机器人的动力学和动力学联系
Happiness is something we all aspire to, yet its key factors are still unclear.
幸福是我们所有人都渴望的东西,但其关键因素仍不清楚。
Some would argue that wealth is the most important condition as it determines one’s living conditions. Nevertheless, some would rightly point out that rich people aren’t systematically happy. On the contrary, one’s social relationships (family, friends, etc.) contribute just as much to his happiness. Finally, some would argue that the policies implemented by governments are what really determine one’s happiness, as they determine indirectly our living conditions and social interactions.
有人会说, 财富是最重要的条件,因为它决定着一个人的生活条件。 不过,有些人会正确地指出,有钱人并不系统地感到高兴。 相反,一个人的社会关系 (家庭,朋友等)对他的幸福同样重要。 最后,有人会说,政府实施的政策真正决定一个人的幸福,因为它们间接地决定了我们的生活条件和社会互动。
These are all general and intuitive ideas about happiness, that we can easily apprehend using our own experience. However, I believe that a data-driven approach would allow for a deeper understanding of happiness drivers. By applying the most advanced techniques of Machine Learning, it would be possible to define the most important factors and measure quantitatively their contribution to one’s happiness.
这些都是关于幸福的一般而直观的想法,我们可以利用自己的经验轻松地理解它们。 但是,我认为,数据驱动的方法可以使人们对幸福驱动力有更深的了解。 通过应用最先进的机器学习技术,可以定义最重要的因素,并定量地衡量它们对一个人的幸福的贡献。
In this context, I analyzed data from The World Happiness Report which is a landmark survey of the state of global happiness. It contains, on the one hand, happiness scores based on the answers gathered from the Gallup World Poll (GWP) and on the other hand, indicators of economic production, social support, life expectancy, freedom, absence of corruption, and generosity.
在这种情况下,我分析了《世界幸福报告》中的数据, 该报告是全球幸福状况的里程碑式调查。 它一方面包含基于盖洛普世界民意测验 (GWP)收集的答案的幸福分数,另一方面包含经济生产,社会支持,预期寿命,自由,没有腐败和慷慨的指标。
This article is the second article of a series, where I share the key findings of the in-depth study I conducted on the subject. In my previous article, I analyzed happiness from a purely descriptive point of view. I discussed thus the complex relationships between happiness and three essential elements: wealth, social relations, and public policy. This data exploratory analysis lifted the veil on the difference that exists between the regions of the world over time.
本文是该系列文章的第二篇,在这里我分享了我对该主题进行的深入研究的主要发现。 在上一篇文章中 ,我从纯粹描述性的角度分析了幸福。 因此,我讨论了幸福与三个基本要素之间的复杂关系:财富,社会关系和公共政策。 这项数据探索性分析揭开了世界各地之间随时间推移而存在的差异的面纱。
However, it is purely descriptive. It tells us nothing about the most crucial questions: what really brings happiness, to what extent, and why? This article intends to answer these questions by focusing on the causal relationship between happiness and the other variables, using statistical tools and Machine Learning.
但是,它纯粹是描述性的。 它没有告诉我们最关键的问题: 什么真正地带来了幸福,程度如何,为什么? 本文旨在通过使用统计工具和机器学习关注幸福感与其他变量之间的因果关系来回答这些问题。
In this perspective, I relied on two approaches: one ‘traditional’ approach commonly used in the field of economics and a new approach based on the state of the art Machine Learning interpretation tools.
从这个角度来看,我依靠两种方法:一种是经济学领域常用的“传统”方法,另一种是基于最新的机器学习解释工具的新方法。
Without more ado, let’s dive into the micro and macro-econometric of happiness!
事不宜迟,让我们深入了解幸福的微观和宏观计量!
Structure:
结构体:
About the Data
关于数据
‘Classical’ Approach
“古典”方法
Machine Learning Approach
机器学习方法
Limitations
局限性
1.关于数据 (1. About the Data)
Here a quick recap of the variables of the World Happiness Report dataset. For more information about the data, you can refer to my previous article here.
