rfm模型分析与客户细分
With some free time at hand in the midst of COVID-19 pandemic, I decided to do pro bono consulting work. I was helping a few e-commerce companies with analyzing their customer data. A common theme I encountered during this work was that the companies were more interested in getting a list of their best customers so they could run some marketing campaign to boost their revenue. My recommendation has always been that every customer segment has some valuable insight to offer and that best customers depend on the immediate company goal/objective at hand. In other words, best customers are not always what we call the high-value customers. In this article, I will highlight various insights RFM segmentation can provide.
在COVID-19大流行期间有一些空闲时间,我决定进行无偿咨询工作。 我曾帮助一些电子商务公司分析其客户数据。 我在这项工作中遇到的一个共同主题是,这些公司对获取最佳客户名单更感兴趣,因此他们可以开展一些营销活动来增加收入。 我的建议一直是,每个客户群都应提供一些有价值的见解,并且最好的客户取决于当前的公司目标。 换句话说,最佳客户并不总是我们所谓的高价值客户。 在本文中,我将重点介绍RFM细分可以提供的各种见解。
使用RFM分析进行细分 (Segmentation using RFM Analysis)
Analysis based on RFM — stands for Recency, Frequency and Monetary — is probably one of the easiest ways to segment the customers given companies usually have the customer purchase information readily available to them.
鉴于公司通常都容易获得客户购买信息,因此基于RFM的分析(代表新近度,频率和货币)可能是细分客户的最简单方法之一。
Here is a sample customer purchase history data that is needed for the RFM analysis. With this, each customer is scored on the RFM attributes on a scale of 1–5 (or 1–4 or 1–3, depending on how granular you want to look at the purchase behavior) with 1 being the least and 5 being the best score.
这是RFM分析所需的样本客户购买历史记录数据。 这样一来,每位客户在RFM属性上的评分为1-5(或1-4或1-3,具体取决于您希望如何看待购买行为),其中1最小,5最小。最好成绩。
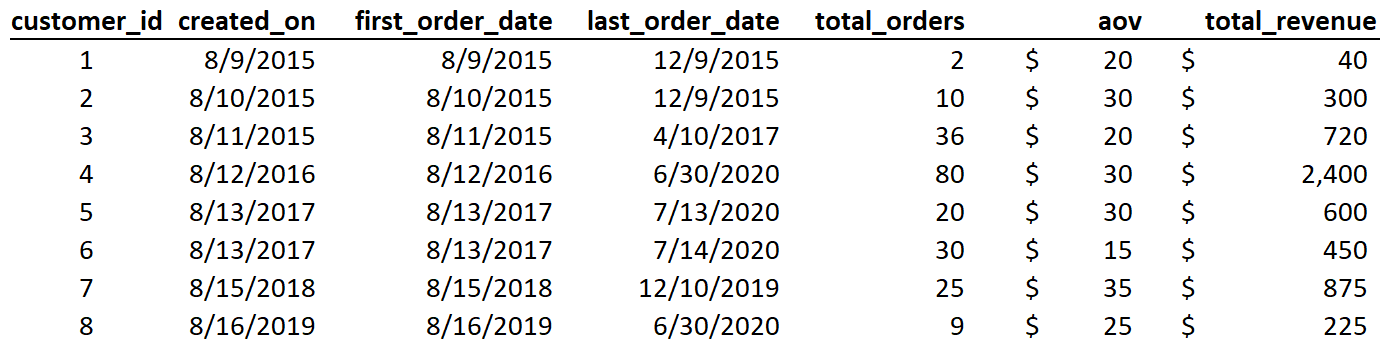
For example, a customer with a most recent purchase will have a score of 5 on Recency, whereas a customer who hasn’t purchased in a while will have a score of 1.
例如,最近购物的客户在Recency上的得分为5,而一段时间内没有购物的客户的得分为1。
Here the important thing to note is that the criteria for scoring varies business by business and by understanding customer purchase cycles, we can come up with the scoring criteria. For this sample data, I used the following recency scoring based on 2-month purchase periods. Once you score on all three attributes, we can create RFM score (sum of these three scores) and RFM Category (when R=4, F=3, M=4, RFM Category = 434). You might have seen cases where RFM score was used to segment customers. This method has some serious pitfalls and its better to use the RFM category instead.
