北方工业大学gpa计算
This is my firts publication here and i will start simple.
这是我的第一篇出版物,这里我将简单介绍 。
I want to make an exploratory data analysis of UFRN’s warehouse and answer some questions about the data using Python and Power BI.
我想对UFRN的仓库进行探索性数据分析,并使用Python和Power BI回答有关数据的一些问题。
I have downloaded the dataset from “dados.gov.br”, wich is an open source website of public data from Brazil. You can have the used dataset here 👈
我已经从“ dados.gov.br ”下载了数据集,该数据集是来自巴西的公共数据的开源网站。 您可以在这里拥有使用的数据集👈
QUESTIONS TO BE ANSWERED:
需要回答的问题:
What are the items with the greatest quantity in stock;
库存量最大的物品是什么?
What are the items with the greatest value in stock;
库存中价值最大的物品是什么?
Wich warehouse has the greatest quantity of items in stock and wich has the lower quantity;
Wich 仓库的库存物品最多,而数量 较少的 仓库 ;
Wich warehouse has the greatest value in stock;
Wich 仓库的库存价值 最大 。
What are the most expensive items.
什么是最昂贵的物品。
So, let’s start importing pandas in the Jupyter Notebook and opening the csv file:
因此,让我们开始在Jupyter Notebook中导入熊猫并打开csv文件:
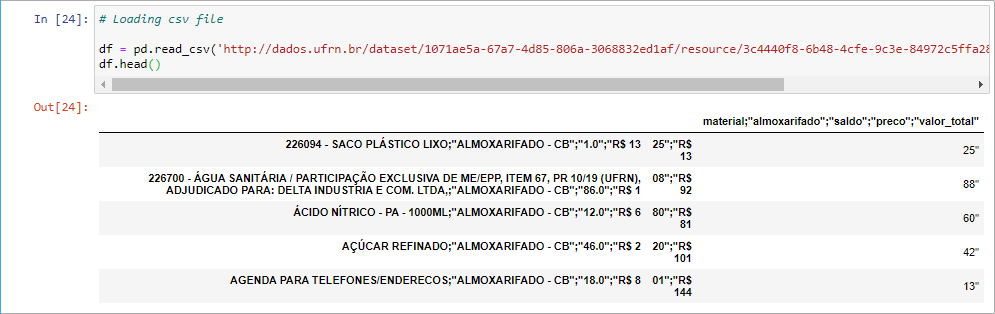
Here ☝ we have our first problem:
☝我们有第一个问题:
File is strange. Seems that all informations are in the same column…
文件很奇怪 。 似乎所有信息都在同一列中…
This factor makes it impossible to analyse the dataframe by column as we can see below:
这个因素使我们无法按列分析数据帧,如下所示:

The problem in this case is the separator. Pandas uses the comma as default delimiter (or separator), and in this file, the delimiter is semicolon.
在这种情况下,问题是分隔符。 Pandas使用逗号作为默认定界符(或分隔符),在此文件中,定界符为分号。
So, we need to inform the delimiter right after the path file:
因此,我们需要在路径文件之后立即通知定界符:
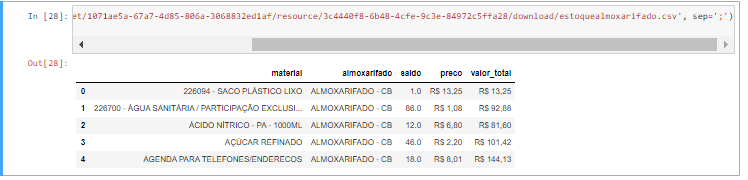
Now, here we go! ✌
现在,我们开始! ✌
Let’s check some informations about the dataset, like columns, data types, lenght, and missing values.
让我们检查有关数据集的一些信息,例如列 , 数据类型 , 长度和缺失 值 。
Note that we have 5 columns:
请注意,我们有5列:


