数据科学领域有哪些技术
Jeremie Harris: “In a way, it’s almost like a data scientist or a data analyst has to be like a private investigator more than just a technical person.”
杰里米·哈里斯(Jeremie Harris) :“ 从某种意义上说,这就像是数据科学家或数据分析师必须像私人调查员,而不仅仅是技术人员。 ”
Ian Scott: “Well, for sure. I have nothing against Kaggle, but when people say Kaggle, I have done this competition, I kind of roll my eyes, because they’re focusing on sort of 10% of the problem. (…) A lot of these projects go to die, because 90% of the other things weren’t done correctly, including stakeholder alignment.”
伊恩·斯科特:“ 嗯,肯定的。 我没有反对Kaggle的事情,但是当人们说Kaggle时,我参加了这场比赛,我有点kind目结舌,因为他们专注于解决10%的问题。 (… ) 这些项目很多都死了,因为90%的其他事情没有正确完成,包括利益相关者的协调。 ”
By reading this, you might think that Ian Scott, Chief Science Officer at Omnia AI, from Deloitte Canada, might think that doing Kaggle competitions is of useless. Think again. This is only an excerpt from an interview taken to Ian by Jeremie Harris, for the TDS podcast (minute 14), and it doesn’t illustrate the bigger picture. I will come back to it again further in the article, when discussing about the importance of context and what can happen when getting information out of its context.
通过阅读此书,您可能会认为来自Deloitte Canada的Omnia AI首席科学官Ian Scott可能会认为参加Kaggle比赛毫无用处。 再想一想 。 这只是杰里米·哈里斯(Jeremie Harris)对TDS播客(第14分钟)对伊恩(Ian)的采访中的摘录,而并未说明大局。 在讨论上下文的重要性以及从上下文中获取信息时可能发生的情况时,我将在本文中再次进行讨论。
Through this article I try to find an answer to the question “ How important domain knowledge really is for data scientist?”, which came to my mind while listening to this interview. And I do so by looking both at the insights provided especially through their discussion and jobs postings for Data Scientists available in the US, in fields such as Health Care, Financial Services, Retail, Real Estate, Government Administration, Market Research, Information Technology etc.
通过这篇文章,我试图找到一个问题“对于数据科学家来说领域知识到底有多重要?”的答案,这是我在听这次采访时想到的。 为此 ,我既要研究提供的见解, 也要针对他们在美国的数据科学家在医疗保健, 金融服务,零售,房地产,政府管理,市场研究,信息技术等
上下文在数据科学中的重要性 (The importance of context in Data Science)
In the interview, Ian talks about things that are in the data that can be analyzed and provide valuable information, and things that are not captured in the data which, as a result, will not be taken into consideration by the models built. How can data scientists deal with these cases? How can features be designed so that all relevant information and contextual data be included in the model?
在采访中,Ian谈论了数据中可以分析并提供有价值信息的事物,以及数据中未捕获的事物,因此构建的模型将不考虑这些事物 。 数据科学家如何处理这些情况? 如何设计要素,以便将所有相关信息和上下文数据包括在模型中?
Here is where domain knowledge comes in. And Ian mentions an approach they sometimes use for getting a better understanding of the problem being solved and of the desired outputs: and that is putting both the members of his team and the clients in the same room for several hours so that they can work together on understanding the real problem, understanding the shared output, and for getting the context collectively built.
这就是领域知识的来源。Ian提到了他们有时用来更好地了解要解决的问题和期望的输出的一种方法:这将他的团队成员和客户放在同一个房间里几个小时,以便他们可以共同理解真正的问题,理解共享的输出并共同构建上下文。
The key word here is context. Ian: “Data trumps context, trumps algorithm. Algorithm is the third thing I think about, not the first thing. What data do you have, give me the context of the data, the things that are in the data, that are about the data that I don’t see in the data.” (minute 7)
这里的关键词是情境 。 伊恩: 数据胜过上下文,胜过算法。 算法是我考虑的第三件事,而不是第一件事。 你有什么数据,给我 的数据 , 是在数据的东西,是有关数据,我没有数据看到 的范围内 。 ”(第7分钟)
One way to interpret this could be:
一种解释方式可能是:
Data is the starting point, as we initially check what data is available, what do we have already.
