate Change)
环境(气候变化)
Leadership & Politics (Democracy, Leadership)
领导与政治(民主,领导)
Health
健康
COVID-19
新冠肺炎
Education
教育
Technology
技术
Human Rights (LGBT, Black Lives Matter, Bullying)
人权(LGBT,重要的黑人生活,欺凌)
Terrorism and Violence
恐怖主义与暴力
Career and Employment
职业与就业
ENVIRONMENT ( Author: Mr. Mateus Broilo)
环境(作者:Mateus Broilo先生)
There is no question that the Environment is a key topic that gathers the concern of the whole society, from youngsters to adults, and to elders. However, the youth of today are the future of tomorrow and for this reason, they are the part of society that most probably will suffer the most in years to come. The environment can not be seen as a cultural movement, simply because it is not. But it must be seen as and dealt like a political movement and as an economical trend where most of the time it serves the will of powerful corporations.
毫无疑问,环境是一个关键话题,引起了整个社会的关注,从年轻人到成年人,再到老年人。 但是,今天的年轻人是明天的未来,因此,他们是社会的一部分,很可能会在未来几年遭受最大的痛苦。 不能仅仅因为环境就将其视为文化运动。 但是,必须将它视为一种政治运动,并将其视为一种经济趋势,在大多数情况下,它服务于强大企业的意愿。
The Word Cloud shows some of the most common and meaningful words related to the Environment topic analysis. Notice that words like climate, change, people, plastic, and others presented in Below Figure may be correlated to the basic concerns of the young people. And not surprisingly they appear as the most common words in over the 380 articles analyzed. Clearly “climate” and “change” are two pieces of a bigram. Climate is changing and that is a fact. “People” are part of the problem, but also can be the solution, mostly the youth. After all, the youth aspirations are a heat map towards where the world actually should be going to. And just for curiosity, have you ever found a “plastic” bottle on the beach? See Below Figures for more clarity.
词云显示与环境主题分析相关的一些最常见和最有意义的词。 请注意,下图中显示的诸如气候,变化,人,塑料等字眼可能与年轻人的基本关切相关。 毫不奇怪,它们在被分析的380篇文章中成为最常见的词。 显然,“气候”和“变化”是二元论的两个部分。 气候在变化,这是事实。 “人”是问题的一部分,但也可以是解决方案,主要是青年。 毕竟,青年的志向是世界应去往何处的热点图。 只是出于好奇,您是否曾经在海滩上找到过“塑料”瓶? 请参阅下面的图以更清楚。
One last analysis is to look for lexicons, in other words, to perform a text analysis across lexical categories. Here the main objective is to connect the text with a broad range of sentiments beyond positive, negative, and neutral, as shown in Figure 3.6.15. On the other hand in Figure 3.6.16 we see the most common levels in which the text articles can be categorized and in 3.6.17 the empath values associated with the most meaningful levels that impacts the environmental movement.
最后一种分析是寻找词典,换句话说,对词汇类别进行文本分析。 此处的主要目的是将文本与正面,负面和中立之外的广泛情感联系起来,如图3.6.15所示。 另一方面,在图3.6.16中,我们看到可以对文本文章进行分类的最常见级别,在3.6.17中,我们看到了与影响环境运动的最有意义的级别相关联的移情值。
2. CARRER AND EDUCATION (Author: Mr. Mario Vasquez Arias, Ms. Adelore Similoluwa Gloria)
2. 就业和 教育(作者:Mario Vasquez Arias先生,Adelore Similoluwa Gloria女士)
Education is one of the chosen categories and, at the same time, fundamental to this study because, if we talk about young people, it should not be lacking. Most young people are at some level of education, be it primary school, high school, or university. Therefore many young people spend a lot of time in educational sites becoming their second home and directly affecting the lives of each young person. As they are considered home, they reflect their personalities, concerns, and other feelings that the young person has at that time, so it is important to analyze this aspect.
教育是选择的类别之一,同时也是这项研究的基础,因为如果我们谈论年轻人,就不应该缺乏教育。 大多数年轻人受过一定程度的教育,无论是小学,高中还是大学。 因此,许多年轻人在教育场所花费大量时间成为他们的第二故乡,并直接影响每个年轻人的生活。 当他们被视为家时,它们反映了年轻人当时的性格,关注和其他感受,因此分析此方面很重要。
We can see in the word cloud that the two words that stand out the most are “school” and “students”, which allude precisely to what education represents, so it is obvious to expect those results. The word “time” also stands out, which we can infer is all the time that young people spend in school, which is a large part of the day for five days and for many years (these words are also visible in the graph). The word “high school” shows that the articles scraped and from which the analyses were made were more focused on a younger population and the target population, precisely. Another key word is “work”, which implies that young people not only study but also work, probably because of economic conditions. Another word that can be visualized is “immigrant”, which is an aspect that has been seen quite a lot in recent years, and education would not be exempt from this. The word “home problem” is seen in a smaller size but also important to note, as this reflects that sometimes students bring home problems to school, affecting their performance on grades and mental health.
