火种 ctf
Originally published at https://www.linkedin.com on March 27, 2020 (data up to date as of March 20, 2020).
最初于 2020年3月27日 在 https://www.linkedin.com 上 发布 (数据截至2020年3月20日)。
Day 3 of social distancing.
社会疏离的第三天。
As I sit on my couch scrolling through my Instagram feed to see yet another drawing of an orange — apparently the latest Instagram challenge to pass the time — I was starting to get… bored. Who would’ve thought that an INTP like myself would succumb to boredom in day 3 of social distancing?
当我坐在沙发上滚动查看我的Instagram提要时,看到另一幅橙色的图画(显然是Instagram最新挑战),我开始感到…… 无聊 。 谁会想到像我这样的INTP会在社交疏远的第三天屈服于无聊?
With no cool restaurants to explore, no plans with friends to hang out, no gyms to go to anytime soon — why not start a project to pass the time?
没有很酷的餐厅可供探索,没有与朋友闲逛的计划,没有健身房可供短期使用-为什么不启动一个打发时间的项目?
But, what project? I know I wanted to do something that would allow me to gain insight on some aspect of my life, and what’s more relevant to a 20 year old’s life than dating? In today’s ultra-digital world, dating has become synonymous with Tinder. I mean, how else are we supposed to meet and connect with people nowadays? Through physical and social communities like friends, mutuals, school, and work as has literally been the case for hundreds of generations prior? Nope, that’s crazy.
但是,什么项目? 我知道我想做些能让我对生活的某些方面有深入了解的事情,与20岁的生活比约会更重要的是什么? 在当今的超数字世界中,约会已成为Tinder的代名词。 我的意思是,我们今天应该如何与其他人见面并建立联系? 通过像朋友,互助,学校和工作这样的物质和社会社区,几百代人以前确实是这样? 不,那太疯狂了 。
Tinder allows us to connect with people within our communities that we would never have met otherwise — for better or for worse. And as with many social media apps, Tinder allows you to request your own personal data.
Tinder使我们能够与社区中的人们保持联系,否则我们将再也见不到,无论好坏。 与许多社交媒体应用程序一样,Tinder允许您请求自己的个人数据。
And so I did.
所以我做到了。
火种数据 (Tinder Data)
The requested Tinder data was in JSON format, and follows this simplified structure:
请求的Tinder数据为JSON格式,并遵循以下简化结构:
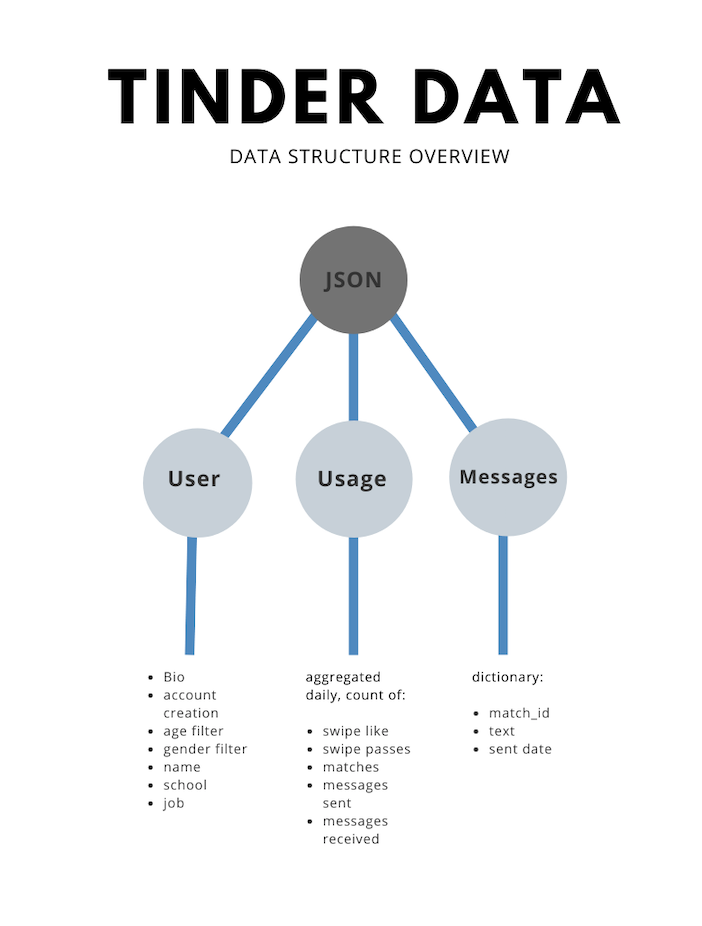
Reading in the data into Python with the following script:
使用以下脚本将数据读入Python:
import json with open('data.json') as json_file:
data = json.load(json_file)
Now, the first problem was taking this data structure and converting it to one that I could easily traverse through to analyze. Because Usage is simply a count aggregated daily, it was natural to convert this into a standard tabular data structure with rows as dates and columns as the aforementioned features.
现在,第一个问题是采用这种数据结构并将其转换为我可以轻松遍历以进行分析的结构。 由于“用法”只是每天汇总的计数,因此很自然地将其转换为标准的表格数据结构,其中行作为日期,列作为上述功能。
import pandas as pd
df = pd.DataFrame(list(data['Usage']['app_opens'].keys())) for x in list(data['Usage'].keys()):
df[x]= list(data['Usage'][x].values())
Here’s the first five rows of the data frame — aka my first 5 days on Tinder:
这是数据框的前五行,也就是我在Tinder上的前五天:
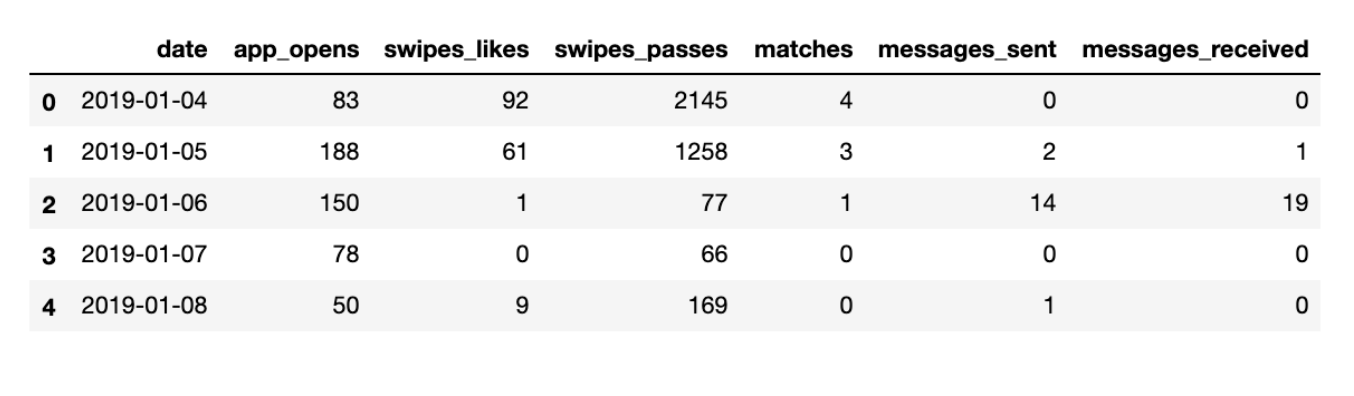
With the Messages, however, I wanted to explore other alternatives. Since an individual message can be viewed as an object with attributes text and sent date, I defined a Message Class/Object, and stored these in a dictionary where the key indicated the unique match ID.
