数据中台是下一代大数据
重点 (Top highlight)
Data science has been an eye-catching field for many years now to young individuals having formal education with a bachelors, masters or Ph.D. in computer science, statistics, business analytics, engineering management, physics, maths, or obviously data science. However, there are a lot of myths that people presume about data science. It’s no more just machine learning and statistics. Over the years, I have spoken to a lot of data science aspirants about breaking into this field. Why is there all the hype about data science? Is it still statistics and machine learning that can help you break into this field? Is it still going to be the future? Even I was in the same boat as you all, but I am now experiencing how the demand has molded currently for the next generation of data scientists breaking into this field. I am not going to teach you how to get into data science as many people on the internet are already doing it.
多年来,数据科学一直是受过本科学历,硕士或博士学位的年轻人的引人注目的领域。 计算机科学,统计,业务分析,工程管理,物理,数学或显然是数据科学。 但是,人们对数据科学有很多神话。 不仅仅是机器学习和统计。 多年来,我已经与许多数据科学领域的有志之士谈论了进入该领域的问题。 为什么会有关于数据科学的所有炒作? 仍然是统计数据和机器学习可以帮助您进入这一领域吗? 仍然是未来吗? 甚至我和你们都在同一条船上,但是我现在正在经历目前对进入该领域的下一代数据科学家的需求如何形成。 我不会教你如何进入数据科学领域,因为互联网上已经有很多人这样做了。
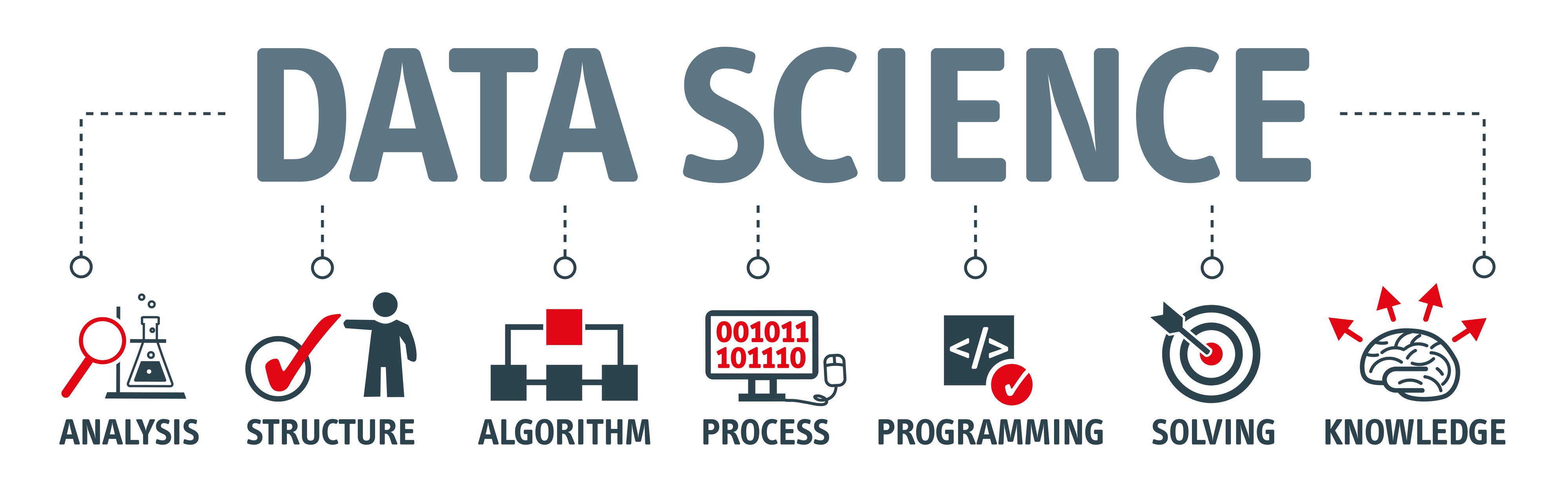
为什么会有关于数据科学的所有炒作? (Why is there all the hype about Data Science?)
Everyone around the corner wants to get into data science. A few years ago, there was a demand-supply problem in the field: supply of data scientists was less, and demand was more after Dr. DJ Patil and Jeff Hammerbacher tossed the term Data Science. But now, in 2020, the situation has turned around. The inflow of formally/MOOCs educated data science enthusiasts has increased, and the demand has grown too, but not to that extent. The term has evolved broader and broader to incorporate most of the supporting functionalities that one needs to do data science. I would like to quote one of my favorite quotes from KD nuggets:
每个角落的人都希望进入数据科学领域。 几年前,该领域存在供需问题:数据科学家的供应量减少了,而DJ Patil博士和Jeff Hammerbacher抛弃了数据科学一词后,需求增加了。 但是现在,到2020年,情况有所好转。 受正规/ MOOC受过教育的数据科学爱好者的流入量有所增加,需求也有所增加,但并未达到这种程度。 该术语已发展得越来越广泛,以包含人们进行数据科学所需的大多数支持功能。 我想引述我最喜欢的KD矿块之一:
“Data Science is like Teenage Sex: Everyone talks about it, No body really knows how to do it, everyone thinks everyone else is doing it, so everyone claims they are doing it.”
