朴素贝叶斯实现分类
Naive Bayes classification is one of the most simple and popular algorithms in data mining or machine learning (Listed in the top 10 popular algorithms by CRC Press Reference [1]). The basic idea of the Naive Bayes classification is very simple.
朴素贝叶斯分类是数据挖掘或机器学习中最简单,最流行的算法之一(在CRC Press Reference [1]列出的十大流行算法中)。 朴素贝叶斯分类的基本思想很简单。
(In case you think video format is more suitable for you, you can jump here you can also go to the notebook.)
(如果您认为视频格式更适合您,则可以跳到此处 ,也可以转到笔记本 。)
基本直觉: (The basic Intuition:)
Let’s say, we have books of two categories. One category is Sports and the other is Machine Learning. I count the frequency of the words of “Match” (Attribute 1) and Count of the word “Algorithm” (Attribute 2). Let’s assume, I have a total of 6 books from each of these two categories and the count of words across the six books looks like the below figure.
假设我们有两类书籍。 一类是运动,另一类是机器学习。 我计算“匹配”(属性1)单词的出现频率和“算法”(属性2)单词的计数。 假设,我总共拥有这六类书中的六本书,这六本书中的单词数如下图所示。
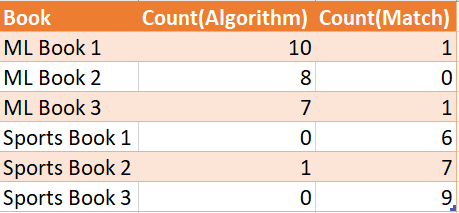
We see that clearly that the word ‘algorithm’ appears more in Machine Learning books and the word ‘match’ appears more in Sports. Powered with this knowledge, Let’s say if I have a book whose category is unknown. I know Attribute 1 has a value 2 and Attribute 2 has a value 10, we can say the book belongs to Sports Category.
我们清楚地看到,“算法”一词在机器学习书籍中出现的次数更多,而“匹配”一词在体育游戏中出现的次数更多。 借助这种知识,假设我有一本书的类别未知。 我知道属性1的值为2,属性2的值为10,可以说这本书属于“体育类别”。
Basically we want to find out which category is more likely, given attribute 1 and attribute 2 values.
基本上,我们希望找出给定属性1和属性2值的可能性更大的类别。
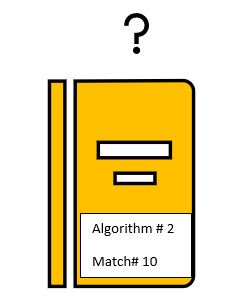
从计数到概率: (Moving from count to Probability:)
This count-based approach works fine for a small number of categories and a small number of words. The same intuition is followed more elegantly using conditional probability.
这种基于计数的方法适用于少量类别和少量单词。 使用条件概率可以更优雅地遵循相同的直觉。

Conditional Probability is again best understood with an example
再举一个例子可以更好地理解条件概率
Let’s assume
假设
Event A: The face value is odd | Event B: The face value is less than 4
事件A:面值是奇数| 事件B:面值小于4
P(A) = 3/6 (Favourable cases 1,3,5 Total Cases 1,2,3,4,5,6) similarly P(B) is also 3/6 (Favourable cases 1,2,3 Total Cases 1,2,3,4,5,6). An example of conditional probability is what is the probability of getting an odd number (A)given the number is less than 4(B). For finding this first we find the intersection of events A and B and then we divide by the number of cases in case B. More formally this is given by the equation
P(A)= 3/6(有利案件1,3,5总案件1,2,3,4,5,6)类似,P(B)也是3/6(有利案件1,2,3总案件) 1,2,3,4,5,6)。 条件概率的一个示例是给定奇数(A)小于4(B)的概率是多少。 为了找到这一点,我们首先找到事件A和B的交集,然后除以案例B中的案例数。更正式地说,由等式给出
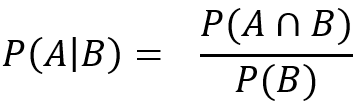
P(A|B) is the conditional probability and is read as the probability of A Given B. This equation forms the central tenet. Let’s now go back again to our book category problem, we want to find the category of the book more formally.
P(A | B)是条件概率,并被解读为A给定B的概率。该等式形成了中心原则。 现在让我们再回到书籍类别问题,我们希望更正式地找到书籍的类别。
朴素贝叶斯分类器的条件概率 (Conditional Probability to Naive Bayes Classifier)
Let’s use the following notation Book=ML is Event A, book=Sports is Event B, and “Attribute 1 = 2 and Attribute 2 = 10” is Event C. The event C is a joint event and we will come to this in a short while.
让我们使用以下表示法Book = ML是事件A,book = Sports是事件B,“属性1 = 2和属性2 = 10”是事件C。事件C是联合事件,我们将在一会儿。
Hence the problem becomes like this we calculate P(A|C) and P(B|C). Let’s say the first one has a value 0.01 and the second one 0.05. Then our conclusion will be the book belongs to the second class. This is a Bayesian Classifier, naive Bayes assumes the attributes are independent. Hence:
因此,问题变得像这样,我们计算P(A | C)和P(B | C)。 假设第一个值为0.01,第二个值为0.05。 那么我们的结论将是该书属于第二类。 这是贝叶斯分类器, 朴素贝叶斯假定属性是独立的。 因此:
P(Attribute 1 = 2 and Attribute 2 = 10) = P(Attribute 1 = 2) * P(Attribute = 10). Let’s call these conditions as x1 and x2 respectively.
