普里姆从不同顶点出发
绘制大流行时期社区的风险群图:以布宜诺斯艾利斯为例 (Map Risk Clusters of Neighbourhoods in the time of Pandemic: a case of Buenos Aires)
介绍 (Introduction)
Every year is unique and particular. But, 2020 brought the world the special planetary pandemic challenge of COVID-19. It spread and penetrated rapidly into different parts of the globe. And, the autonomous city of Buenos Aires (CABA: Ciudad Autonoma de Buenos Aires) is not an exception.
每年都是独一无二的。 但是,2020年给世界带来了COVID-19的特殊行星大流行挑战。 它Swift传播并渗透到全球各地。 而且,布宜诺斯艾利斯自治市(CABA:布宜诺斯艾利斯自治城市)也不例外。
In this particular setting, in order to craft the settings for my capstone project, I contemplated a hypothetical corporate client in the food industry (catering business) from abroad (The Client), that is planning to relocate their representative family to the city of Buenos Aires (CABA) for their future entry into Argentina once the pandemic-related restrictions are lifted. Since this would be its very first entry to Buenos Aires, the city is still an unknown territory for the Client.
在这个特殊的环境中,为了完善我的顶峰项目的环境,我考虑了一个来自国外(客户)食品行业(餐饮业务)的假设企业客户,该公司计划将其代表家庭搬到布宜诺斯艾利斯市一旦取消与大流行有关的限制,Aires(CABA)便会在未来进入阿根廷。 由于这将是它第一次进入布宜诺斯艾利斯,因此该城市对于客户来说仍然是一个未知的领域。
Very concerned with the two risks — the general security risk (crime) and the pandemic risk (COVID-19) — the Client wants to exclude high risk neighbourhoods in the selection of the location for the plan. In addition, the Client wants to capture the characteristics of neighbourhoods based on popular commercial venue categories such as restaurants, shops, and sports facilities. In this context, the Client hired me as an independent data analyst to conduct a preliminary research for its future plan.
客户非常关注这两种风险-一般安全风险(犯罪)和大流行风险(COVID-19)-客户希望在选择计划的地点时排除高风险社区。 此外,客户希望根据受欢迎的商业场所类别(例如餐厅,商店和体育设施)来捕捉社区的特征。 在这种情况下,客户聘请我担任独立数据分析师,以对其未来计划进行初步研究。
The Client stressed that this is the first-round preliminary analysis for a further extended study for business expansion. And based on the finding from this preliminary analysis, the Client wants to explore the scope of the future analysis. Simply put, the Client wants to conduct the preliminary analysis within a short period of time under a small budget to taste the flavour of the subject.
客户强调,这是为进一步扩展业务扩展研究而进行的第一轮初步分析。 并且,基于此初步分析的结果,客户希望探索未来分析的范围。 简而言之,客户希望在短时间内以少量预算进行初步分析,以品尝主题的味道。
The Client sets the following three objectives for this preliminary assignment.
客户为此初步任务设定以下三个目标。
- Identify outlier high risk neighbourhoods (the Outlier Neighbourhood/Cluster) in terms of these two risks — the general security risk (crime) and the pandemic risk (COVID-19). 从这两个风险(一般安全风险(犯罪)和大流行风险(COVID-19))中识别异常高风险社区(异常社区/集群)。
- Segment non-outlier neighbourhoods into several clusters (the Non-Outlier Clusters) and rank them based on a single quantitative risk metric (a compound risk metric of the general security risk and the pandemic risk). 将非离群的邻域划分为多个群集(非离群的群集),并基于单个定量风险度量(一般安全风险和大流行风险的复合风险度量)对它们进行排名。
- Use Foursquare API to characterize the Non-Outlier Neighbourhoods regarding popular venues. And if possible, segment Non-Outlier Neighbourhoods according to Foursquare venue profiles. 使用Foursquare API来描述有关受欢迎场所的非离群社区。 并且,如果可能,请根据Foursquare场地配置文件对非离群区域进行细分。
The autonomous city of Buenos Aires (CABA) is a densely populated city: the total population of approximately 3 million in the area of 203 km2. And each neighbourhood has its own distinct size of area and population. The city is divided into 48 administrative division, aka ‘barrios’, to which I will refer simply as ‘neighbourhoods’ in this report.
布宜诺斯艾利斯自治市(CABA)是一个人口稠密的城市:总人口约300万,面积203平方公里。 每个邻域都有其自己独特的面积和人口规模。 该市分为48个行政区,又名“ barrios”,在本报告中,我将其简称为“社区”。
The Client expressed their concern about the effect of the variability of population density among neighbourhoods. These two risks of the Client’s concern — the general security risk (crime) and the pandemic risk (COVID-19) — are likely affected by the population density profiles. Especially, the fact that ‘social distancing’ is a key to the prevention of COVID-19 suggests that population density is a significant attribute for the pandemic risk. In other words, the higher the population density, the higher the infection rate. The similar can be true for the general security risk. Obviously, this preconception needs to be assessed based on the actual data in the course of the project. This needs to be kept in mind for the analysis. Nevertheless, the Client ask me to scale risk metrics by ‘population density’ for the first round of the project.
客户对邻里人口密度变化的影响表示关注。 客户关注的这两个风险(一般安全风险(犯罪)和大流行风险(COVID-19))可能会受到人口密度状况的影响。 特别是,“社会隔离”是预防COVID-19的关键这一事实表明,人口密度是大流行风险的重要属性。 换句话说,人口密度越高,感染率越高。 对于一般的安全风险也是如此。 显然,需要根据项目过程中的实际数据来评估这种先入之见。 分析时必须牢记这一点。 但是,客户要求我在项目的第一轮中按“人口密度”来衡量风险指标。
Overall, the Client demonstrated high enthusiasm about Machine Learning and requested me to use machine learning models to achieve all these three objectives aforementioned.
总体而言,客户表现出了对机器学习的高度热情,并要求我使用机器学习模型来实现上述所有三个目标。
That is the background (business problem) scenario for this capstone project. On one hand, the scenario setting is totally hypothetical. On the other hand, the project handles real data.
这是此顶点项目的背景(业务问题)方案。 一方面,方案设置完全是假设的。 另一方面,项目处理实际数据。
Cut a long story short, for these three objectives presented above, I performed three different clustering machine-learning models. And I got three different lessons out of them. All of them are valuable. And in Discussion section of this article I will stress these different implications from the perspective of Data Science project management.
简而言之,对于上述三个目标,我执行了三种不同的集群机器学习模型。 我从中学到了三堂课。 所有这些都是有价值的。 在本文的“ 讨论”部分,我将从数据科学项目管理的角度强调这些不同的含义。
For now, I will invite you to walk through the process of the analysis.
现在,我将邀请您逐步进行分析。
The code of the project could be viewed in the following link of my GitHub repository:
可以在我的GitHub存储库的以下链接中查看项目的代码:
· Code: https://github.com/Hyper-Phronesis/Capstone-1/blob/master/Capstone%20Three%20Different%20Lessons%20from%20Three%20Different%20Clusterings.ipynb
·代码: https : //github.com/Hyper-Phronesis/Capstone-1/blob/master/Capstone%20Three%20Different%20Lessons%20from%20Three%20Different%20Clusterings.ipynb
Now, let’s start.
现在,让我们开始。
业务理解与分析方法 (Business Understanding and Analytical Approach)
At the beginning of a Data Science project, we need to clarify the following two basic questions
在数据科学项目开始时,我们需要澄清以下两个基本问题
- what needs to be solved. (Business Understanding) 需要解决的问题。 (业务理解)
- what kind of approach we need to make in order to achieve the objective. (Analytical Approach) 为了达到目标,我们需要采取哪种方法。 (分析方法)
For the case of this project, the Client already has specified both. What the Client wants are risk profiling, venue profiling, and clustering of neighbourhoods. These are all about analysis of the status quo, in other words, descriptive analysis; or potentially, it might involve diagnostic (what happened or what are happening). In other words, the Client is not asking for a forecast (predictive analysis) or how to solve the problem (prescriptive analysis) — at least at this preliminary stage. These navigate the overall direction of our analysis.
对于此项目,客户端已经指定了两者。 客户需要的是风险剖析,场所剖析和社区聚类。 这些都是关于现状的分析,换句话说就是描述性分析。 或可能涉及诊断(发生了什么或正在发生什么)。 换句话说,至少在这个初步阶段,客户并没有要求进行预测(预测分析)或如何解决问题(描述性分析)。 这些将指导我们分析的总体方向。
Now, all clear. Let’s mover to the next. Now, we start talking about data.
现在,一切都清楚了。 让我们前进到下一个。 现在,我们开始讨论数据。
A.数据部分 (A. Data Section)
A1。 资料需求: (A1. Data Requirements:)
By an analogy to cooking, Data Requirements is like a recipe, what ingredients we would need for cooking the dish: thus, what kind of data we would need for the analysis. The three objectives set by the Client determine the data requirements as follow:
类似于烹饪,“数据需求”就像一个食谱,说明我们烹饪菜肴所需的食材:因此,我们需要哪种数据进行分析。 客户设定的三个目标确定数据要求如下:
(1) Basic information about the neighbourhoods in Buenos Aires.
(1)关于布宜诺斯艾利斯居民区的基本信息。
- The area and the population for each neighbourhood 每个社区的面积和人口
- The geographical coordinates to determine the administrative border of each neighbourhood (for map visualization) 确定每个邻域的行政边界的地理坐标(用于地图可视化)
(2) Risk statistics:
(2)风险统计:
For the first and the second objectives, I would need to gather the following historical statistics to construct a compound risk metric to profile neighbourhoods from the perspectives of both the general insecurity risk (crime) and the pandemic risk (COVID-19).
对于第一个和第二个目标,我将需要收集以下历史统计数据,以从一般不安全风险(犯罪)和大流行风险(COVID-19)的角度构建复合风险度量标准,以对街区进行概要分析。
- general security risk statistics (crime incidences) by neighbourhoods 社区的一般安全风险统计(犯罪发生率)
- pandemic risk statistics (COVID-19 confirmed cases) by neighbourhoods 社区的大流行风险统计(COVID-19确诊病例)
(3) Foursquare Data:
(3)Foursquare数据:
For the third objective, the Client requires me to specifically use Foursquare API in order to characterise each Non-Outlier Neighbourhood.
对于第三个目标,客户要求我专门使用Foursquare API来表征每个非离群社区。
A2。 数据源 (A2. Data Sources)
Based on the data requirements, I explored the publicly available data. Then, I encountered the following relevant sources.
根据数据需求,我探索了公开可用的数据。 然后,我遇到了以下相关资源。
(1) Basic info of the neighbourhoods of CABA:
(1)CABA社区的基本信息:
the area and the population of all the relevant neighbourhoods from Wikipedia: https://en.wikipedia.org/wiki/Neighbourhoods_of_Buenos_Aires
维基百科上所有相关社区的面积和人口: https : //en.wikipedia.org/wiki/Neighbourhoods_of_Buenos_Aires
The city government of Buenos Aires provides a GeoJson file that contains the geographical coordinates which defines the administrative boundary of Barrios (the neighbourhoods) of Buenos Aires. https://data.buenosaires.gob.ar/dataset/barrios/archivo/1c3d185b-fdc9-474b-b41b-9bd960a3806e
布宜诺斯艾利斯市政府提供了一个GeoJson文件,其中包含地理坐标,该地理坐标定义了布宜诺斯艾利斯Barrios(社区)的行政边界。 https://data.buenosaires.gob.ar/dataset/barrios/archivo/1c3d185b-fdc9-474b-b41b-9bd960a3806e
(2) Historical risk statistics.
(2)历史风险统计。
Crime Statistics: A csv file which is compiled and uploaded by Rama in his GitHub depository: https://github.com/ramadis/delitos-caba/releases/download/3.0/delitos.csv
犯罪统计数据:一个由Rama在其GitHub存储库中编译并上传的csv文件: https : //github.com/ramadis/delitos-caba/releases/download/3.0/delitos.csv
COVID-19 Statistics: the city government’s website provides the COVID-19 statistics by neighbourhood: https://cdn.buenosaires.gob.ar/datosabiertos/datasets/salud/casos-covid-19/casos_covid19.xlsx
COVID-19统计信息:市政府的网站按邻居提供COVID-19统计信息: https ://cdn.buenosaires.gob.ar/datosabiertos/datasets/salud/casos-covid-19/casos_covid19.xlsx
(3) Foursquare Data for Popular Venues by Neighbourhood:
(3)各地区热门场所的Foursquare数据:
As per the Client’s requirement, I would specifically use Foursquare API in order to characterise each Non-Outlier Neighbourhood.
根据客户的要求,我将专门使用Foursquare API来表征每个非离群社区。
A3。 数据采集 (A3. Data Collection)
What follow now are data collection, data understanding, and data preparation. These parts altogether usually occupy a majority of time for the project, e.g. in a range of 60–70%.
现在,接下来是数据收集,数据理解和数据准备。 这些部分通常总共占项目的大部分时间,例如占60-70%。
For this article, I would compress the description of these time-consuming parts, by only outlining highlights.
对于本文,我将仅概述重点内容来压缩这些耗时部分的描述。
After downloading all the relevant data from the data sources above, I have made data reconciliation — cleaning data and transforming it in a coherent format. Thereafter, I consolidated all the relevant data into two datasets: “Risk Profile of Neighbourhoods” dataset and “Foursquare Venue Profile” dataset. The first 5rows of each dataset are presented below to illustrate their components.
从上面的数据源下载了所有相关数据之后,我进行了数据对帐-清理数据并将其转换为一致的格式。 之后,我将所有相关数据合并为两个数据集:“街区风险概况”数据集和“四方场地概况”数据集。 下面介绍了每个数据集的前5行,以说明它们的组成。
The first 5 rows of “Risk Profile of Neighbourhoods”:
“邻里风险概况”的前5行:

