hadoop将消亡
Harvard Business Review marked the boom of Data Scientists in their famous 2012 article “Data Scientist: Sexiest Job”, followed by untenable demand in the past decade. [3]
《哈佛商业评论 》在2012年著名的文章“数据科学家:最性感的工作”中标志着数据科学家的蓬勃发展,随后十年来需求持续不振。 [3]
“..demand has raced ahead of supply. Indeed, the shortage of data scientists is becoming a serious constraint in some sectors.”
“ ..需求已经超越了供应。 实际上,在某些领域,数据科学家的短缺正在成为严重的制约因素。”
McKinsey & Co just published an article (Aug 2020) suggesting we rethink how many Data Scientists we really need in light of newer automation technologies (AutoML).[4]
麦肯锡公司 ( McKinsey&Co)刚刚发表了一篇文章(2020年8月),建议我们根据更新的自动化技术(AutoML)重新考虑真正需要多少数据科学家。[4]
“Over the long term, purely technical data scientists will still be needed, but simply far fewer than most currently predict.”
“从长远来看,仍将需要纯技术数据科学家,但远远少于目前大多数人的预测。”
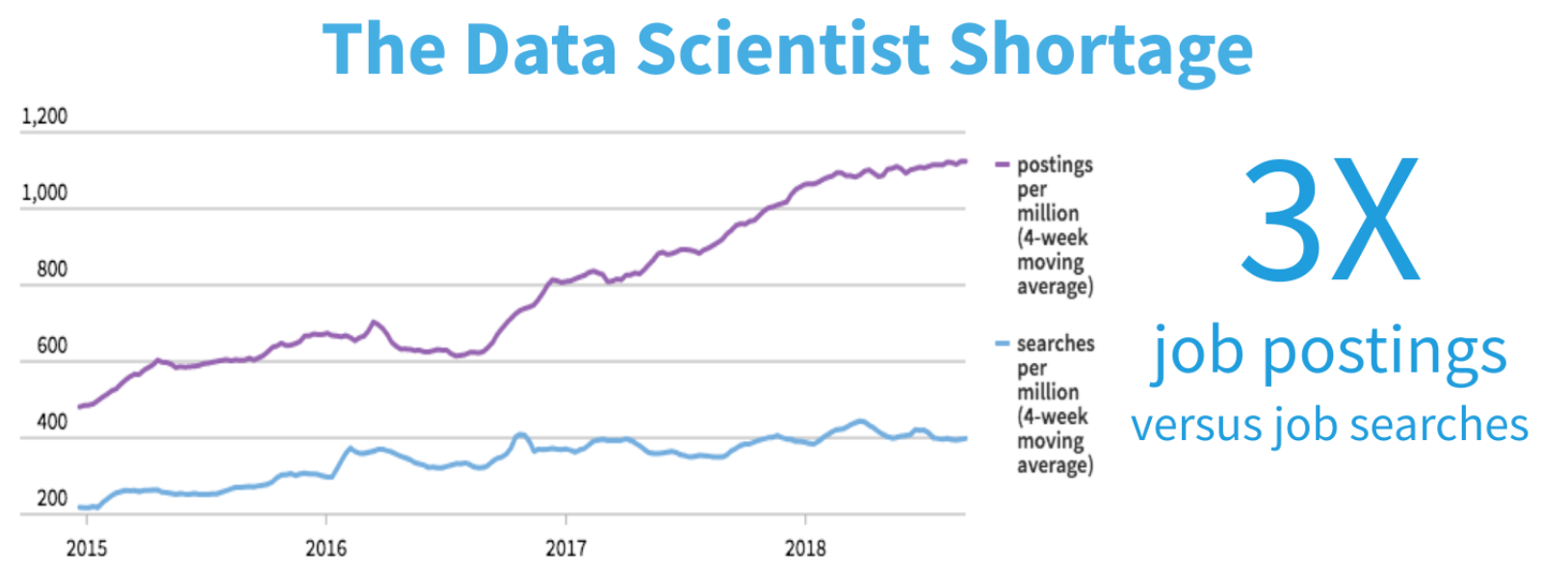
In every boom cycle you have a shortage of talent and an influx of imposters or just less qualified people (eg, dot.com y2k if you could spell Java you were a software engineer). As domains mature, tools and automation weed out those who aren’t really qualified or aren’t doing high value work. Data Science is no different.
