泰坦尼克数据集预测分析
Data is simply useless until you don’t know what it’s trying to tell you.
除非您不知道数据在试图告诉您什么,否则数据将毫无用处。
With this quote we’ll continue on our quest to find the hidden secrets of the Titanic. ‘The Unsinkable’, as it was claimed by its designers and makers proved that even the best of human engineering may sometimes fail when nature comes on to test it.
用这句话,我们将继续寻找泰坦尼克号的秘密。 正如其设计师和制造商所宣称的,“坚不可摧”证明了,即使人类最好的工程学,有时也会由于自然的考验而失败。
In last article, we saw the different attributes of the data and had quick glance on what the data looked like. If you haven’t read part 1 of this blog , I recommend you to kindly read it by clicking here before continuing. In this article we’ll look at the relationships of each of the attributes to the survival of the passenger and to continue with our quest to find out whether you would’ve survived the Titanic Sinking or not.
在上一篇文章中,我们看到了数据的不同属性,并快速浏览了数据的外观。 如果您还没有阅读本博客的第1部分,建议您在继续之前单击此处 ,请仔细阅读。 在本文中,我们将研究每个属性与乘客生存的关系,并继续我们的探索,以找出您是否会在《泰坦尼克号沉没》中幸存。
1.旅客舱位与生存的关联 (1. Co-Relation of Passenger Class with the survival)
Since, there are 3 classes present in the ship. Let’s find out the count of each passengers in each class.
此后,船上共有3个班级。 让我们找出每个班级的每位乘客人数。
Output:
输出:

Now, let’s find out the the total number of survivors from each class
现在,让我们找出每个班级的幸存者总数
Output:
输出:

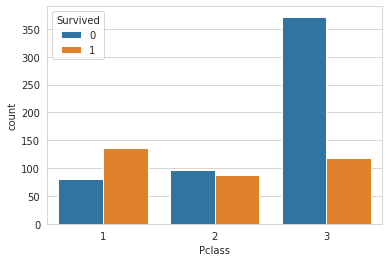
As you can see, the percentage of the passengers belonging to Upper Class who survived is better than the rest of the two having a survival percentage of around 62.96%.
如您所见,幸存的上层阶级乘客百分比要好于其余两个的生存百分比(约62.96%)。
The Survival Percentage of Middle Class Passengers is around 47.28% which better than the lower class but worse than that of the Upper Class
中产阶级乘客的生存率大约为47.28%,高于低层阶级,但低于上层阶级
The Lower Class was hit the most, having a survival percentage of just 24.23% which is significantly lower than the above two classes.
下层阶级受到的打击最大,生存率仅为24.23%,明显低于上述两个阶级。
The results indicate that the survival of the Titanic Sink was largely affected by the class in which you belong indicating the discrimination based on the class.
结果表明,泰坦尼克号水槽的生存在很大程度上受到您所属类别的影响,表明基于类别的歧视。
2.性别与生存的关系 (2. Co-Relation of Gender with the survival)
Let’s start by printing the number of passengers of each gender.
让我们开始打印每种性别的乘客数量。
Output:
输出:

Now, let’s find out the survival percentage of the passengers belonging to each gender.
现在,让我们找出每种性别的乘客的生存率。
Output:
输出:

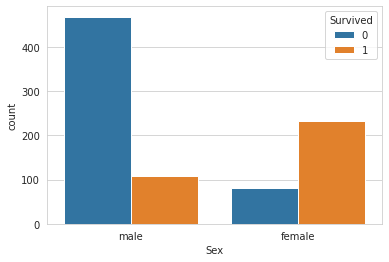
The information suggests that the women were given the highest priority while saving lives. Almost 74.2% of the women survived and 18.89% of men survived. (How pure these gentlemen were!😢❤️)
信息表明,在挽救生命的同时,妇女被赋予最高优先权。 几乎有74.2%的女性得以幸存,而18.89%的男性得以幸存。 (这些先生们真是纯洁!😢❤️)
3.年龄与生存的关系 (3. Co-Relation of Age with Survival)
Now, let’s look at the effect of age on the survival. But first, let’s have a quick glance on some stats of the age along with the values that are missing in the data-set.
现在,让我们看看年龄对生存的影响。 但是首先,让我们快速浏览一下年龄的一些统计数据以及数据集中缺少的值。
Output:
输出:


There are a total of 177 missing values i.e. the age of 177 Passengers are missing in the data-set. These missing values may pose some problems while predicting and hence, need to be addressed.
共有177个缺失值,即数据集中缺少177岁的乘客。 这些缺失值在预测时可能会带来一些问题,因此需要解决。
Now, let’s visualize by plotting some histograms on the basis of the data
现在,让我们根据数据绘制一些直方图以进行可视化
kde = True gives Kernel Density Function for the histogram and rug are the small markings which plots the exact point at which the data were recorded.
kde = True给出了直方图的内核密度函数,而rug是小的标记,它们绘制了记录数据的精确点。
Output:
输出:
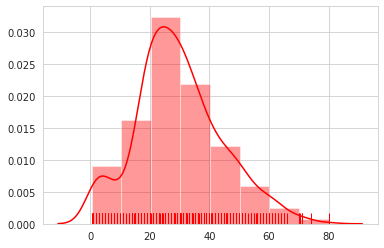
Now, let’s check out the survival in each group by plotting the following graph with kde. The y-axis actually denotes probability density function for the kernel density estimation and the area under the kde curve give the probability of respective points in x-axis.
现在,让我们用kde绘制下图来检查每组的存活率。 y轴实际上表示用于核密度估计的概率密度函数,而kde曲线下的面积给出了x轴上各个点的概率。
Output:
输出:
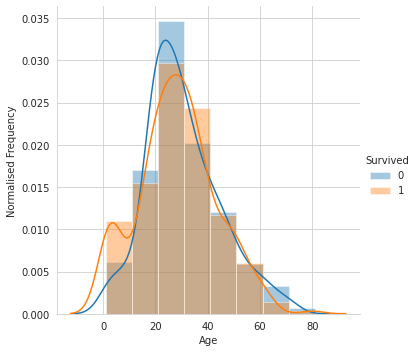
The following plot show the distribution of gender in each age group.
下图显示了各个年龄段的性别分布。
Output:
输出:
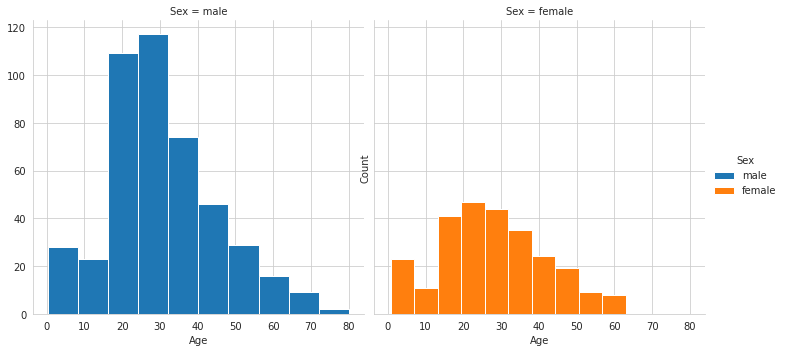
Now, let’s find out comparison of survival in each of these groups using kde plot.
现在,让我们使用kde图找出这些组中每个组的生存率比较。
Output:
输出:
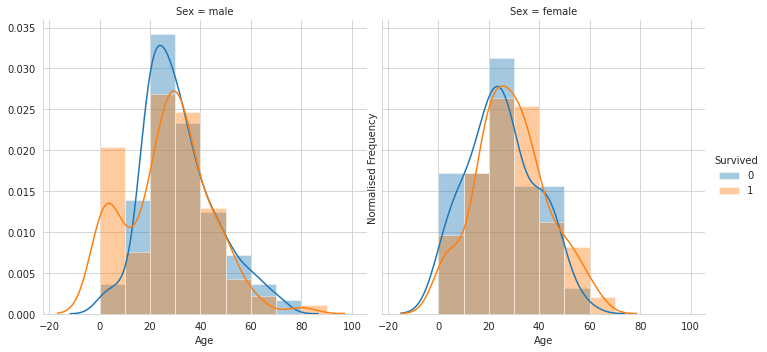
We can also understand what’s represented in these histograms as follows:
我们还可以理解这些直方图中的表示形式,如下所示:
Output:
输出:
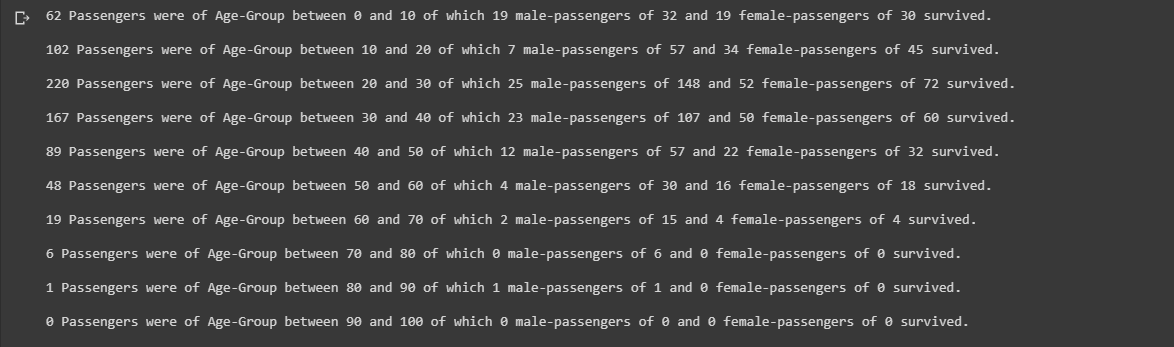
4.否的关联 幸存旅客的兄弟姐妹/配偶 (4. Co-Relation of no. of Siblings/Spouses of the passenger with Survival)
Let’s start by understanding the distribution of values of this attribute.
让我们首先了解该属性的值的分布。
Output:
输出:

Now, let’s plot the histogram describing the survival of the passengers having respective number of Siblings/Spouses.
现在,让我们绘制直方图,描述具有相应数量的兄弟姐妹/配偶的乘客的生存情况。
Output:
输出:
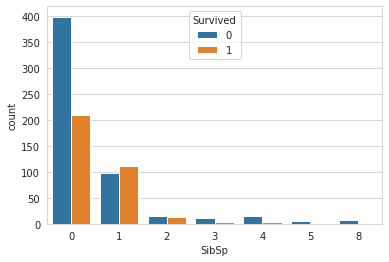
The inference of the above histogram can be derived using the following code:
可以使用以下代码推导以上直方图的推论:
Output:
输出:

5.父母/子女人数与生存率的相互关系 (5. Co-relation of No. of Parents/Children with survival)
The distribution of the number of Parents/Children are as follows
父母子女数的分配如下
Output:
输出:

Here are the two different plots denoting the survival of passengers having respective no. of Parents/Children. The first one using ‘distplot’ and the second one using ‘countplot’
这是两个不同的图,分别表示编号分别为的乘客的生存情况。 父母/子女。 第一个使用“ distplot”,第二个使用“ countplot”
Output:
输出:
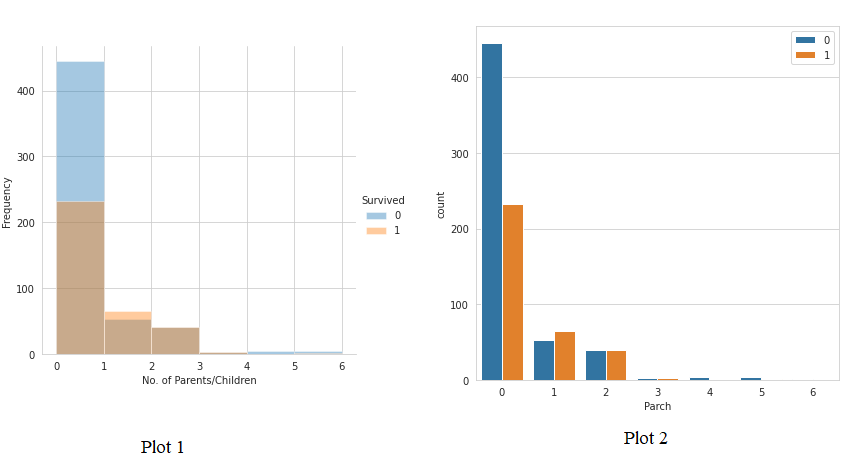
6.票价与生存的关联 (6. Co-relation of Fare with survival)
Now, let’s try to understand if there was any regularity in the fare and whether there’s any relation with the survival. The code describes the distribution of the fare.
现在,让我们尝试了解票价是否有规律性以及与生存率是否有关系。 该代码描述了票价的分配。
Output:
输出:

Let’s plot the distribution of the Fare classified by the Survival
让我们绘制按幸存分类的票价分布
Output:
输出:
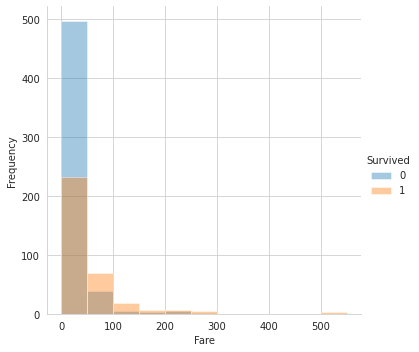
Let’s check whether the passengers were charged uniformly or not. If yes, let’s try to understand what are the factors that decided the fare for the tickets.
让我们检查一下乘客是否被统一收费。 如果是,让我们尝试了解决定门票价格的因素是什么。
To check whether ‘Gender’ was the factor to decide the fare of the tickets, here’s the plot for each embarkation followed by the inference of it.
要检查“性别”是否是决定票价的因素,以下是每次登机的情节,然后进行推断。
Output:
输出:
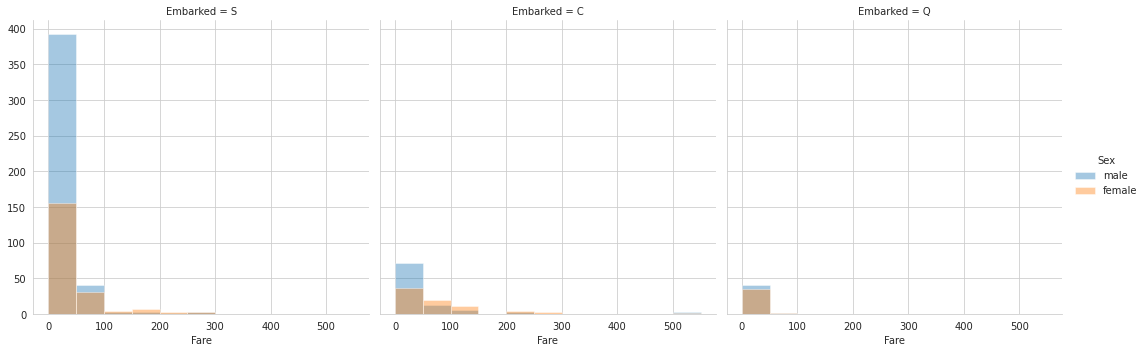
Output:
输出:



Thus, as per the data, mean fare charged for women were significantly higher in Cherbourg and Southampton.
因此,根据数据,瑟堡和南安普敦的女性平均车费要高得多。
To check whether ‘Embarkation’ , ‘Class’ and ‘Age’ were the factor deciding the fare of the tickets, here’s the plot for each embarkation and class classified with ‘Survival’ Status followed by the inference of it.
要检查“入库”,“舱位”和“年龄”是否是决定票价的因素,这是按“生存”状态归类的每个登乘舱位和舱位的图,然后进行推断。
Output:
输出:
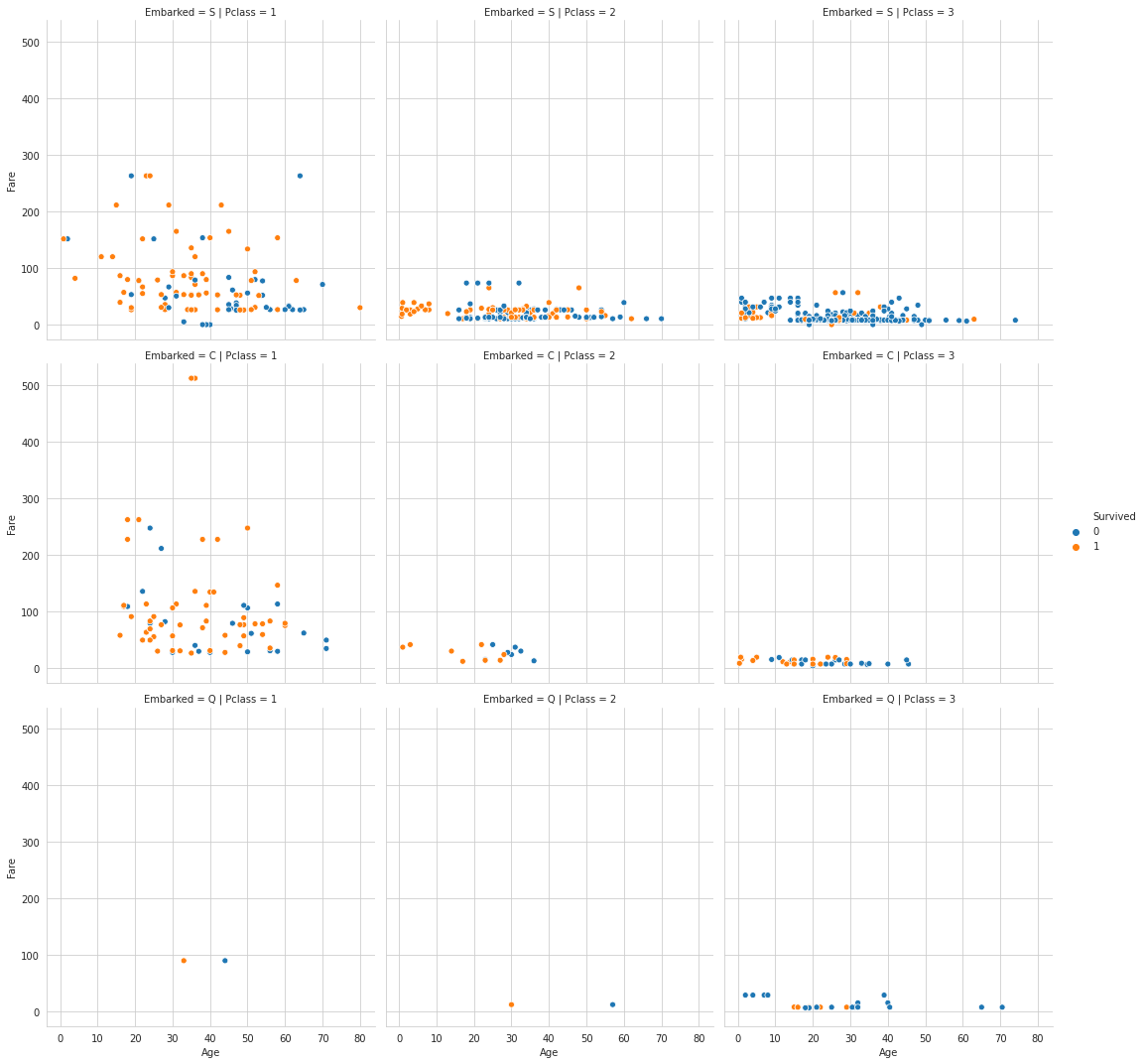
Thus, it is evident from the data that tickets were priced mostly on the basis of Pclass and the point of Embarkation but not on the basis of Age.
因此,从数据中可以明显看出,机票的定价主要基于Pclass和登机地点,而不是基于年龄。
7.登船与生存的关系 (7. Co-relation of Embarkation with survival)
We have seen the description of the data having numerical attributes till now. Here’s a look at the description of the categorical data.
到目前为止,我们已经看到了对具有数值属性的数据的描述。 这里是对分类数据的描述。
Output:
输出:

Here’s a plot describing the ratio of the survival of passengers from each port of Embarkation.
这是一张描述每个登船口岸旅客生存率的图表。
Output:
输出:
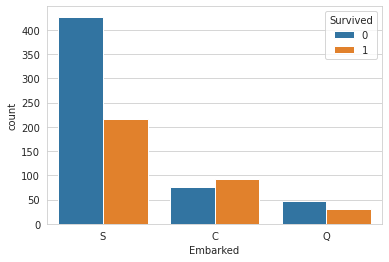
And now here’s the pair-plot of each of the attributes that we have discussed till now.
现在,这是到目前为止我们讨论过的每个属性的配对图。
Output:
输出:
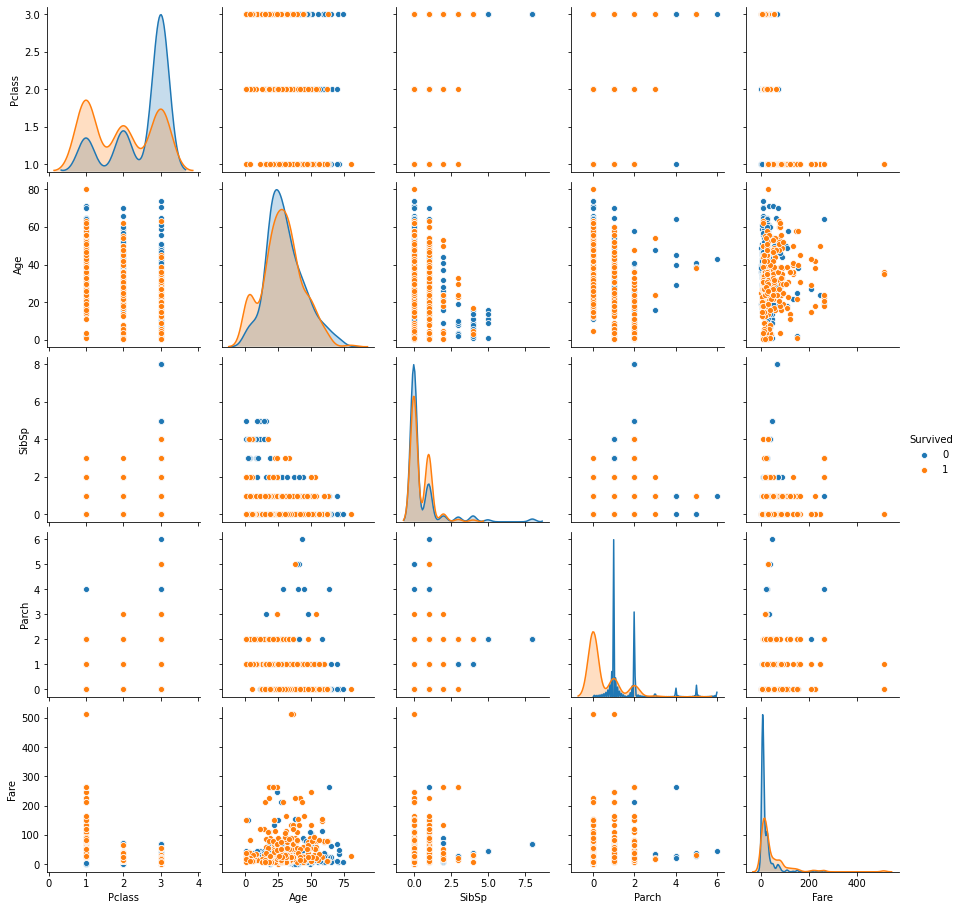
As you might have noticed we’ve ignored Passenger_Id, Name of the Passenger, Ticket and Cabin No. as they play little to no role in determining the survival of the passenger.
您可能已经注意到,我们已经忽略了Passenger_Id , 乘客 姓名 , 机票和机舱号 。 因为它们在决定乘客的生存方面几乎没有作用。
Thus, we tried to understand the data by visualizing using various techniques and uncovered various mysteries related to Titanic. In next Article we’ll be understanding the types of data and why some type of data need to be converted into the specific format to be able to fit various Machine Learning models on it. Thank you for joining throughout this journey of exploration and hope, you’ve got the experience of being a detective!🕵
因此,我们试图通过使用各种技术进行可视化来理解数据,并发现与泰坦尼克号有关的各种奥秘。 在下一篇文章中,我们将了解数据的类型以及为什么需要将某种类型的数据转换为特定格式才能适合其上的各种机器学习模型。 感谢您加入探索和希望的整个旅程,您已经成为一名侦探!🕵
Link to the Notebook: Click Here
链接到笔记本: 单击此处
Link to Part 1 of this Blog: Click Here
链接到此博客的第1部分: 单击此处
翻译自: https://medium.com/@bapreetam/exploratory-data-analysis-a-case-study-on-titanic-data-set-part-2-96a9f3df963a
泰坦尼克数据集预测分析
本文来自互联网用户投稿,该文观点仅代表作者本人,不代表本站立场。本站仅提供信息存储空间服务,不拥有所有权,不承担相关法律责任。如若转载,请注明出处:http://www.mzph.cn/news/389287.shtml
如若内容造成侵权/违法违规/事实不符,请联系多彩编程网进行投诉反馈email:809451989@qq.com,一经查实,立即删除!