The first 5 rows of “Foursquare Venue Profile”:
“四方场地简介”的前5行:

Here is an outline of data limitation below.
以下是数据限制的概述。
(1) Crime Statistics: “Crime Severity Score”
(一)犯罪统计:“犯罪等级”
The compiled crime data covers only the period between Jan 1, 2016 and Dec 31, 2018. For the purpose of the project, I would make an assumption that the data during the available period would be good enough to serve a representative proxy for the risk characteristic of each neighbourhood.
汇总的犯罪数据仅涵盖2016年1月1日至2018年12月31日期间。就本项目而言,我假设可用期间的数据足以为风险提供代表性代表每个社区的特征。
The original crime statistics had 7 crime categories. They were weighted according to the severity of crime category and transformed to generate one single metric “Crime Severity Score”.
原始犯罪统计数据有7种犯罪类别。 根据犯罪类别的严重程度对它们进行加权,然后转换为一个度量“犯罪严重度评分”。
(2) COVID-19 Statistics: “COVID-19 Confirmed Cases”
(2)COVID-19统计:“ COVID-19确诊病例”
In order to measure the pandemic risk, I simply extracted the cumulative confirmed cases of COVID-19 for each neighbourhood. I did not net out the recovered cases from the data. Thus, the COVID-19 statistics in this analysis is a gross figure. My assumption here is that the gross data will proxy the empirical risk profile of COVID-19 infection.
为了衡量大流行的风险,我只提取了每个社区累积的确诊的COVID-19病例。 我没有从数据中扣除恢复的案件。 因此,此分析中的COVID-19统计数据为毛值。 我在这里的假设是,总数据将替代COVID-19感染的经验风险概况。
(3) Foursquare Data:
(3)Foursquare数据:
Foursquare API allows the user to explore venues within a user specified radius from one single location point. In other words, the user needs to specify the following parameters:
Foursquare API允许用户从一个单一位置点探索用户指定半径内的场地。 换句话说,用户需要指定以下参数:
- The geographical coordinates of one single starting point 一个单一起点的地理坐标
- ‘radius’: The radius to set the geographical scope of the query. 'radius':设置查询地理范围的半径。
This imposes a critical constraint in exploring venues within a neighbourhood from corner to corner. Since there is no uniformity in the area size among neighbourhoods, a compromise would be inevitable, while we want to capture the venue profile of a neighbourhood from corner to corner within its geographical border. Thus, the dataset that I would analyse for Foursquare venue analysis would be a geographically restrained sample set. I will use geopy’s Nominatim to obtain the representative single location point for each Neighbourhood.
这在探索社区内各个角落的场所时施加了严格的约束。 由于各社区之间的面积大小并不一致,因此在我们希望捕获某个社区在其地理边界内从一个角落到另一个角落的场地概况时,将不可避免地要做出折衷。 因此,我将对Foursquare场所分析进行分析的数据集将是一个受地理约束的样本集。 我将使用geopy的Nominatim为每个街区获得代表性的单个位置点。
A4。 数据理解 (A4. Data Understanding)
By now, the required data has been collected and reconciled. By an analogy to cooking, I have already cleaned and chopped the required ingredients according to the cook book. Now, I need to check the characteristics of the prepared ingredients: if they are representative of what we expected according to the cook book or othewise. Analogously, in this step of ‘data understanding’, I need to get an insight about the given data.
到现在为止,所需的数据已被收集和核对。 打个比方,我已经按照烹饪书清洗并切碎了所需的食材。 现在,我需要检查准备好的食材的特性:它们是否代表我们根据烹饪书或其他所期望的内容。 类似地,在“数据理解”这一步骤中,我需要对给定数据有一个见解。
Repeatedly, I consolidated all the relevant data into two datasets: “Risk Profile of Neighbourhoods” dataset and “Foursquare Venue Profile” dataset. Let me analyse one by one.
我反复地将所有相关数据合并为两个数据集:“街区风险概况”数据集和“四方场地概况”数据集。 让我一一分析。
(1) “Risk Profile of Neighbourhoods” dataset:
(1)“邻里风险概况”数据集:
For data understanding, there are several basic tools that helps us shape insights about the data distribution. And I performed the following three basic visualizations and generated one basic descriptive statistics:
为了了解数据,有几种基本工具可帮助我们形成有关数据分布的见解。 我执行了以下三个基本可视化,并生成了一个基本的描述统计数据:
a) Scatter Matrix:
a)散布矩阵:
The scatter matrix below displays two types of distribution:
下面的分散矩阵显示两种分布类型:
- the individual distribution of each feature variable on the diagonal cells; 每个特征变量在对角线上的单独分布;
- the pair-wise distribution of data points for two feature variables. 两个特征变量的数据点的成对分布。
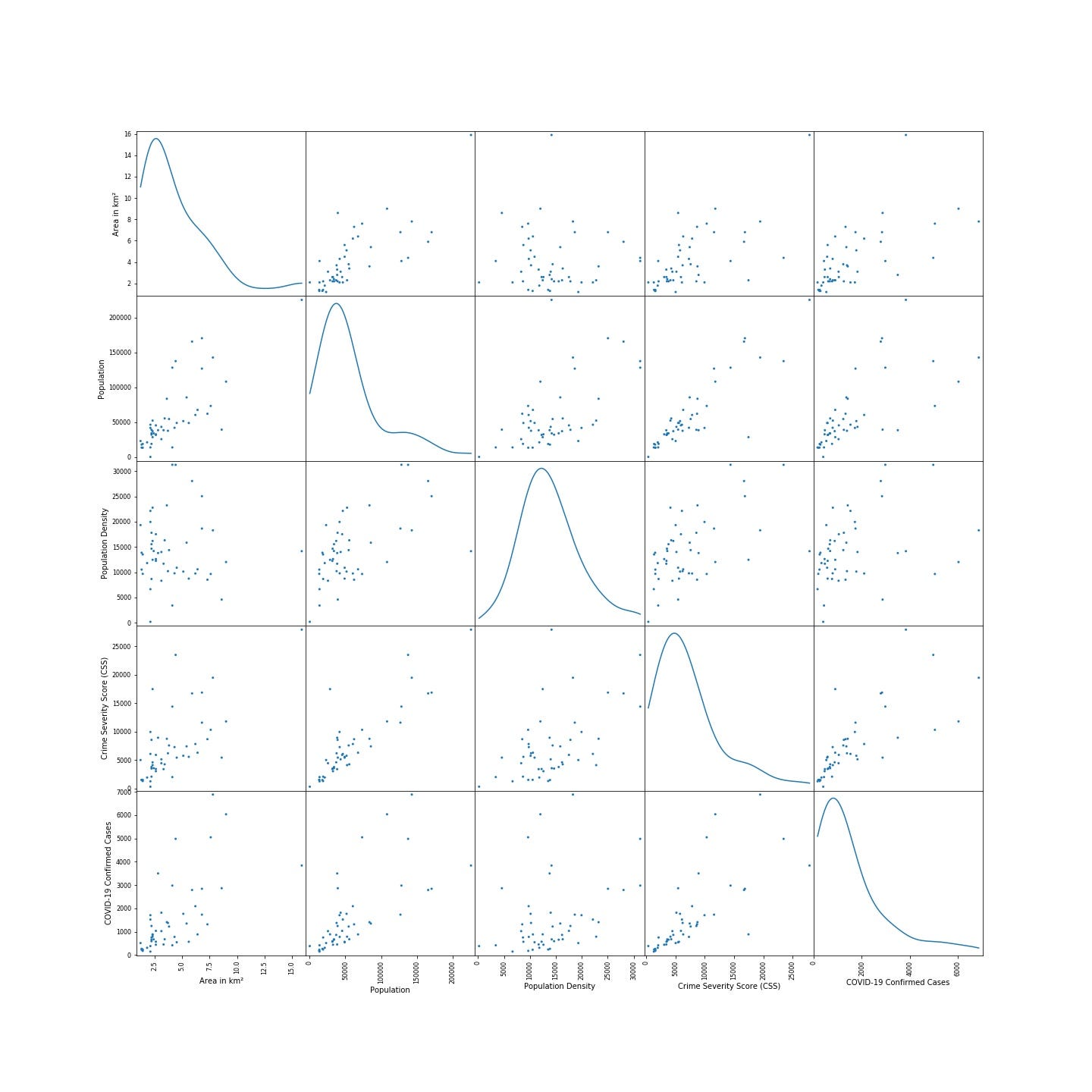
Here are some insights that I can derived from the scatter plot:
以下是我可以从散点图中得出的一些见解:
- On the diagonal cells of the scatter matrix, all the data except ‘population density’ demonstrate highly skewed individual distributions, suggesting the presence of outliers. 在散点图矩阵的对角线上,除“人口密度”外,所有数据均显示出高度偏斜的个体分布,表明存在异常值。
- In the off-diagonal cells, the most of the pair-wise plots suggest positive correlations in one way or another: except ‘population density’ with the area size and ‘COVID-19 Confirmed Cases’. 在非对角线细胞中,大多数成对图以一种或另一种方式表明正相关:除了“人口密度”与面积大小和“ COVID-19确诊病例”。
b) Correlation Matrix:
b)相关矩阵:
In order to quantitatively capture the second insight above in one single table, I plotted the correlation matrix below.
为了在一个表格中定量地获得上述第二个见解,我在下面绘制了相关矩阵。