我们可以在词云中看到,最突出的两个词是“学校”和“学生”,这恰好暗示了教育所代表的含义,因此可以预期得到这些结果。 “时间”一词也很突出,我们可以推断出年轻人在学校上的所有时间,这是一天中大部分时间,持续五天和很多年(这些单词在图表中也可见)。 “高中”一词表明,准确地刮掉并进行分析的文章更加侧重于年轻人口和目标人群。 另一个关键词是“工作”,这意味着年轻人不仅学习而且工作,这可能是由于经济状况所致。 可以形象化的另一个词是“移民”,这是近年来已经出现的很多方面,而且教育也不能免除这一点。 “家庭问题”一词看起来较小,但也要注意,因为这反映出有时学生将家庭问题带到学校,影响他们在年级和心理健康方面的表现。
Empath Analysis:
移情分析 :
The four values of empathy with the highest level are school (which is also the most repeated), reading, social networks, and holidays. In this range of time, it is what the young people but have emphasized in their thoughts, the school that already we said that it is like its second home; the habit of the reading that is something that has been increasing, or in physical or digital means; the social networks that these were a boom in the society and practically all the young people know and handle this type of technological services; and the vacations that are a few dates enough waited by the young people to enjoy the free time, their hobbies and the rest. Another value to highlight is technology, which appears at a lower level, but is still relevant for young people, due to the great advance of technology and the great proliferation of services and devices that are available to anyone, especially to this young population.
同理心最高的四个值是学校(也是重复次数最多的),阅读,社交网络和假期。 在这段时间里,正是年轻人在思想中强调的,我们已经说过的学校就像是第二故乡。 以某种形式或以物理或数字方式增加的阅读习惯; 这些社交网络正在社会中蓬勃发展,几乎所有年轻人都知道并使用这种技术服务; 还有一些假期足以让年轻人等着享受空闲时间,他们的业余爱好和其他时间。 值得强调的另一个价值是技术,由于技术的飞速发展以及任何人(尤其是这个年轻人口)可以使用的服务和设备的广泛普及,它的出现水平较低,但仍然与年轻人相关。
We can observe the average of the sentiment values for each year, where we have the highest peak in 2016 and the lowest in 2020, the latter could be due to the negative feelings generated by the pandemic generated by the coronavirus, which generates feelings of anxiety, confinement due to quarantine, loneliness, and depression, among others.
我们可以观察到每年的情绪平均值,其中我们在2016年达到最高峰,而在2020年达到最低峰,后者可能是由于冠状病毒引起的大流行所产生的负面情绪,从而产生了焦虑感,由于隔离,孤独和沮丧等原因导致的禁闭。
3. TERRORISM AND VIOLENCE (Author: Ms. Shanya Sharma)
3. 恐怖主义与暴力(作者:Shanya Sharma女士)
Sentiments Trends over time:
情绪随时间变化的趋势:
The dip in sentiments for 2019 can be associated to 2019 Oakland Gun Violence. The same can be inferred from the keywords extracted from 2019 terrorism articles.
2019年情绪下降可能与2019年奥克兰枪支暴力有关。 从2019年恐怖主义文章中提取的关键词可以推断出同样的情况。
Keywords like domestic violence can be seen for the year 2020 which can have a direct relation to COVID-19 and lockdown
在2020年可以看到像家庭暴力这样的关键词,它可能与COVID-19和锁定有直接关系
Police brutality is also a frequent keyword for 2020 data indicating that police brutality for imposing lockdown (for e.g. India) or that surrounding George Floyd’s case kept the youth in terror.
警察暴行也是2020年数据的常见关键词,表明警察因实施封锁而暴行(例如印度)或周围的乔治·弗洛伊德(George Floyd)案使年轻人感到恐怖。
4. HUMAN RIGHTS(Author: Mr. Opeyemi Fabiyi)
4 。 人权(作者:奥贝米·法比伊先生)
Keywords for certain locations
特定位置的关键字
Let’s Look the Emotion Trends:
让我们看看情绪趋势:
- A gradually increasing trend for negative emotions wrt human rights is concerning 与人权有关的负面情绪逐渐增加的趋势令人担忧
- Similarly, hate can be seen to be increasing gradually 同样,可以看出仇恨正在逐渐增加
- A higher value for positive emotions can indicate that the articles might also be hopeful about certain aspects 积极情绪的价值较高,表明该文章也可能对某些方面充满希望
- An analysis for finding the reason for the peak in 2018 showed that most articles written during these times were about how the writers want to fight the wrongdoings around them and indicated hope. 通过分析发现2018年达到峰值的原因,分析发现,这段时期内撰写的大多数文章都是关于作家如何应对周围的错误行为并表示希望的。
- Some common causes of concerns were: 引起关注的一些常见原因是:
Racism
种族主义
Violence
暴力
Poverty
贫穷
Immigration
出入境
Homophobia
恐同
6. Some concerning insights that came were:
6.一些有关的见解包括:
Youth in India is worried about Menstrual Hygiene
印度的年轻人担心月经卫生
Sex-Trafficking is a cause of concern in developed nations like the US.