数据是起点,因为我们最初检查哪些数据可用,我们已经拥有什么。
Context. Some say that context is important as the data itself. For it we must go beyond what the data shows us and look even at things such as changes in the political landscapes. Or, as I heard in an example presented by Michael Bamberger, Independent Evaluation Consultant, in a presentation regarding “Evaluation in the Age of Big Data”, information collected from phone apps might be irrelevant when it comes to finding useful insights regarding women in certain areas, as their access to phones might be somewhat restricted by their partners or other family members. That is why, we need to have a good grasp of the contextual factors of the problems we wish to solve through Data Science, that might even influence the results. One other example, would be to look only at certain information from this interview with Ian Scott. If one would look only at the following statement, “Well, for sure. I have nothing against Kaggle, but when people say Kaggle, I have done this competition, I kind of roll my eyes.”, without listening to the entire interview, he/she would think that Ian Scott considers that doing Kaggle competitions is of no value. When instead, it seems to me that he only wants to underline that technical skills alone are not enough, but also the way they think and their ability to go for the simple answers — “we ask a lot of questions around people’s thought processes, as opposed to what they know”. This is what taking information out of the context means, which can be very harmful sometimes.
上下文。 有人说上下文对于数据本身很重要 。 为此,我们必须超越数据显示的范围,甚至要看诸如政治格局变化之类的事情。 或者,正如我在独立评估顾问Michael Bamberger在一个有关“大数据时代的评估”的演讲中所听到的一个例子中所听到的那样,从电话应用程序收集的信息对于寻找某些女性的有用见解可能无关紧要。地区,因为他们的电话或其他伴侣可能会限制他们使用手机。 因此,我们需要充分了解我们希望通过数据科学解决的问题的背景因素 ,这些因素甚至可能影响结果。 另一个例子是,只看这次采访中来自伊恩·斯科特(Ian Scott)的某些信息。 如果只看下面的陈述,“ 那当然。 我没有反对Kaggle的任何东西,但是当人们说Kaggle时,我参加了这场比赛,我有点eyes目结舌。” 他/她会认为Ian Scott认为参加Kaggle比赛没有任何价值。 相反,在我看来,他似乎只想强调技术技能还不够 ,还要强调他们的思维方式和寻求简单答案的能力-“ 我们围绕人们的思维过程提出了很多问题,反对他们知道的东西 。 这就是将信息移出上下文的含义,有时可能非常有害。
Algorithm: an algorithm that now makes great use of both the data available and its context.
算法 :一种算法,现在充分利用了可用数据及其上下文。
Interpretation and presentation of results. I am adding this fourth element as I believe that, here, context should take the center stage again. Interpretation and presentation of results should be done in accordance with stakeholders/ clients needs. And Data Scientists need to be able need to be able to communicate their obtained results in an adequate manner even to non-technical audiences.
结果的解释和表示 。 我添加第四个要素是因为我相信上下文在这里应该再次成为焦点。 结果的解释和陈述应根据利益相关者/客户的需求进行。 数据科学家需要能够以适当的方式将其获得的结果传达给非技术受众。
I remember how, in my first evaluation job, I had to learn fast a lot of domain specific knowledge. Even though I was part of a team with specialists from that field, I needed to know what they were talking about and be able to build an evaluation model that was appropriate to that specific context. So, beyond working on the task, I tried as much as possible to document myself on the respective topic.