material (Item): object;almoxarifado (Warehouse): object;saldo (balance): float;preco (price): object;valor_total (Amount): object;
材料 (项目):对象; almoxarifado (仓库):对象; saldo (平衡):浮动; preco (价格):对象; valor_total (金额):对象;
If we had to make some operations between columns, we should probably change some object types to integer or float values, but in this case we won’t need.
如果必须在列之间进行某些操作,则可能应将某些对象类型更改为整数或浮点值,但在这种情况下,我们将不需要。
Now we can analyse data through columns
现在我们可以通过列分析数据
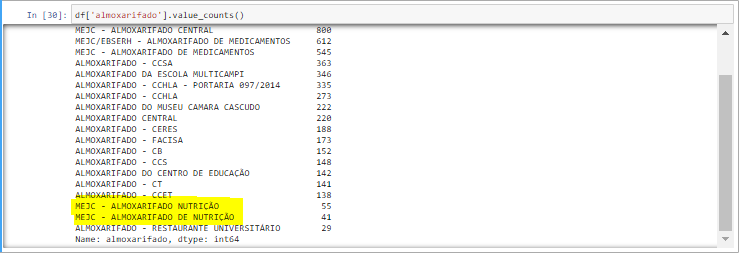
☝ Above we have the second issue:
☝以上是第二个问题:
There are 2 different names for the same warehouse: “MEJC — ALMOXARIFADO NUTRIÇÃO” and “MEJC — ALMOXARIFADO DE NUTRIÇÃO”.
有对同一仓库2个不同的名字:“MEJC - ALMOXARIFADONUTRIÇÃO”和“MEJC - ALMOXARIFADO DENUTRIÇÃO”。
It’s a typo, so let’s make them one.
这是一个错字,因此让我们将它们设为一个。

Done!
做完了!
A very quick and easy way to have a lot of information in one code line is using Pandas Profilling.
使用Pandas Profilling是在一个代码行中具有很多信息的非常快速,简便的方法。
Analysing the earlier infos and Pandas Profilling below, we have: 9087 rows and 4536 distinct items. 49.9% unique items and 0 missing cells.
分析下面的早期信息和Pandas Profilling,我们有9087行和4536个不同的项目。 49.9%的独特物品和0个丢失的单元格。
It means that dataframe doesn’t need much effort in cleaning and treatment.
这意味着数据帧在清理和处理方面不需要太多的工作。

This dataset is small and brings us just a few columns. Like i said, first publication, simple things (sorry 😬)
这个数据集很小,只给我们带来了几列。 就像我说的那样,第一次出版,简单的事情(对不起😬)
Excepting “saldo”, “preco” and “valor_total”, the columns are categorical data. So now, we can go to Power BI to make some visualizations and answer some questions.
除“ saldo”,“ preco”和“ valor_total”外,这些列均为分类数据。 现在,我们可以去Power BI进行可视化并回答一些问题。
But first, we need to export the dataset we edited in Jupyter Notebook:
但是首先,我们需要导出在Jupyter Notebook中编辑的数据集:

With the file saved in the same folder of the actual project, we just need to open it in Power BI
将文件保存在实际项目的同一文件夹中,我们只需要在Power BI中打开它
The data is configured by default as a “Western European” source. Because of this, some words in brazilian portuguese are misspelled
默认情况下,数据配置为“西欧”来源。 因此,巴西葡萄牙语中的某些单词 拼写错误
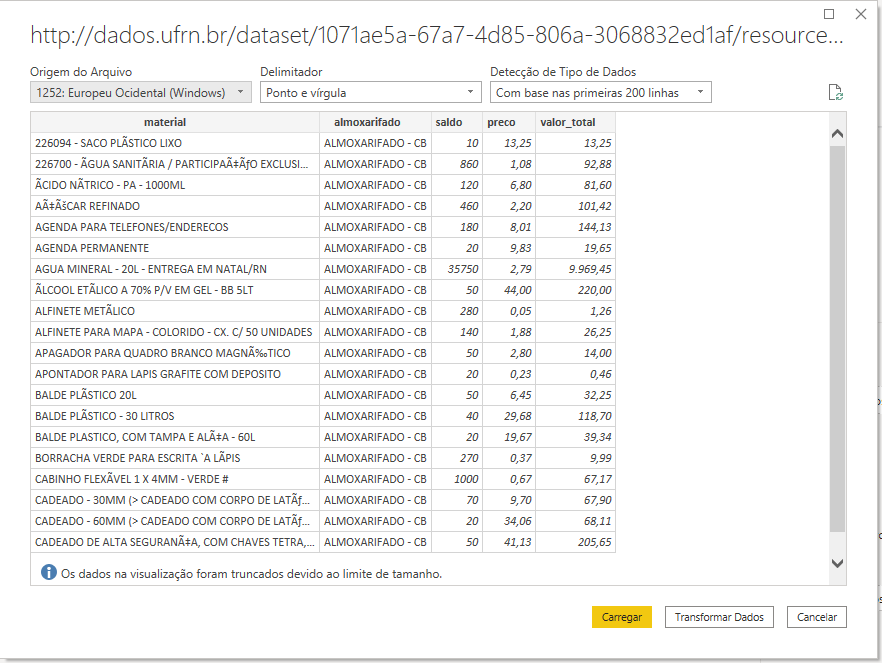
We solve this issue choosing “Unicode — UTF8” as the file source
我们选择“ Unicode — UTF8”作为文件源来解决此问题