Overall, “population density” stands out in the sense that it demonstrates relatively lower correlation with these two risk-metrics. On the other hand, population demonstrates the highest correlation with these two risk-metrics. This would raise a question: which feature — ‘area’, ‘population’ or ‘population density’ — would be the best to scale these two risk-metrics, ‘Crime Severity Score (CSS)’ and ‘COVID-19 Confirmed Cases’? This question needs to be reserved for a suggestion for the second round of this project.
总体而言,“人口密度”在与这两个风险指标的相关性相对较低的意义上突出。 另一方面,人口与这两个风险指标的相关性最高。 这就提出了一个问题:“面积”,“人口”或“人口密度”哪个特征将是最好的衡量这两个风险指标的指标,“犯罪严重度评分(CSS)”和“ COVID-19确诊病例” ? 这个问题需要保留,以便对该项目第二轮提出建议。
Nevertheless, for this first round, as per the Client’s request to scale the risk metrics by population density, I scale these two-risk metrics with population density, by simply dividing the two risk-metrics by population density. As result, we have ‘CSS Index’ and ‘COVID-19 Index’.
不过,在第一轮中,根据客户要求按人口密度缩放风险指标的要求,我将这两个风险度量值按人口密度进行了缩放,只需将两个风险指标除以人口密度即可。 结果,我们有了“ CSS索引”和“ COVID-19索引”。
In order to study individual distributions for these newly created indices, I made the following two basic types of visualizations. Here are two pairs of histogram and boxplot, the first pair for ‘CSS Index’ and the second pair for ‘COVID-19 Index’.
为了研究这些新创建的索引的个体分布,我进行了以下两种基本类型的可视化处理。 这是两对直方图和箱线图,第一对为“ CSS索引”,第二对为“ COVID-19索引”。
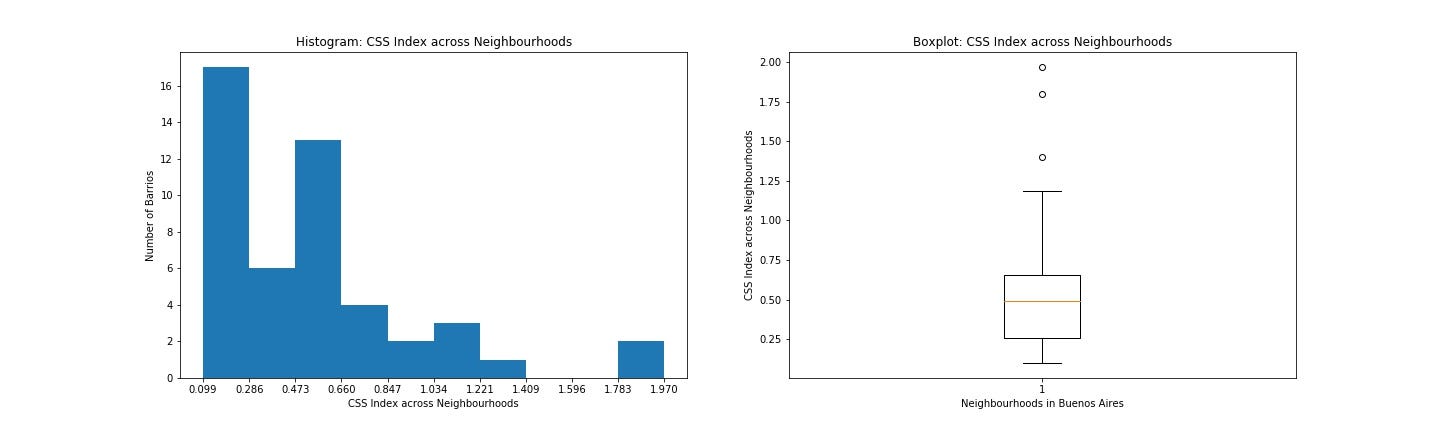
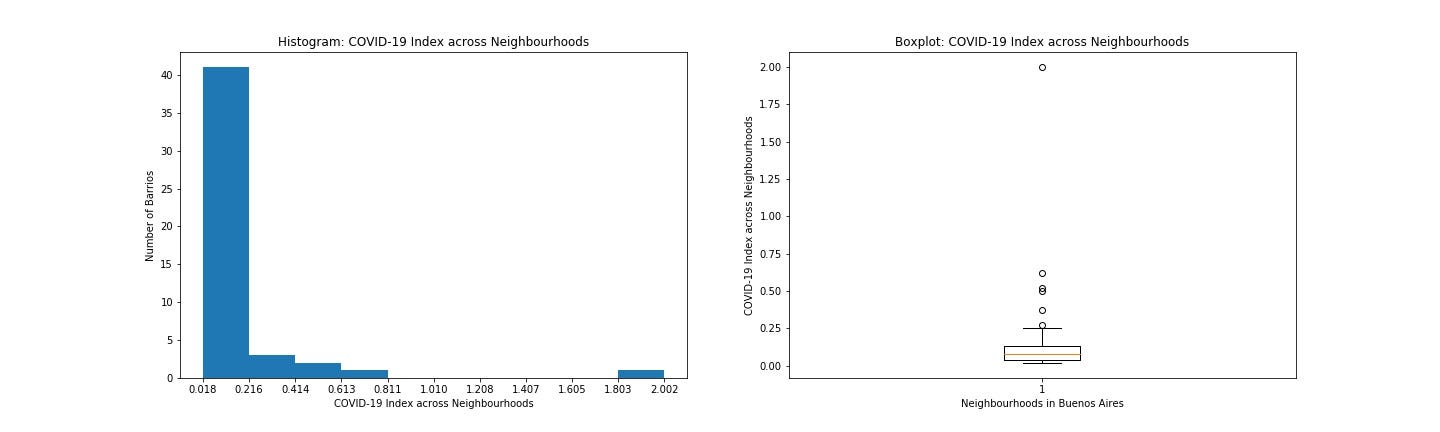
c) Histogram:
c)直方图:
A histogram is useful to capture the shape of the distribution. It displays the distribution of data points across a pre-specified number of segmented ranges of the feature variable called bins. These two histograms (both on the left side) above visually warn the presence of outliers.
直方图对于捕获分布的形状很有用。 它显示在预先指定数量的称为bins的特征变量的分段范围内的数据点分布。 上方的这两个直方图(均在左侧)警告存在异常值。
d) Boxplot:
d)箱线图:
A boxplot displays the distribution of data according to descriptive statistics of percentiles: e.g. 25%, 50%, 75%. For our data, the boxplots above (on the right side) isolated outliers over their top whiskers. The tables below present more detailed info about these outliers from these two boxplots.
箱形图根据百分位的描述性统计显示数据分布:例如25%,50%,75%。 对于我们的数据,上方(右侧)的箱线图将其顶部晶须上的离群值隔离了。 下表列出了来自这两个箱形图的这些离群值的详细信息。

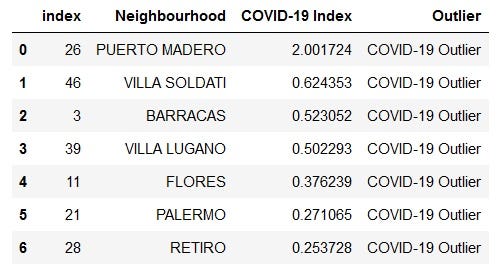
There are some overlapping outlier neighbourhoods between these two lists. Consolidating them, here is the list of 8 overall risk outliers.
这两个列表之间存在一些重叠的离群邻域。 合并它们,以下是8个总体风险异常值的列表。

Now, let me plot the neighbourhoods on the two-dimensional risk space: ‘CSS Index’ and ‘COVID-19 Index’. The scatter plot below also helps us confirm these outliers visually.
现在,让我在二维风险空间上绘制邻域:“ CSS索引”和“ COVID-19索引”。 下面的散点图还有助于我们从视觉上确认这些异常值。
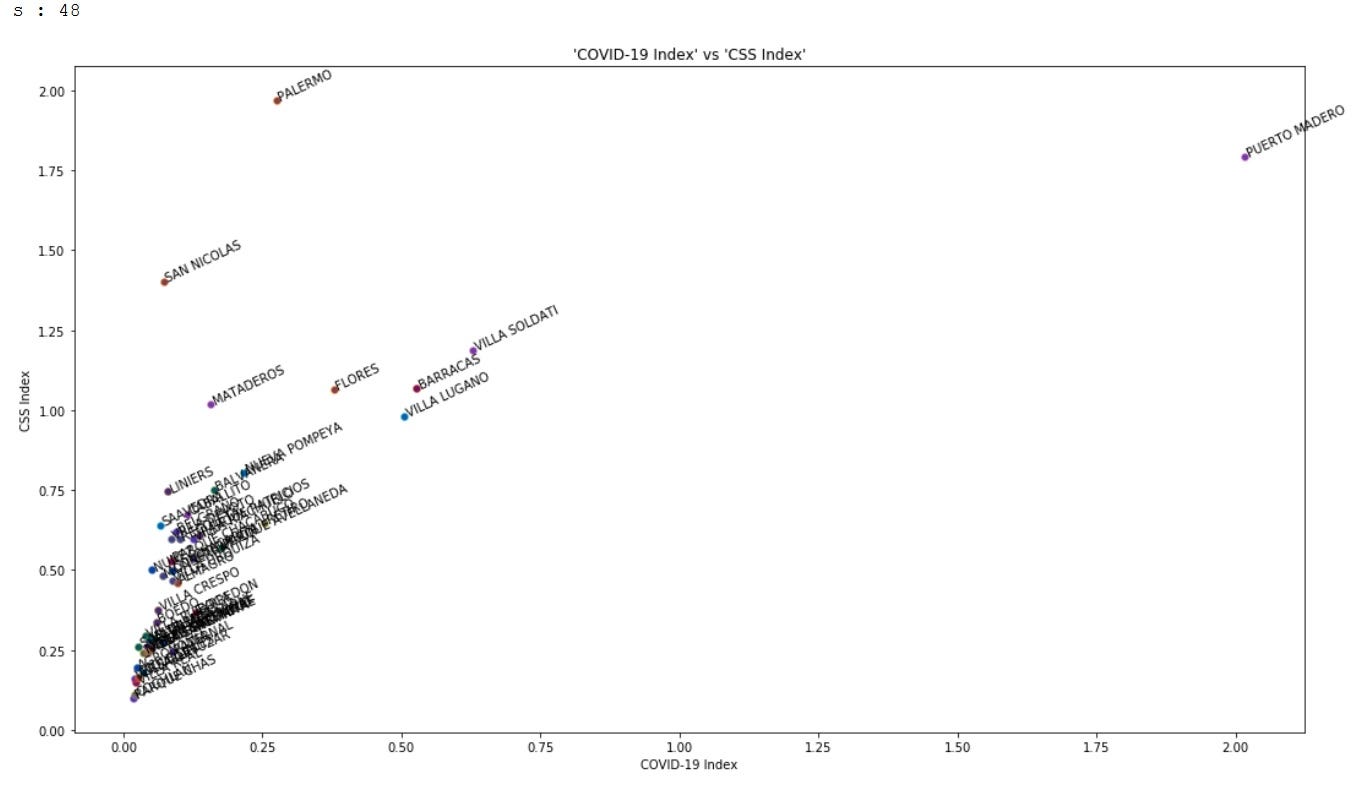
These simple visualizations and descriptive statistics can be a very powerful tool and it helps us shape an insight about the data at the stage of Data Understanding. In a way, before clustering analysis, the boxplot and the scatter plot have already spotted outliers.
这些简单的可视化和描述性统计信息可能是一个非常强大的工具,它可以帮助我们在“数据理解”阶段塑造有关数据的见解。 在某种程度上,在进行聚类分析之前,箱线图和散点图已经发现了异常值。
(2) “Foursquare Venue Profile” dataset:
(2)“四方场地概况”数据集:
Here is the summary of the Foursquare response to my query. In order to obtain an insight about the distribution of the response across different neighbourhoods, the histogram and the boxplot are presented below.
这是对我的查询的Foursquare响应的摘要。 为了深入了解不同社区的响应分布,下面介绍了直方图和箱形图。