在每个繁荣周期中,您都会缺乏人才,冒名顶替的人或缺乏资格的人会涌入(例如,如果您可以拼写Java,那么dot.com y2k就是您是一名软件工程师)。 随着领域的成熟,工具和自动化将淘汰那些没有真正资格或没有从事高价值工作的人。 数据科学也是如此。
肮脏的秘密 (The Dirty Secret)
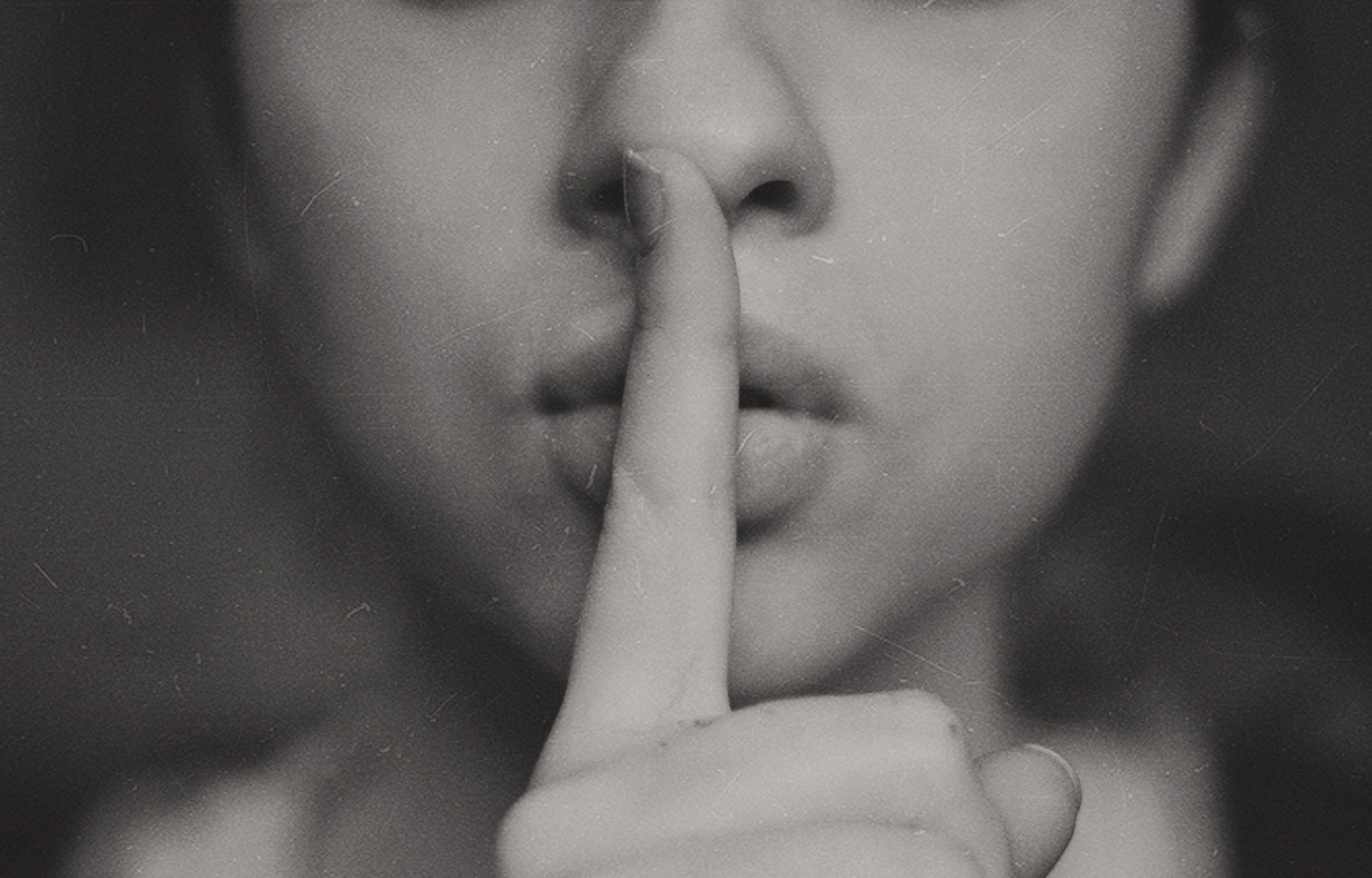
Data Science secrets are not as exciting as celebrity sex secrets unfortunately. Behind this “sexy” job is the large amount of grunt work required of Data Science projects— some of which include:
不幸的是,数据科学的秘密并不像名人性秘密那样令人兴奋。 这项“性感”工作的背后是数据科学项目所需的大量繁琐工作,其中包括:
- Data sourcing, validation and cleanup 数据来源,验证和清理
- Trying feature combinations and engineered features 尝试功能组合和工程功能
- Testing different models and model parameters 测试不同的模型和模型参数
Most agree that data-prep work is 80% of any ML/DS project [1] which has given rise to the Data Engineer specialty [2]. The remaining time is spent trying out features and testing models to squeeze out a few % pt’s of accuracy. It simply takes a lot of time — and while experience, intuition and luck allow a scientist to narrow down the scenarios, sometimes the best solution requires trying many extra atypical (almost random) scenarios. One solution is automation and utilizing brute-force compute cycles using the new breed of tools named AutoML.