我记得在我的第一份评估工作中,我必须如何快速学习很多特定领域的知识。 即使我是该领域专家组成的团队的一员,我也需要知道他们在说什么,并能够建立适合特定情况的评估模型。 因此,除了完成任务之外,我还尝试着尽可能多地记录下有关相应主题的内容。
Later on, in another case, when I had to add input for an evaluation of a program in healthcare, I felt that evaluators who had previous experience in that field were ahead of me. Yes, I knew methods of evaluation, but I had no knowledge about the history of the program and its specificity. However, the structure and the dynamic of the team was different, and the input expected from me was rather technical than specific to the subject matter. Within the team, we complemented each other and managed to provide the needed outputs.
后来,在另一种情况下,当我不得不增加对医疗保健计划的评估的投入时,我感到在该领域具有先前经验的评估师领先于我。 是的,我知道评估方法,但是我对该程序的历史及其特殊性一无所知。 但是,团队的结构和动态是不同的,我期望我提供的输入内容是技术性的,而不是针对主题的。 在团队中,我们相互补充并设法提供了所需的输出。
美国的Data Science工作一览 (A look into Data Science jobs in the US)
Now, let’s look at some Data Science jobs postings from different fields, as they appear on LinkedIn (on August, the 16th and 17th).
现在,让我们看看来自不同领域的一些Data Science职位发布,它们出现在LinkedIn(8月16日和17日)上。
And we will start with Hospital and Health Care, this being the field where I would most expect for domain knowledge to be required.
我们将从医院和医疗保健开始,这是我最期望需要领域知识的领域。
Hospital and Health Care
医院与卫生保健
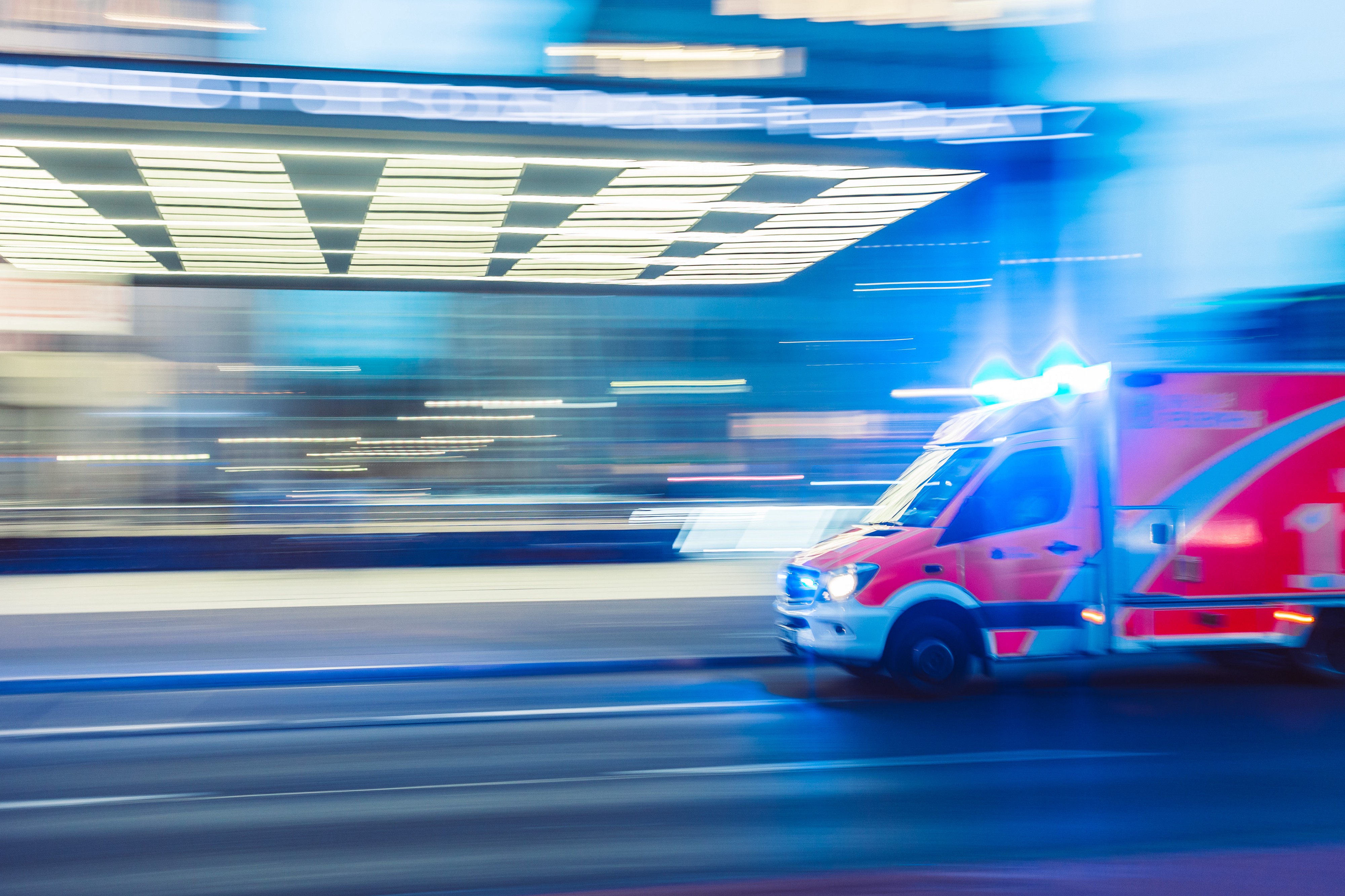
For this topic, I looked at job postings from all levels of experience: internships, entry-level jobs, Associate/ Mid-Senior level and Executive and Director levels.
在本主题中,我从各个级别的经验着眼于职位发布:实习,入门级职位,协理/中高级级别以及执行和董事级别。
At the highest Seniority levels (Executive and Director), there were only five all-time published job postings, from which three included previous experience and knowledge in Healthcare as a must, while one mentioned it as being an advantage for potential job candidates, and another didn’t mention it at all.
