mad离群值
An outlier is a data point in a data set that is distant from all other observations. A data point that lies outside the overall distribution of the dataset. Or in a layman term, we can say, an outlier is something that behaves differently from the combination/collection of the data.
离群值是数据集中的一个数据点,该数据点与所有其他观察值相距较远。 位于数据集总体分布之外的数据点。 或用外行术语来说,离群值是与数据的组合/收集不同的行为。
Outliers can be very informative about the subject-area and data collection process. It’s essential to understand how outliers occur and whether they might happen again as a normal part of the process or study area. To understand outliers, we need to go through these points:
异常值可能对主题区域和数据收集过程很有帮助。 了解离群值如何发生以及在过程或研究区域的正常部分是否会再次发生离群值至关重要。 要了解异常值,我们需要经历以下几点:
- what causes the outliers? 是什么导致异常值?
- Impact of the outlier 离群值的影响
- Methods to Identify outliers 识别异常值的方法
是什么导致异常值? (What causes the outliers?)
Before dealing with the outliers, one should know what causes them. There are three causes for outliers — data entry/An experiment measurement errors, sampling problems, and natural variation.
在处理异常值之前,应该知道是什么导致了异常值。 造成异常的原因有三点:数据输入/实验测量错误,采样问题和自然变化。
- Data entry /An experimental measurement error 数据输入/实验测量错误
An error can occur while experimenting/entering data. During data entry, a typo can type the wrong value by mistake. Let us consider a dataset of age, where we found a person age is 356, which is impossible. So this is a Data entry error.
实验/输入数据时可能会发生错误。 在数据输入期间,错字可能会错误输入错误的值。 让我们考虑年龄的数据集,我们发现一个人的年龄是356,这是不可能的。 因此,这是一个数据输入错误。

These types of errors are easy to identify. If you determine that an outlier value is an error, we can fix this error by deleting the data point because you know it’s an incorrect value.
这些类型的错误很容易识别。 如果您确定异常值是错误的,我们可以通过删除数据点来解决此错误,因为您知道该值是错误的。
2. Sampling problems
2.抽样问题
Outliers can occur while collecting random samples. Let us consider an example where we have records of bone density of various subjects, but there is an unusual growth of bone in a subject, after analyzing this has been discovered that the subject had diabetes, which affects bone health. The goal was to model bone density growth in girls with no health conditions that affect bone growth. Since the data is not a part of the target population so we will not consider this.
收集随机样本时可能会出现异常值。 让我们考虑一个示例,在该示例中,我们记录了各个受试者的骨密度,但是在分析了该受试者患有糖尿病并影响骨骼健康之后,该受试者的骨骼异常生长。 目的是在没有影响骨骼生长的健康状况的女孩中模拟骨骼密度的增长。 由于数据不是目标人群的一部分,因此我们不会考虑这一点。
3. Natural variation
3.自然变异
Suppose we need to check the reliability of a machine. The normal process includes standard materials, manufacturing settings, and conditions. If something unusual happens during a portion of the study, such as a power failure or a machine setting drifting off the standard value, it can affect the products. These abnormal manufacturing conditions can cause outliers by creating products with atypical strength values. Products manufactured under these unusual conditions do not reflect your target population of products from the normal process. Consequently, you can legitimately remove these data points from your dataset.
假设我们需要检查机器的可靠性。 正常过程包括标准材料,制造设置和条件。 如果在研究的一部分过程中发生异常情况,例如电源故障或机器设置偏离标准值,则可能会影响产品。 这些异常的制造条件可能会通过创建具有非典型强度值的产品而导致异常值。 在这些异常条件下制造的产品不能反映正常过程中目标产品的数量。 因此,您可以合法地从数据集中删除这些数据点。
离群值的影响 (Impact of the outlier)
Outliers can change the results of the data analysis and statistical modeling. Following are some impacts of outliers in the data set:
离群值可以更改数据分析和统计建模的结果。 以下是数据集中异常值的一些影响:
- It may cause a significant impact on the mean and the standard deviation 可能会对平均值和标准偏差产生重大影响
- If the outliers are non-randomly distributed, they can decrease normality 如果离群值是非随机分布的,则它们可能会降低正态性
- They can bias or influence estimates that may be of substantive interest 它们可能会偏向或影响可能具有实质意义的估计
- They can also impact the basic assumption of Regression, ANOVA, and other statistical model assumptions. 它们还会影响回归,ANOVA和其他统计模型假设的基本假设。
To understand the impact deeply, let’s take an example to check what happens to a data set with and without outliers in the data set.
为了深入了解其影响,让我们举一个例子来检查在数据集中有无异常时数据集会发生什么情况。
Let’s examine what can happen to a data set with outliers. For the sample data set:
让我们研究带有异常值的数据集会发生什么。 对于样本数据集:
1, 1, 2, 2, 2, 2, 3, 3, 3, 4, 4
1,1,2,2,2,2,3,3,3,4,4
We find the following mean, median, mode, and standard deviation:
我们发现以下平均值,中位数,众数和标准差:
Mean = 2.58
均值= 2.58
Median = 2.5
中位数= 2.5
Mode = 2
模式= 2
Standard Deviation = 1.08
标准偏差= 1.08
If we add an outlier to the data set:
如果我们向数据集添加离群值:
1, 1, 2, 2, 2, 2, 3, 3, 3, 4, 4, 400
1,1,2,2,2,2,3,3,3,4,4,400
The new values of our statistics are:
我们的统计信息的新值是:
Mean = 35.38
均值= 35.38
Median = 2.5
中位数= 2.5
Mode = 2
模式= 2
Standard Deviation = 114.74
标准偏差= 114.74
As you can see, having outliers often has a significant effect on your mean and standard deviation.
如您所见,离群值通常会对平均值和标准差产生重大影响。
识别异常值的方法 (Methods to Identify outliers)
There are various ways to identify outliers in a dataset, following are some of them:
在数据集中识别异常值的方法有多种,以下是其中的一些方法:
- Sorting the data 排序数据
- Using graphical Method 使用图形方法
- Using z score 使用z分数
- Using the IQR interquartile range 使用IQR四分位距
排序数据 (Sorting the data)
Sorting the dataset is the simplest and effective method to check unusual value. Let us consider an example of age dataset:
对数据集进行排序是检查异常值的最简单有效的方法。 让我们考虑年龄数据集的示例:
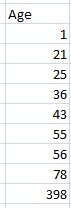
In the above dataset, we have sort the age dataset and get to know that 398 is an outlier. Sorting data method is most effective on the small dataset.
在上面的数据集中,我们对年龄数据集进行了排序,并且知道398是一个离群值。 排序数据方法对小型数据集最有效。
使用图形方法 (Using graphical Method)
We can detect outliers with the help of graphical representation like Scatter plot and Boxplot.
我们可以借助散点图和Boxplot等图形表示来检测异常值。

