熊猫数据集
Data aggregation is the process of gathering data and expressing it in a summary form. This typically corresponds to summary statistics for numerical and categorical variables in a data set. In this post we will discuss how to aggregate data using pandas and generate insightful summary statistics.
数据聚合是收集数据并以摘要形式表示的过程。 这通常对应于数据集中数字和分类变量的摘要统计量。 在这篇文章中,我们将讨论如何使用熊猫聚合数据并生成有洞察力的摘要统计信息。
Let’s get started!
让我们开始吧!
For our purposes, we will be working with The Wines Reviews data set, which can be found here.
为了我们的目的,我们将使用“葡萄酒评论”数据集,可在此处找到。
To start, let’s read our data into a Pandas data frame:
首先,让我们将数据读取到Pandas数据框中:
import pandas as pd
df = pd.read_csv("winemag-data-130k-v2.csv")
Next, let’s print the first five rows of data:
接下来,让我们打印数据的前五行:
print(df.head())
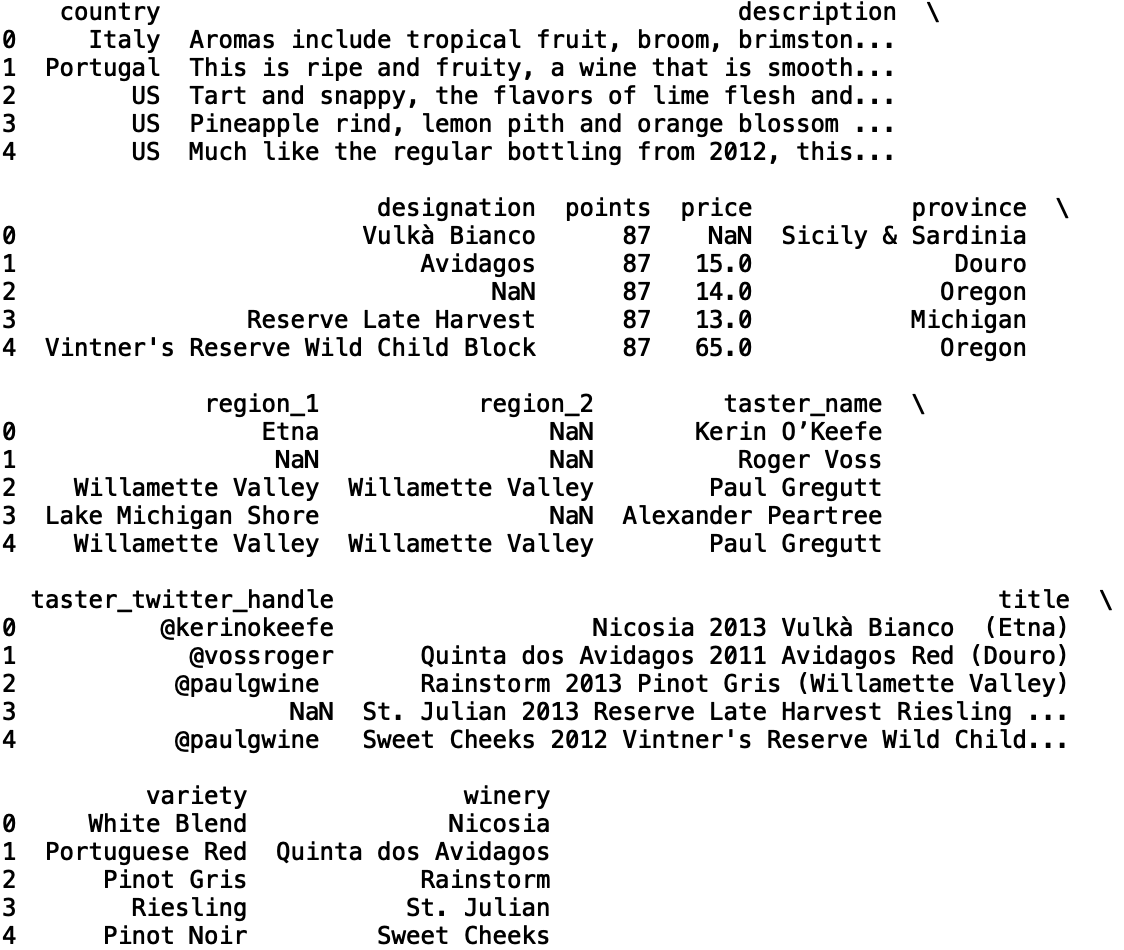
使用DESCRIBE()方法 (USING THE DESCRIBE() METHOD)
The ‘describe()’ method is a basic method that will allow us to pull summary statistics for columns in our data. Let’s use the ‘describe()’ method on the prices of wines:
'describe()'方法是一种基本方法,它使我们能够提取数据中列的摘要统计信息。 让我们对葡萄酒的价格使用'describe()'方法:
print(df['price'].describe())
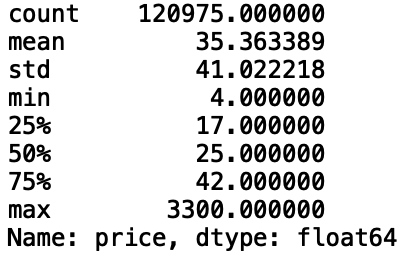
We see that the ‘count’, number of non-null values, of wine prices is 120,975. The mean price of wines is $35 with a standard deviation of $41. The minimum value of the price of wine is $4 and the maximum is $3300. The ‘describe()’ method also provides percentiles. Here, 25% of wines prices are below $17, 50% are below $25, and 75% are below $42.