P(属性1 = 2和属性2 = 10)= P(属性1 = 2)* P(属性= 10)。 我们将这些条件分别称为x1和x2。
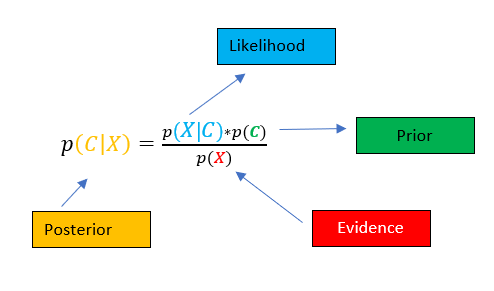
Hence, using the likelihood and Prior we calculate the Posterior Probability. And then we assume that the attributes are independent hence likelihood is expanded as
因此,使用似然和先验,我们计算后验概率。 然后我们假设属性是独立的,因此可能性随着

The above equation is shown for two attributes, however, can be extended for more. So for our specific scenario, the equation get’s changed to the following. It is shown only for Book=’ML’, it will be done similarly for Book =’Sports’.
上面的公式显示了两个属性,但是可以扩展更多。 因此,对于我们的特定情况,方程式get更改为以下形式。 仅在Book ='ML'中显示,对于Book ='Sports'也将类似地显示。
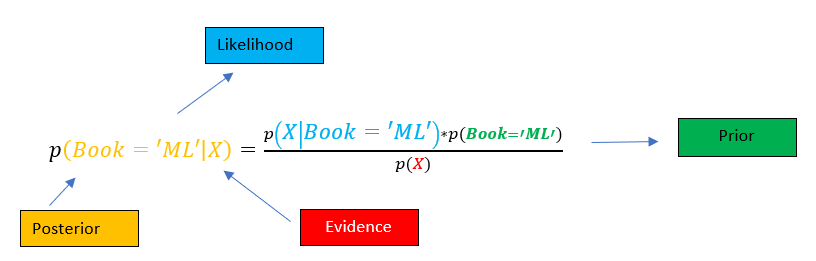
实现方式: (Implementation:)
Let’s use the famous Flu dataset for naive Bayes and import it, you can change the path. You can download the data from here.
让我们将著名的Flu数据集用于朴素贝叶斯并将其导入,即可更改路径。 您可以从此处下载数据。
Importing Data:
汇入资料:
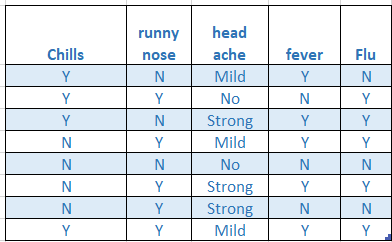
nbflu=pd.read_csv('/kaggle/input/naivebayes.csv')
Encoding the Data:
编码数据:
We store the columns in different variables and encode the same
我们将列存储在不同的变量中并进行相同的编码
# Collecting the Variables
x1= nbflu.iloc[:,0]
x2= nbflu.iloc[:,1]
x3= nbflu.iloc[:,2]
x4= nbflu.iloc[:,3]
y=nbflu.iloc[:,4]# Encoding the categorical variables
le = preprocessing.LabelEncoder()
x1= le.fit_transform(x1)
x2= le.fit_transform(x2)
x3= le.fit_transform(x3)
x4= le.fit_transform(x4)
y=le.fit_transform(y)# Getting the Encoded in Data Frame
X = pd.DataFrame(list(zip(x1,x2,x3,x4)))
Fitting the Model:
拟合模型:
In this step, we are going to first train the model, then predict for a patient
在这一步中,我们将首先训练模型,然后为患者预测
model = CategoricalNB()# Train the model using the training sets
model.fit(X,y)#Predict Output#['Y','N','Mild','Y']
predicted = model.predict([[1,0,0,1]])
print("Predicted Value:",model.predict([[1,0,0,1]]))
print(model.predict_proba([[1,0,0,1]]))
Output:
输出:
Predicted Value: [1]
[[0.30509228 0.69490772]]
The output tells the probability of not Flu is 0.31 and Flu is 0.69, hence the conclusion will be Flu.
输出表明非Flu的概率为0.31,Flu为0.69,因此结论为Flu。
Conclusion:
结论 :
Naive Bayes works very well as a baseline classifier, it’s fast, can work on less number of training examples, can work on noisy data. One of the challenges is it assumes the attributes to be independent.
朴素贝叶斯(Naive Bayes)作为基线分类器的效果非常好,速度很快,可以处理较少数量的训练示例,可以处理嘈杂的数据。 挑战之一是它假定属性是独立的。
Reference:
参考:
[1] Wu X, Kumar V, editors. The top ten algorithms in data mining. CRC Press; 2009 Apr 9.
[1] Wu X,Kumar V,编辑。 数据挖掘中的十大算法。 CRC出版社; 2009年4月9日。
[2] https://towardsdatascience.com/all-about-naive-bayes-8e13cef044cf
[2] https://towardsdatascience.com/all-about-naive-bayes-8e13cef044cf
翻译自: https://towardsdatascience.com/a-short-tutorial-on-naive-bayes-classification-with-implementation-2f69183d8ce1
朴素贝叶斯实现分类
本文来自互联网用户投稿,该文观点仅代表作者本人,不代表本站立场。本站仅提供信息存储空间服务,不拥有所有权,不承担相关法律责任。如若转载,请注明出处:http://www.mzph.cn/news/391022.shtml
如若内容造成侵权/违法违规/事实不符,请联系多彩编程网进行投诉反馈email:809451989@qq.com,一经查实,立即删除!