At the Power Query editor, we can see that the columns are already in the right format (Text, Text, Role Number, Currency, Currency). So it doesn’t need any transformation, just close and apply.
在Power Query编辑器中,我们可以看到列的格式已经正确(文本,文本,角色编号,货币,货币)。 因此,它不需要任何转换,只需关闭并应用即可。

LET’S GO TO THE QUESTIONS!
让我们去解决问题!
What are the items with the greatest quantity in stock:
什么是物品 库存数量最多的库存:
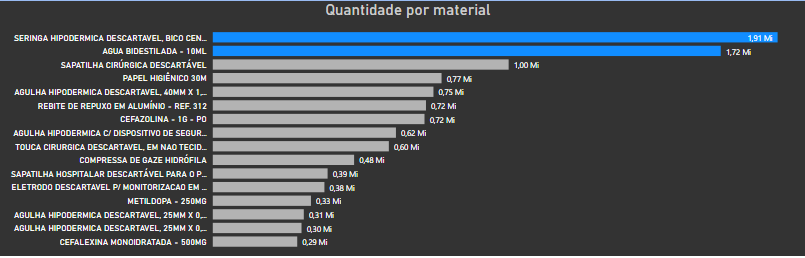
Almost 2 millions syringes and double-distilled water.
近200万支注射器和双蒸馏水 。
2. What are the items with the greatest value in stock:
2.什么是物品 库存最大的价值 :