但是,对于“消息”,我想探索其他替代方法。 由于可以将一条单独的消息视为具有属性文本和发送日期的对象,因此我定义了一个消息类别/对象,并将它们存储在字典中,其中的键表示唯一的匹配ID。
class Message:
'''Fields: Text (Str)
Date (Datetime)'''
def __init__(self, text, date):
self.text = text
self.date = date
def __repr__(self):
message_rep = "{}: {}"
return message_rep.format(self.date, self.text)
message_dict={}
for x in data['Messages']:
match_id=x['match_id'].split()[-1]
sent = []
for messages in x['messages']:
sent_date = " ".join(messages['sent_date'].split()[0:-1])[:-3]
sent.append(Message(messages['message'].lower(),sent_date))
message_dict[match_id]=sent
Now, we need more Python to parse through the messages to derive basic insights. Here’s an excerpt containing the basic idea:
现在,我们需要更多的Python来解析消息以得出基本见解。 以下是包含基本思想的摘录:
day_count, time_count, emoji_count = {}, {}, {}
day_time, date_count, word_count = {}, {}, {}
for matches in message_dict:
messages = message_dict[matches]
for msg in messages:
date=msg.date.split(" ")
day=date[0][:-1]
time = date[-1][:2]+':00'
dt="-".join(date[1:4])
words=msg.text.split(" ")
check_lst = [[day, day_count], [time, time_count],
[day_time, day_time],[dt, date_count],
[words, word_count]]
i=0
while i < 4:
x=check_lst[i]
key=x[0]
dictionary = x[1]
if key not in dictionary.keys():
dictionary[key]=1
i=i+1
else:
dictionary[key]=dictionary[key]+1
i=i+1
for x in words:
t = str.maketrans(dict.fromkeys(string.punctuation))
x = x.translate(t)
stripped = list(x)
for char in stripped:
if char in emojis:
if char not in emoji_count.keys():
emoji_count[char]=1
else:
emoji_count[char]=emoji_count[char]+1