这里要注意的重要一点是,评分标准因企业而异,并且通过了解客户购买周期,我们可以提出评分标准。 对于此样本数据,我基于2个月的购买期使用了以下新近度评分。 在所有三个属性上得分后,我们可以创建RFM得分(这三个得分的总和)和RFM类别(当R = 4,F = 3,M = 4,RFM类别= 434时)。 您可能已经看到过使用RFM分数细分客户的情况。 此方法有一些严重的陷阱,最好使用RFM类别。
Using a 5 point scale could lead up to 125 RFM categories. Next step is to group these RFM categories into various segments. While the standard RFM analysis doesn’t take into account other data points such as length of the relationship of a customer, but this is the time to look at the purchase data holistically and assign segments to the RFM categories. For example, a customer with long relationship with the business, most recent purchases but low monetary might have used promotional offers more frequently than a customer with similar recency, monetary but less frequent and shorter relationship with us. One could be segmented into Deal Seeker and the other one could be segmented into Newcomers.
使用5分制可能会导致多达125个RFM类别。 下一步是将这些RFM类别分为不同的细分。 尽管标准的RFM分析未考虑其他数据点,例如客户关系的长度,但这是时候全面查看购买数据并将细分分配给RFM类别的时候了。 例如,与企业有长期关系,最近购买但货币较少的客户可能比具有类似新近度,货币但频率较低,与我们关系较短的客户更频繁地使用促销优惠。 一个可以细分为Deal Seeker ,另一个可以细分为Newcomers 。

In the above example, I have identified 6 segments with uniquely distinguishable purchase behaviors.
在上面的示例中,我确定了6个具有独特可区分购买行为的细分。
Dropouts: In other words these are the lost customers. They became our customers through initial promotional offer and either didn’t come back or made 1–2 subsequent purchases.
辍学:换句话说,这些是失去的客户。 他们通过最初的促销活动成为我们的客户,要么没有回来,要么随后进行了1-2次购买。
Early Enthusiasts: These customers were with the company for a relatively longer period of time than Dropouts and made frequent purchases and spent lot of money. However, they were lost along the way. These will be the best customers if the objective is to reactivate the lost customers.
早期发烧友:与Dropouts相比,这些客户在公司呆的时间相对较长,并且经常购买商品并花了很多钱。 但是,他们一路迷路了。 如果目标是重新激活失去的客户,这些将是最好的客户。
Newcomers: As the name suggests, this segment consists of customers who are relatively new and active. Any marketing efforts for this segment could be to promote them to loyalty programs or offer promotions to try other products.
新移民:顾名思义,该细分市场由相对较新且活跃的客户组成。 此细分市场的任何营销工作可能是将其推广到会员计划或提供促销以尝试其他产品。
Deal Seekers: These customers have relatively long relationship with the company and are currently active. They are interested in making frequent purchases but usually on look out for promotional offers. These will be the best customers if running a campaign to boost sales.
寻求交易者:这些客户与公司的关系相对较长,并且目前处于活跃状态。 他们有兴趣频繁购买商品,但通常会寻找促销优惠。 如果开展宣传活动以提高销量,这些将是最好的客户。
Potential High Value Customers: These are relatively newer customers compared to Deal Seekers but are less sensitive to the price or are less keen on promotional offers. In shorter period of time, they made frequent purchases and spent lot of money with the company. Nudge them towards High Value Customers and sign them up for loyalty program.
潜在的高价值客户:与“寻求交易者”相比,这些客户相对较新,但对价格不太敏感或对促销活动不感兴趣。 在较短的时间内,他们经常购买商品,并在公司花了很多钱。 将他们推向高价值客户,并签署他们的会员计划。
High-Value Customers: These are the most loyal customers. They are active with frequent purchases and high monetary value. They could be the brand evangelists and should focus on serving them well. They could be the best customers to get feedback on any new product launches or be the early adopters or promoters.
高价值客户:这些是最忠诚的客户。 他们很活跃,经常购买并且具有很高的货币价值。 他们可能是品牌传播者,应该专注于为他们提供良好的服务。 他们可能是获得任何新产品发布反馈的最佳客户,或者是早期采用者或推广者。
从可视化中获取见解 (Getting Insights from Visualization)
Once the customer segments have been identified using RFM analysis, it is always a good idea to visualize these segments not only for a sanity check but also to derive actionable insights. I used Tableau to visualize the segments.