这里是世界幸福报告数据集变量的快速回顾。 有关数据的更多信息,您可以在此处参考我的上一篇文章。
Economic metrics:
经济指标:
GDP per capita: in terms of Purchasing Power Parity (PPP) adjusted to constant 2011 international dollars.
人均GDP:根据购买力平价(PPP)调整为2011年不变美元。
Healthy life expectancy at birth.
出生时的健康预期寿命 。
Happiness metrics:
幸福指标:
Happiness indicators mainly come from the Gallup World Poll (GWP), which continually surveys residents of different countries using randomly selected, nationally representative samples. These indicators are the following:
幸福感指标主要来自G allup世界民意测验(GWP), 继续使用随机选择的具有国家代表性的样本对不同国家的居民进行调查。 这些指标如下:
Cantril Scale, or Life Ladder: it consists of asking respondents to rate their lives on a “ladder” scale ranging from 0 to 10, where 0 means the worst possible life.
Cantril量表或生命阶梯 :它包括要求受访者以0到10的“阶梯”量表对他们的生活进行评分,其中0表示可能的最坏生活。
Positive affect: it is the average of previous-day affect measures for happiness, laughter, and enjoyment.
正面影响 :这是前一天对幸福,笑声和享受的平均影响程度。
Negative affect: it represents the average of previous-day affect measures for worry, sadness, and anger.
负面影响 :它表示前一天担心,悲伤和愤怒的平均影响程度。
Social indicators:
社会指标:
Social indicators also come from GWP surveys. They include:
社会指标也来自全球升温潜能值调查。 它们包括:
Social support: it represents the national average of the binary responses (either 0 or 1) to the question “If you were in trouble, do you have relatives or friends you can count on to help you whenever you need them, or not?”
社会支持 :它代表对问题“如果有麻烦,您是否有亲戚或朋友可以依靠,无论何时需要,都可以帮助您”的二元答复的全国平均值(0或1) 。
Generosity: it is the residual of regressing the national average of GWP responses to the question “Have you donated money to a charity in the past month?” on GDP per capita.
慷慨大方 :对“您在过去一个月是否向慈善机构捐款了吗?”这一问题,对全球升温潜能值React的全国平均值进行了回归。 人均GDP。
Freedom to make life choices: it represents the national average of binary responses to the question “Are you satisfied or dissatisfied with your freedom to choose what you do with your life?”
做出生活选择的自由 :它代表对“ 您是否对选择自己的生活做选择的自由感到满意或不满意 ”的二进制答案的全国平均水平。
Perceptions of corruption: it represents the national average of binary answers to two questions: “Is corruption widespread throughout the government or not?” and “Is corruption widespread within businesses or not?”
对腐败的看法 :它代表对以下两个问题的全国平均答案: “腐败是否在整个政府中广泛存在?” 和“ 腐败在企业内部是否普遍存在? ”
2.“经典”方法 (2. ‘Classical’ Approach)
2.1。 选择变量 (2.1. Selection of Variables)
The first question I had to address is: what variables to keep for the modeling of happiness? In order words, what variables really matter in explaining Happiness?
我必须要解决的第一个问题是:为幸福建模留哪些变量? 顺便说一句,什么变量在解释幸福方面真正重要?
For this purpose, I performed a Principal Component Analysis (PCA). This method extracts the most important information from data by constructing a smaller number of representative variables that collectively explain most of the variability in the original set.
为此,我进行了主成分分析 (PCA)。 该方法通过构造较少数量的代表变量来共同从原始数据集中解释大多数变量,从而从数据中提取最重要的信息。
The new variables, called Principal components, are directions in feature space along which the original data is highly variable. Therefore, by analyzing their composition, it is possible to determine the variables to keep for the study.
新的变量称为P主成分,是特征空间中原始数据高度可变的方向。 因此,通过分析其组成,可以确定要保留用于研究的变量。
For more information about PCA, you can read this article.