在最高资历级别 (执行官和总监)下,只有五次公开发布的职位空缺,其中三个必须包括以前在医疗保健方面的经验和知识,而其中一个则提到这对于潜在的求职者是有利的,并且另一个完全没有提及。
For the Associate/ Mid-Senior levels I looked at the postings from the last 24 hours, so as to get a feel on how much domain knowledge is required. From the 10 relevant job announcements, 6 asked explicitly for previous experience in the Health Care industry, while 2 considered it to be preferable or a plus, and the other two, which were in fact from Health, Wellness and Fitness, did not ask at all for domain related knowledge.
对于助理/中高级水平,我查看了过去24小时的帖子,以了解需要多少领域知识。 在10个相关的职位公告中,有6个明确要求提供医疗保健行业的从业经验,而2个则认为更可取或更好,而另外2个实际上来自健康,保健和健身行业,则没有要求全部用于领域相关知识。
When it comes to those asking for previous experience, the requirements sound as following: “Familiarity with a health care environment and EHR data”; “Familiarity with genomics and medical imaging data”; “A year or more in healthcare, preferably at a healthcare provider or health system”; “2+ years experience working with healthcare data (EHR/EMR healthcare information systems preferred)”, etc.
对于需要以前经验的人,要求听起来如下:“熟悉医疗环境和EHR数据”; “熟悉基因组学和医学影像数据”; “一年或一年以上的医疗保健经验,最好是在医疗保健提供者或卫生系统工作”; “具有2年以上处理医疗保健数据的经验(首选EHR / EMR医疗保健信息系统)”等。
Regarding entry-level jobs, the situation is similar to that of senior level jobs. There are postings that ask for previous experience and some that don’t. Example: “Must be knowledgeable of healthcare terminology, concepts and value chain, with intermediate knowledge of one or more key healthcare domains, e.g. client, customer, product, provider, and/or claim.”