1. Scatter Plot
1.散点图
Scatter plots often have a pattern. We call a data point an outlier if it doesn’t fit the pattern. Here we have a scatter plot of Weight vs height. Notice how two of the points don’t fit the pattern very well. There is no special rule that tells us whether or not a point is an outlier in a scatter plot. When doing more advanced statistics, it may become helpful to invent a precise definition of “outlier”.
散点图通常具有某种模式。 如果数据点不适合模式,我们称其为离群值 。 这是重量与高度的散点图。 请注意,有两个点不太适合该模式。 没有特殊的规则可以告诉我们点在散点图中是否是异常值。 在进行更高级的统计时,发明精确定义“异常值”可能会有所帮助。

2. Box-Plot
2.箱线图
Box-plot is one of the most effective ways of identifying Outliers in a dataset. When reviewing a box plot, an outlier is defined as a data point that is located outside the box of the box plot. As seen in the box plot of bill vs days. Box-Plot uses the Interquartile range(IQR) to detect outliers.
箱线图是识别数据集中异常值的最有效方法之一。 查看箱形图时,离群值定义为位于箱形图框外部的数据点。 如票据与天数的方框图所示。 箱线图使用四分位间距(IQR)来检测离群值。
使用z分数 (Using z-score)

Z-score (also called a standard score) gives you an idea of how many standard deviations away a data point is from the mean.. But more technically it’s a measure of how many standard deviations below or above the population mean a raw score is.
Z分数(也称为标准分数 )使您了解一个数据点与平均值之间有多少标准偏差。但是从技术上讲,它衡量的是低于或高于总体的多少标准偏差表示原始分数是多少。 。
Z score = (x -mean) / std. deviation
Z分数=(x-均值)/ std。 偏差
In a normal distribution, it is estimated that
在正态分布中,估计
68% of the data points lie between +/- 1 standard deviation.
68%的数据点位于+/- 1标准偏差之间。
95% of the data points lie between +/- 2 standard deviation.
95%的数据点在+/- 2标准偏差之间。
99.7% of the data points lie between +/- 3 standard deviation.
99.7%的数据点位于+/- 3标准偏差之间。
Formula for Z score = (Observation — Mean)/Standard Deviation
Z分数的公式=(观测值–平均值)/标准差
z = (X — μ) / σ
z =(X —μ)/σ
Let us consider a dataset:
让我们考虑一个数据集:


使用IQR四分位距 (Using the IQR interquartile range)

Interquartile range(IQR), is just the width of the box in the box-plot which can be used as a measure of how spread out the values are. An outlier is any value that lies more than one and a half times the length of the box from either end of the box.
四分位数间距(IQR)只是箱形图中箱形的宽度,可用作度量值分布的程度。 离群值是从框的两端到框长度的一半以上的任何值。
Steps
脚步
- Arrange the data in increasing order 按升序排列数据
- Calculate first(q1) and third quartile(q3) 计算第一个(q1)和第三个四分位数(q3)
- Find interquartile range (q3-q1) 查找四分位数范围(q3-q1)
- Find lower bound q1*1.5 求下界q1 * 1.5
- Find upper bound q3*1.5 求上限q3 * 1.5
Anything that lies outside of lower and upper bound is an outlier
上下限以外的任何东西都是异常值
Let us take the same example as of Z-score:
让我们以与Z分数相同的示例为例:


As you can see we have found Lower and upper values that is: 7.5 and 19.5, so anything that lies outside these values is an outlier.
如您所见,我们发现下限值和上限值分别是:7.5和19.5,因此超出这些值的任何值都是异常值。
This is all we have about outliers, I hope you enjoyed reading. Thank you
这就是关于异常值的全部内容,希望您喜欢阅读。 谢谢
翻译自: https://medium.com/analytics-vidhya/its-all-about-outliers-cbe172aa1309
mad离群值
本文来自互联网用户投稿,该文观点仅代表作者本人,不代表本站立场。本站仅提供信息存储空间服务,不拥有所有权,不承担相关法律责任。如若转载,请注明出处:http://www.mzph.cn/news/389822.shtml
如若内容造成侵权/违法违规/事实不符,请联系多彩编程网进行投诉反馈email:809451989@qq.com,一经查实,立即删除!