如何成为数据科学家
Data science is one of the new, emerging fields that has the power to extract useful trends and insights from both structured and unstructured data. It is an interdisciplinary field that uses scientific research, algorithms, and graphs to uncover the patterns within the chaos and use these patterns to create amazing things.
数据科学是新兴的领域之一,可以从结构化和非结构化数据中提取有用的趋势和见解。 它是一个跨学科领域,它使用科学研究,算法和图形来揭示混乱中的模式,并使用这些模式来创造令人惊奇的事物。
As a data scientist, you need to know some basic mathematics, programming, and have a keen eye for patterns and trends finding. Due to the inter-disciplinary nature of the field, data scientists will find themselves working on different and broad aspects of technology.
作为数据科学家,您需要了解一些基本的数学知识,编程知识,并对模型和趋势发现有敏锐的洞察力。 由于该领域具有跨学科性质,因此数据科学家将发现自己正在研究技术的广泛领域。
Before we get into what you need to become a data scientist, let’s first talk about what a job in data science entails.
在深入探讨成为数据科学家所需的知识之前,让我们先谈谈数据科学工作需要做什么。
数据科学家做什么? (What do data scientists do?)
Working in data science is similar to riding a roller-coaster. Some aspects of the job are slow and steady, while others are fast and crazy. Other parts of it are just like going in a loop, and you repeat things over and over again.
从事数据科学工作类似于坐过山车。 工作的某些方面缓慢而稳定,而另一些方面则快速而疯狂。 它的其他部分就像循环一样,您一遍又一遍地重复。
Whenever a data scientist starts a new project, they will go through a known set of steps to get to their final conclusion.
每当数据科学家开始一个新项目时,他们都会经过一系列已知的步骤来得出最终结论。
Any data science project starts with data and ends with data, and in between, the magic happens.
任何数据科学项目都以数据开始,以数据结束,在这两者之间,魔术就发生了。
If you look through the internet, you will find many articles that address a different number of steps in a data science project. However, regardless of the number of steps, the core aspects are the same. For me, any data science project goes through 6 main steps.
如果您浏览互联网,则会发现许多文章涉及数据科学项目中不同步骤的内容。 但是,无论步骤数如何,核心方面都是相同的。 对我来说,任何数据科学项目都要经历6个主要步骤。

步骤№1:了解数据背景。 (Step №1: Understand data background.)
Whenever we start a data science project, we are usually aiming to solve a problem, enhance performance, or predict future trends. To do any of that, we first need to grasp the history of the source of the data and how it’s produced.
每当我们启动数据科学项目时,我们通常旨在解决问题,提高性能或预测未来趋势。 为此,我们首先需要掌握数据源的历史及其产生方式。
步骤№2:收集数据。 (Step №2: Collect data.)
Once we understood the background of that data, we need to collect the data to start working on it. Based on the nature of the project, there are different approaches to gather data. We can get it from a database, from an API, or — if you’re a beginner or just working on your skills — from an open data source. Another option to collect data is to scarp the wen for publically available information.
一旦了解了这些数据的背景,就需要收集数据以开始处理它。 根据项目的性质,有多种收集数据的方法。 我们可以从数据库,API或(如果您是初学者或只是在从事技能的人)从开放数据源中获取它。 收集数据的另一种方法是在网上获取公开信息。
步骤№3:清理并转换数据。 (Step №3: Clean and transform the data.)
Most — if not all — of the time, the data we collect from the source are pure and raw. That kind of data is not suitable to be used in algorithms and future steps. So, the first thing we do when we get new data is clean it up, categorize and tag it, and make sense of it.
在大多数时间(如果不是全部时间)中,我们从源头收集的数据是纯原始数据。 这类数据不适合用于算法和将来的步骤。 因此,当我们获取新数据时,我们要做的第一件事就是清理,分类和标记数据,并弄清数据。
步骤№4:分析和探索数据。 (Step №4: Analyze and explore the data.)
Once our data is clean and structured, we can start analyzing it and attempt to find patterns in it. This can be done by visualizing the data and looking for repetitions or spikes.
一旦我们的数据是干净的和结构化的,我们就可以开始分析它并尝试在其中找到模式。 这可以通过可视化数据并查找重复或峰值来完成。
步骤№5:对数据建模。 (Step №5: Model the data.)
We finally reach the magical step! After we explore and analyze our data, it’s time to feed into a machine learning algorithm and use it to predict future outcomes. This is truly the power of data science.
我们终于达到了神奇的一步 ! 在探索和分析我们的数据之后,是时候引入机器学习算法并用它来预测未来的结果了。 这确实是数据科学的力量。
步骤№6:可视化和交流结果。 (Step №6: Visualize and communicate results.)