分析与见解 (Analysis & Insights)
Quick stats as of March 20th 2020:
截至2020年3月20日的快速统计数据:
- 10,083 total app opens 共有10,083个应用打开
- Swiped right on 3,331 profiles, with a daily max of 92 on January 4 2019 在3,331个配置文件上向右滑动,2019年1月4日每天最多92个
- Swiped left on 38,132 profiles, with daily max of 2,145 profiles on January 4 2019 向左滑动38,132个配置文件,2019年1月4日每天最多2,145个配置文件
- 349 matches, with daily max of 12 matches on March 18 2020 349场比赛,2020年3月18日每天最多12场比赛
- 1,164 total messages sent 共发送1,164条消息
- 1,289 total messages received 共收到1,289条消息
- 125 unique conversations 125个独特的对话
- 32 social media/number exchanges 32个社交媒体/号码交换
- 16 meet ups 16个聚会
- countless dollars spent on bubble tea 花在泡沫茶上的钱不计其数
Traversing through my sent messages, we get the following word cluster:
遍历我发送的消息,我们得到以下单词簇:
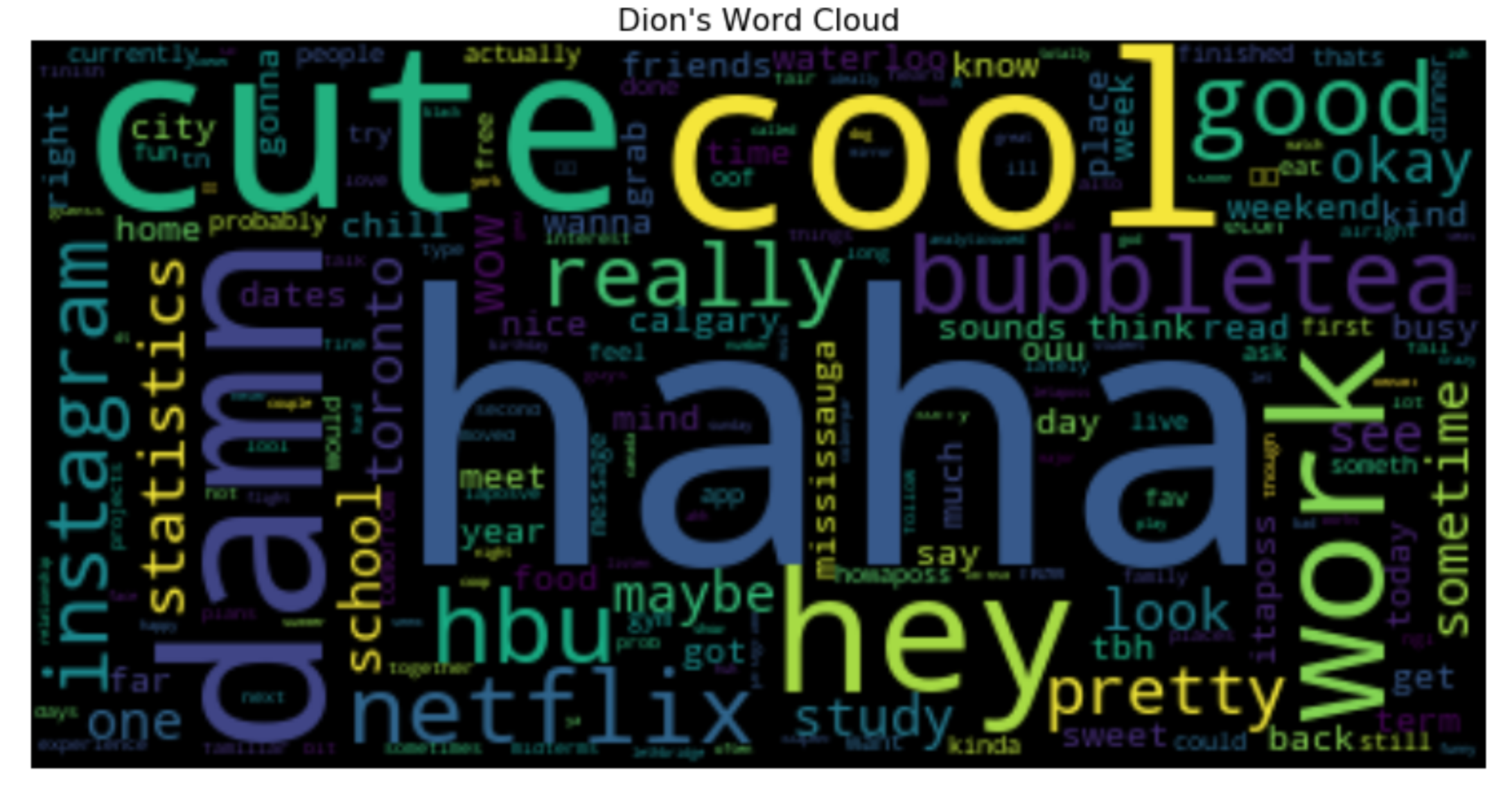
Looking at my top words:
看我的热门话:
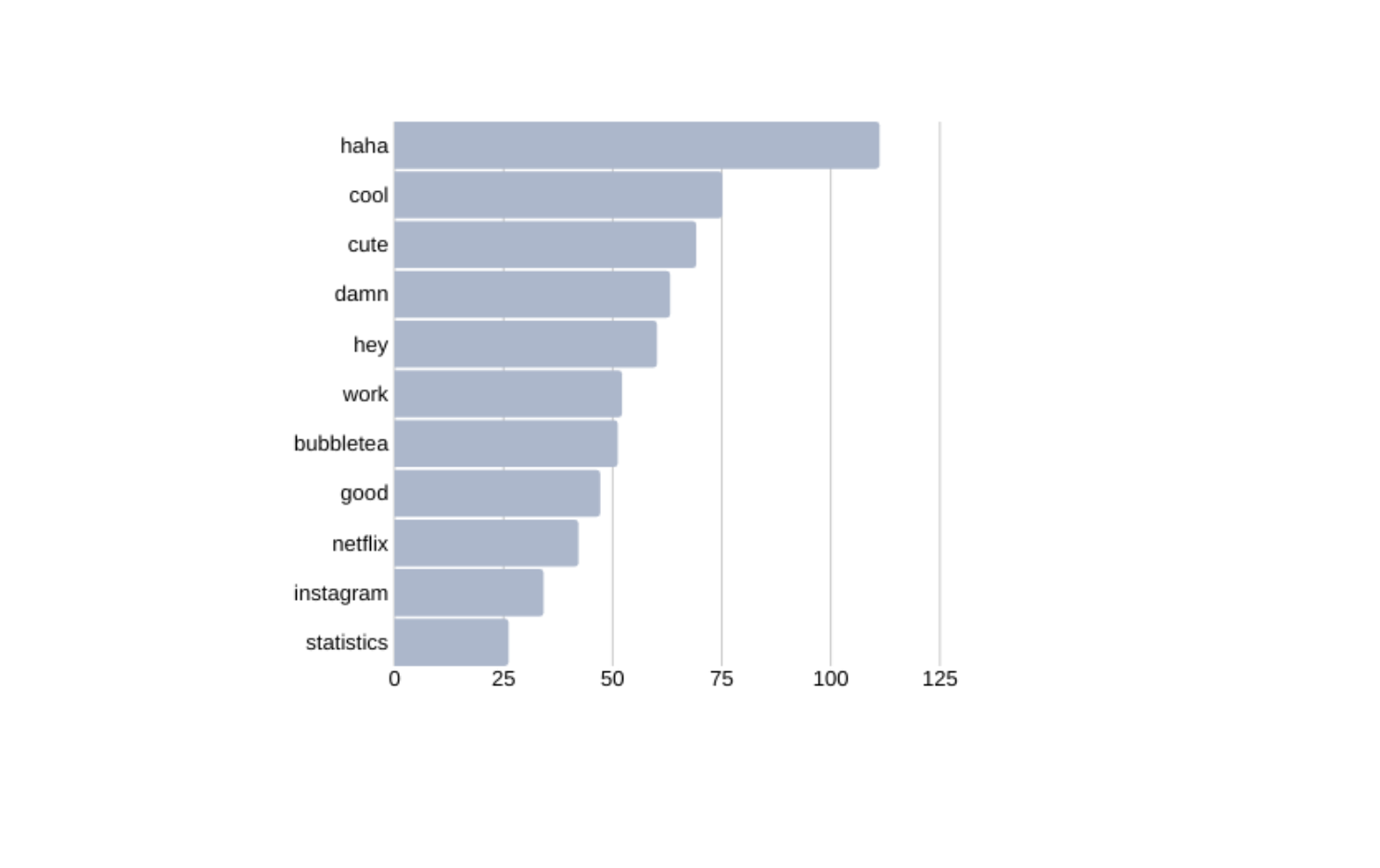
“damn you’re cute wanna grab bubbletea ? haha”
“该死的你很可爱,想去买泡泡茶吗? 哈哈”
Interesting. Seems fairly normal in the context of Tinder. Now, I’m curious as to why statistics is one of my top words…
有趣。 在Tinder的上下文中似乎很正常。 现在,我很好奇为什么统计是我的热门词汇之一……
Now, among the messages sent, about 4% of these were emojis. Evidently, emojis are well integrated into digital messaging. Here are my top 5 sent emojis:
现在,在发送的消息中,其中约4%是表情符号。 显然,表情符号已很好地集成到数字消息中。 这是我发送的前5个表情符号:
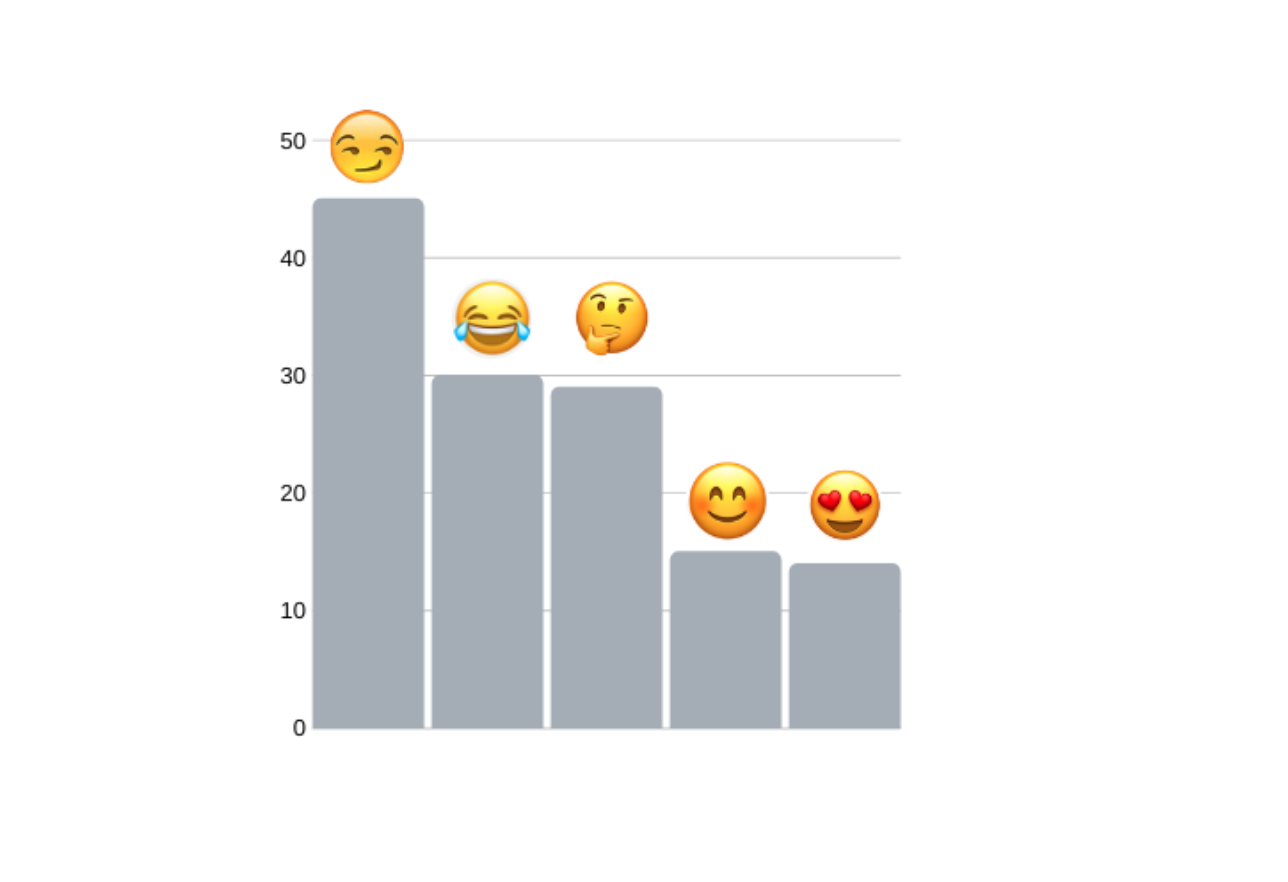
Moreover, data indicates that 15% of my sent messages had only 6 words — with 38% of my sent messages falling between the 5–7 word count range.