The histogram might suggest that there might be some issues in the coherency of data quality and availability across different neighbourhoods. If that is the case, this might affect the quality of the result of clustering machine learning.
直方图可能表明不同社区之间数据质量和可用性的一致性可能存在一些问题。 如果真是这样,这可能会影响群集机器学习结果的质量。
Just in case, I would like to see if there is any relationship between the Foursquare’s response and the three basic profiles of neighbourhoods. I generated the correlation matrix and the scatter matrix.
为了以防万一,我想看看Foursquare的回答和三个基本街区之间是否有任何关系。 我生成了相关矩阵和散射矩阵。


Here is an intuitive outcome. Venue response has the highest correlation with population density and the least correlation with the area size of neighbourhoods. In other words, the scatter matrix and the correlation matrix suggest that the higher the population density, the more venue information Foursquare has for neighbourhoods. It appeals to our common sense in a way: densely populated busy neighbourhoods have more venues.
这是一个直观的结果。 场地响应与人口密度的相关性最高,而与社区面积的相关性则最小。 换句话说,散布矩阵和相关矩阵表明,人口密度越高,Foursquare提供给附近社区的场所信息越多。 它在某种程度上吸引了我们的常识:人口稠密的繁忙社区拥有更多场地。
For the rest of my work in data collection, data understanding, and data preparation, I would leave it up to the reader to see more detail in my code in the link above.
在数据收集,数据理解和数据准备的其余工作中,我将留给读者以查看上面链接中的代码中的更多细节。
方法论与分析 (B. Methodology & Analysis)
Now, the data is prepared for analysis. So, I can move on to analysis
现在,数据已准备好进行分析。 所以,我可以继续分析
The three objectives set by the Client at the outset and the data availability that I confirmed determine the scope of methodology. Cut a long story short, I run three clustering machine learning models for three different objectives and I got three very different lessons from them.
客户一开始设定的三个目标以及我确认的数据可用性决定了方法的范围。 简而言之,我针对三个不同的目标运行了三个集群机器学习模型,从中我得到了三个非常不同的教训。
Before proceeding further, let me review the three objectives here.
在继续进行之前,让我在这里回顾三个目标。
- Identify outlier high risk neighbourhoods (outlier neighbourhoods/clusters) in terms of these two risks — the general security risk (crime) and the pandemic risk (COVID-19). 从这两个风险(一般安全风险(犯罪)和大流行风险(COVID-19))中识别异常高风险社区(异常社区/集群)。
- Segment non-outlier neighbours into several clusters (the non-outlier neighbourhoods/clusters) and rank them based on a single quantitative risk metric (a compound risk metric of the general security risk and the pandemic risk). 将非离群的邻居划分为几个集群(非离群的邻居/集群),并基于单个定量风险度量(一般安全风险和大流行风险的复合风险度量)对它们进行排名。
- Use Foursquare API to characterize the Non-Outlier Neighbourhoods regarding popular venues. And if possible, segment Non-Outlier Neighbourhoods according to popular venue profiles. 使用Foursquare API来描述有关受欢迎场所的非离群社区。 并且,如果可能的话,请根据受欢迎的场所概况细分非离群地区。
Now, there presents one common salient feature among these three objectives. We have no ‘a priori knowledge’ about the underlying cluster structure of any of the subjects: outlier neighbourhoods, non-outlier neighbourhoods, and popular venue profiles among non-outlier neighbourhoods. Simply put, unlike supervised machine learning models, we have no labelled data to train: we have no empirical data about the dependent variable. All these three objectives demand us to discover hidden labels, or unknown underlying cluster structures in the dataset.
现在,这三个目标之间呈现出一个共同的显着特征。 我们没有关于任何主题的基础集群结构的“ 先验知识”:离群社区,非离群社区以及非离群社区中的热门场馆概况。 简而言之,与有监督的机器学习模型不同,我们没有要训练的标记数据:没有因变量的经验数据。 所有这三个目标都要求我们发现数据集中的隐藏标签或未知的基础簇结构。
This feature would naturally navigate us to the territory of unsupervised machine learning, and more specifically, ‘Clustering Machine Learning’ in our context.
此功能很自然地使我们导航到无监督机器学习的领域,更具体地讲,在我们的上下文中是“集群机器学习”。
By its design — in the absence of the labelled data (empirical data for the dependent variable) — it would be difficult to automate the validation/evaluation process for an unsupervised machine learning, simply because there is no empirical label to compare the model outputs with. According to Dr. Andrew Ng, there seems no widely accepted consensus about clear cut methods to assess the goodness of fit for clustering machine learning models. This creates an ample room for human insight, such as domain/business expertise, to get involved in the validation/evaluation process.
通过其设计-在没有标记数据(因变量的经验数据)的情况下-很难自动化无监督机器学习的验证/评估过程,这仅仅是因为没有经验标签可以将模型输出与。 据吴安德(Andrew Ng)博士说,似乎没有一种明确的方法可以用来评估聚类机器学习模型的适用性 。 这为诸如域/业务专业知识之类的人类见识创造了足够的空间,以参与验证/评估过程。
In this context, for this project, I will put more emphasis on tuning the model a priori rather than pursuing the automation of the a posteriori validation/evaluation process.
在这种情况下,对于这个项目,我将更加强调先验地调整模型,而不是追求后验验证/评估过程的自动化。
As one more important thing to mention, we need to normalize/standardize all the input data before passing them to machine learning models.
值得一提的是,我们需要在将所有输入数据传递到机器学习模型之前对其进行标准化/标准化。
Now, I will discuss the methodologies for each objective one by one.
现在,我将逐个讨论每个目标的方法。
B1。 针对目标1的DBSCAN集群: (B1. DBSCAN Clustering for Objective 1:)
The first objective is to identify ‘Outlier Neighbourhoods’.
第一个目标是确定“异常地区”。
Now, in the scatter plot below, all the neighbourhoods are plotted in the two-dimensional risk space: ‘CSS Index’ vs ‘COVID-19 Index’ space.
现在,在下面的散点图中,所有邻域都在二维风险空间中绘制:“ CSS索引”与“ COVID-19索引”空间。
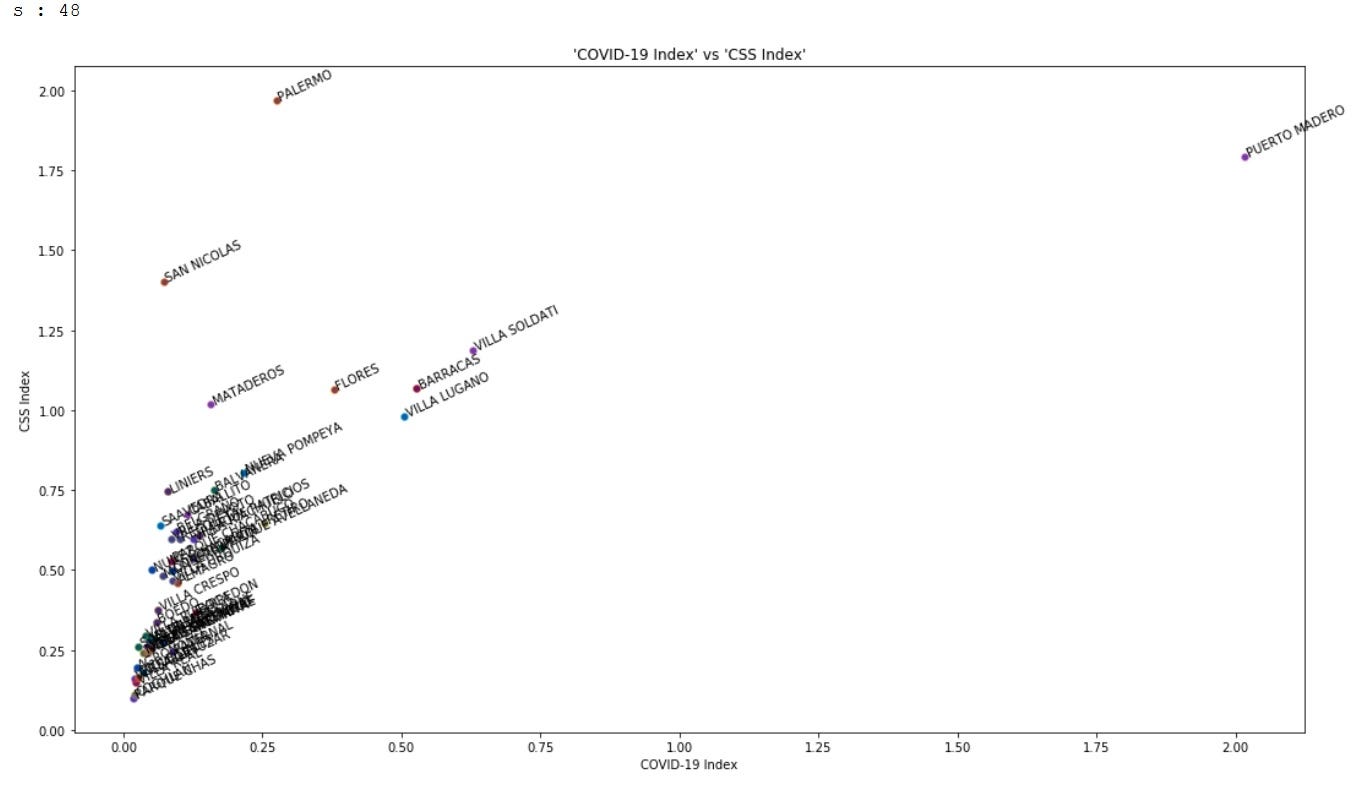
In order to identify outliers out of these “two-dimensional spatial data points”, I chose DBSCAN Clustering model, or Density-based Spatial Clustering of Applications with Noise. As its name suggests, DBSCAN is a density-based clustering algorithm and deemed appropriate for examining spatial data. Especially, I am very interested in how the density-based clustering algorithm would process outliers which are expected to demonstrate extremely sparse density.
为了从这些“二维空间数据点”中识别离群值,我选择了DBSCAN聚类模型 ,即基于噪声的应用程序的基于密度的空间聚类 。 顾名思义,DBSCAN是基于密度的聚类算法,被认为适合检查空间数据 。 尤其是,我对基于密度的聚类算法如何处理离群值异常稀疏的异常非常感兴趣。
There are several hyperparameters for DBSCAN. And the one considered as the most crucial is ‘eps’. According to the Skit-learn.org website, ‘eps’ is:
DBSCAN有几个超参数。 被认为是最关键的一个是“ eps ”。 根据Skit-learn.org网站,“ eps ”为:
“the maximum distance between two samples for one to be considered as in the neighborhood of the other. This is not a maximum bound on the distances of points within a cluster. This is the most important DBSCAN parameter to choose appropriately for your data set and distance function.” (https://scikit-learn.org/stable/modules/generated/sklearn.cluster.DBSCAN.html)
“一个样本的两个样本之间的最大距离应视为另一个样本的邻域。 这不是群集中点的距离的最大界限。 这是 为您的数据集和距离函数适当选择 的最重要的DBSCAN参数 。” ( https://scikit-learn.org/stable/modules/generated/sklearn.cluster.DBSCAN.html )
In order to tune ‘eps’, I will use KneeLocator of the python library kneed to identify the knee point (or elbow point).
为了调谐“EPS”,我将使用KneeLocator的Python库用膝盖 识别拐点 (或肘点)。
What is the knee point?
拐点是什么?
One way to interpret the knee point is that it is a point where the tuning results start converging within a certain acceptable range. Simply put, it is a point where further tuning enhancement would no longer yield a material incremental benefit. In other words, the knee point determines a cost-benefit boundary for model hyperparameter tuning enhancement.(Source: https://ieeexplore.ieee.org/document/5961514)
一种解释拐点的方法是,在该点上,调整结果开始在某个可接受的范围内收敛。 简而言之,在这一点上进一步的调优将不再产生实质性的增量收益。 换句话说, 拐点确定了模型超参数调整增强的成本效益边界 。(来源: https : //ieeexplore.ieee.org/document/5961514 )
In order to discover the knee point of the model hyperparameter, ‘eps’, for DBSCAN model, I passed the normalized/standardized data of these two risk indices — namely ‘Standardized CSS Index’ and ‘Standardized COVID-19 Index’ — into the KneeLocator.
为了发现模型超参数“ EPS” 的拐点 ,对于DBSCAN模型,我将这两个风险指数(即“标准化CSS指数”和“标准化COVID-19指数”)的标准化/标准化数据传递给了KneeLocator 。
And here is the plot result:
这是绘图结果:

The crossing point between the distance curve and the dotted straight vertical line identifies the knee point. Above the chart, KneeLocator also returned the one single value, 0.494, as the knee point. KneeLocator is telling me to choose this value as ‘eps’ to optimize the DBSCAN model. Accordingly, I plug it into DBSCAN. And here is the result.
距离曲线和垂直虚线之间的交点表示拐点。 在图表上方, KneeLocator还返回了一个单一值0.494作为拐点 。 KneeLocator告诉我选择此值作为'eps'以优化DBSCAN模型。 因此,我将其插入DBSCAN 。 这就是结果。

With this plot, I can confirm that DBSCAN distinguished the sparsely distributed outliers from others, yielding two clusters for them: the cluster -1 (light green) and the cluster 1 (orange). Below, I list up all the neighbourhoods of these two sparse clusters.
通过此图,我可以确认DBSCAN可以将稀疏分布的异常值与其他异常值区分开,从而为它们生成两个聚类:聚类-1(浅绿色)和聚类1(橙色)。 下面,我列出了这两个稀疏群集的所有邻域。

Furthermore, in order to assess if the result at the knee point is good or not, I run DBSCAN with other different values of ‘eps’. Here is the result:
此外,为了评估拐点处的结果是否良好,我使用其他不同的“ eps”值运行DBSCAN。 结果如下:
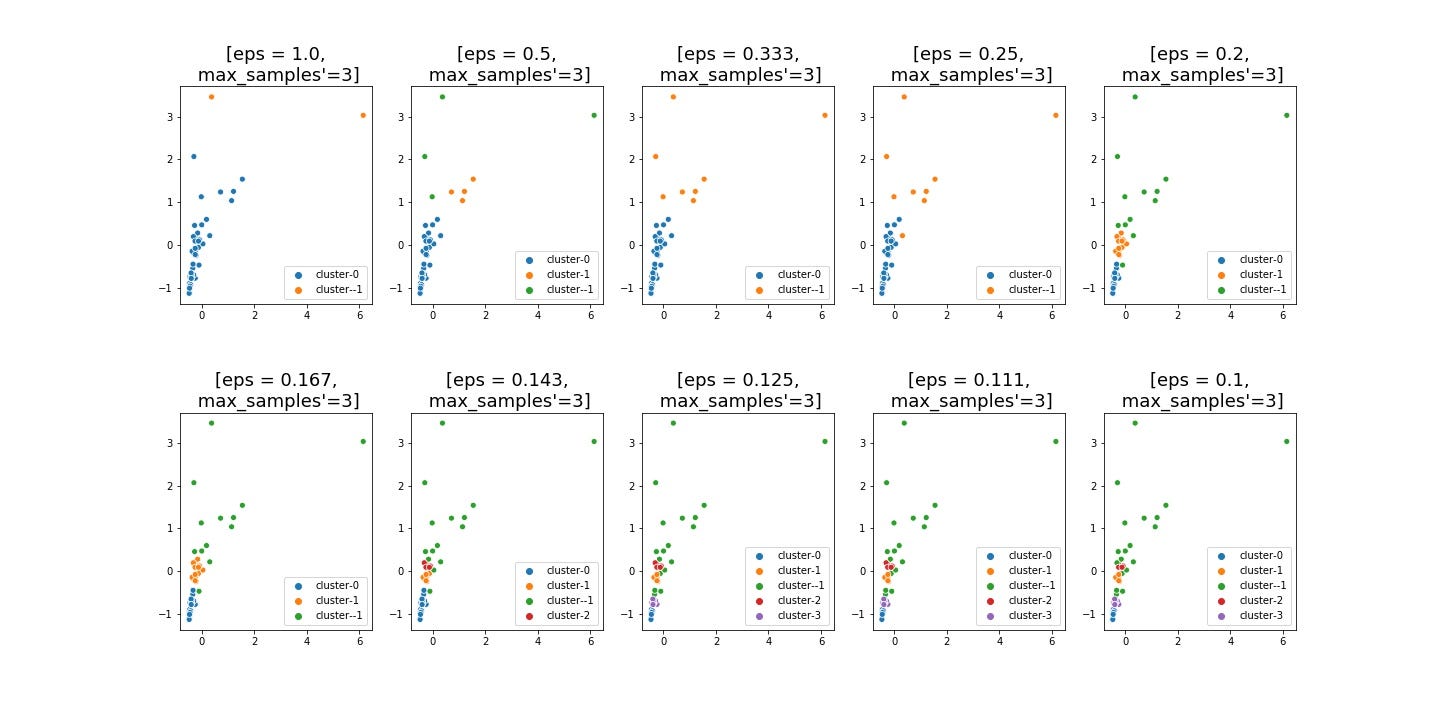
Compared with the result of the knee point ‘eps’, no alternative above would give us a better convincing result. Thus, I will not reject the knee point, the output of KneeLocator, as the value for the hyperparameter, ‘eps’.
与拐点 “ eps”的结果相比,上述任何选择都不能给我们带来更好的说服力。 因此,我不会拒绝拐点,即KneeLocator的输出,作为超参数“ eps”的值。
When I look at the result of DBSCAN, I realise that this clustering result isolated into two clusters the same neighbourhoods as the outliers that the boxplot visualization identified during the Data Understanding stage.
当我查看DBSCAN的结果时,我意识到该聚类结果被隔离为两个聚类,它们与盒形图可视化在数据理解阶段确定的离群值相同。
For your reminder, here is the result of the boxplot once again.
提醒您,这是箱线图的结果。

The contents of these two results are identical (except for the order of the list). What does it tell us?
这两个结果的内容是相同的(列表的顺序除外)。 它告诉我们什么?
Now, the question worthwhile to ask would be: if we needed to perform a sophisticated and expensive model such as DBSCAN to identify outliers, when the simple boxplot can do that job.
现在,值得提出的问题是:当简单的箱形图可以完成此工作时,是否需要执行复杂且昂贵的模型(例如DBSCAN)来识别异常值。
In the perspective of cost-benefit management, the simple boxplot did the same job for the less cost — almost no cost. This might not be true when we have different data: especially, in a high-dimensional datapoints.
从成本效益管理的角度来看,简单的箱线图以较低的成本完成了相同的工作-几乎没有成本。 当我们拥有不同的数据时,尤其是在高维数据点中,情况可能并非如此。
At least, we should take this lesson in modesty so that we should not underestimate the power of simple methods like the boxplot visualisation.
至少,我们应该谨慎地学习本课,以免低估箱形图可视化等简单方法的功能。
B2。 第二个目标的层次聚类 (B2. Hierarchical Clustering for the second objective)
Now, the second objective can be broken down into the following core sub-objectives:
现在,第二个目标可以分解为以下核心子目标:
- Segmentation of ‘Non-Outlier Neighbourhoods’. “非离群社区”的细分。
- Construction of a single compound risk metric to measure both the general security risk and the pandemic risk. 构建一个单一的复合风险度量以同时测量一般安全风险和大流行风险。
- Measuring the risk profile at cluster level (not datapoints/neighbourhoods level). 在群集级别(不是数据点/社区级别)上测量风险状况。
a) Segmentation of ‘Non-Outlier Neighbourhoods’.
a) “非离群社区”的细分。
Given the result of the first objective, now I can remove “Outlier Neighbourhoods” from our dataset and focus only on “Non-Outlier Neighbourhoods” for further clustering segmentations.
有了第一个目标的结果,现在我可以从数据集中删除“离群值邻域”,而仅关注“非离群值邻域”以进一步进行聚类分割。
This time, I choose Hierarchical Clustering model. Here are the reasons why I selected this particular model for the second objective:
这次,我选择层次聚类模型。 这是我选择此特定模型作为第二个目标的原因:
I have no advance knowledge how many underlying clusters are expected in the dataset. Many clustering models, paradoxically, require the number of clusters as a hyperparameter input to tune the models a priori. But, Hierarchical Clustering doesn’t.
我尚不了解在数据集中需要多少个基础群集。 矛盾的是,许多聚类模型要求将聚类的数量作为超参数输入来对模型进行先验调整。 但是,分层聚类却不是。
- In addition, Hierarchical Clustering algorithm can generate a dendrogram that illustrates a tree-like cluster structure based on the hierarchical structure of the pairwise spatial distance distribution. The ‘dendrogram’ appeals to our human intuition in discovering the underlying cluster structure. 另外,分层聚类算法可以基于成对空间距离分布的分层结构生成树状图,该树状图说明树状聚类结构。 “树状图”吸引了我们人类的直觉,从而发现了潜在的簇结构。
What is a dendrogram? Seeing is understanding! Maybe. Here you go:
什么是树状图? 眼见为谅! 也许。 干得好:
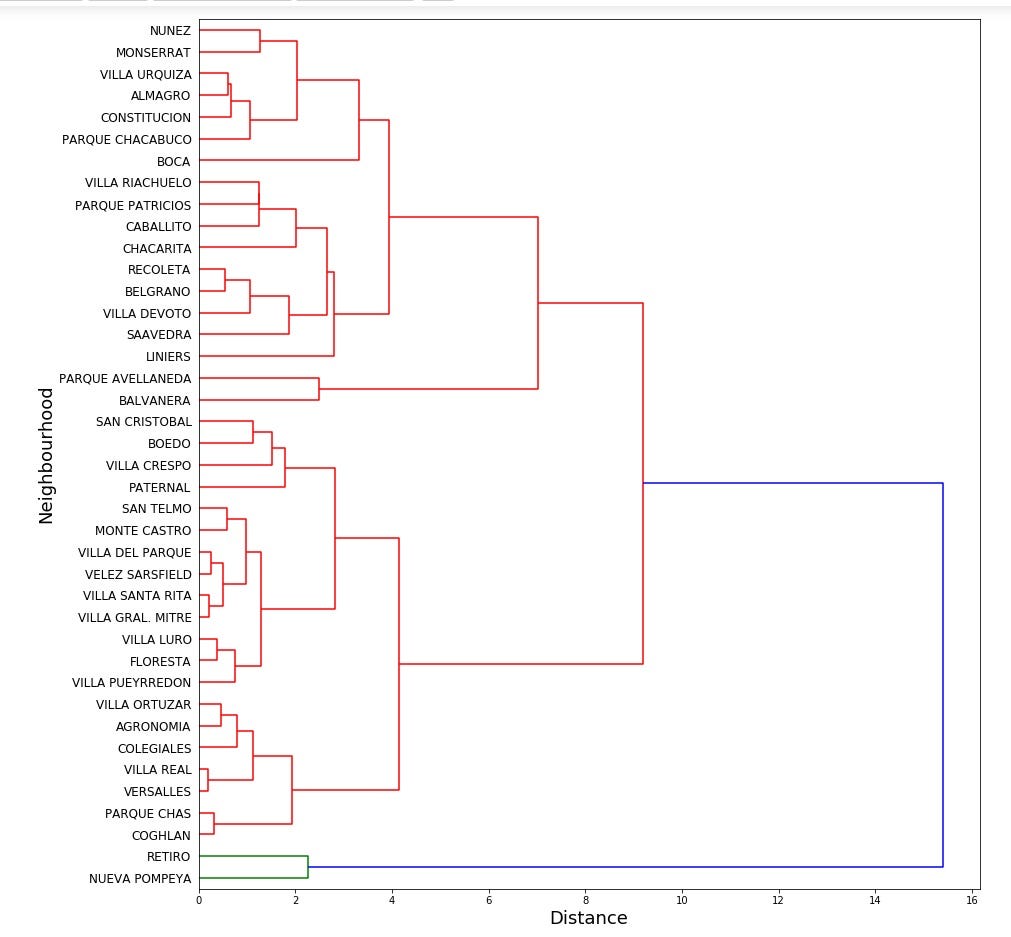
The dendrogram allows the user to study the hierarchical structure of distances among datapoints and the underlying layers of cluster hierarchy. The dendrogram analyses and displays the hierarchical structure of all the potential clusters automatically. The resulting dendrogram illustrates a tree-like cluster structure based on the pairwise distance distribution. In this way, the dendrogram allows the user to design how many clusters to be made for further analysis. We can visually confirm the hierarchy of the distances among data points and the layers of cluster structure in the dendrogram.
树状图允许用户研究数据点之间的距离的层次结构以及群集层次结构的基础层。 树状图自动分析并显示所有潜在簇的层次结构。 生成的树状图显示了基于成对距离分布的树状群集结构。 这样,树状图使用户可以设计要进行进一步分析的群集数。 我们可以从视觉上确认数据点之间的距离的层次结构以及树状图中的簇结构层。
- From this dendrogram, I choose 4 (at the distance of 5 or 6 on the x-axis in the dendrogram) as the number of clusters to be shaped. 从该树状图中,我选择4(在树状图的x轴上距离5或6)作为要成形的簇的数量。
- Then, I run Hierarchical Cluster Model for the second time, this time with the specification of the number of the clusters, 4. 然后,我第二次运行Hierarchical Cluster Model,这是在指定簇数为4的情况下进行的。
Accordingly, I got the 4 clusters of the neighbourhoods. The following two charts present the clustered neighbourhoods on the two risk-metrics space: one with neighbourhoods’ names and the other without.
因此,我得到了周围的4个集群。 以下两个图表显示了两个风险度量空间上的聚类邻域:一个带有邻域名称,另一个没有邻域名称。


