vc6.0 绘制散点图
Scatterplots are one of the most popular visualization techniques in the world. Its purposes are recognizing clusters and correlations in ‘pairs’ of variables. There are many variations of scatter plots. We will look at some of them.
散点图是世界上最流行的可视化技术之一。 其目的是识别变量“对”中的聚类和相关性。 散点图有很多变体。 我们将研究其中的一些。
Strip Plots
带状图
Scatter plots in which one attribute is categorical are called ‘strip plots’. Since it is hard to see the data points when we plot the data points as a single line, we need to slightly spread the data points, you can check the above and we can also divide the data points based on the given label.
其中一种属性是分类的散点图称为“条形图”。 由于在将数据点绘制为单线时很难看到数据点,因此我们需要稍微散布数据点,您可以检查上述内容,也可以根据给定的标签划分数据点。
Scatterplot Matrices (SPLOM)
散点图矩阵(SPLOM)
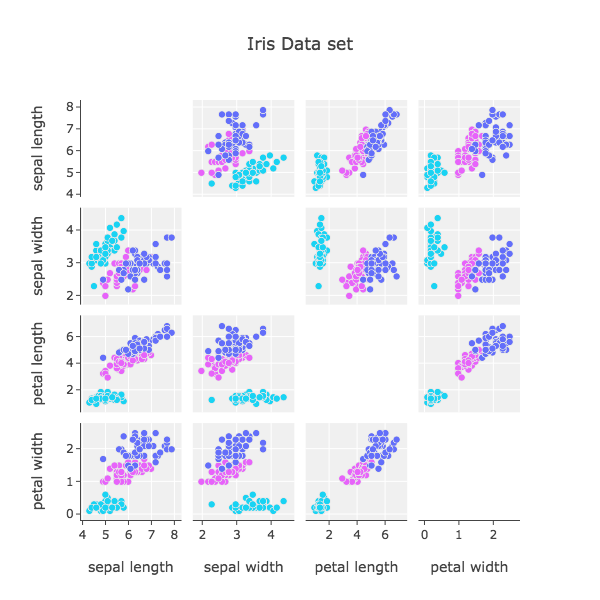
SPLOM produce scatterplots for all pairs of variables and place them into a matrix. Total unique scatterplots are (p²-p)/2. The diagonal is filled with KDE or histogram most of the time. As you can see, there is an order of scatterplots. Does the order matter? It cannot affect the value of course but it can affect the perception of people.
SPLOM为所有变量对生成散点图,并将它们放入矩阵中。 总唯一散点图为(p²-p)/ 2。 对角线大部分时间都充满KDE或直方图。 如您所见,有一个散点图顺序。 顺序重要吗? 它不会影响课程的价值,但会影响人们的感知。

Therefore we need to consider the order of it. Peng suggests the ordering that similar scatterplots are located close to each other in his work in 2004 [Peng et al. 2004]. They distinguish between high-cardinality and low cardinality(number of possible values > number of points means high cardinality.) and sort low-cardinality by a number of values. They rate the ordering of high-cardinality dimensions based on their correlation. Pearson Correlation Coefficient is used for sorting.
因此,我们需要考虑它的顺序。 Peng建议在2004年的工作中将相似的散点图放置在彼此附近的顺序[Peng等。 2004]。 它们区分高基数和低基数(可能值的数量>点数表示高基数),并通过多个值对低基数进行排序。 他们根据它们的相关性对高基数维度的排序进行评分。 皮尔逊相关系数用于排序。