WOW! Wait..close to 1 million $ in coffee??? Seems that teachers are driven by coffee! 😂
哇! 等待..接近一百万美元的咖啡 ??? 看来老师是被咖啡驱动的! 😂
Note that the value of these 2 items is very different from the others (could they be outliers?)
请注意,这两项的价值与其他项有很大不同 (它们可能是离群值吗?)
3. Wich warehouse has the greatest quantity of items in stock and wich has the lower quantity:
3. Wich 仓库中的物品数量最多 ,而其中的物品数量 较少:
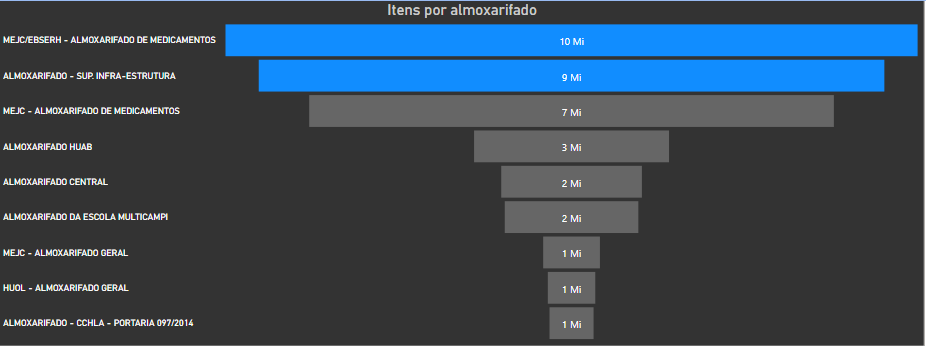
Despite these values are being rounded, the medicine warehouse has the largest number of items, followed by the infra-structure warehouse.
尽管对这些值进行了四舍五入,但药品仓库的物品数量最多 ,其次是基础结构仓库。
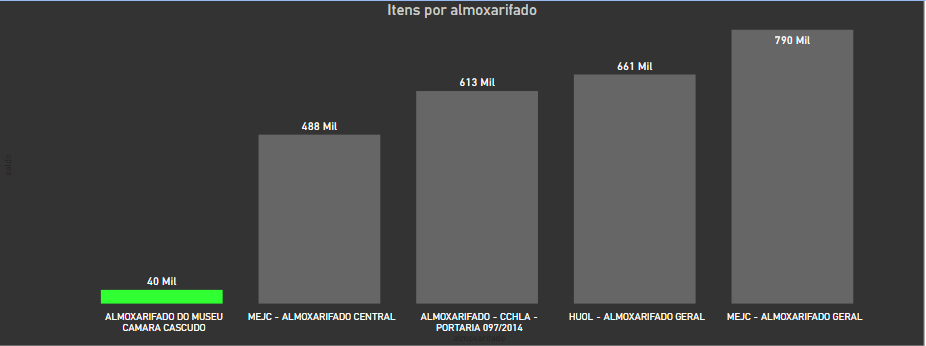
And the museum warehouse has de lower number of items.
博物馆仓库的物品数量较少 。
4. Wich warehouse has the greatest value in stock:
4. Wich 仓库的库存最大 价值 :
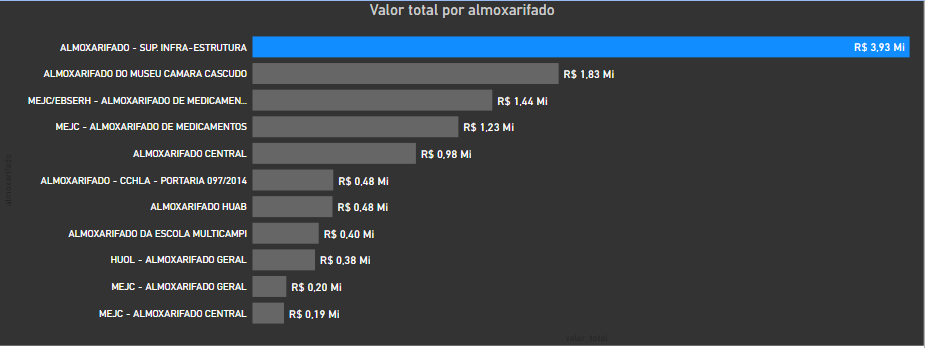
We saw earlier that the Medicine Warehouse has the largest number of items, slightly larger than that of Infrastructure. But the stock value of the Infra Warehouse is twice that of Medicine.
前面我们看到, 药品仓库的物品数量最多 ,比基础设施的数量略大。 但是Infra仓库的库存价值 是医学的两倍 。
5. What are the most expensive items.
5.什么是最昂贵的物品。