In order to assign these clusters risk values. I will construct one single compound risk metric.
为了分配这些集群风险值。 我将构建一个单一的复合风险度量。
b) Construction of Compound Risk Metric
b)构建复合风险度量
I need to compress the two risk profiles of clusters (‘CSS’ and ‘COVID-19’) together into one single compound metric in order to achieve one of the Client’s requirement.
我需要将群集的两个风险概况(“ CSS”和“ COVID-19”)压缩到一个单一的复合指标中,以实现客户的要求之一。
For this purpose, I formulated a compound risk metric as follows.
为此,我制定了如下的复合风险度量。
Compound Risk Metric =
复合风险指标=
[(Standardized CSS Index — Standardized Origin of CSS Index)² +
[((标准化CSS索引-标准化CSS索引的来源)²+
(Standardized COVID-19 Index — Standardized Origin of COVID-19 Index)² ]^0.5
(标准化的COVID-19索引-标准化的COVID-19索引来源)²] ^ 0.5
Although the formula might appear not straightforward, its basic intent is very simple: to measure the risk position of each neighbourhood from the risk-free point in the two-dimensional risk space.
尽管该公式可能看起来并不简单,但是其基本意图却非常简单:从二维风险空间中的无风险点测量每个邻域的风险位置。
For the raw data, the risk-free point is at the origin of the two-risk-metrics space, which is (0,0): 0 represents no risk in the raw data. The formula above is measuring the risk position of a data point from the risk-free point after the standardization/normalization transformation. It is because in order to pass the data into the machine learning model, the data needs to be normalized/standardized. In that sense, the formula above measures the distance between the standardized data points and the standardized risk-free position.
对于原始数据,无风险点位于两个风险度量空间的起点,即(0,0):0表示原始数据中无风险。 上面的公式从标准化/规范化转换后的无风险点开始测量数据点的风险位置。 这是因为为了将数据传递到机器学习模型中,需要对数据进行标准化/标准化。 从这个意义上讲,上面的公式测量了标准化数据点和标准化无风险头寸之间的距离。
Nothing else. That’s all and simple.
没有其他的。 就是这么简单。
b) Risk Profile of Cluster
b)集群风险简介
Now, my ultimate purpose here is to quantify the risk profile at cluster level, not at data point/neighbourhood level.
现在,我的最终目的是在集群级别而不是数据点/社区级别量化风险状况。
Each cluster has its own unique centre, aka “centroid”. Thus, in order to measure the risk profile of each cluster, I can refer to the centroid for each cluster. In this way, I can grade and rank all these clusters according to the compound risk metric of their centroids.
每个簇都有自己独特的中心,又称“ 质心 ”。 因此,为了衡量每个群集的风险状况,我可以参考每个群集的质心。 这样,我可以根据其质心的复合风险度量对所有这些聚类进行分级和排名。
Accordingly, I measure the compound risk metric of the centroids of all these 5 Non-Outlier Clusters and assign each of them a grade.
因此,我测量了所有这5个非异常值聚类的质心的复合风险度量,并为其分配了一个等级。
Here is the result.
这是结果。

The higher the grade, the riskier the cluster. I merged this result with the master dataset and assigned the cluster grade 5 to the 2 outlier clusters. Then, I mapped these cluster grades of all the neighbourhoods across CABA in the following Choropleth Map.
等级越高,集群的风险就越高。 我将此结果与主数据集合并,并将5级聚类分配给2个离群聚类。 然后,在下面的Choropleth映射中,我绘制了CABA中所有邻域的这些聚类等级。

This map visually summarises the findings for these first two objectives. It allows the user to visually distinguish neighbourhood clusters across the autonomous city of Buenos Aires based on their cluster risk grade.
该地图直观地总结了前两个目标的发现。 它使用户能够根据布宜诺斯艾利斯自治城市的聚类风险等级在视觉上区分其附近的聚类。
B1。 第三个目标的Foursquare分析 (B1. Foursquare Analysis for the third objective)
For the third objective, I used Foursquare data to carry out two analyses: Popular Venue Analysis; and Segmentation of Neighbourhoods based on Venue Composition.
对于第三个目标,我使用Foursquare数据进行了两个分析:流行场地分析; 场地组成的邻域细分。
a) Popular Venue Analysis:
a)流行场地分析:
I apply One Hot Encoding algorithm to transform the data structure of venue category for further data transformation.
我应用一种热编码算法来转换会场类别的数据结构,以进行进一步的数据转换。
With Foursquare data, which has venue-base information, I will use Pandas’ “groupby” method to transform it to a neighbourhood-base data and summarise the top 5 popular venue categories for each of 40 ‘Non-Outlier Neighbourhoods’. The result is a very long list thus, I only display the first 7 lines.
借助具有场地基础信息的Foursquare数据,我将使用Pandas的“ groupby”方法将其转换为基于邻域的数据,并总结40个“非离群邻域”中每一个的前5个热门场所类别。 结果是一个很长的列表,因此,我只显示前7行。
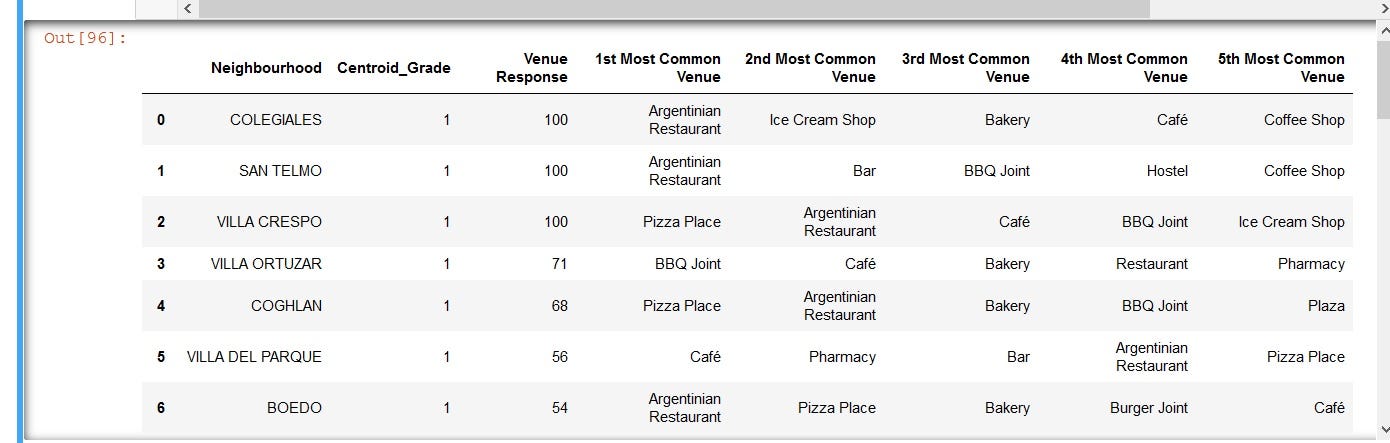
b) Segmentation of Neighbourhoods based on Venue Profile
b)根据场地概况对邻域进行细分
Next, I need to segment the Foursquare venue profile of each neighbourhood. For this purpose, I contemplate K-Means Clustering Machine Learning.
接下来,我需要细分每个社区的Foursquare场地概况。 为此,我打算使用K-Means集群机器学习。
For a successful K-Means clustering result, I need to determine one of its hyperparameters, n_clusters: the number of clusters to form, thus, the number of centroids to generate. (source: https://scikit-learn.org/stable/modules/generated/sklearn.cluster.KMeans.html)
为了获得成功的K均值聚类结果,我需要确定其超参数之一n_clusters:要形成的簇数,因此要生成的质心数。 (来源: https : //scikit-learn.org/stable/modules/generated/sklearn.cluster.KMeans.html )
I will run two hyperparameter tuning methods — K-Means Elbow Method and Silhouette Score Analysis — to tune its most important hyperparameter, n_clusters. These tuning methods would give me an insight about how to cluster the data for a meaningful analysis. Based on the findings from these tuning methods, I would decide how to implement the K-Means Clustering machine learning model.
我将运行两种超参数调整方法-K-Means弯头方法和Silhouette Score分析-调整其最重要的超参数n_clusters。 这些调优方法将使我对如何对数据进行聚类进行有意义的分析有深刻的了解。 基于这些调整方法的发现,我将决定如何实施K-Means聚类机器学习模型。
‘K-Means Elbow Method’
“ K-均值肘法”
The spirit of ‘K-Means Elbow Method’ is the same as the knee point method that I explained earlier. Elbow locates a point where further tuning enhancement would no longer yield a material incremental benefit. In other words, Elbow determines a cost-benefit boundary for model hyperparameter tuning enhancement. Here is the result of K-Means Elbow Method:
“ K均值肘部弯曲法”的精神与我之前介绍的拐点法相同。 弯头定位在一个点上,进一步的调音增强将不再产生实质性的增量收益。 换句话说, Elbow确定了模型超参数调整增强的成本效益边界。 这是K-均值肘法的结果:
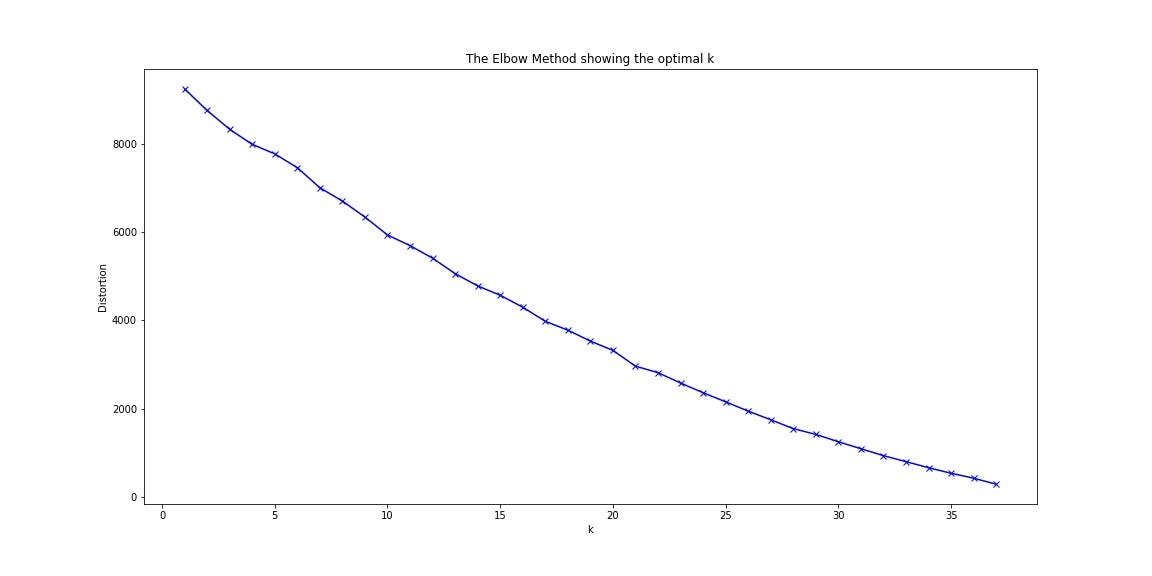
As the number of clusters increases, the response does not converge into any range; instead, it keeps dropping. There is no knee/elbow, the cost-benefit boundary, in the entire space. This suggests that there might be no meaningful cluster structure in the dataset. This is a disappointing result.
随着簇数的增加,响应不会收敛到任何范围。 相反,它一直在下降。 整个空间中没有膝盖/肘部,即成本效益边界。 这表明数据集中可能没有有意义的聚类结构。 这是令人失望的结果。
Silhouette Score Analysis
轮廓分数分析
https://scikit-learn.org/stable/auto_examples/cluster/plot_kmeans_silhouette_analysis.html
https://scikit-learn.org/stable/auto_examples/cluster/plot_kmeans_silhouette_analysis.html
Silhouette analysis can be used to study the separation distance between the resulting clusters. The silhouette plot displays a measure of how close each point in one cluster is to points in the neighbouring clusters and thus provides a way to assess parameters like number of clusters visually. This measure has a range of [-1, 1].
轮廓分析可用于研究所得簇之间的分离距离。 轮廓图显示了一个群集中的每个点与相邻群集中的点的接近程度的度量,从而提供了一种直观地评估参数(如群集数)的方法。 此度量的范围为[-1,1]。
Cut a long story short, the best value is 1, the worst -1.
简而言之,最佳值为1,最差为-1。
- Silhouette coefficients (as these values are referred to as) near +1 indicate that the sample is far away from the neighbouring clusters. Which means, the sample is distinguished from the points belonging to other clusters. 接近+1的轮廓系数(称为这些值)表示样本距离相邻簇很远。 这意味着,样本与属于其他聚类的点是有区别的。
- A value of 0 indicates that the sample is on or very close to the decision boundary between two neighbouring clusters. 值为0表示样本在两个相邻聚类之间的决策边界上或非常接近。
- Negative values, (-1,0), indicate that those samples might have been assigned to the wrong cluster. 负值(-1,0)表示这些样本可能已分配给错误的群集。
I run the Silhouette Coefficient Analysis for 4 scenarios: n_cluster = [ 2, 3, 4, 5] to see which value of n_cluster yields the result closest to 1. And here are the results:
I run the Silhouette Coefficient Analysis for 4 scenarios: n_cluster = [ 2, 3, 4, 5] to see which value of n_cluster yields the result closest to 1. And here are the results:





All results are close to 0, suggesting that the sample is on or very close to the decision boundary between two neighbouring clusters. In other words, there is no apparent indication of an underlying cluster structure in the dataset.
All results are close to 0, suggesting that the sample is on or very close to the decision boundary between two neighbouring clusters. In other words, there is no apparent indication of an underlying cluster structure in the dataset.
Both K-Means Elbow Method and Silhouette Analysis suggest that we cannot confirm an indication about the presence of the underlying cluster structure in the data set. It might be due to the characteristics of the city. Or it could be due to the quality of available data.
Both K-Means Elbow Method and Silhouette Analysis suggest that we cannot confirm an indication about the presence of the underlying cluster structure in the data set. It might be due to the characteristics of the city. Or it could be due to the quality of available data.
Whatever real reason it might be, all we know from these tuning results is that there is no convincing implication regarding the underlying cluster structure in the given data. In order to avoid an unreliable, and potentially misleading, recommendation, I would rather refrain from performing K-Means Clustering Model for the given dataset.
Whatever real reason it might be, all we know from these tuning results is that there is no convincing implication regarding the underlying cluster structure in the given data. In order to avoid an unreliable, and potentially misleading, recommendation, I would rather refrain from performing K-Means Clustering Model for the given dataset.
C. Discussion (C. Discussion)
C1. Three Lessons from Three Different Clustering Analyses (C1. Three Lessons from Three Different Clustering Analyses)
Lesson from the first objective:
Lesson from the first objective:
The first objective was to segregate outliers out of the dataset.
The first objective was to segregate outliers out of the dataset.
Before conducting clustering analysis, two simple boxplots automatically isolated outliers above their top whiskers from the rest: 8 in total for both of these two risk indices — the general security risk metric (Crime Severity Index) and the pandemic risk metric (COVID-19 Index).
Before conducting clustering analysis, two simple boxplots automatically isolated outliers above their top whiskers from the rest: 8 in total for both of these two risk indices — the general security risk metric (Crime Severity Index) and the pandemic risk metric (COVID-19 Index).
Then, DBSCAN clustering algorithm segmented these exactly identical 8 datapoints that the box plots identified as two remote clusters of sparsely distributed datapoints. Simply put, the machine learning model only confirmed the validity of the boxplots’ earlier automatic identification of those outliers.
Then, DBSCAN clustering algorithm segmented these exactly identical 8 datapoints that the box plots identified as two remote clusters of sparsely distributed datapoints. Simply put, the machine learning model only confirmed the validity of the boxplots' earlier automatic identification of those outliers.
This case tells us a lesson that a sophisticated method is not necessarily superior to a simpler method. Both of them did exactly the same job. We should take this lesson in modesty from the cost-benefit management perspective.
This case tells us a lesson that a sophisticated method is not necessarily superior to a simpler method. Both of them did exactly the same job. We should take this lesson in modesty from the cost-benefit management perspective.
Lesson from the second objective
Lesson from the second objective
The second objective was to segment ‘non-outlier’ neighbourhoods according to a compound risk metric (of CSS Index and COVID-19 Index).
The second objective was to segment 'non-outlier' neighbourhoods according to a compound risk metric (of CSS Index and COVID-19 Index).
The dendrogram of Hierarchical Clustering Model arranged 40 non-outlier neighbourhoods accordingly to their pairwise distance hierarchy. In other words, the dendrogram analysed and displayed the hierarchical structure of all the potential clusters automatically. And it allowed the user to explore and compare various cluster structures across different hierarchical levels. It’s worth running Hierarchical Clustering Model to generate the dendrogram because it visually helps the user shape human insight about the underlying cluster structural hierarchy. There is no other easier alternative to do the same job. It actually helped me to decide how many clusters to generate with Hierarchical Clustering algorithm for the second run.
The dendrogram of Hierarchical Clustering Model arranged 40 non-outlier neighbourhoods accordingly to their pairwise distance hierarchy. In other words, the dendrogram analysed and displayed the hierarchical structure of all the potential clusters automatically. And it allowed the user to explore and compare various cluster structures across different hierarchical levels. It's worth running Hierarchical Clustering Model to generate the dendrogram because it visually helps the user shape human insight about the underlying cluster structural hierarchy. There is no other easier alternative to do the same job. It actually helped me to decide how many clusters to generate with Hierarchical Clustering algorithm for the second run.
This presents a successful case that a machine learning model can play a productive role in supporting human decision-making process. A user can leverage one’s own profound domain expertise or human insight in the use of the dendrogram and effectively achieve the given objective.
This presents a successful case that a machine learning model can play a productive role in supporting human decision-making process. A user can leverage one's own profound domain expertise or human insight in the use of the dendrogram and effectively achieve the given objective.
The lesson here is that the user can proactively interact with machine learning algorithm to optimise the performance of machine learning and make a better decision.
The lesson here is that the user can proactively interact with machine learning algorithm to optimise the performance of machine learning and make a better decision.
Lesson from the third objective
Lesson from the third objective
The third objective was to cluster the neighbourhoods according to the Foursquare venue profile.
The third objective was to cluster the neighbourhoods according to the Foursquare venue profile.
I performed two hyperparameter tuning methods (K-Means Elbow Method and Silhouette Score Analysis) to discover the best n_clusters, one of the hyperparameters for K-Mean Clustering algorithm. Unfortunately, neither of them yielded a convincing implication about the underlying cluster structure in the Foursquare venue dataset. This suggests that a clustering model would unlikely yield a reliable result for the given dataset.
I performed two hyperparameter tuning methods (K-Means Elbow Method and Silhouette Score Analysis) to discover the best n_clusters , one of the hyperparameters for K-Mean Clustering algorithm. Unfortunately, neither of them yielded a convincing implication about the underlying cluster structure in the Foursquare venue dataset. This suggests that a clustering model would unlikely yield a reliable result for the given dataset.
The output of the machine learning is as good as the data input. The disappointing hyperparameter tuning result might have something to do with earlier concern about the quality of the Foursquare data.
The output of the machine learning is as good as the data input. The disappointing hyperparameter tuning result might have something to do with earlier concern about the quality of the Foursquare data.
Or, possibly there could be actually no particular underlying venue-based cluster structure among the neighbourhoods in CABA. That case, there would be no reason for running a clustering model for the dataset.
Or, possibly there could be actually no particular underlying venue-based cluster structure among the neighbourhoods in CABA. That case, there would be no reason for running a clustering model for the dataset.
Which is correct? This question, requiring a comparative study with data from other sources, might be a good topic for the second round of the study.
Which is correct? This question, requiring a comparative study with data from other sources, might be a good topic for the second round of the study.
Nonetheless, whatever real reason it might be, all I know from these tuning results is that there is no convincing implication regarding the underlying cluster structure in the given data. The lesson here would be: in the absence of supporting indication for the use of machine learning, I would be better off refraining from performing it in order to avoid a potentially misleading inference. Instead, I could rather provide more basic materials that can assist the Client use their human insight/domain expertise to analyse the subject.
Nonetheless, whatever real reason it might be, all I know from these tuning results is that there is no convincing implication regarding the underlying cluster structure in the given data. The lesson here would be: in the absence of supporting indication for the use of machine learning, I would be better off refraining from performing it in order to avoid a potentially misleading inference. Instead, I could rather provide more basic materials that can assist the Client use their human insight/domain expertise to analyse the subject.
Overall, with these different implications given, it would be naïve to believe that we can simply automate machine learning process from the beginning to the end. Overall, all these cases support that human involvement could make machine learning more productive.
Overall, with these different implications given, it would be naïve to believe that we can simply automate machine learning process from the beginning to the end. Overall, all these cases support that human involvement could make machine learning more productive.
C2. Suggestions for Future Development (C2. Suggestions for Future Development)
As the Client stressed at the outset of the project, this analysis was the preliminary analysis for a further extended study for their business expansion. Now, based on the findings from this analysis I would like to contribute some suggestions for the next round. Let me start.
As the Client stressed at the outset of the project, this analysis was the preliminary analysis for a further extended study for their business expansion. Now, based on the findings from this analysis I would like to contribute some suggestions for the next round. Let me start.
Different Local Source for Venue Data
Different Local Source for Venue Data
Unfortunately, for the second part of the third objective — to segment non-outlier neighbourhoods into clusters based on their venue profile — I could not derive any convincing inference regarding the underlying cluster structure among non-outlier neighbourhoods. There were two possibilities as aforementioned. As one possibility, there is some issue in the quality of the Foursquare data. As the other possibility, there is actually no underlying cluster structure in the actual subject.
Unfortunately, for the second part of the third objective — to segment non-outlier neighbourhoods into clusters based on their venue profile — I could not derive any convincing inference regarding the underlying cluster structure among non-outlier neighbourhoods. There were two possibilities as aforementioned. As one possibility, there is some issue in the quality of the Foursquare data. As the other possibility, there is actually no underlying cluster structure in the actual subject.
For the former case, I would suggest that the Client might benefit from exploring other sources than Foursquare to examine the venue profiles of these neighbourhoods. That would allow the Client to assess by comparison if the Foursquare data is representative of the actual state of popular venues in this particular city.
For the former case, I would suggest that the Client might benefit from exploring other sources than Foursquare to examine the venue profiles of these neighbourhoods. That would allow the Client to assess by comparison if the Foursquare data is representative of the actual state of popular venues in this particular city.
Furthermore, for the latter case, the Client might benefit from exploring other analysis than clustering in order to better understand the subject.
Furthermore, for the latter case, the Client might benefit from exploring other analysis than clustering in order to better understand the subject.
Different Scaling
Different Scaling
At the outset of the project, the Client specifically requested to scale risk metrics by ‘population density’. Nevertheless, it is not really clear whether ‘population density’ is the best feature for scaling. There are two other possible alternatives in the dataset: ‘area’ and ‘population’. An alternative scaling might yield a different picture about the risk profile of the neighbourhoods. For the second round of the study I would strongly suggest that the Client explore other scaling alternatives as well.
At the outset of the project, the Client specifically requested to scale risk metrics by 'population density'. Nevertheless, it is not really clear whether 'population density' is the best feature for scaling. There are two other possible alternatives in the dataset: 'area' and 'population'. An alternative scaling might yield a different picture about the risk profile of the neighbourhoods. For the second round of the study I would strongly suggest that the Client explore other scaling alternatives as well.
As a reminder, the characteristics of these three features’ data were presented at the stage of Data Understanding.
As a reminder, the characteristics of these three features' data were presented at the stage of Data Understanding.
Effective Data Science Project Management Policy Making
Effective Data Science Project Management Policy Making
At last, from the perspective of an effective Data Science project management, I would recommend that the Client should incorporate into their data analysis policy the following two lessons from this project.
At last, from the perspective of an effective Data Science project management, I would recommend that the Client should incorporate into their data analysis policy the following two lessons from this project.
- When a basic tool can achieve the intended objective, it would be cost-effective to embrace it in deriving a conclusion/inference, rather than blindly implementing an advanced tool, such as machine learning. When a basic tool can achieve the intended objective, it would be cost-effective to embrace it in deriving a conclusion/inference, rather than blindly implementing an advanced tool, such as machine learning.
- Unless we are certain that the given data is suitable for the design of a machine learning model — or whatever model, actually — it might be unproductive to run it. In such a case, there would be no point in wasting the precious resource to end up yielding a potentially misleading result. Unless we are certain that the given data is suitable for the design of a machine learning model — or whatever model, actually — it might be unproductive to run it. In such a case, there would be no point in wasting the precious resource to end up yielding a potentially misleading result.
Due to the hype for Machine Learning among the public, some clients demonstrate some blind craving for it, assuming that such an advanced tool would yield a superior result. Nevertheless, this project yielded a mixed set of answers of both ‘yes’ and ‘no’. Moreover, machine learning is not a panacea.
Due to the hype for Machine Learning among the public, some clients demonstrate some blind craving for it, assuming that such an advanced tool would yield a superior result. Nevertheless, this project yielded a mixed set of answers of both 'yes' and 'no'. Moreover, machine learning is not a panacea.
Especially since the Client demonstrated an exceptional enthusiasm towards Machine Learning for their future business decision making, I believe that it would be worthwhile reflecting these lessons in this report for their future productive conduct of data analysis.
Especially since the Client demonstrated an exceptional enthusiasm towards Machine Learning for their future business decision making, I believe that it would be worthwhile reflecting these lessons in this report for their future productive conduct of data analysis.
D. Final Products (D. Final Products)
Now, as the final products for this preliminary project, I decided to present the following summary materials — a pop-up Choropleth map, two scatter plots, and a summary table — that can help the Client use their domain expertise for their own analysis.
Now, as the final products for this preliminary project, I decided to present the following summary materials — a pop-up Choropleth map, two scatter plots, and a summary table — that can help the Client use their domain expertise for their own analysis.
D1. Pop Up Choropleth Map (D1. Pop Up Choropleth Map)
In order to summarize the results for all these objectives, I incorporated a pop-up feature into the choropleth map that I had created for the objective 1 and 2. Each pop-up would display the following additional information of the corresponding ‘non-outlier neighbourhood’.
In order to summarize the results for all these objectives, I incorporated a pop-up feature into the choropleth map that I had created for the objective 1 and 2. Each pop-up would display the following additional information of the corresponding 'non-outlier neighbourhood'.
- Name of the Neighbourhood Name of the Neighbourhood
- Cluster Risk Grade: to show ‘Centroid_Grade’, the cluster risk profile of the neighbourhood. Cluster Risk Grade: to show 'Centroid_Grade', the cluster risk profile of the neighbourhood.
- Top 3 Venue Categories Top 3 Venue Categories
The map below illustrates an example of the pop-up feature.
The map below illustrates an example of the pop-up feature.