“数据科学就像十几岁的性行为:每个人都在谈论它,没有人真正知道如何做,每个人都认为其他人正在做,所以每个人都声称自己正在做。”
Jokes apart, These are some of the things which I feel why data science has taken over all the hype:
开个玩笑,这些是我认为数据科学接管所有炒作的原因:
- The mystery behind the title data scientist 标题数据科学家背后的谜团
- High job satisfaction 高工作满意度
- Huge business impact 巨大的业务影响
- Many job sites rating it as the hottest Job (last 3 years as hottest Job in the US by Glassdoor) 许多工作网站将其评为最热门的工作(最近3年被Glassdoor评为美国最热门的工作)
- Cutting edge developments 前沿发展
- Increasing influx of data generation 越来越多的数据生成
- Thanks to many great/not so great schools and boot camps providing degrees in data science 感谢许多提供数据科学学位的优秀/不太优秀的学校和新兵训练营
- data is beautiful! (Not literally :p) 数据真漂亮! (从字面上不是:p)
自称数据科学家的人? (People who call themselves Data Scientists?)
Someone is going to say it, so let me spill some truth about the current industry situation. Due to increase in demand and prestige of the shiny Data Scientist title, Many companies have started switching data scientist titles with product analyst, business intelligence analyst, business analyst, supply chain analyst, data analyst, and statistician because people were leaving their jobs to get the data scientist titles at companies which were giving them for doing the same job. It’s all the matter of respect that many roles get due to this minor change in the words. So, companies have started twisting titles, in the same way, to make it more shiny and desirable like data scientist-analytics, product data scientist, data scientist-growth, data scientist-supply chain, data scientist-visualization, or data scientist - what not?.
有人会这么说,所以让我就当前的行业状况讲一些真相。 由于需求的增加和闪亮的数据科学家头衔的声望,许多公司已开始与产品分析师,商业情报分析师,业务分析师,供应链分析师,数据分析师和统计师交换数据科学家头衔,因为人们离开工作岗位来获得数据科学家在那些给他们做相同工作的公司的头衔。 尊重的问题是,由于单词的微小变化,许多角色都得到了尊重。 因此,公司已经开始以相同的方式扭曲标题,以使其更闪亮和更令人期望,例如数据科学家分析,产品数据科学家,数据科学家增长,数据科学家供应链,数据科学家可视化或数据科学家-什么不?
Most people pursuing education/online training have a misconception that all data scientists build fancy machine learning models, but that’s not always true. At least that was the case with me when I started pursuing my masters in applied data science, I assumed that most data scientists do machine learning but when I entered the internship and job market in the US, that’s when I came to know about the real truth. The force driving people towards pursuing data science is due to the hype around artificial intelligence and its business impact.
大多数追求教育/在线培训的人都有一个误解,认为所有数据科学家都建立了精美的机器学习模型,但这并不总是正确的。 至少当我开始攻读应用数据科学的硕士时,我就是这样,我以为大多数数据科学家都是机器学习的,但是当我进入美国的实习和工作市场时,那才是我真正的知识所在。真相。 推动人们走向数据科学的力量归因于对人工智能及其业务影响的炒作。
下一代数据科学家-机器学习 (Next Generation of Data Scientists — Machine Learning)
For people who want to do applied machine learning as a Data Scientist-ML(That’s how I am going to name the title because it’s not data scientist-analytics :p)in 2020 without a Ph.D., there’s a lot more to it now instead of just knowing to apply machine learning to datasets which almost anyone today can do. There are a few other crucial things which I figured out from my experience, which can help you nail the data scientist role hunting for the interview process or even to get shortlisted:
对于想要以数据科学家-ML的身份进行应用机器学习的人(这就是我要命名的标题,因为它不是数据科学家-分析:p)在没有博士学位的情况下,还有更多的东西现在,不仅仅是知道将机器学习应用于如今几乎任何人都可以做的数据集。 我从经验中发现了其他一些关键问题,可以帮助您确定在采访过程中甚至入围的数据科学家的角色:
Distributed Data Processing/Machine Learning: Getting hold of hands-on experience with technologies such as Apache Spark, Apache Hadoop, Dask, etc. can help you prove that you can create Data/ML pipelines at scale. Having experience with anyone of them should be good to go, but I would recommend Apache Spark(either in Python or Scala) the go-to.
分布式数据处理/机器学习 :掌握诸如Apache Spark ,Apache Hadoop,Dask等技术的动手经验,可以帮助您证明可以大规模创建数据/ ML管道。 与任何人都有经验应该是不错的选择,但是我还是建议使用Apache Spark(使用Python或Scala)。
Production ML/Data Pipelines: If you can get hands-on experience with Apache Airflow, a standard open-source job orchestration tool for creating data and machine learning pipelines. This is currently used in the industry so, it’s recommended to learn and get some projects around it.