在美国等发达国家,性贩运问题引起人们的关注。
SEX TRAFFICKING:
性交易:
- Almost all articles were from the United States 几乎所有文章都来自美国
- Most articles are written in 2019 大多数文章写于2019年
5. POLITICS(Author: Ms. Kriti Rai Saini)
5.政治(作者:Kriti Rai Saini女士)
Lexicons Associated with Positive.
Lexicons与阳性相关。
Lexicons Associated with negative.
Lexicons与阴性相关。
Let’s analyze yearly changes on sentiments over the years due to politics on different topics.
让我们分析一下多年来由于不同主题的政治而导致的情绪年度变化。


- The steep increase in disappointment due to the #MeToo movement, US Presidential results announcement, and the Facebook scandal. 由于#MeToo运动,美国总统结果公告和Facebook丑闻,令人失望的人数急剧增加。
- A steep decrease in hate in 2018 maybe due to the royal wedding and increase in 2020 due to the #BLM movement. 2018年的仇恨急剧下降可能是由于皇家婚礼,而#BLM运动则导致了2020年仇恨的急剧增加。
- Steep Increase in poor in 2020 due to the COVID pandemic. 由于COVID大流行,2020年的贫困人口将急剧增加。
- An increase in death in 2020 can be attributed to the COVID pandemic. 2020年死亡人数增加可归因于COVID大流行。
- Increase in anger due to constant discontent with the political situations over the years. 多年来,由于对政治局势的不满,导致愤怒增加。
- Increase in violence in 2020 due to lockdown imposed, the #BLM movement, and the peak in 2017 due to the #MeToo movement, mass violence in the USA(Texas, Las Vegas). 由于实施了封锁,#BLM运动,2020年的暴力事件增加;由于#MeToo运动,美国的大规模暴力(德克萨斯州拉斯维加斯),2017年的暴力事件增加。

- An increase in contentment and optimism in 2020 maybe because the pandemic has made people realize the importance of little things. The peak in contentment in 2017 due to the royal wedding. 2020年满足感和乐观情绪的提高可能是因为大流行使人们意识到了小事情的重要性。 由于皇家婚礼,2017年的满足感达到了顶峰。
- The peak in lexicon love in 2017 due to the royal wedding. 由于皇家婚礼,2017年词典爱情达到了顶峰。
- The peak in lexicon strength in 2017 due to the Women’s march wherein 1 million women stood up for women's rights and in 2020 due to the BLM movement. 在2017年,由于妇女大游行(其中有100万名妇女捍卫妇女权利)而导致词典力量达到顶峰,而在2020年,由于BLM运动,词典力量达到了顶峰。
6. COVID 19(Author: Ms. Monalisa Panda)
6. COVID 19(作者:Monalisa Panda女士)
In the above fig, we can see that there are only a few articles present in covid that is only the year 2020.
在上图中,我们可以看到,只有2020年,covid中只有几篇文章。
Mean Sentiments over different Months before lockdown vs after lockdown.
锁定前与锁定后不同月份的平均情绪。
Word clouds of all the articles based on the topic COVID-19:
基于主题COVID-19的所有文章的词云:
Based on Positive Sentiments:
基于积极情绪:
So these are listed as positive sentiments on the topic of COVID-19, mostly the words detected are:
因此,这些被列为COVID-19主题的积极情绪,大部分检测到的单词是:
People, Time: Most people get time to spend with their families and Relatives.
人,时间:大多数人有时间陪伴家人和亲戚。
Based on Negative Sentiments:
基于负面情绪:
So here in this word cloud, we can see that misinformation and racism, discrimination is some of the negative key holders in the case of the COVID topic.