For high risk ‘outlier neighbourhoods’, I controlled the pop-up feature, since the Client wants to exclude them from consideration.
For high risk 'outlier neighbourhoods', I controlled the pop-up feature, since the Client wants to exclude them from consideration.
As a precaution, the colour on the map represents the Risk Cluster, not the Venue Cluster. Since I refrained from generating Venue Cluster, there is no Venue Cluster information on the map. This map would allow the Client to explore the popular venue profile for each neighbourhood individually.
As a precaution, the colour on the map represents the Risk Cluster, not the Venue Cluster. Since I refrained from generating Venue Cluster, there is no Venue Cluster information on the map. This map would allow the Client to explore the popular venue profile for each neighbourhood individually.
D2. Scatter Plots (D2. Scatter Plots)
The following two scatter plots display the same underlying risk cluster structure: the first one with the names of neighbourhoods; the second without the names. In the first plot, the densely plotted names at the left bottom are obstructing the view of individual datapoints (‘non-outlier neighbourhoods’) in the first plot. In the second one without the name, the entire view of the cluster structure can be seen clearly.
The following two scatter plots display the same underlying risk cluster structure: the first one with the names of neighbourhoods; the second without the names. In the first plot, the densely plotted names at the left bottom are obstructing the view of individual datapoints ('non-outlier neighbourhoods') in the first plot. In the second one without the name, the entire view of the cluster structure can be seen clearly.


Top 5 Popular Summary (Top 5 Popular Summary)
In addition, I also include a summary table to present the top 5 popular venue categories for each neighbourhood, sorted by the cluster’s risk profile (in ascending order of Centroid_Grade) and the number of Foursquare venue response (in descending order of Venue Response). In this sorted order, the Client can view the list of neighbourhoods in the following manner: from the safest cluster to more risker ones; from presumably the commercially busiest neighbourhood to less busier ones.
In addition, I also include a summary table to present the top 5 popular venue categories for each neighbourhood, sorted by the cluster's risk profile (in ascending order of Centroid_Grade) and the number of Foursquare venue response (in descending order of Venue Response). In this sorted order, the Client can view the list of neighbourhoods in the following manner: from the safest cluster to more risker ones; from presumably the commercially busiest neighbourhood to less busier ones.
Since the table is very long, here I would present only the top 7 rows of the table.
Since the table is very long, here I would present only the top 7 rows of the table.
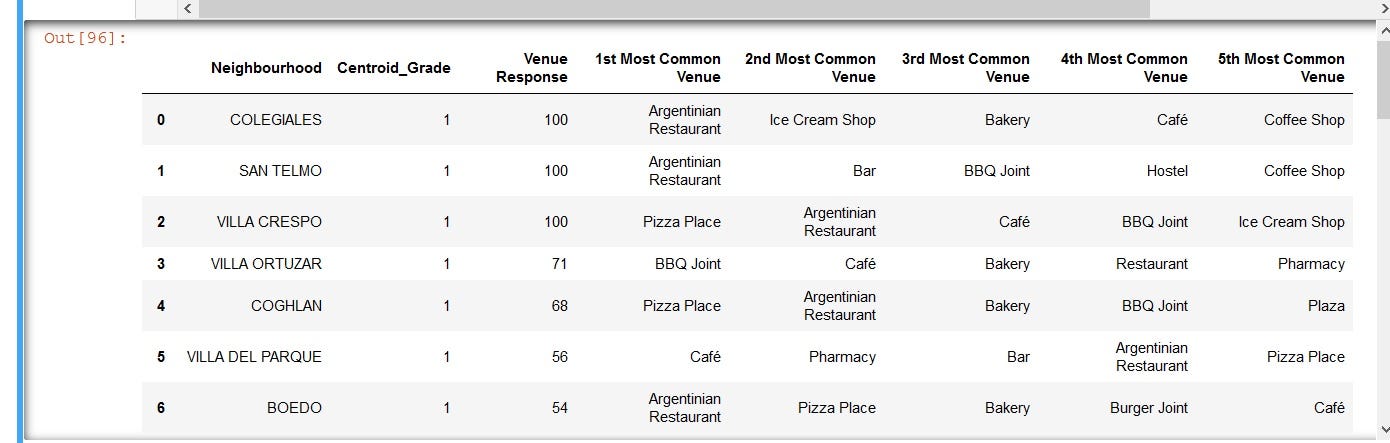
That’s all about my very first Data Science Capstone Project.
That's all about my very first Data Science Capstone Project.
Thank you very much for reading through this article.
Thank you very much for reading through this article.
Michio Suginoo
Michio Suginoo
翻译自: https://medium.com/@msuginoo/three-different-lessons-from-three-different-clustering-analyses-data-science-capstone-5f2be29cb3b2
普里姆从不同顶点出发
本文来自互联网用户投稿,该文观点仅代表作者本人,不代表本站立场。本站仅提供信息存储空间服务,不拥有所有权,不承担相关法律责任。如若转载,请注明出处:http://www.mzph.cn/news/389885.shtml
如若内容造成侵权/违法违规/事实不符,请联系多彩编程网进行投诉反馈email:809451989@qq.com,一经查实,立即删除!