一旦使用RFM分析确定了客户群,将这些细分形象化以进行健全性检查并获得可行的见解始终是一个好主意。 我使用Tableau可视化了细分。

Recency and Frequency score matrix chart is usually a good one for sanity check. As R score and F score increase, the monetary value increases. Higher R score may not necessarily lead to higher monetary value given that the business may have acquired new customers recently.
新近度和频率得分矩阵图通常是进行健全性检查的好方法。 随着R分数和F分数增加,货币价值增加。 鉴于企业最近可能获得了新客户,因此较高的R分数不一定会导致较高的货币价值。

The treemap shows how each customer segment is contributing to the total revenue and how much each customer within a given segment is contributing to the total revenue. In a way, this helps predict how consistent revenues will be given how many customers have churned and how active other segments are.
树状图显示了每个客户群如何为总收入做出贡献,以及给定细分市场中的每个客户为总收入做出了多少贡献。 从某种意义上说,这有助于预测将有多少客户流失以及其他细分市场的活跃程度如何带来稳定的收入。
When there is geographic information for the customers, plotting them on a map will give some unique insights. One of the interesting cases I have seen is for an e-commerce company with some retail presence. Mapping the customer geographic data helped explain clusters of online shoppers around geographies where the company has retail store presence. Interestingly enough, these clusters of online customers were acquired organically. Accordingly, you can evaluate strategies to expand retail presence that could potentially boost e-commerce business.
当有客户的地理信息时,将其绘制在地图上将提供一些独特的见解。 我所看到的一个有趣的案例是一家拥有零售业务的电子商务公司。 映射客户地理数据有助于解释公司在零售商店中存在的地理位置周围的在线购物者群体。 有趣的是,这些在线客户群是有机收购的。 因此,您可以评估策略来扩展零售业务,这可能会促进电子商务业务。


Another thing is the channel performance. Customer segmentation helps with understanding channel performance better. By looking at customer lifetime value (LTV) per segment and what portion of each segment is attributable to a channel, acquisition cost associated with that channel, we can evaluate channel performance more accurately than without segmentation.
另一件事是频道性能。 客户细分有助于更好地了解渠道绩效。 通过查看每个细分的客户生命周期价值(LTV)以及每个细分的哪个部分可归因于某个渠道以及与该渠道相关的购置成本,我们可以比不进行细分来更准确地评估渠道效果。
结论 (In Conclusion)
Usually, startups tend to be growth focused rather than retention focused. Even though they are resource constrained, startups are better off investing some of their limited resources on understanding their existing customers so they can device effective retention strategies that could, in turn, help them with acquiring new customers. After all, it may end up being much cheaper to retain your existing customer than to acquire a new one. Word of mouth is still a major customer acquisition channel and you want your customers to be the evangelists for your product.