大多数人都认为数据准备工作是任何ML / DS项目的80%[1],这引起了数据工程师的专长[2]。 剩下的时间用于测试功能和测试模型,以减少百分之几点的准确性。 它仅花费大量时间 ,而经验,直觉和运气使科学家可以缩小方案的范围, 有时最好的解决方案需要尝试许多额外的非典型(几乎随机)方案。 一种解决方案是自动化,并使用名为AutoML的新型工具利用蛮力计算周期。
AutoML —就像天网吗? (AutoML — Is it like Skynet ?)
Automated Machine Learning (AutoML) is software that automates of the repetitive work for you in an organized way. (Get a demo of H2O or DataRobot and see for yourself). Feed it the data, set the goal, and take a nap while it grinds thru iterations of features, models, and parameters. While it lacks domain expertise and precision, it makes up for it with brute force and superb bookkeeping/reporting (with some logic and heuristics of course) .
自动化机器学习(AutoML)是一种软件,可以有组织地自动执行重复性工作。 (获取H2O或DataRobot的演示,然后亲自看看)。 在通过要素,模型和参数的迭代进行研磨时,向其提供数据,设定目标并小睡一会。 尽管它缺乏领域专业知识和准确性,但它用蛮力和出色的簿记/报告(当然有一些逻辑和启发式)来弥补它。
When and if it replaces Scientists was polled on KDNuggets 5yrs ago — recent thinking is that time for some of us is very soon.