此外,数据表明,我发送的邮件中有15%仅包含6个单词-我发送的邮件中有38%位于5-7个字数范围内。
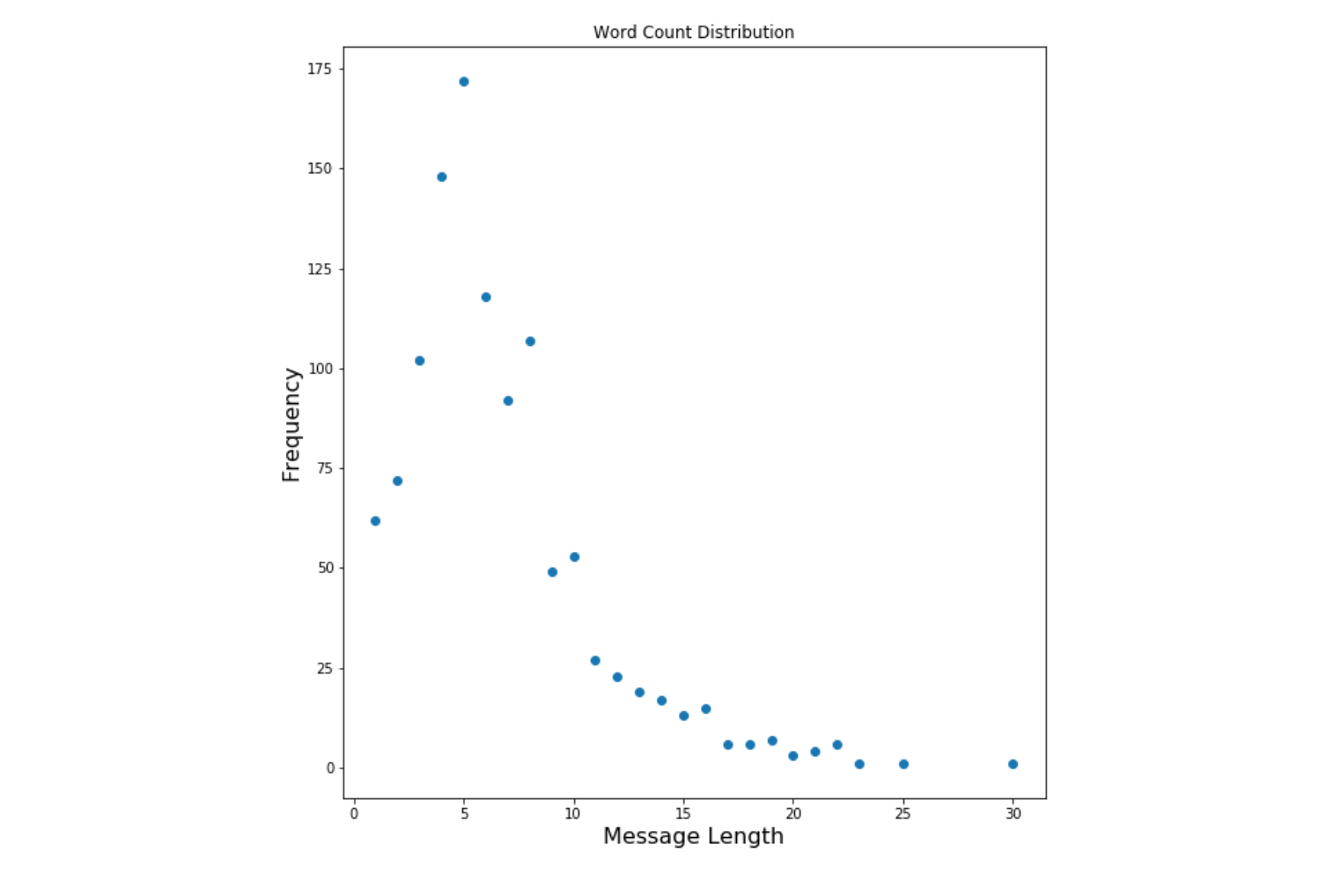
Looking at the distribution of conversation length measured in days, we see a left-skewed distribution — with 67% of conversations having tenure of less than one day.
查看以天为单位的会话长度分布,我们看到一个左偏分布-67%的会话的任期少于一天。

Among these single day conversations, a majority of them are dead-end: in other words, no messages were sent after my initial recorded message.
在这些单日对话中,大多数对话都是死胡同:换句话说,在我最初记录的消息之后没有发送任何消息。

Now, before hammering down on my one-liners, there is a slight caveat: because I only have data on my sent messages, I used my first and last message within a match as a proxy for conversation length. As such, it is unclear which participant actually ended the conversation. So these ‘no responses’ could have been messages that I didn’t follow up on.
现在,在敲定单行代码之前,有一点警告:由于我的发送消息中只有数据,因此我将比赛中的第一条和最后一条消息用作对话长度的代理。 因此,不清楚哪个参与者实际结束了对话。 因此,这些“没有回应”可能是我没有跟进的消息。
In fact, looking at the count of messages sent versus received indicates that my messages are generally answered — at least when aggregating on the monthly level. So maybe my one liners are somewhat effective — sureeee.
