多变量线性相关分析
现实世界中的数据科学 (Data Science in the Real World)
This article aims to present two ways of calculating non linear correlation between any number of discrete variables. The objective for a data analysis project is twofold : on the one hand, to know the amount of information the variables share with each other, and therefore, to identify whether the data available contain the information one is looking for ; and on the other hand, to identify which minimum set of variables contains the most important amount of useful information.
本文旨在介绍两种计算任意数量的离散变量之间的非线性相关性的方法。 数据分析项目的目标是双重的:一方面,了解变量之间共享的信息量,从而确定可用数据是否包含人们正在寻找的信息; 另一方面,确定哪些最小变量集包含最重要的有用信息量。
变量之间的不同类型的关系 (The different types of relationships between variables)
线性度 (Linearity)
The best-known relationship between several variables is the linear one. This is the type of relationships that is measured by the classical correlation coefficient: the closer it is, in absolute value, to 1, the more the variables are linked by an exact linear relationship.
几个变量之间最著名的关系是线性关系。 这是用经典相关系数衡量的关系类型:绝对值越接近1,变量之间通过精确的线性关系链接的越多。
However, there are plenty of other potential relationships between variables, which cannot be captured by the measurement of conventional linear correlation.
但是,变量之间还有许多其他潜在的关系,无法通过常规线性相关性的测量来捕获。
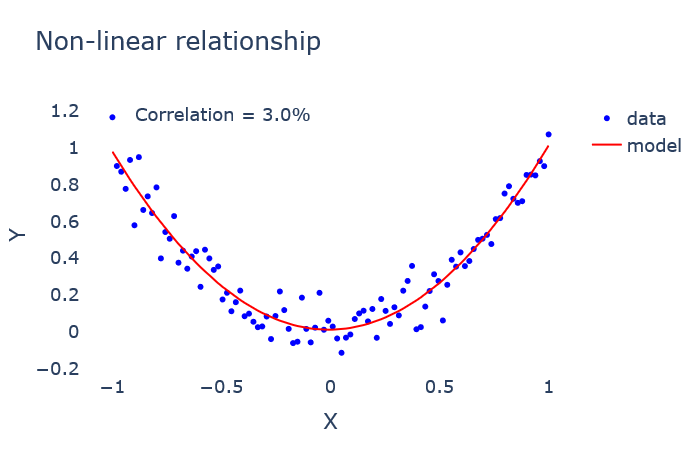
To find such non-linear relationships between variables, other correlation measures should be used. The price to pay is to work only with discrete, or discretized, variables.
为了找到变量之间的这种非线性关系,应该使用其他相关度量。 要付出的代价是仅对离散变量或离散变量起作用。
In addition to that, having a method for calculating multivariate correlations makes it possible to take into account the two main types of interaction that variables may present: relationships of information redundancy or complementarity.
除此之外,拥有一种用于计算多元相关性的方法,可以考虑变量可能呈现的两种主要交互类型:信息冗余或互补性的关系。
冗余 (Redundancy)
When two variables (hereafter, X and Y) share information in a redundant manner, the amount of information provided by both variables X and Y to predict Z will be inferior to the sum of the amounts of information provided by X to predict Z, and by Y to predict Z.
当两个变量(以下,X和Y)以冗余的方式共享信息,由两个变量X和Y中提供的信息来预测的Z量将不如由X所提供的预测的Z信息的量的总和,和由Y预测Z。
In the extreme case, X = Y. Then, if the values taken by Z can be correctly predicted 50% of the times by X (and Y), the values taken by Z cannot be predicted perfectly (i.e. 100% of the times) by the variables X and Y together.
在极端情况下, X = Y。 然后,如果可以通过X (和Y )正确地预测Z所取的值的50%时间,则变量X和Y不能一起完美地预测Z所取的值(即100%的时间)。
╔═══╦═══╦═══╗
║ X ║ Y ║ Z ║
╠═══╬═══╬═══╣
║ 0 ║ 0 ║ 0 ║
║ 0 ║ 0 ║ 0 ║
║ 1 ║ 1 ║ 0 ║
║ 1 ║ 1 ║ 1 ║
╚═══╩═══╩═══╝
互补性 (Complementarity)
The complementarity relationship is the exact opposite situation. In the extreme case, X provides no information about Z, neither does Y, but the variables X and Y together allow to predict perfectly the values taken by Z. In such a case, the correlation between X and Z is zero, as is the correlation between Y and Z, but the correlation between X, Y and Z is 100%.