Ok, so a 250g package of coffee costs 30.4 thousands?? Sounds weird 🤔
好的,一包250克咖啡的价格为30,400欧元? 听起来很奇怪🤔
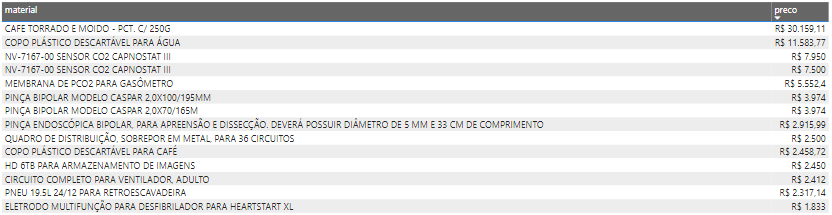
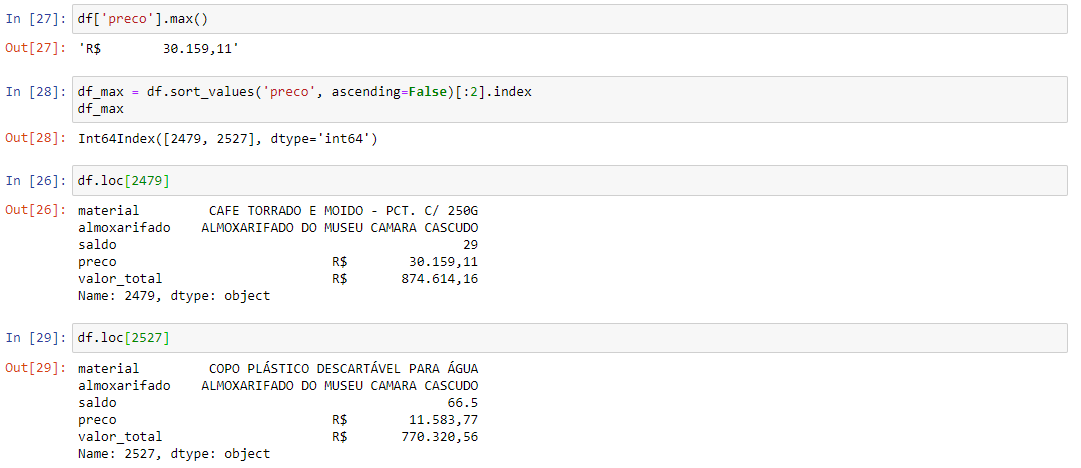
Well, it’s true! 😐 Also 11 thousand $ in plastic cups.
好吧,这是真的! 😐还有一万一千美元的塑料杯。
Another strange thing that i realize is that 107 items have 1 cent as a price:
我意识到的另一件奇怪的事是107件商品的价格为1美分 :
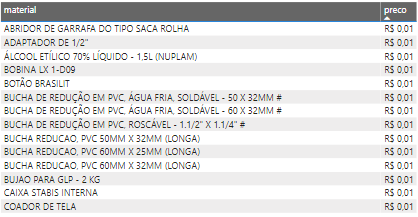
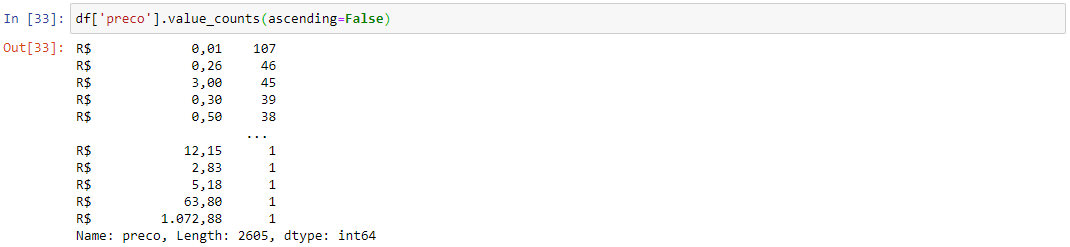
Does all these items really costs just 1 cent? Someone will need to answer it.
所有这些物品真的只花费1美分吗? 有人需要回答。
SO WHAT??
所以呢??
Analysing this dataset we have seen that there is A LOT of money spent in coffe and plastic cup. ALMOST 1 MILLION!!!??? 💸💸💸
通过分析该数据集,我们发现在咖啡和塑料杯上花费了很多钱。 几乎一百万!!!!!! 💸💸💸
Maybe something it’s wrong…With this information i would probably check the veracity of the facts. Perhaps, the item’s description is not as much meaningfull as it could be (how big is this package?)
也许有些问题……有了这些信息,我可能会检查事实的真实性。 也许,该项目的描述没有那么有意义(此包装有多大?)
Some inconclusions can turn into conclusions. In this case, some data curation could be needed.
一些结论可以得出结论。 在这种情况下,可能需要一些数据管理。
If you have some tips, comments or any suggestions, i would be very happy to learn more with you guys. I’m just a begginer.
如果您有任何提示,意见或建议,我将非常高兴与你们一起学习更多。 我只是一个初学者。
Thank you for your time! 🙏
感谢您的时间! 🙏
翻译自: https://medium.com/análises-exploratórias-de-dados/exploratory-analysis-of-ufrn-universidade-federal-do-rio-grande-do-norte-warehouses-e6f2ff334b0f
北方工业大学gpa计算
本文来自互联网用户投稿,该文观点仅代表作者本人,不代表本站立场。本站仅提供信息存储空间服务,不拥有所有权,不承担相关法律责任。如若转载,请注明出处:http://www.mzph.cn/news/389288.shtml
如若内容造成侵权/违法违规/事实不符,请联系多彩编程网进行投诉反馈email:809451989@qq.com,一经查实,立即删除!