实际上,查看已发送消息与已接收消息的数量表明,我的消息通常得到答复-至少在按月汇总时会得到答复。 因此,也许我的一支班轮比较有效- 保证人 。
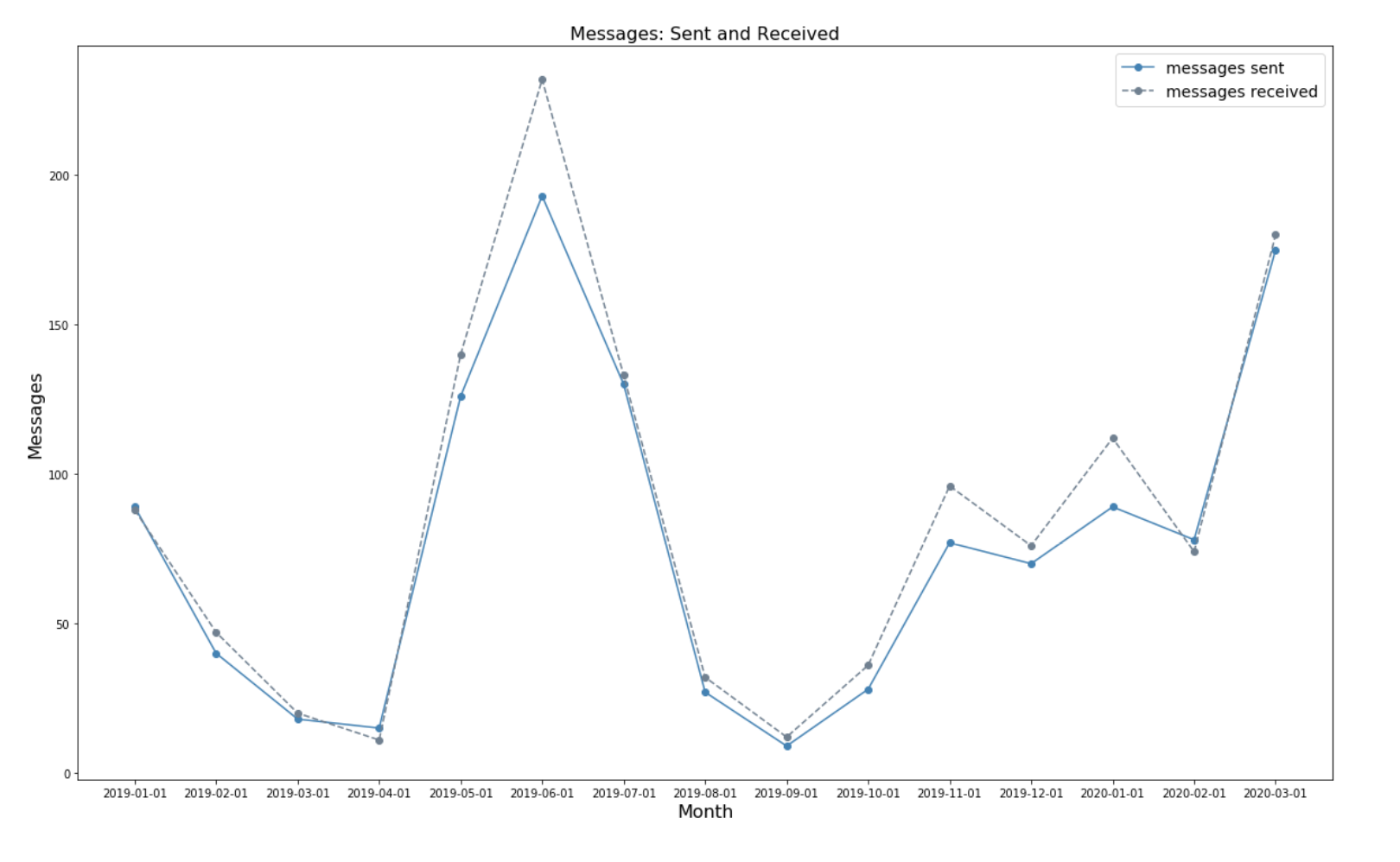
When are these messages actually sent out?
这些消息何时真正发出?
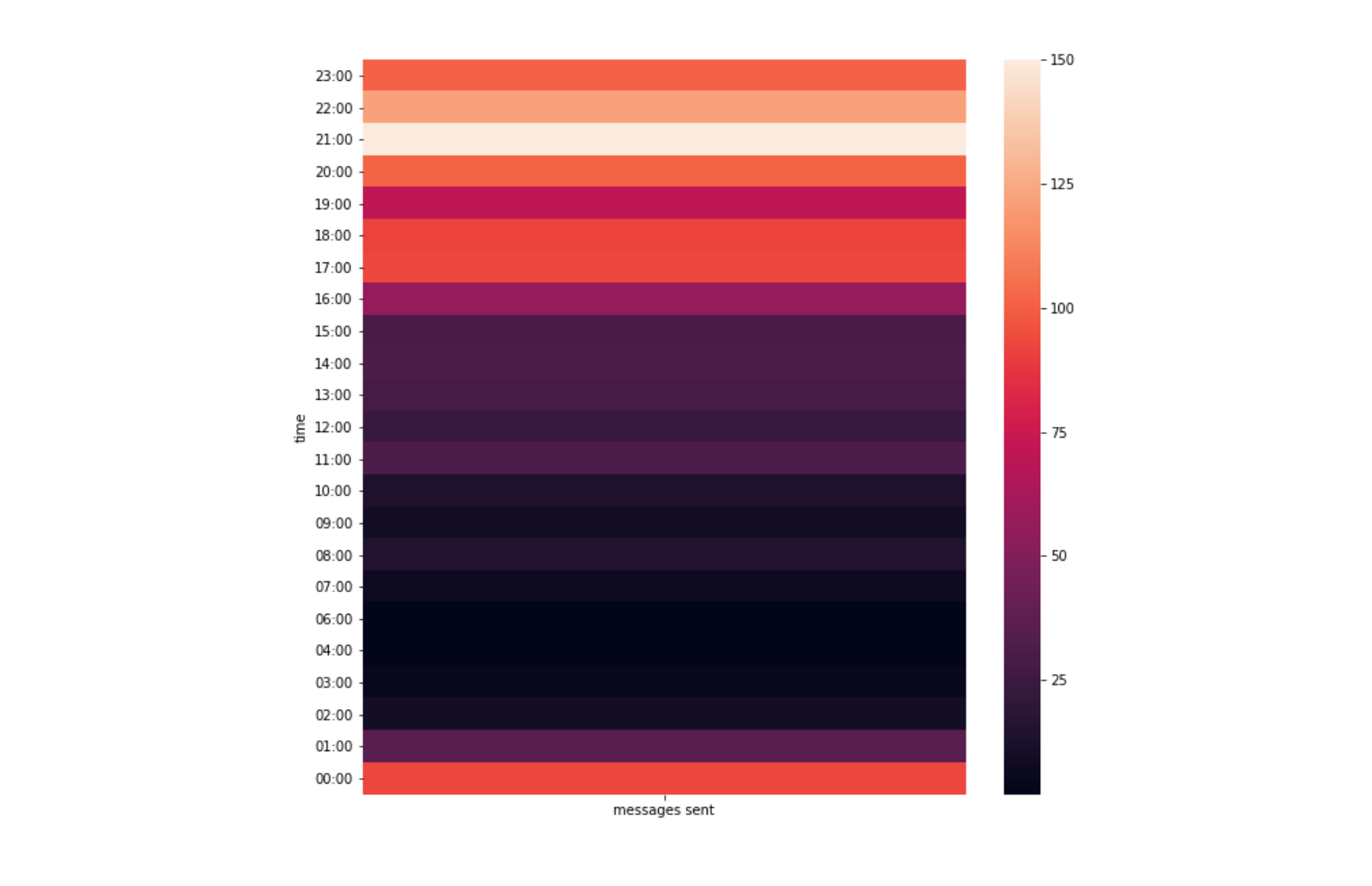
Data indicates that peak messaging time occurs at 9 pm.