互补关系是完全相反的情况。 在极端情况下, X不提供有关Z的信息, Y也不提供任何信息,但是变量X和Y一起可以完美地预测Z所取的值。 在这种情况下, X和Z之间的相关性为零, Y和Z之间的相关性也为零,但是X , Y和Z之间的相关性为100%。
These complementarity relationships only occur in the case of non-linear relationships, and must then be taken into account in order to avoid any error when trying to reduce the dimensionality of a data analysis problem: discarding X and Y because they do not provide any information on Z when considered independently would be a bad idea.
这些互补关系仅在非线性关系的情况下发生,然后在尝试减小数据分析问题的维数时必须考虑到它们以避免错误:丢弃X和Y,因为它们不提供任何信息在Z上单独考虑时,将是一个坏主意。
╔═══╦═══╦═══╗
║ X ║ Y ║ Z ║
╠═══╬═══╬═══╣
║ 0 ║ 0 ║ 0 ║
║ 0 ║ 1 ║ 1 ║
║ 1 ║ 0 ║ 1 ║
║ 1 ║ 1 ║ 0 ║
╚═══╩═══╩═══╝
“多元非线性相关性”的两种可能测度 (Two possible measures of “multivariate non-linear correlation”)
There is a significant amount of possible measures of (multivariate) non-linear correlation (e.g. multivariate mutual information, maximum information coefficient — MIC, etc.). I present here two of them whose properties, in my opinion, satisfy exactly what one would expect from such measures. The only caveat is that they require discrete variables, and are very computationally intensive.
存在(多元)非线性相关性的大量可能度量(例如多元互信息,最大信息系数MIC等)。 我在这里介绍他们中的两个,我认为它们的性质完全满足人们对此类措施的期望。 唯一的警告是它们需要离散变量,并且计算量很大。
对称测度 (Symmetric measure)
The first one is a measure of the information shared by n variables V1, …, Vn, known as “dual total correlation” (among other names).
第一个是对n个变量V1,…,Vn共享的信息的度量,称为“双重总相关”(在其他名称中)。
This measure of the information shared by different variables can be characterized as:
不同变量共享的信息的这种度量可以表征为:

where H(V) expresses the entropy of variable V.
其中H(V)表示变量V的熵。
When normalized by H(V1, …, Vn), this “mutual information score” takes values ranging from 0% (meaning that the n variables are not at all similar) to 100% (meaning that the n variables are identical, except for the labels).
当用H(V1,…,Vn)归一化时,该“互信息分”取值范围从0%(意味着n个变量根本不相似)到100%(意味着n个变量相同,除了标签)。
This measure is symmetric because the information shared by X and Y is exactly the same as the information shared by Y and X.
此度量是对称的,因为X和Y共享的信息与Y和X共享的信息完全相同。
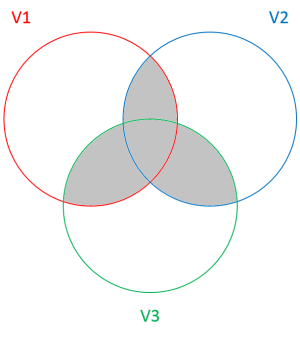
The Venn diagram above shows the “variability” (entropy) of the variables V1, V2 and V3 with circles. The shaded area represents the entropy shared by the three variables: it is the dual total correlation.