什么时候以及是否取代它,五年前就在KDNuggets上对《科学家》进行了调查-最近的想法是,对于我们中的某些人来说,这是很快的事情。
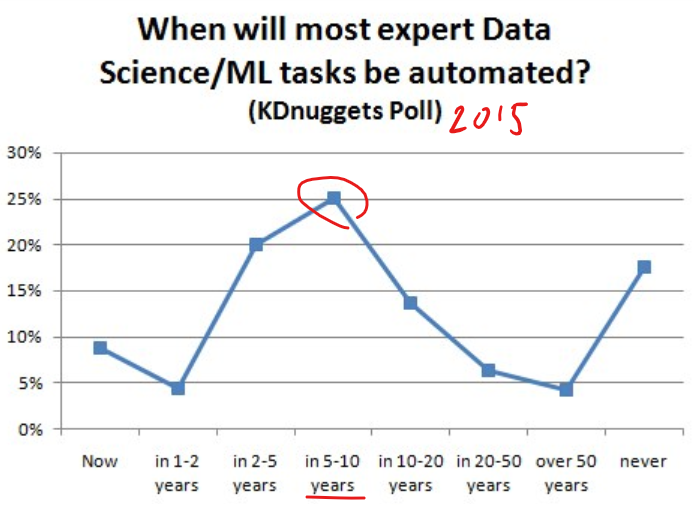
Not everyone agrees of course.
当然,并非所有人都同意。
Rachel Thomas of Fast.AI: “There are frequent media headlines about both the scarcity of machine learning talent and about the promises of companies claiming their products automate machine learning and eliminate the need for ML expertise altogether.” [7]
Fast.AI的Rachel Thomas: “关于机器学习人才的稀缺以及关于声称其产品实现机器学习自动化并完全消除ML专业知识需求的公司的承诺的媒体头条经常出现。” [7]
Dr. Thomas seems to feel AutoML is misconstrued and a fair amount of hype. She makes compelling points to help us understand the full ML cycle and what AutoML is and what it isn’t. It does not replace the work of experts but it does highly augments their work — not yet Skynet but give it some time...
托马斯博士似乎觉得AutoML被误解了,并且大肆宣传。 她让引人注目分,帮助我们理解全ML周期,什么AutoML 是什么,它不是 。 它不能代替专家的工作,但是可以极大地增强他们的工作-还不是天网,但要花点时间...
那我的工作要走了吗? (So Is My Job Going Away ?)
Google Brain co-founder Andrew Ng often states concern of imminent jobs losses caused by AI and ML [5]— however most analysis has been focused on operational and blue collar work. What about our cushy Data Science jobs? McKinsey describes the possible future awaiting us:
Google Brain的联合创始人安德鲁·伍(Andrew Ng)经常表示担心由AI和ML造成的即将失业的工作[5],但是大多数分析都集中在运营和蓝领工作上。 那我们轻松的数据科学工作呢? 麦肯锡描述了等待我们的未来:
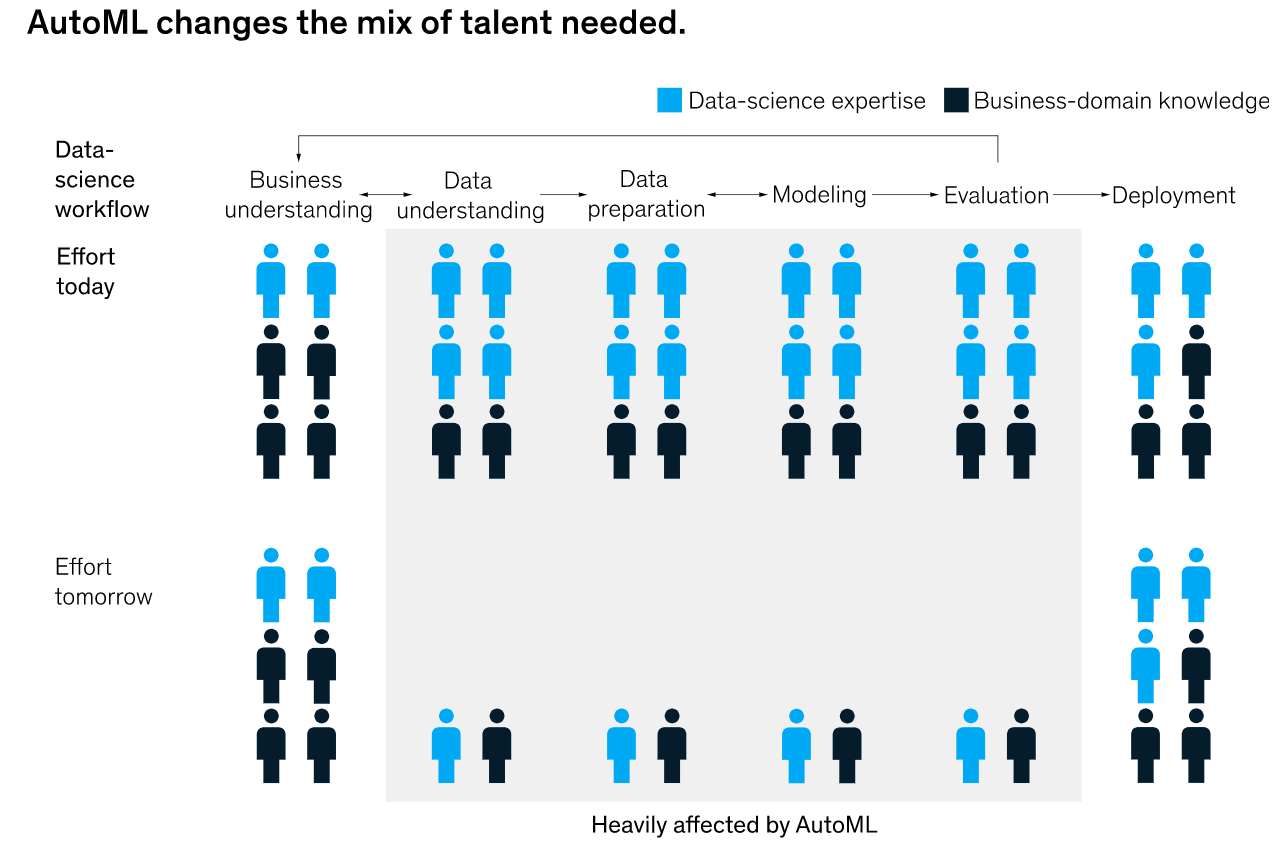