数据表明高峰消息传递时间发生在晚上9点。
Cool — but these insights are only applicable once a match has actually occurred. We all know that 90% of Tinder consists of swiping.
很酷-但这些见解仅在实际发生匹配后才适用。 我们都知道90%的Tinder是刷卡。
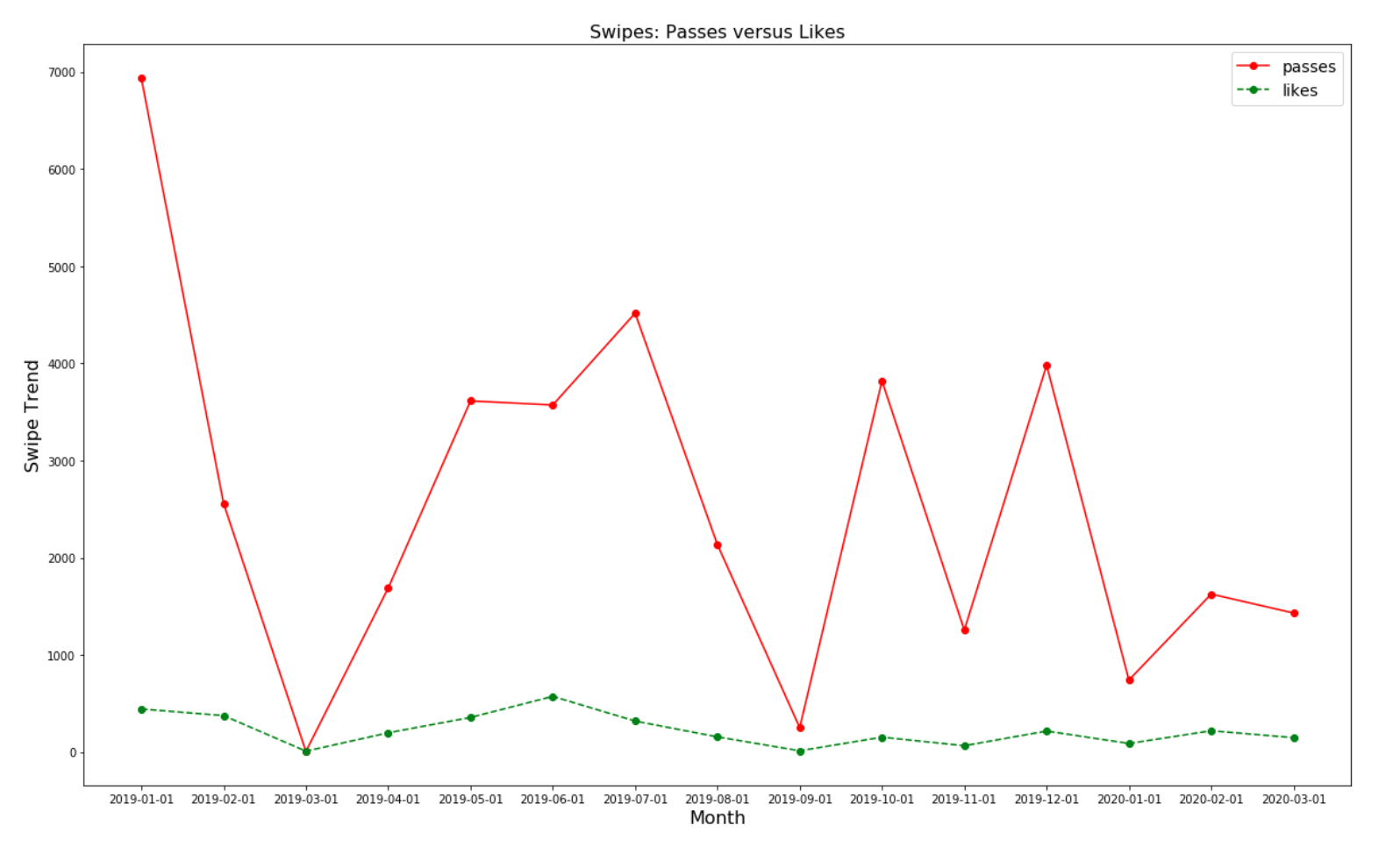
It’s interesting to see that 18% of total swipes were done in my first month of Tinder.
有趣的是,在Tinder的第一个月中,刷卡总数就达到了18%。
Defining match rate as the proportion of matches to swipe rights, we see that my match rate generally hovers at around 12.5% — with the highest match rate of 45% in March 2019 despite its low matches.
将匹配率定义为匹配权与滑动权的比例,我们看到我的匹配率通常徘徊在12.5%左右,尽管匹配率较低,但2019年3月的最高匹配率为45%。

Assuming independence in swipes and holding the probability of a match fixed, we can think of each swipe right as a Bernoulli trial — where a successful outcome is a match.
假设刷卡独立,并且将比赛的可能性固定不变,那么我们可以将每次刷卡都视为一次伯努利试验-成功的结果就是一场比赛。
Mathematically, we have a random variable, Y, that follows a binomial distribution:
在数学上,我们有一个随机变量Y,它遵循二项式分布:
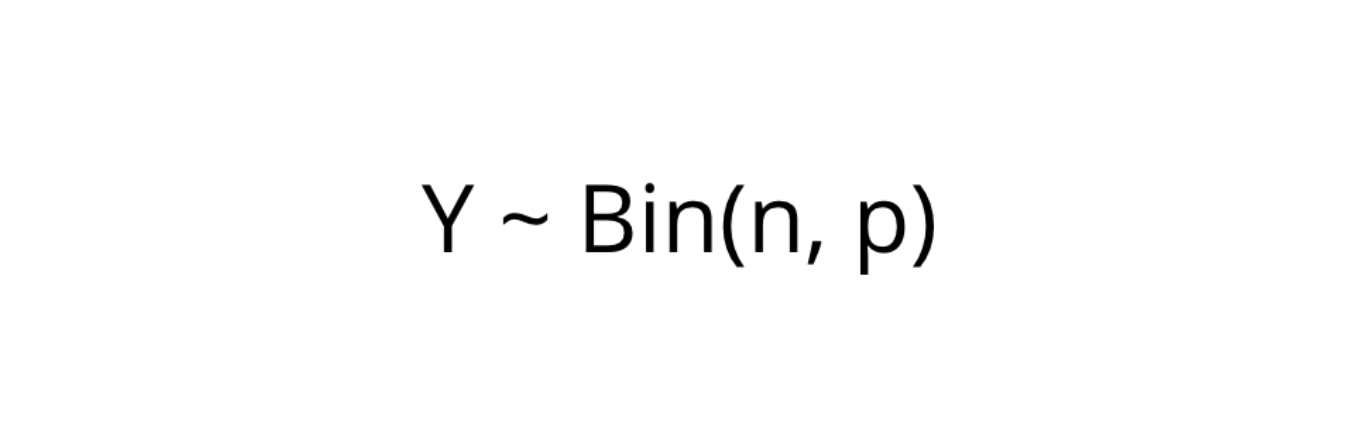
Or in our context:
或在我们的上下文中:

Given my Tinder data and assuming a fixed probability of success (p), the maximum likelihood estimate of the parameter p is simply the estimated match rate.