上方的维恩图用圆圈显示变量V1 , V2和V3的“变异性”(熵)。 阴影区域表示三个变量共享的熵:它是对偶总相关。
不对称测度 (Asymmetric measure)
The symmetry property of usual correlation measurements is sometimes criticized. Indeed, if I want to predict Y as a function of X, I do not care if X and Y have little information in common: all I care about is that the variable X contains all the information needed to predict Y, even if Y gives very little information about X. For example, if X takes animal species and Y takes animal families as values, then X easily allows us to know Y, but Y gives little information about X:
常用的相关测量的对称性有时会受到批评。 的确,如果我想将Y预测为X的函数,则我不在乎X和Y是否有很少的共同点信息:我只关心变量X包含预测Y所需的所有信息,即使Y给出关于X的信息很少。 例如,如果X取动物种类而Y取动物种类作为值,则X容易使我们知道Y ,但Y几乎没有提供有关X的信息:
╔═════════════════════════════╦══════════════════════════════╗
║ Animal species (variable X) ║ Animal families (variable Y) ║
╠═════════════════════════════╬══════════════════════════════╣
║ Tiger ║ Feline ║
║ Lynx ║ Feline ║
║ Serval ║ Feline ║
║ Cat ║ Feline ║
║ Jackal ║ Canid ║
║ Dhole ║ Canid ║
║ Wild dog ║ Canid ║
║ Dog ║ Canid ║
╚═════════════════════════════╩══════════════════════════════╝
The “information score” of X to predict Y should then be 100%, while the “information score” of Y for predicting X will be, for example, only 10%.
那么,用于预测Y的X的“信息分数”应为100%,而用于预测X的Y的“信息分数”仅为例如10%。
In plain terms, if the variables D1, …, Dn are descriptors, and the variables T1, …, Tn are target variables (to be predicted by descriptors), then such an information score is given by the following formula:
简而言之,如果变量D1,...,Dn是描述符,变量T1,...,Tn是目标变量(将由描述符预测),则这样的信息得分将由以下公式给出:

where H(V) expresses the entropy of variable V.
其中H(V)表示变量V的熵。
This “prediction score” also ranges from 0% (if the descriptors do not predict the target variables) to 100% (if the descriptors perfectly predict the target variables). This score is, to my knowledge, completely new.
此“预测分数”的范围也从0%(如果描述符未预测目标变量)到100%(如果描述符完美地预测目标变量)。 据我所知,这个分数是全新的。

The shaded area in the above diagram represents the entropy shared by the descriptors D1 and D2 with the target variable T1. The difference with the dual total correlation is that the information shared by the descriptors but not related to the target variable is not taken into account.
上图中的阴影区域表示描述符D1和D2与目标变量T1共享的熵。 与双重总相关的区别在于,不考虑描述符共享但与目标变量无关的信息。
实际中信息分数的计算 (Computation of the information scores in practice)
A direct method to calculate the two scores presented above is based on the estimation of the entropies of the different variables, or groups of variables.
计算上述两个分数的直接方法是基于对不同变量或变量组的熵的估计。
In R language, the entropy function of the ‘infotheo’ package gives us exactly what we need. The calculation of the joint entropy of three variables V1, V2 and V3 is very simple:
在R语言中,“ infotheo”程序包的熵函数提供了我们所需的信息。 三个变量V1 , V2和V3的联合熵的计算非常简单:
library(infotheo)df <- data.frame(V1 = c(0,0,1,1,0,0,1,0,1,1), V2 = c(0,1,0,1,0,1,1,0,1,0), V3 = c(0,1,1,0,0,0,1,1,0,1))entropy(df)[1] 1.886697
The computation of the joint entropy of several variables in Python requires some additional work. The BIOLAB contributor, on the blog of the Orange software, suggests the following function:
Python中几个变量的联合熵的计算需要一些额外的工作。 BIOLAB贡献者在Orange软件的博客上建议了以下功能:
import numpy as np
import itertools
from functools import reducedef entropy(*X): entropy = sum(-p * np.log(p) if p > 0 else 0 for p in
(np.mean(reduce(np.logical_and, (predictions == c for predictions, c in zip(X, classes))))
for classes in itertools.product(*[set(x) for x in X]))) return(entropy)V1 = np.array([0,0,1,1,0,0,1,0,1,1])V2 = np.array([0,1,0,1,0,1,1,0,1,0])V3 = np.array([0,1,1,0,0,0,1,1,0,1])entropy(V1, V2, V3)1.8866967846580784
In each case, the entropy is given in nats, the “natural unit of information”.