有关PCA的更多信息,您可以阅读本文 。
The graph below shows the percentage of variance explains by each principal component. The two first ones explain most of the variance.
下图显示了每个主成分说明的差异百分比。 前两个解释了大部分差异。

The two graphs below analyses the constitution of the principal components and their correlation to the original variables.
下面的两个图形分析了主要成分的构成及其与原始变量的相关性。


The graphs strongly suggest that there are 6 key variables to keep in the modeling: GDP per capita, Social support, Healthy life expectancy at birth, Freedom to make life choices, Generosity, and Perception of corruption. Taken together, these six variables explain most of the variation in national annual average ladder scores among countries.
图表强烈暗示了模型中应保留6个关键变量: 人均GDP,社会支持,出生时的健康预期寿命,自由选择生活,慷慨大方和腐败感。 综上所述,这六个变量解释了各国之间国家年度平均阶梯得分的大部分差异。
Furthermore, it is possible to visualize how strongly each variable influences the two first principal components in a biplot. This graph also shows the correlation between variables through the angles between their representative vectors: the lower the angle between is the more the variables are positively correlated.
此外,可能的可视化每个变量如何强烈地影响在一个双标图两个第一主成分。 此图还通过变量代表矢量之间的角度显示了变量之间的相关性:角度越小,变量之间的正相关性就越高。
Therefore, Life Ladder, GDP per capita, Healthy life expectancy at birth, and Social Support explains most of the first principal component and are strongly correlated to each other.
因此, 生活阶梯 , 人均GDP ,出生时的健康预期寿命和社会支持解释了大多数第一主要要素,并且它们之间具有很强的相关性。

2.2。 幸福的模型 (2.2. Modeling of Happiness)
Correlations are useful to get quick insights about data. But, things can get tricky when it comes to identifying whether the correlation between two or more variables represents a causal relationship.
关联对于快速了解数据很有用。 但是,要确定两个或多个变量之间的相关性是否代表因果关系,事情可能会变得棘手。
Let’s take a famous example. In 2012, Messerli published a paper where he pointed out the high correlation between a country’s level of chocolate consumption and its population’s cognitive function. Does it mean that eating more chocolate would make you smatter? Unfortunately, probably not! For more information about causality, you can read this article or watch the video below.
让我们举一个著名的例子。 2012年,梅塞利(Messerli)发表了一篇论文 ,指出了一个国家的巧克力消费水平与其人口的认知功能之间的高度相关性。 这是否意味着多吃巧克力会使您感到不舒服? 不幸的是,可能不是! 有关因果关系的更多信息,您可以阅读本文或观看下面的视频。
演示地址
And yet, establishing causal relationships is crucial in decision making. For instance, countries with a high Healthy life expectancy at birth tend to be happier on average. Does it mean that ensuring a good healthcare system would increase people happiness? It depends as healthy people can still badly evaluate their life.
然而,建立因果关系对于决策至关重要。 例如, 出生时健康预期寿命高的国家通常会更快乐。 这是否意味着确保良好的医疗体系会增加人们的幸福感? 这取决于健康的人仍然无法正确评估他们的生活。
Therefore, to answer these kinds of questions, it is necessary to use statistical tools, such as regression analysis. Regressions are often used by economists. They enable them not only to quantify the relationship between one variable and the other variables but also its relevance relationships through statistical tests.
因此,要回答此类问题,必须使用统计工具,例如回归分析。 经济学家经常使用回归。 它们使他们不仅可以量化一个变量与其他变量之间的关系,还可以通过统计检验量化其相关性。
方法 (Methodology)
When performing a regression, it is important to control, on the one hand, for variables that are constant across countries but vary over time. For example, economic crises, such as the subprime crisis in 2008, had an impact on people's happiness globally.
进行回归分析时,一方面,重要的是要控制各个国家之间恒定但随时间变化的变量。 例如,经济危机(例如2008年的次贷危机)对全球人们的幸福感产生了影响。
On the other hand, it is essential to control for time-invariant unobserved individual characteristics, such as the country weather. These controls enable us to really capture the causal relationships between the explanatory variables and happiness.