关于入门级职位 ,情况与高级职位相似。 有些帖子要求提供以前的经验,有些则不需要。 示例:“必须具有医疗保健术语,概念和价值链的知识,并具有一个或多个关键医疗保健领域的中级知识,例如客户,客户,产品,提供者和/或索赔。”
When it comes to internships, things change, as there are no references to previous experience or educational background in the healthcare field. Besides the usual technical skills, some ask for a genuine interest in the industry or in their mission.
关于实习 ,情况发生了变化,因为没有提及医疗领域的以往经验或教育背景。 除了通常的技术技能,有些人还要求对行业或其使命有真正的兴趣。
Financial Services
金融服务
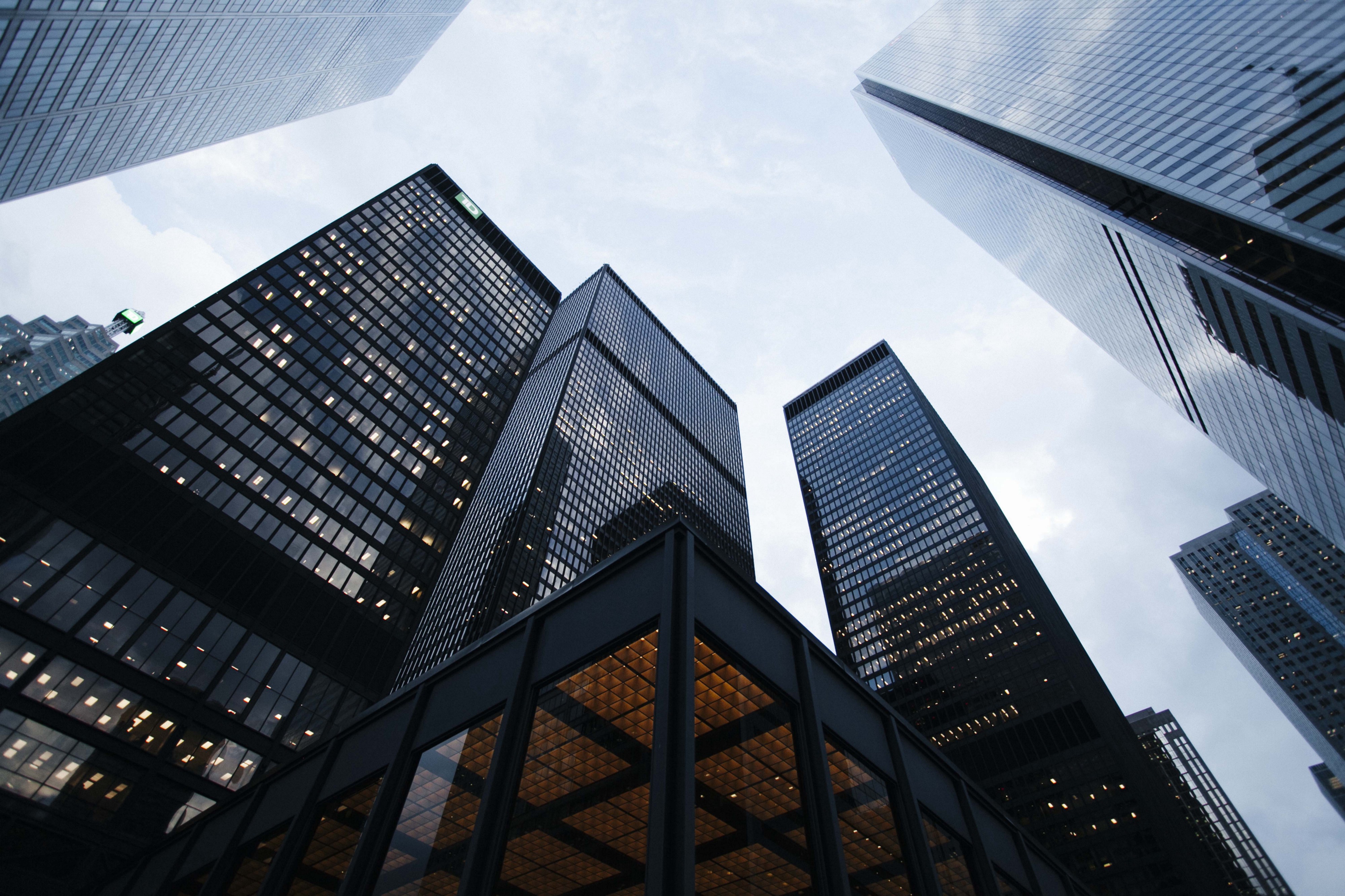
In this case, even though I have applied a filter for jobs in Financial Services, LinkedIn showed results from other sectors too, such as Retail, Staffing and Recruiting, or Information Technology. However, from the postings I have managed to look at, I have noticed that some jobs indeed require things such as: product design, experience in providing business insights, Passion for building data products, particularly in the real estate/financial space; Proven ability to apply findings to business problems to lift revenue and profits; be a Business Acumen — Leverages business judgment to shape strategy, based on understanding of operational, financial, and organizational requirements and capabilities, etc. In this case, as same as in Retail and Real Estate, some jobs postings ask for previous experience within a business setting, or mention it as being preferable, but there are also jobs that only ask for the necessary technical requirements.