The bright side is that Data Scientists are not being fully replaced (graphic shows 29% … )— but let’s focus on McKinsey’s point to rethink the number and skillset of scientists needed. The number of scientists may drop per project as you add AutoML to your team (bots like TARS, R2D2 or HAL), but most research still suggest that aggregate demand for humans (scientists) will continue to increase for the next 5yrs+ at least.
好的一面是,数据科学家还没有被完全取代(图形显示为29%…),但是让我们关注麦肯锡的观点,重新考虑所需的科学家数量和技能。 当您向团队中添加AutoML(像TARS,R2D2或HAL之类的机器人)时,每个项目的科学家人数可能会减少,但是大多数研究仍然表明,至少在接下来的5年以上,对人类(科学家)的总需求将继续增长。
The bulk of online articles [9] make it clear Data Scientists are not dead after all. But most agree AutoML has come of age and is changing the makeup of projects and staffing even today. We all need to evolve, and as a Data Scientist you need to learn to leverage AutoML and related tech improvement or risk falling behind.
大量在线文章[9]清楚地表明,数据科学家毕竟还没有死。 但是,大多数人都同意AutoML已经成熟,并且即使在今天也正在改变项目和人员配置。 我们每个人都需要发展,作为数据科学家,您需要学习利用AutoML和相关的技术改进,否则风险就会落伍。
Automation is a good thing — we can focus on higher value work and eliminate boring and repetitive tasks (albeit the the boring, repetitive work paid pretty well …). I think we know it makes sense, why pay us when they can pay a cheaper robot? Thus next time you’re on a project, ask yourself am I doing expert Data Scientist work, an impostor, or are my days numbered ?
自动化是一件好事—我们可以专注于更高价值的工作,并消除无聊的重复性工作(尽管无聊的重复性工作的报酬很好……)。 我认为我们知道这是有道理的,为什么当他们可以付钱购买更便宜的机器人时,为什么要付钱给我们呢? 因此,下次您进行项目时,请问自己是我在做数据科学家方面的专家工作,是骗子,还是我的工作日已过?