生产ML /数据管道 :如果您可以亲身体验Apache Airflow ,这是一种用于创建数据和机器学习管道的标准开源作业编排工具。 目前,该行业已在使用它,因此建议学习并围绕它进行一些项目。
DevOps/Cloud: DevOps is very much neglected by most of the data science aspirants. If you don’t have an infrastructure, how would you build ML pipelines? It’s not as easy as we do in the coursework to build notebooks or code that run on your local machine. The code that you write should be scalable across infrastructure that you or other folks might create on your team. Many companies might not have the ML infrastructure already laid out and might be looking for someone to start with. Getting familiar with Docker, Kubernetes, and building ML applications with frameworks like Flask should be your standard practice even during your coursework. I love Docker as it’s scalable and you can build infrastructure images and replicate the same things on servers/cloud on Kubernetes clusters.
DevOps / Cloud :大多数数据科学的追求者都非常忽略DevOps。 如果您没有基础架构,您将如何构建ML管道? 要构建在本地计算机上运行的笔记本或代码,并不像我们在课程中所做的那样容易。 您编写的代码应可跨您或其他人可能在团队中创建的基础结构进行扩展。 许多公司可能尚未布局ML基础架构,并且可能正在寻找入门人员。 即使在课程学习中 ,熟悉Docker , Kubernetes和使用Flask之类的框架构建ML应用程序也应该是您的标准做法。 我喜欢Docker,因为它具有可扩展性,您可以构建基础架构映像,并在Kubernetes集群上的服务器/云上复制相同的内容。
Databases: Knowing databases and query languages is a must. SQL is very much neglected, but It’s still the industry standard, be it on any cloud platform or databases. Start practicing complex SQLs on leetcode, which is gonna help you with some part of coding interviews in DS profiles as you will be responsible for bringing in data from warehouses with on-the-go preprocessing, which will ease up your job on preprocessing before running ML models. Most of the feature engineering can be done on-the-go while getting the data to your models with SQL, which is an aspect many people neglect.
数据库 :必须了解数据库和查询语言。 尽管SQL非常被忽略,但是无论在任何云平台或数据库上,它仍然是行业标准。 开始在leetcode上练习复杂SQL,这将帮助您在DS概要文件中进行部分编码采访,因为您将负责通过正在进行的预处理从仓库中导入数据,这将简化您在运行前进行预处理的工作ML模型。 大多数功能工程可以随时随地完成,而使用SQL将数据传输到模型中时,这是很多人忽略的一个方面。
Programming Languages: The recommended programming languages for data science are Python, R, Scala, and Java. Knowing anyone of them is fine and can do the trick. For ML kind of roles, there’s going to be live coding rounds in the interview process so you need to practice wherever you are comfortable — Leetcode, Hackerrank, or anything you prefer.
编程语言 :推荐用于数据科学的编程语言是Python,R,Scala和Java。 了解他们中的任何一个都可以,并且可以解决问题。 对于ML角色,在面试过程中将进行现场编码回合,因此您需要在任何舒适的地方练习-Leetcode,Hackerrank或您喜欢的任何东西。
So, This is the time when knowing only Machine Learning or Statistics is not gonna get you into data science to do ML unless you are lucky, have some great connections in the industry(you should obviously do networking which is very important!) or have an exceptional research record already in your name. Business applications and domain knowledge tends to come with experience and can’t be learned beforehand other than doing internships in relevant industries.
因此,这是时候仅了解机器学习或统计学并不能让您进入数据科学领域去学习ML的时候,除非您很幸运,在行业中有一些重要的联系(显然应该进行非常重要的联网!)或拥有以您的名字命名的卓越研究记录。 业务应用程序和领域知识往往带有经验,除了在相关行业进行实习以外,是无法事先学习的。
我怎么了 (What’s up with me?)
Two months back, I joined the media power-house ViacomCBS as a Data Scientist straight out of grad school without any prior full-time industry experience except research assistantships and internships. My responsibilities here include building ML Products from ideation — development — production where I use most of the things listed above. I hope this will be helpful for all the aspiring Data Scientists and Machine Learning Engineers who are trying to break into this field.
两个月前,我以数据科学家的身份加入了媒体巨头维亚康姆广播公司( ViacomCBS) ,直接从研究生院毕业,除了研究助理和实习生以外,没有任何以前的全职行业经验。 我在这里的职责包括从构想(开发)到生产ML产品,在这些产品中,我使用了上面列出的大多数内容。 我希望这将对所有有志于进军这一领域的有抱负的数据科学家和机器学习工程师有所帮助。
Shoot your questions on [myLastName][myFirstName] at gmail dot com or let’s connect on LinkedIn.
在gmail点com上的[myLastName] [myFirstName]上提问,或者在LinkedIn上连接。
翻译自: https://towardsdatascience.com/full-stack-data-science-the-next-gen-of-data-scientists-cohort-82842399646e
数据中台是下一代大数据
本文来自互联网用户投稿,该文观点仅代表作者本人,不代表本站立场。本站仅提供信息存储空间服务,不拥有所有权,不承担相关法律责任。如若转载,请注明出处:http://www.mzph.cn/news/389293.shtml
如若内容造成侵权/违法违规/事实不符,请联系多彩编程网进行投诉反馈email:809451989@qq.com,一经查实,立即删除!