在每种情况下,熵都以nat(“信息的自然单位”)给出。
For a high number of dimensions, the information scores are no longer computable, as the entropy calculation is too computationally intensive and time-consuming. Also, it is not desirable to calculate information scores when the number of samples is not large enough compared to the number of dimensions, because then the information score is “overfitting” the data, just like in a classical machine learning model. For instance, if only two samples are available for two variables X and Y, the linear regression will obtain a “perfect” result:
对于大量维,信息分数不再可计算,因为熵计算的计算量很大且很耗时。 同样,当样本数量与维数相比不够大时,也不希望计算信息分数,因为就像经典的机器学习模型一样,信息分数会使数据“过度拟合”。 例如,如果对于两个变量X和Y只有两个样本可用,则线性回归将获得“完美”的结果:
╔════╦═════╗
║ X ║ Y ║
╠════╬═════╣
║ 0 ║ 317 ║
║ 10 ║ 40 ║
╚════╩═════╝
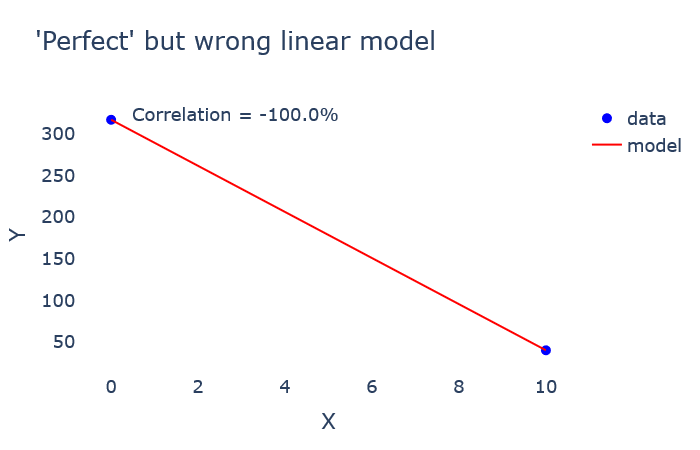
Similarly, let’s imagine that I take temperature measures over time, while ensuring to note the time of day for each measure. I can then try to explore the relationship between time of day and temperature. If the number of samples I have is too small relative to the number of problem dimensions, the chances are high that the information scores overestimate the relationship between the two variables:
同样,让我们想象一下,我会随着时间的推移进行温度测量,同时确保记下每个测量的时间。 然后,我可以尝试探索一天中的时间与温度之间的关系。 如果我拥有的样本数量相对于问题维度的数量而言太少,则信息分数很有可能高估了两个变量之间的关系:
╔══════════════════╦════════════════╗
║ Temperature (°C) ║ Hour (0 to 24) ║
╠══════════════════╬════════════════╣
║ 23 ║ 10 ║
║ 27 ║ 15 ║
╚══════════════════╩════════════════╝
In the above example, and based on the only observations available, it appears that the two variables are in perfect bijection: the information scores will be 100%.
在上面的示例中,并且基于仅可用的观察结果,看来这两个变量完全是双射的:信息得分将为100%。
It should therefore be remembered that information scores are capable, like machine learning models, of “overfitting”, much more than linear correlation, since linear models are by nature limited in complexity.
因此,应该记住,信息评分像机器学习模型一样,具有“过拟合”的能力,远远超过了线性相关性,因为线性模型天生就受到复杂性的限制。
预测分数使用示例 (Example of prediction score use)
The Titanic dataset contains information about 887 passengers from the Titanic who were on board when the ship collided with an iceberg: the price they paid for boarding (Fare), their class (Pclass), their name (Name), their gender (Sex), their age (Age), the number of their relatives on board (Parents/Children Aboard and Siblings/Spouses Aboard) and whether they survived or not (Survived).
泰坦尼克号数据集包含有关当泰坦尼克号与冰山相撞时在船上的887名乘客的信息:他们所支付的登船价格( 车费 ),其舱位( Pclass ),姓名( Name ),性别( Sex ) ,他们的年龄( Age ),在船上的亲戚数( 父母/子女和兄弟姐妹/配偶 )以及他们是否幸存( Survived )。
This dataset is typically used to determine the probability that a person had of surviving, or more simply to “predict” whether the person survived, by means of the individual data available (excluding the Survived variable).