在这种情况下,即使我为金融服务业的职位应用了过滤器,LinkedIn也显示了其他行业的结果,例如零售,人员配备和招聘或信息技术。 但是,从我设法查看的帖子中,我注意到某些工作确实需要执行以下任务:产品设计,提供业务见解的经验,对数据产品的热情,特别是在房地产/金融领域; 具有将发现应用于业务问题以提高收入和利润的能力; 成为业务敏锐度—基于对运营,财务,组织要求和能力等的理解,利用业务判断力制定战略。在这种情况下, 与零售和房地产行业一样 ,一些职位要求在职位范围内寻求以前的经验业务环境,或者说它是更可取的,但是有些工作只要求必要的技术要求。
Government Administration
政府行政
Here, there are jobs postings of both types: that don’t require subject matter expertise, and some that ask for it. In addition, they might require to be an U.S. citizen and to pass a background check, which is to be expected, as the employers are governmental agencies.
在这里,有两种类型的职位发布:不需要主题专业知识,而有些则需要。 此外,由于雇主是政府机构,他们可能需要成为美国公民并通过背景调查,这是可以预料的。
Sectors that are less likely to ask for specific domain knowledge, as they appear on LinkedIn: Staffing and Recruiting, Information Technology, Internet, Market Research, Marketing & Advertising, Media Production.
出现在LinkedIn上的, 不太可能要求特定领域知识的行业 :人员配备和招聘,信息技术,互联网,市场研究,市场营销与广告,媒体制作。
结论和要点 (Conclusions and key takeaways)
Domain knowledge definitely helps in better making sense of the data and of the problem’s context. In some cases, Data Scientists might need to also have strong subject matter expertise additional to the technical skills, but in other cases, depending also on the industry or on the way in which the organization for which he/ she works is structured, that might not be the same. In cases such as that from OMNIA AI (Deloitte Canada], even though Data Scientists are required to have certain soft skills in addition to technical ones, they work within larger teams, and are able to draw upon the expertise of their team members or even clients. In some cases, as same as in evaluation, teams can be of great use, and what Data Scientists don’t know can be covered with the help of subject matter experts.
领域知识无疑有助于更好地理解数据和问题背景。 在某些情况下,数据科学家可能还需要除技术技能外还具有较强的主题专业知识,但在其他情况下,还取决于行业或他/她工作的组织的组织方式,不一样。 在诸如OMNIA AI(加拿大德勤)的案例中,尽管数据科学家除了必须具备一定的软技能外,他们还需要在较大的团队中工作,并能够利用团队成员甚至是专家的专业知识。在某些情况下,就像在评估中一样,团队可能会发挥很大作用,而数据科学家不知道的内容可以在主题专家的帮助下解决。
When it comes to Data Science jobs postings some of them do ask for previous experience working in a specific field, but not all of them. There are sectors which require subject matter expertise more strongly (such as Health Care], and some who mostly require the technical skills and expertise (such as Market Research, Marketing & Advertising etc.]. Others, who are somehow in the middle, ask for previous experience in a business setting, or consider it to be preferable.