因此,在这个词云中,我们可以看到,就COVID主题而言,错误信息和种族主义,歧视是负面因素的一部分。
Racism
种族主义
The peak in the negative emotions can be associated with US Presidential Elections 2016
负面情绪的高峰可能与2016年美国总统大选有关
Fear wrt racism was gradually decreasing but saw a slight rise in 2020
恐惧种族主义正在逐渐减少,但在2020年会略有上升
Region-based Positive and Negative sentiments on the topic of COVID:
基于区域的正面和负面情绪,涉及COVID:
Positive Sentiments:
积极情绪:

Negative Sentiments Regions:
负面情绪区域:
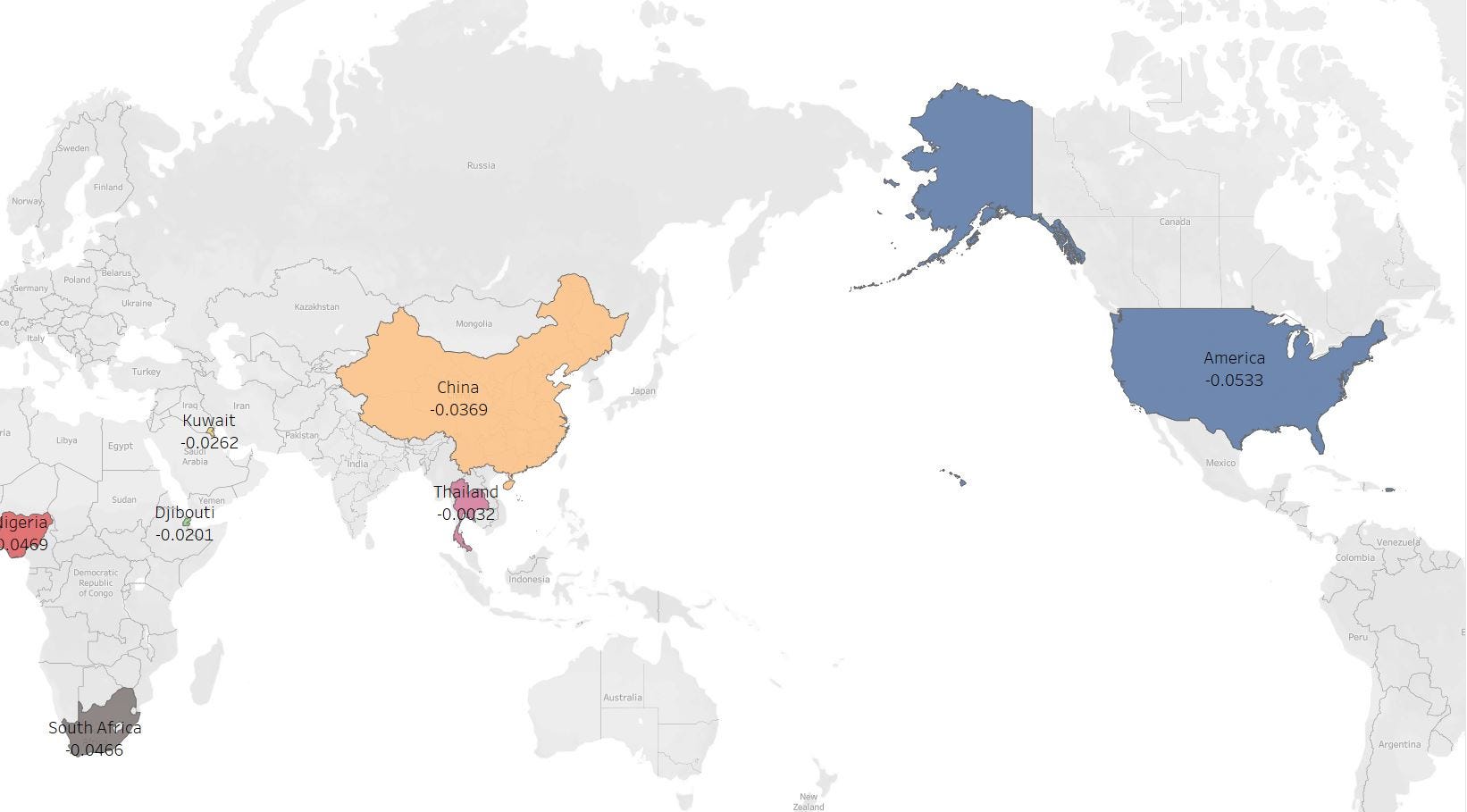
So these are the Whole analysis with all the topics mentioned in the Top. Once again I would like to thank all Omdena to give this Wonderful Opportunity to work on this project.
因此,这些是整体分析,上面列出了所有主题。 我要再次感谢所有Omdena给予这个工作的美好机会。
To visit for Upcoming Projects Go to Omdena
要访问即将进行的项目,请访问Omdena
Thank You!
谢谢!
Monalisa Panda
蒙娜丽莎·熊猫(Monalisa Panda)
翻译自: https://medium.com/omdena/understanding-youths-sentiments-c25ccbdb5702
青年报告
本文来自互联网用户投稿,该文观点仅代表作者本人,不代表本站立场。本站仅提供信息存储空间服务,不拥有所有权,不承担相关法律责任。如若转载,请注明出处:http://www.mzph.cn/news/389819.shtml
如若内容造成侵权/违法违规/事实不符,请联系多彩编程网进行投诉反馈email:809451989@qq.com,一经查实,立即删除!