Finally, and the most crucial step of the process is to visualize and present the results of the project effectively.
最后,该过程中最关键的一步是有效地可视化并呈现项目结果。
Once those steps are done, a new project comes in, and it’s time to start all over again.
完成这些步骤后,就会出现一个新项目,该是重新开始的时候了。
数据科学需要哪些技能? (What skills are needed for data science?)
Every step of the data project lifecycle requires a specific set of knowledge and skills. To better connect the skills needed, I will pair each phase of the project with the necessary skill to complete that step.
数据项目生命周期的每个步骤都需要一组特定的知识和技能。 为了更好地连接所需的技能,我将项目的每个阶段与必要的技能配对以完成该步骤。
To perform data investigation, you only need a curious mind, a pen, and a paper. You sit down and either ask the data source some questions to understand the data better or if it is an open-source data, read the documentation that accompanies the data.
要执行数据调查,您只需要好奇的头脑,一支笔和一张纸。 您坐下来,或者问数据源一些问题以更好地理解数据,或者如果它是开源数据,请阅读数据随附的文档。
To perform data collection, you will need to know how to communicate with databases and APIs. Understanding the basic structure and mechanics of such techniques will make your data collection a breeze. If you’re using an open-source dataset, then learning how to look for datasets and some good sources can make a huge difference.
要执行数据收集,您将需要知道如何与数据库和API通信。 了解此类技术的基本结构和机制将使您的数据收集轻而易举。 如果您使用的是开源数据集,那么学习如何查找数据集和一些好的资源可能会产生很大的不同。
To perform data cleaning, you need some good knowledge of basic data mining and cleaning techniques. You will need to tag your data and categorize it properly. Moreover, you can use regular expressions to look for misspellings or use special tools created to make this process easier for you.
要执行数据清理 ,您需要一些基本的数据挖掘和清理技术方面的知识。 您将需要标记数据并进行正确分类。 此外,您可以使用正则表达式查找拼写错误,也可以使用创建的特殊工具使此过程更轻松。
To perform data exploration, you will need some basics statistics and probability theory. Some knowledge of data visualization and experimental design can help you a lot at this stage.
要进行数据探索,您将需要一些基础统计和概率论。 在此阶段,一些数据可视化和实验设计方面的知识可以为您提供很多帮助。
To perform data modeling, you will need to know a few machine learning algorithms and how they work. You don’t need to understand everything 100%; if you can use them correctly and apply them to the correct form of data, you will be fine.
要执行数据建模,您将需要了解一些机器学习算法及其工作方式。 您无需100%理解所有内容; 如果您可以正确使用它们并将它们应用于正确的数据形式,则可以。