给定我的Tinder数据,并假设成功的概率为固定值(p),则参数p的最大似然估计值就是估计的匹配率。

Holding the number of my received swipe rights constant, we can construct the following cumulative binomial probability distributions:
在我收到的刷卡权利数量不变的情况下,我们可以构建以下累积二项式概率分布:

The figure above shows the probability of at least one match given a fixed probability of success, p. We can see that the probability of at least one match increases with the number of swipe rights. In other words, a match is inevitable as you swipe right — this is, of course, holding the number of received swipe rights constant. This resulting convergence is a consequence of the Law of Large Numbers.
上图显示了在给定固定成功概率p的情况下至少一场比赛的概率。 我们可以看到,至少一项匹配的可能性随刷卡权限的数量而增加。 换句话说,当您向右滑动时,匹配是不可避免的-当然,这将使接收到的滑动权限的数量保持恒定。 最终的收敛是大数定律的结果。
Given my current swiping behaviour (p=0.10), it would take at least 30 swipes to get at least one match — emphasis on at least: meaning the number of matches could range from 1 to the number of swipe rights inclusive.
考虑到我目前的滑动行为(p = 0.10),至少需要进行30次滑动才能获得至少一场比赛- 至少要强调:意味着比赛次数的范围可以从1到包括滑动次数在内。

Holding the number of my received swipe rights constant, a quick way to increase the probability of at least one match is to increase the number of swipe rights given. However, more doesn’t necessarily mean better: the trade-off between quality and quantity is more nuanced, so I’ll leave it at that.
保持我收到的刷卡权利数量不变,一种增加至少一场比赛的可能性的快速方法是增加所给定的刷卡权利数量。 但是,更多并不一定意味着更好:质量和数量之间的权衡更加细微,因此我将保留它。
A natural question that follows is how many of these matches actually lead to coffee or bubble tea? Data indicates a 12.8% conversion rate among my engaged matches. A 95% confidence interval estimate indicates a lower bound of 7% and an upper bound of 19% — the 6% margin of error could be telling of external factors, such as proximity, that could affect one’s interest to meet up.
随之而来的自然问题是,这些匹配中有多少实际上产生了咖啡或泡泡茶? 数据显示我参与的比赛中的转化率为12.8%。 95%的置信区间估计值指示下限为7%,上限为19%-6%的误差幅度可能表示外界因素(例如接近程度)可能会影响一个人满足兴趣的外部因素。
Now, assuming independence among engaged matches and that each person is equally open to meet up, we can think of this as yet again another Bernoulli trial — where a successful outcome is a meet up.
现在,假设参与比赛的独立性,并且每个人都同样愿意聚会,我们可以将其视为伯努利的又一次审判-成功的结局就是聚会。

Given my Tinder data and assuming a fixed probability of success (p), the maximum likelihood estimate of the parameter p is simply the estimated conversion rate.
给定我的Tinder数据并假设成功的概率为固定值(p),则参数p的最大似然估计值就是估计的转换率。
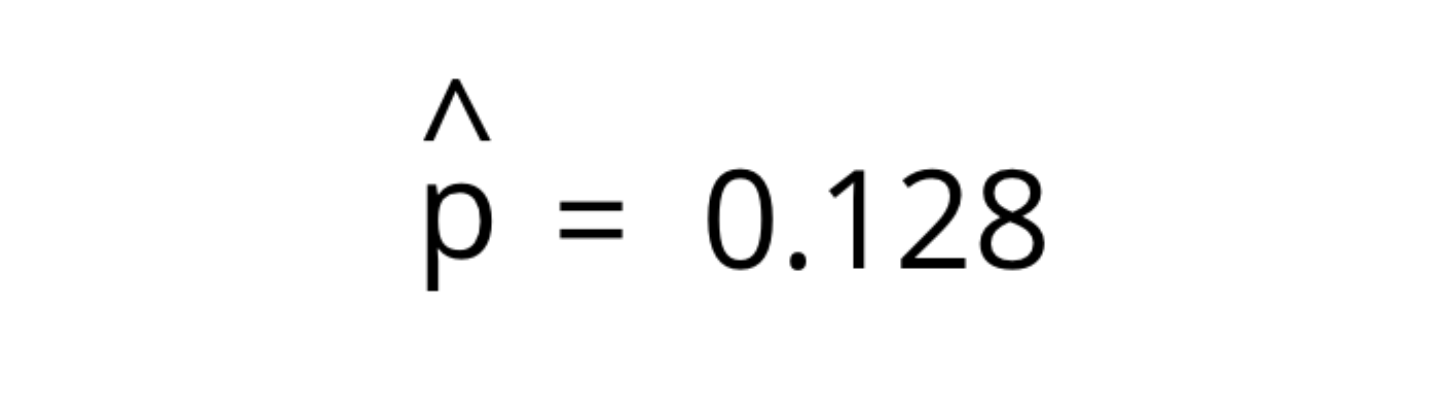
With these assumptions, we can make inferences on future outcomes such as calculating the probability of getting x number of meet ups — in other words, Prob(meet up = x | p = 0.128).
有了这些假设,我们就可以推断出未来的结果,例如计算获得x次见面的概率—换句话说,Prob(见面= x | p = 0.128)。
Pretty cool.
很酷
This is especially useful when it comes to allocating budgets for dates. Personally, first dates for me are around the $10 — $20 ball park — though, the variance on that is somewhat high. Assuming that I allocate $35 per month on dates and each date is $15, we can run simulations with 100 engaged matches over 6 months:
在分配日期预算时,这特别有用。 就我个人而言,第一次约会大约是10美元(20美元球场),但是,这方面的差异有些大。 假设我每月在日期上分配$ 35,每个日期为$ 15,我们可以在6个月内进行100次参与式比赛的模拟:

Since the number of engaged matches is large (n=100), the binomial distribution can be approximated by a Gaussian probability density. This resulting convergence in distribution is a consequence of the Central Limit Theorem.
由于参与比赛的数量很大(n = 100),因此可以通过高斯概率密度来近似二项式分布。 分布的最终收敛是中央极限定理的结果。
The probability of a deficit can be calculated as the area under the curve to the left of the red dotted line. Hence we can calculate this probability easily using the Gaussian approximation:
赤字的概率可以计算为红色虚线左侧曲线下方的面积。 因此,我们可以使用高斯近似轻松地计算该概率:

Since the budget remaining is a linear function of meet ups — which we estimate through a Gaussian random variable — then, the budget remaining also follows a Gaussian distribution:
由于剩余预算是满足率的线性函数(我们通过高斯随机变量估算),因此,剩余预算也遵循高斯分布:

With these assumptions, the probability of a deficit is 0.3594. Yikes — this is somewhat concerning given that I’m on a student budget.
根据这些假设,出现赤字的概率为0.3594。 Yikes-考虑到我的学生预算有限,这有点令人担忧。
So, it’s probably not financially viable to message 100 matches over 6 months given my current conversion rate. To stay on budget, I either: decrease the number of messaged matches or decrease my conversion rate. Hmm, tough call — I’d have to go with the former.