We find all other pairs of x,y scatter plots with clutter measure. It calculates all correlation and compares it with each pair (x,y ) of high-cardinality dimensions. If its results are smaller than the threshold we choose that scatter plot as an important one. However, it takes a lot of computing power because its big-o-notation is O(p² * p!). They suggest random swapping, it chooses the smallest one and keeps it and again and again.
我们发现所有其他对具有散乱度量的x,y散点图。 它计算所有相关并将其与高基数维的每对(x,y)进行比较。 如果其结果小于阈值,则选择该散点图作为重要散点图。 但是,由于它的big-o表示法是O(p²* p!),因此需要大量的计算能力。 他们建议随机交换,它选择最小的交换并一次又一次地保留。
Selecting Good Views
选择好的观点
Correlation is not enough to choose the nice scatterplots when we are trying to find out the cluster based on the given label or we can get the label from clustering.
当我们尝试根据给定标签找出聚类时,或者仅从聚类中获取标签时,相关性不足以选择合适的散点图。
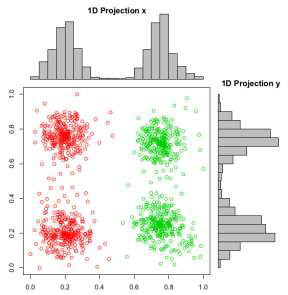
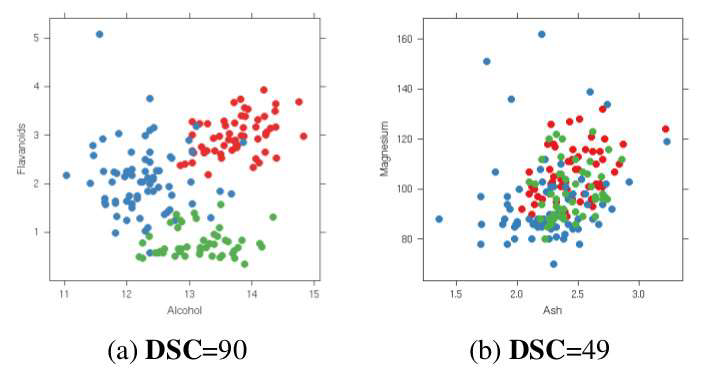
If you don’t have given labels in the left graph, you can pick x-axis projection or y-axis projection because there are no many differences but there are labels. Therefore, we can know the x-axis projection is correct., DSC is introduced with respect to this view that the method checks how good our scatterplot is. More good separation, more good scatterplots.
如果在左图中没有给出标签,则可以选择x轴投影或y轴投影,因为它们之间没有太多差异,但是有标签。 因此,我们可以知道x轴投影是正确的。为此,介绍了DSC,该方法检查了散点图的质量。 更好的分离,更好的散点图。
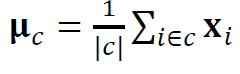

First of all, we calculate the center of each cluster and measure the distance between each data point and each cluster center. If the distance from its own cluster is shorter than other clusters distance, we increase the cardinality and we normalized it by the number of clusters and multiply 100. This method is similar to the k-means clustering method. Since it only considers distance, it has a limitation to applying.
首先,我们计算每个聚类的中心并测量每个数据点与每个聚类中心之间的距离。 如果距其自身群集的距离短于其他群集的距离,我们将增加基数,并通过群集数对其进行归一化并乘以100。此方法类似于k均值群集方法。 由于仅考虑距离,因此在应用方面存在局限性。
Distribution Consistency (DC)
分配一致性(DC)
DC is the upgrade(?) version of DSC. DC measures the score based on penalizing local entropy in high-density regions. DSC assumes the particular cluster shapes but DC does not assume the shapes.
DC是DSC的升级版本。 DC基于惩罚高密度区域中的局部熵来测量分数。 DSC假定特定的群集形状,但DC不假定这些形状。
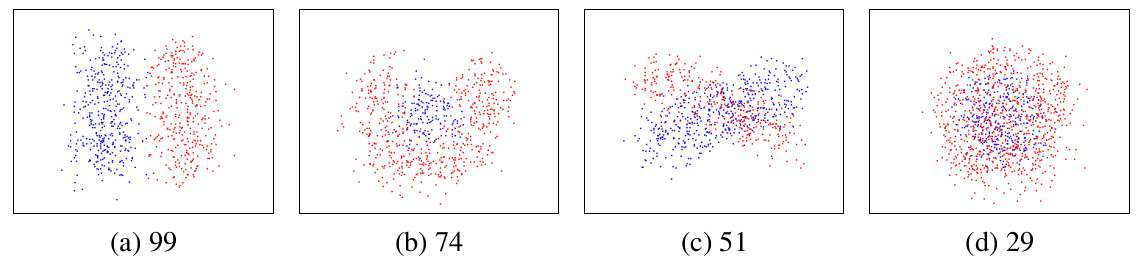

This equation is from information theory and it considers how much information in a specific distribution. The data should be estimated using KDE before we apply the entropy function, p(x,y) means the KDE. This equation means it gives smaller(Look at the minus) when the region we measure is mixed with other clusters and its minimum is 0 and the maximum is log2|C|.
该方程式来自信息理论,它考虑特定分布中有多少信息。 在应用熵函数之前,应使用KDE估算数据,p(x,y)表示KDE。 该方程式意味着当我们测量的区域与其他簇混合且其最小值为0且最大值为log2 | C |时,它的值较小(看一下负值)。