“Will the real data scientist please stand up?”
“请真正的数据科学家站起来吗?”
The net takeaway — the future of DS/ML is bright but you need to embrace changes or you’ll go from Data Scientist to Dead Scientist. “Resistance is Futile” — but in this case assimilating will pay off.
最终的结果-DS / ML的未来是光明的,但是您需要拥抱变化,否则您将从数据科学家到死去的科学家。 “ 抵抗是徒劳的 ”-但在这种情况下,同化将奏效 。
参考和启示 (References and Inspirations)
[1] Ruiz, “The 80/20 data science dilemna” — https://www.infoworld.com/article/3228245/the-80-20-data-science-dilemma.html
[1] Ruiz,“ 80/20数据科学难题” — https://www.infoworld.com/article/3228245/the-80-20-data-science-dilemma.html
[2] Angelov, “Rise of the Data Engineer” — https://towardsdatasciencte.com/the-rise-of-the-data-strategist-2402abd62866?_branch_match_id=764068755630717009
[2] Angelov ,“数据工程师的崛起” — https://towardsdatasciencte.com/the-rise-of-the-data-strategist-2402abd62866?_branch_match_id=764068755630717009
[3] HBR’s Sexiest job article— https://hbr.org/2012/10/data-scientist-the-sexiest-job-of-the-21st-century
[3] HBR上最性感的工作文章-https : //hbr.org/2012/10/data-scientist-the-sexiest-job-of-the-the-21st世纪
[4] McKinsey on Rethinking AI Talent — https://www.mckinsey.com/business-functions/mckinsey-analytics/our-insights/rethinking-ai-talent-strategy-as-automated-machine-learning-comes-of-age
[4]麦肯锡(McKinsey)关于对AI人才的重新思考— https://www.mckinsey.com/business-functions/mckinsey-analytics/our-insights/rethinking-ai-talent-strategy-as-automated-machine-learning-comes-of -年龄
[5] Andrew Ng’s thoughts on Jobs and AI — https://www.youtube.com/watch?v=aU4RQD--Lec
[5]吴安德(Andrew Ng)关于乔布斯和人工智能的思想-https: //www.youtube.com/watch?v= aU4RQD-- Lec
[6] Looking back at the 2015 Poll on AutoML — https://www.kdnuggets.com/2020/03/poll-automl-replace-data-scientists-results.html
[6]综观2015轮询上AutoML背面- https://www.kdnuggets.com/2020/03/poll-automl-replace-data-scientists-results.html
[7] FastAI’s Rachel Thomas on the AutoML hype, what ML Scientists do and what AutoML can do — https://www.fast.ai/2018/07/12/auto-ml-1/
[7] FastAI的Rachel Thomas对AutoML的炒作,ML科学家做什么以及AutoML可以做什么— https://www.fast.ai/2018/07/12/auto-ml-1/
[8] Various references to Sci-Fi AI/robots — TARS from Interstellar, HAL from 2001, Borg assimilation from Star Trek, and of course Terminator’s Skynet.
[8]关于科幻AI /机器人的各种参考文献:《星际穿越》中的TARS,2001年以来的HAL,《星际迷航》中的博格同化,当然还有终结者的天网。
[9] Various articles on AutoML vs Humans KDNuggets, Wired, and Medium.
[9]有关AutoML与人的KDNuggets的各种文章, Wired和Medium 。
翻译自: https://towardsdatascience.com/data-scientists-adapt-or-die-2f009ebe4935
hadoop将消亡
本文来自互联网用户投稿,该文观点仅代表作者本人,不代表本站立场。本站仅提供信息存储空间服务,不拥有所有权,不承担相关法律责任。如若转载,请注明出处:http://www.mzph.cn/news/390600.shtml
如若内容造成侵权/违法违规/事实不符,请联系多彩编程网进行投诉反馈email:809451989@qq.com,一经查实,立即删除!