Finally, to perform data communication, you might use some essential science communication 101. Which are knowing your audience, their background knowledge, and choosing wimple words to explain complex concepts? Additionally, effective data visualization can make or break your project at this stage.
最后, 要进行数据交流,您可能会使用一些基本的科学交流101。哪些能了解您的听众,他们的背景知识以及选择愚蠢的单词来解释复杂的概念? 此外,有效的数据可视化可以在此阶段创建或破坏您的项目。
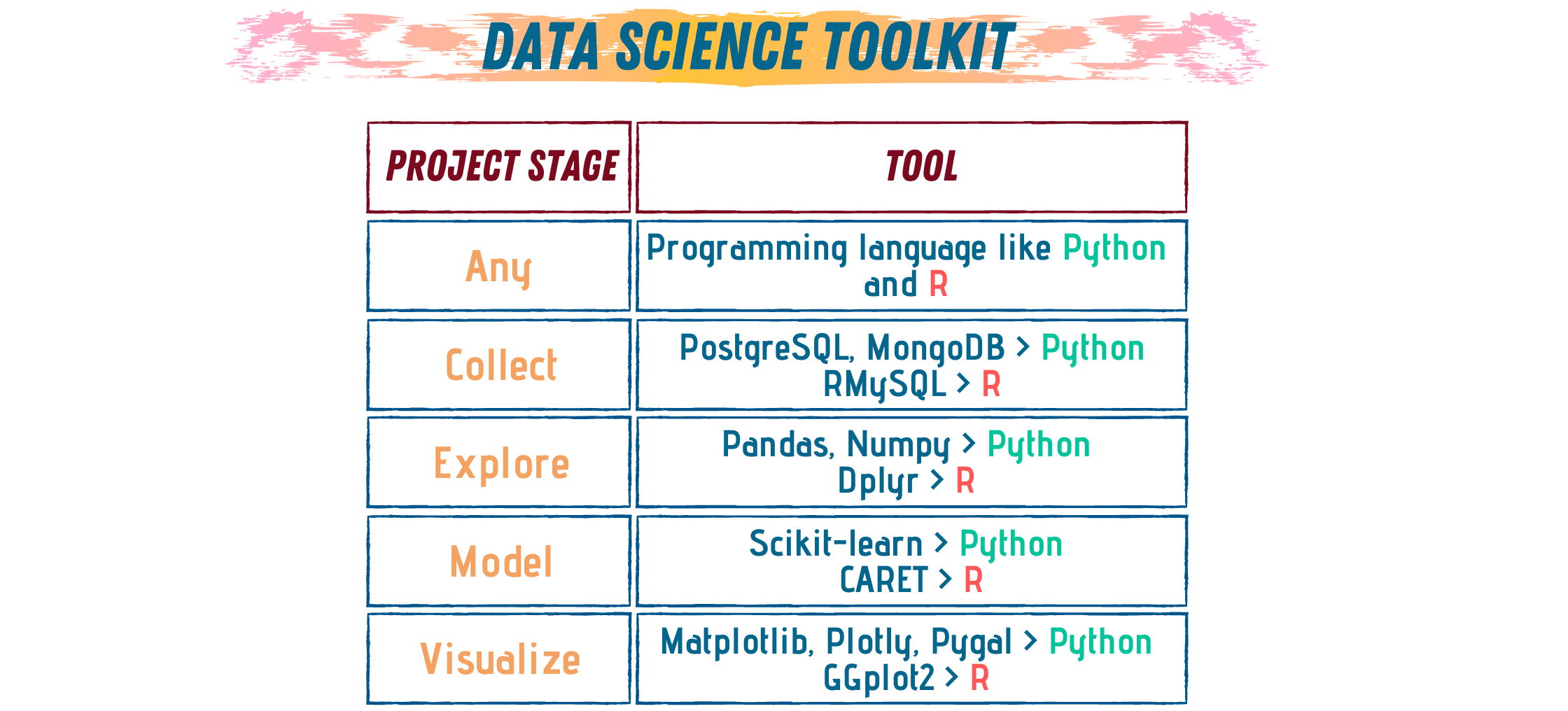
技术工具 (Technical tools)
Some of the skills we just talked about require a programming language, an algorithm, or special packages.
我们刚才谈到的一些技能需要编程语言,算法或特殊程序包。
- Programming languages: Python, R. 编程语言:Python,R。
For handling and creating databases: MySQL, PostgreSQL, MongoDB, or SQLite in Python. If you’re using R, then you can use RMySQL.
用于处理和创建数据库:Python中的MySQL , PostgreSQL , MongoDB或SQLite 。 如果使用R,则可以使用RMySQL。
Packages for data exploration and transformation: in Python Pandas, Numpy, or Scipy. Or in R GGplot2 and Dplyr.
数据探索和转换的软件包:Python Pandas , Numpy或Scipy 。 或在R GGplot2和Dplyr中。
Python libraries for visualizations: Matplotlib, Plotly, Pygal.
用于可视化的Python库: Matplotlib , Plotly , Pygal 。
Basic machine learning package for Python Scikit-learn and CARET in R.
R中用于Python Scikit-learn和CARET的基本机器学习包。
结论 (Conclusion)
You don’t need to know everything about statistics, math, machine learning, or be a professional programmer to start with data science. You only need the basics of this knowledge. As you work on different projects and build your profile, your knowledge base will expand, and your “data science sense” will improve automatically.
您不需要了解统计,数学,机器学习的全部知识,也不需要成为专业的程序员就可以开始数据科学。 您只需要这些知识的基础。 当您从事不同的项目并建立个人档案时,您的知识库将会扩大,并且您的“数据科学意识”也会自动提高。
So, don’t be intimidated by the field, or by how many things you need to “master” to be a good data scientist. Just start with the basics and work your way through to the advanced topics. Be patient and give it your all, and you will get there.
因此,不要被该领域或要成为一名出色的数据科学家所需要掌握的几件事所吓倒。 只是从基础开始,然后逐步学习高级主题。 耐心点,全力以赴,您将到达那儿。
翻译自: https://towardsdatascience.com/what-do-you-need-to-know-to-become-a-data-scientist-1ed52e0e1ad
如何成为数据科学家
本文来自互联网用户投稿,该文观点仅代表作者本人,不代表本站立场。本站仅提供信息存储空间服务,不拥有所有权,不承担相关法律责任。如若转载,请注明出处:http://www.mzph.cn/news/389876.shtml
如若内容造成侵权/违法违规/事实不符,请联系多彩编程网进行投诉反馈email:809451989@qq.com,一经查实,立即删除!