我们看到葡萄酒价格的“计数”(非空值数量)为120,975。 葡萄酒的平ASP格为35美元,标准差为41美元。 葡萄酒价格的最小值为$ 4,最大值为$ 3300。 'describe()'方法还提供百分位数。 在这里,有25%的葡萄酒价格低于17美元,有50%的葡萄酒低于25美元,有75%的葡萄酒低于42美元。
Let’s look at the summary statistics using ‘describe()’ on the ‘points’ column:
让我们在“点”列上使用“ describe()”查看摘要统计信息:
print(df['points'].describe())

We see that the number of non-null values of points is 129,971, which happens to be the length of the data frame. The mean points is 88 with a standard deviation of 3. The minimum value of the points of wine is 80 and the maximum is 100. For the percentiles, 25% of wines points are below 86, 50% are below 88, and 75% are below 91.
我们看到点的非空值的数量是129,971,恰好是数据帧的长度。 平均值为88,标准偏差为3。葡萄酒的最小值为80,最大值为100。对于百分位数,25%的葡萄酒分数低于86,50%的分数低于88,而75%低于91。
使用GROUPBY()方法 (USING THE GROUPBY() METHOD)
You can also use the ‘groupby()’ to aggregate data. For example, if we wanted to look at the average price of wine for each variety of wine, we can do the following:
您也可以使用“ groupby()”来汇总数据。 例如,如果我们要查看每种葡萄酒的平ASP格,我们可以执行以下操作:
print(df['price'].groupby(df['variety']).mean().head())
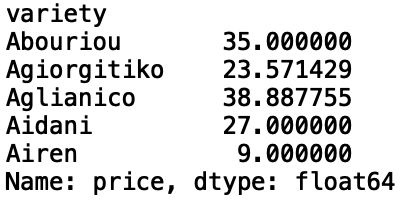
We see that the ‘Abouriou’ wine variety has a mean of $35, ‘Agiorgitiko’ has a mean of $23 and so forth. We can also display the sorted values:
我们看到“ Abouriou”葡萄酒的ASP为35美元,“ Agiorgitiko”葡萄酒的ASP为23美元,依此类推。 我们还可以显示排序后的值:
print(df['price'].groupby(df['variety']).mean().sort_values(ascending = False).head())
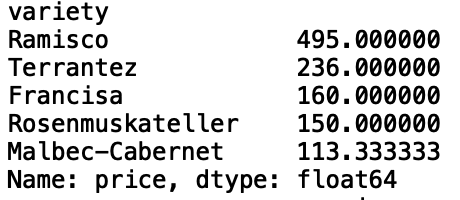
Let’s look at the sorted mean prices for each ‘province’:
让我们看一下每个“省”的排序平ASP格:
print(df['price'].groupby(df['province']).mean().sort_values(ascending = False).head())
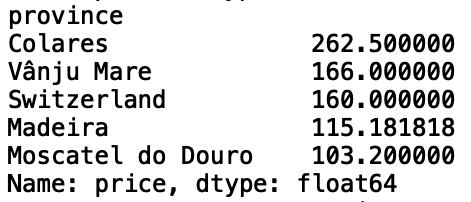
We can also look at more than one column. Let’s look at the mean prices and points across ‘provinces’:
我们还可以查看不止一列。 让我们看一下“省”的平ASP格和点数:
print(df[['price', 'points']].groupby(df.province).mean().head())
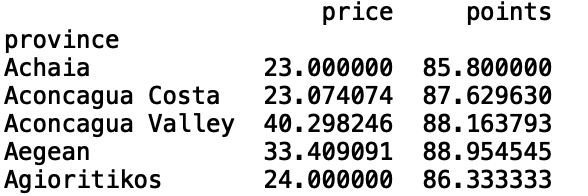
I’ll stop here but I encourage you to play around with the data and code yourself.
我将在这里停止,但我鼓励您尝试使用数据并自己编写代码。
结论 (CONCLUSION)
To summarize, in this post we discussed how to aggregate data using pandas. First, we went over how to use the ‘describe()’ method to generate summary statistics such as mean, standard deviation, minimum, maximum and percentiles for data columns. We then went over how to use the ‘groupby()’ method to generate statistics for specific categorical variables, such as the mean price in each province and the mean price for each variety. I hope you found this post useful/interesting. The code from this post is available on GitHub. Thank you for reading!
总而言之,在本文中,我们讨论了如何使用熊猫聚合数据。 首先,我们讨论了如何使用“ describe()”方法生成汇总统计信息,例如数据列的均值,标准差,最小值,最大值和百分位数。 然后,我们讨论了如何使用“ groupby()”方法来生成特定类别变量的统计信息,例如每个省的平ASP格和每个品种的平ASP格。 我希望您发现这篇文章有用/有趣。 这篇文章中的代码可在GitHub上找到 。 感谢您的阅读!
翻译自: https://towardsdatascience.com/mastering-data-aggregation-with-pandas-36d485fb613c
熊猫数据集
本文来自互联网用户投稿,该文观点仅代表作者本人,不代表本站立场。本站仅提供信息存储空间服务,不拥有所有权,不承担相关法律责任。如若转载,请注明出处:http://www.mzph.cn/news/389303.shtml
如若内容造成侵权/违法违规/事实不符,请联系多彩编程网进行投诉反馈email:809451989@qq.com,一经查实,立即删除!