当涉及到数据科学工作职位时 ,其中一些确实要求在特定领域工作的以往经验,但并不是全部。 有些部门对主题的专业知识要求更高(例如“医疗保健”),而有些部门则最需要技术技能和专业知识(例如“市场研究”,“市场营销和广告”等)。以前在业务环境中的经验,或认为更可取。
Senior level jobs are more likely to ask for domain specific knowledge compared to internships and entry-level jobs, which is to be expected. However, this does not happen in all cases. And this might also be because the companies in certain sectors only now start adding Data Scientists to their teams.
与预期的实习和入门级工作相比,高级职位更可能要求特定领域的知识。 但是,并非在所有情况下都会发生这种情况。 这也可能是因为某些部门的公司现在才开始将数据科学家添加到其团队中。
When preparing to become a Data Scientist also try to identify the field in which you wish to work in, if you haven’t done so already, and look what employers look for in job candidates. Make a list of those requirements, see how you fit them and make a plan on honing the necessary skills. Additionally, try to do projects in the field you targeted and provide solutions that are already relevant to certain potential employers.
在准备成为一名数据科学家时,还应尝试确定您希望从事的领域(如果您尚未从事该领域的话),并寻找雇主在求职者中寻找的东西。 列出这些要求,查看您如何适应它们并制定磨练必要技能的计划。 此外,尝试在您所针对的领域中进行项目,并提供已经与某些潜在雇主相关的解决方案。
Sources:
资料来源:
“Data Science at Deloitte”, Interview taken to Ian Scott by Jeremie Harris for the TDS Podcast, https://towardsdatascience.com/data-science-at-deloitte-133457084a5
“ Deloitte的数据科学”,Jeremie Harris接受Ian Scott采访的TDS播客, https://towardsdatascience.com/data-science-at-deloitte-133457084a5
LinkedIn Job postings for Data Scientist in the US, https://www.linkedin.com/
美国数据科学家的领英职位发布, https://www.linkedin.com/
FLOWINGDATA, Article “ Why context is as important as the data itself”, interview with John Allen Paulos, math professor at Temple University, May 21, 2010, https://flowingdata.com/2010/05/21/why-context-is-as-important-as-the-data-itself/
FLOWINGDATA,文章“为什么背景与数据本身同样重要”,坦普尔大学数学教授John Allen Paulos访谈,2010年5月21日, https://flowingdata.com/2010/05/21/why-context-作为数据本身很重要/
IEG gLocal Event: Rewiring Evaluation Approaches, June 3, 2020, Presentation given by Michael Bamberger on “Evaluation in the Age of Big Data”, https://www.youtube.com/watch?v=bFRzcaDc0lU&list=PL23ljg-NcGGf_X-90O_WhTHSI8xvuU6Hn&index=37&t=3214s
IEG gLocal事件:重新评估方法,2020年6月3日,Michael Bamberger在“大数据时代的评估”中的演示, https://www.youtube.com/watch?v = bFRzcaDc0lU&list = PL23ljg-NcGGf_X-90O_WhTHSI8xvuU6Hn&index = 37&t = 3214s
翻译自: https://towardsdatascience.com/how-important-domain-knowledge-really-is-in-data-science-19d833d98698
数据科学领域有哪些技术
本文来自互联网用户投稿,该文观点仅代表作者本人,不代表本站立场。本站仅提供信息存储空间服务,不拥有所有权,不承担相关法律责任。如若转载,请注明出处:http://www.mzph.cn/news/390585.shtml
如若内容造成侵权/违法违规/事实不符,请联系多彩编程网进行投诉反馈email:809451989@qq.com,一经查实,立即删除!