通常,初创公司往往注重增长而不是保留。 尽管他们受到资源的限制,但初创公司最好将一些有限的资源用于了解他们的现有客户,以便他们可以制定有效的保留策略,从而帮助他们获得新客户。 毕竟,保留您的现有客户可能比购买新客户便宜得多。 口耳相传仍然是主要的客户获取渠道,您希望您的客户成为产品的传播者。
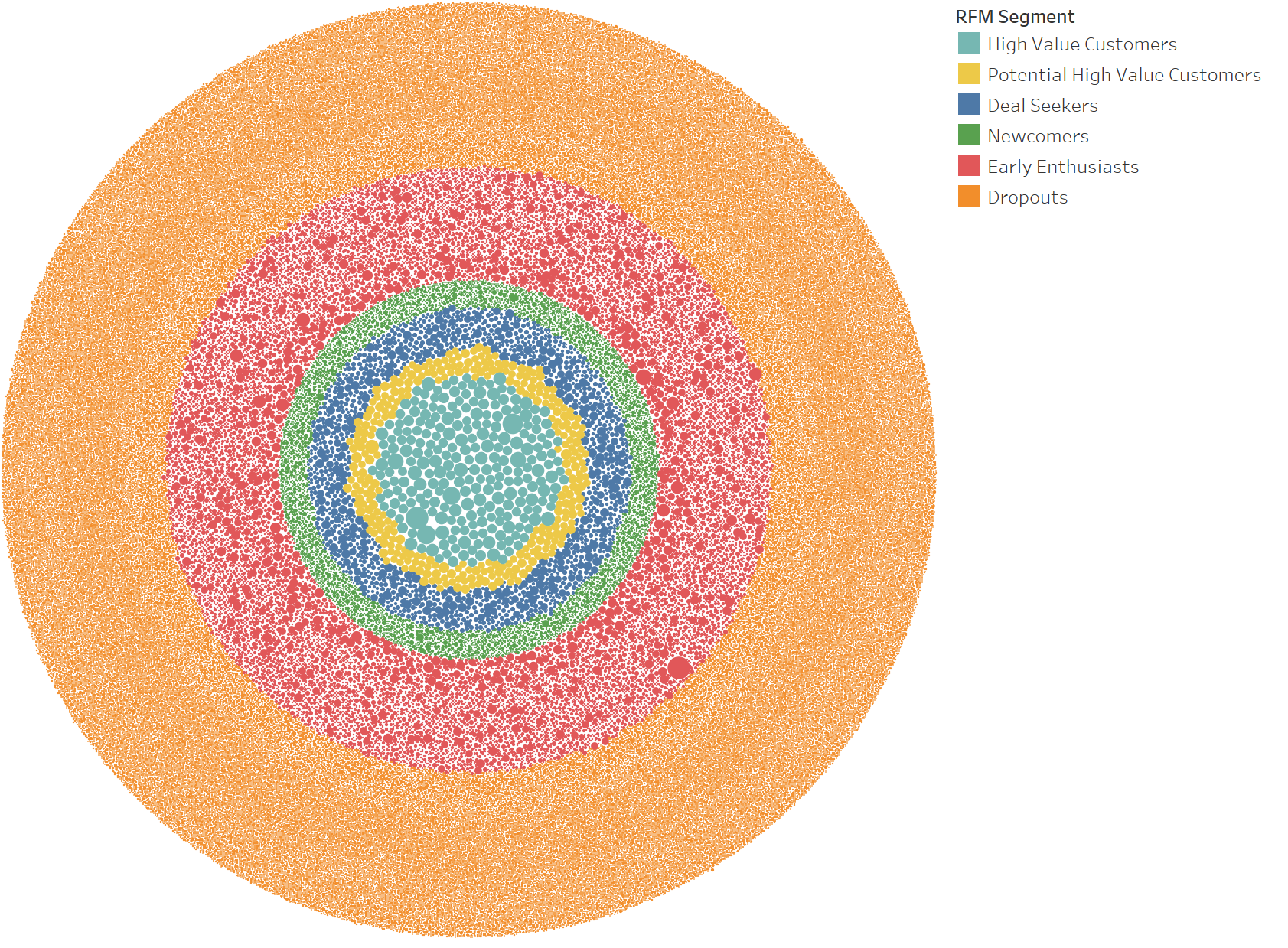
Reiterating the quest for the best customers, it may seem obvious that high-value customers are the best customers. When there is a goal at hand — say to run a marketing campaign to boost revenue — we should dig a little deeper to find out who the best customer would be for that specific goal or which customer segment is at the bullseye.
重申对最佳客户的追求,高价值客户显然是最佳客户。 当有目标时(例如,开展营销活动以增加收入),我们应该进行更深入的研究,以找出谁是实现该特定目标的最佳客户,或者哪个客户群处于靶心位置。
翻译自: https://towardsdatascience.com/how-to-identify-the-best-customers-using-rfm-based-segmentation-a0a16c34a859
rfm模型分析与客户细分
本文来自互联网用户投稿,该文观点仅代表作者本人,不代表本站立场。本站仅提供信息存储空间服务,不拥有所有权,不承担相关法律责任。如若转载,请注明出处:http://www.mzph.cn/news/391420.shtml
如若内容造成侵权/违法违规/事实不符,请联系多彩编程网进行投诉反馈email:809451989@qq.com,一经查实,立即删除!