因此,考虑到我目前的转化率,在6个月内发送100个匹配消息可能在财务上不可行。 为了节省预算,我要么:减少信息匹配的次数,要么降低转化率。 嗯,艰难的举动-我不得不跟前一个去。
Tweaking the parameters in the binomial simulation we get the following results:
在二项式仿真中调整参数可获得以下结果:
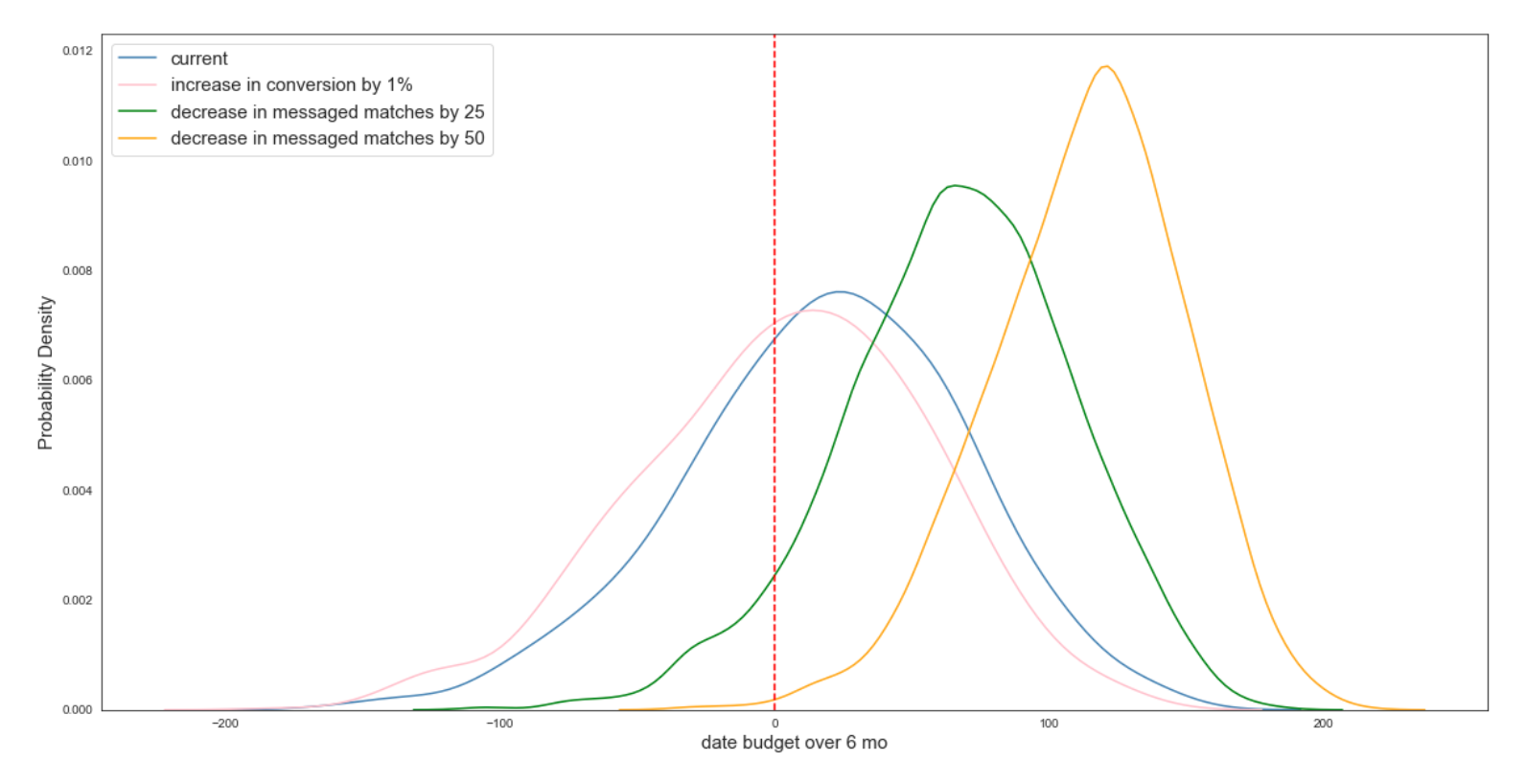
Now, the probability of going over budget when I reduce my engagement to 75 matches is 0.06 (green density) — much better. Having said that, I also don’t want to end up with a big surplus since that would imply little to no dates (yellow). Hence, I should engage with 75 to 85 matches over the course of 6 months to fully utilize my budget.
现在,当我将参与度降低到75场比赛时,超出预算的可能性为0.06(绿色密度),好得多。 话虽如此,我也不想结余很多,因为那意味着很少甚至没有约会(黄色)。 因此,我应该在6个月的时间内进行75到85场比赛,以充分利用我的预算。
Cool. Now I have some new insights about my current Tinder behaviour — however, by no means is this analysis exhaustive. If you happen to have Python installed and have your own personal Tinder data — or if you just want to look at the back-end logic of the Python functions used in this analysis — feel free to check out the code that I wrote for this project:
凉。 现在,我对当前的Tinder行为有了一些新见解-但是,该分析绝不是详尽无遗的。 如果您恰好安装了Python并拥有自己的个人Tinder数据-或仅想查看此分析中使用的Python函数的后端逻辑-请随时查看我为该项目编写的代码:
https://github.com/dionbanno/dion_creates
https://github.com/dionbanno/dion_creates
对进一步项目的建议: (Recommendations for further projects:)
- Can we perform A/B testing on certain key words and phrases to see if they increase the probability of a response/meet up? 我们可以对某些关键词和短语进行A / B测试,以查看它们是否增加了回应/见面的可能性?
- It would be cool to have a repository of individual Tinder data classified per user attribute such as location, gender, age, etc. and doing a regression analysis to see if certain user attributes affect success 拥有按用户属性(例如位置,性别,年龄等)分类的单个Tinder数据存储库,并进行回归分析以查看某些用户属性是否影响成功,这将很酷。
最后的话 (Final words)
Data from this analysis indicates that 64% of matches go un-messaged. So shoot your shot. Go ignite those matches — who knows? It might be worth while.
来自该分析的数据表明,有64%的匹配未发送消息。 因此,射击。 点燃那些比赛-谁知道? 也许值得。
Feel free to leave your comments, and connect with me on LinkedIn. I’d also be curious to know — what metrics would you have chosen to analyze, and how?
随时发表您的评论,并在LinkedIn上与我联系。 我也很想知道-您将选择分析哪些指标,以及如何选择?
翻译自: https://medium.com/swlh/analyzing-my-tinder-data-3b4f05a4a34f
火种 ctf
本文来自互联网用户投稿,该文观点仅代表作者本人,不代表本站立场。本站仅提供信息存储空间服务,不拥有所有权,不承担相关法律责任。如若转载,请注明出处:http://www.mzph.cn/news/389404.shtml
如若内容造成侵权/违法违规/事实不符,请联系多彩编程网进行投诉反馈email:809451989@qq.com,一经查实,立即删除!