该数据集通常用于通过可用的个人数据(不包括生存变量)来确定一个人生存的可能性,或更简单地“预测”该人是否生存 。
So, for different possible combinations of the descriptors, I calculated the prediction score with respect to the Survived variable. I removed the nominative data (otherwise the prediction score would be 100% because of the overfitting) and discretized the continuous variables. Some results are presented below:
因此,对于描述符的不同可能组合,我针对生存变量计算了预测得分。 我删除了名义数据(否则,由于过度拟合,预测得分将为100%),并离散化了连续变量。 一些结果如下所示:
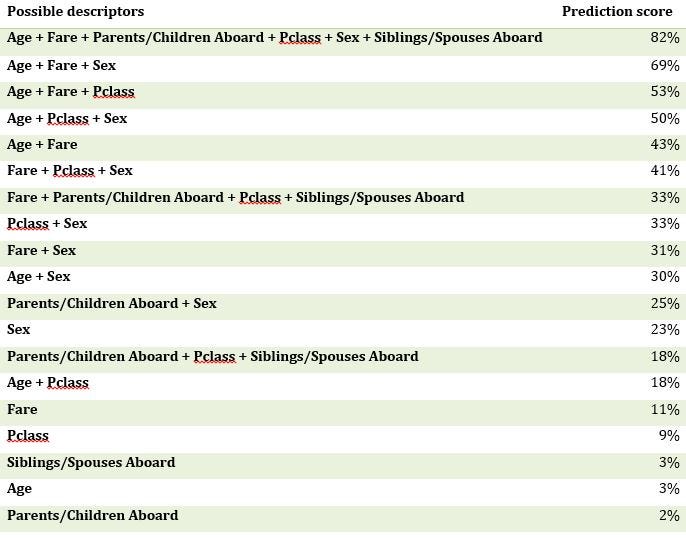
The first row of the table gives the prediction score if we use all the predictors to predict the target variable: this score being more than 80%, it is clear that the available data enable us to predict with a “good precision” the target variable Survived.
如果我们使用所有预测变量来预测目标变量,则表的第一行将给出预测得分:该得分超过80%,很明显,可用数据使我们能够“精确”地预测目标变量幸存下来 。
Cases of information redundancy can also be observed: the variables Fare, PClass and Sex are together correlated at 41% with the Survived variable, while the sum of the individual correlations amounts to 43% (11% + 9% + 23%).
信息冗余的情况下,也可以观察到:变量票价 ,PClass和性别在与幸存变量41%一起相关,而各个相关性的总和达43%(11%+ 9%+ 23%)。
There are also cases of complementarity: the variables Age, Fare and Sex are almost 70% correlated with the Survived variable, while the sum of their individual correlations is not even 40% (3% + 11% + 23%).
还有互补的情况: 年龄 , 票价和性别变量与生存变量几乎有70%相关,而它们各自的相关总和甚至不到40%(3%+ 11%+ 23%)。
Finally, if one wishes to reduce the dimensionality of the problem and to find a “sufficiently good” model using as few variables as possible, it is better to use the three variables Age and Fare and Sex (prediction score of 69%) rather than the variables Fare, Parents/Children Aboard, Pclass and Siblings/Spouses Aboard (prediction score of 33%). It allows to find twice as much useful information with one less variable.
最后,如果希望减少问题的范围并使用尽可能少的变量来找到“足够好”的模型,则最好使用年龄 , 票价和性别这三个变量(预测得分为69%),而不是变量票价 , 家长 / 儿童 到齐 ,Pclass和兄弟姐妹 / 配偶 到齐 (33%预测得分)。 它允许查找变量少一倍的有用信息。
Calculating the prediction score can therefore be very useful in a data analysis project, to ensure that the data available contain sufficient relevant information, and to identify the variables that are most important for the analysis.
因此,在数据分析项目中,计算预测分数可能非常有用,以确保可用数据包含足够的相关信息,并确定对于分析最重要的变量。
翻译自: https://medium.com/@gdelongeaux/how-to-measure-the-non-linear-correlation-between-multiple-variables-804d896760b8
多变量线性相关分析
本文来自互联网用户投稿,该文观点仅代表作者本人,不代表本站立场。本站仅提供信息存储空间服务,不拥有所有权,不承担相关法律责任。如若转载,请注明出处:http://www.mzph.cn/news/391403.shtml
如若内容造成侵权/违法违规/事实不符,请联系多彩编程网进行投诉反馈email:809451989@qq.com,一经查实,立即删除!