We calculated the entropy with KDE and we don’t want to calculate the whole region at the same weight because there are many vacant regions. Finally, we normalize the results. This gives the DC score. We can choose scatterplots based on thresholds that we can choose.
我们使用KDE来计算熵,我们不想以相同的权重来计算整个区域,因为有许多空置区域。 最后,我们将结果标准化。 这给出了DC得分。 我们可以根据选择的阈值来选择散点图。
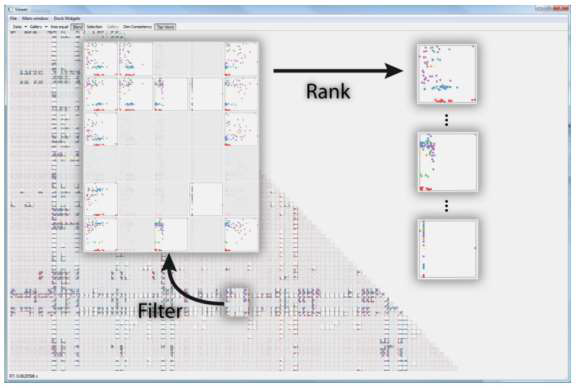
This dataset is from the WHO, 194 countries, 159 attributes, and 6 HIV risk groups. They focus on DC > 80 and they can eliminate 97% of the plots. It is a highly efficient method.
该数据集来自WHO,194个国家,159个属性和6个HIV风险组。 他们专注于DC> 80,并且可以消除97%的地块。 这是一种高效的方法。
Other than these methods that it only considers the clusters, there are many ways to consider other specific patterns, e.g. fraction of outliers, sparsity, convexity, and e.t.c. You can take a look at [Wilkinson et al. 2006]. PCA also can be used as an alternative way to group similar plots together.
除了仅考虑聚类的这些方法以外,还有许多方法可以考虑其他特定模式,例如,异常值的分数,稀疏性,凸度等。您可以看一下[Wilkinson等。 2006]。 PCA也可以用作将相似地块组合在一起的替代方法。
SPLOM Navigation
SPLOM导航

Since the SPLOM shares one axis with the neighboring plots, it is possible to project on to 3D space.
由于SPLOM与相邻的图共享一个轴,因此可以投影到3D空间。
The limitation of scatterplots: Overdraw
散点图的局限性:透支

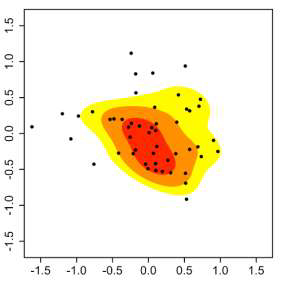
Too many data points lead to overdraw. We can solve this with KDE but it becomes no longer see individual points. The second problem is high dimensional data because it gives too many scatterplots. We discussed the solution of the second problem. Now we are going to look at the first problem.
太多的数据点导致透支。 我们可以使用KDE解决此问题,但不再看到单个点。 第二个问题是高维数据,因为它提供了太多的散点图。 我们讨论了第二个问题的解决方案。 现在我们要看第一个问题。
Splatterplots
飞溅图

Splatterplots properly combine the KDE and Scatterplots. The high-density region is represented by colors and the low-density region is represented by a single data point. We need to choose a proper kernel width for KDE. Splatterplots define the kernel width in screen space, how many data points in the unit screen space. However, we need to choose the threshold by ourselves.
Splatterplots正确地将KDE和Scatterplots结合在一起。 高密度区域由颜色表示,低密度区域由单个数据点表示。 我们需要为KDE选择合适的内核宽度。 Splatterplots定义屏幕空间中的内核宽度,即单位屏幕空间中有多少个数据点。 但是,我们需要自己选择阈值。
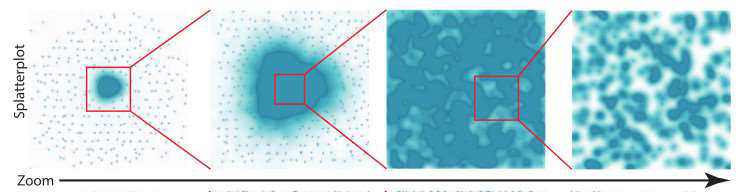
If clusters are mixed, then colors are matter. High luminance and saturation can cause the miss perception that people can recognize the mixed cluster as a different cluster. Therefore, we need to reduce the saturation and luminance to indicate it is mixed clusters.
如果群集混合在一起,那么颜色就很重要。 高亮度和饱和度可能会导致人们误以为人们会将混合群集识别为另一个群集。 因此,我们需要降低饱和度和亮度以表明它是混合簇。
This post is published on 9/2/2020.
此帖发布于2020年9月2日。
翻译自: https://medium.com/@jeheonpark93/vc-everything-about-scatter-plots-467f80aec77c
vc6.0 绘制散点图
本文来自互联网用户投稿,该文观点仅代表作者本人,不代表本站立场。本站仅提供信息存储空间服务,不拥有所有权,不承担相关法律责任。如若转载,请注明出处:http://www.mzph.cn/news/389587.shtml
如若内容造成侵权/违法违规/事实不符,请联系多彩编程网进行投诉反馈email:809451989@qq.com,一经查实,立即删除!