另一方面,必须控制随时间变化的未观察到的个人特征,例如乡村天气。 这些控制使我们能够真正掌握解释变量与幸福之间的因果关系。
To do so, I relied on the well-known technique of fixed effects. More specifically, I considered 3 settings depending on the fixed effects included in the regression.
为此,我依靠众所周知的固定效果技术。 更具体地说,我根据回归中包括的固定效果考虑了3种设置 。
- Year effects 年效应
- Year and Regional effects 年份和区域影响
- Year and Country effects 年和国家影响
This approach is similar to the one carried out in The World Happiness Report, except the fact that I rescaled the variable through a min-max normalization. This explains the difference in the results.
这种方法与《世界幸福报告》中执行的方法类似,不同之处在于我通过最小-最大规格化对变量进行了重新缩放。 这解释了结果的差异。
结果 (Results)

Unsurprisingly, all the variables are significant under the 3 scenarios. More precisely:
毫不奇怪,在这三种情况下,所有变量都是显着的。 更确切地说:
Log GDP per capita is consistently the most important factor with a coefficient value ranging from 0.29 to 0.99.
对数人均GDP一直是最重要的因素,系数值在0.29到0.99之间。
Social support comes in the first position in the first scenario and the second position in the two others, with a coefficient value between 0.21 and 0.29.
社会支持在第一种情况下排在第一位,在其他两种情况下排在第二位,系数值在0.21和0.29之间。
Healthy life expectancy at birth is only significantly different from 0 when considering the variance in the first scenario. It signs is even negative in the third scenario. This suggests that the results of the second scenario are the most reliable.
当考虑第一种情况的方差时,出生时的健康预期寿命仅与0显着不同。 在第三种情况下,这种迹象甚至是负面的。 这表明第二种情况的结果是最可靠的。
Generosity and Perception of corruption are relatively less important and their variance is high.
慷慨大方和腐败感相对不太重要,并且差异很大。
3.机器学习方法 (3. Machine Learning Approach)
Over the past decade, the field of Machine Learning has known an important success. It has been applied to many areas and has proven to be very efficient, often outperforming usual statistical methods.
在过去的十年中, 机器学习领域取得了重要的成功。 它已应用于许多领域,并被证明是非常有效的,通常优于常规的统计方法。
However, research first has focused on developing the most performant algorithms in terms of accuracy of prediction or classification. It is only in recent years that it has shifted towards the need for establishing causal interference. Today, it is a great source of enthusiasm within the community of data scientists.
但是,研究首先集中在开发预测或分类的准确性方面性能最高的算法。 只是在最近几年,它才转向建立因果干扰的需要。 如今,它已成为数据科学家社区中热情的重要来源。
In this context, I decided to apply the state-of-the-art interpretation techniques used in Machine Learning to get a better understanding of happiness drivers.
在这种情况下,我决定应用机器学习中使用的最新解释技术,以更好地了解幸福驱动因素。
方法 (Methodology)
To do so, I proceeded in 4 steps:
为此,我分四个步骤进行:
a. Modeling of the national average happiness: I tested different Machine Learning algorithms on the dataset and fine-tuned their hyperparameters. b. Comparison of the relative importance of variables: I computed the relative importance of each variable using the best model.c. Analysis of the marginal impact of the variables on happiness: Go further by analyzing the partial dependence of explanatory variables on happiness.d. Analysis of happiness dynamics by region: Refine the analysis by taking a closer look at the region level.
一个。 全国平均幸福度建模:我在数据集上测试了不同的机器学习算法,并对它们的超参数进行了微调。 b。 变量相对重要性的比较:我使用最佳模型计算了每个变量的相对重要性。 C。 分析变量对幸福的边际影响:通过分析解释变量对幸福的部分依赖性,进一步分析。 d。 按区域分析幸福动态:通过仔细研究区域级别来完善分析。
The explanatory variables include the 6 key indicators (GDP per capita, Social support, Healthy life expectancy at birth, Freedom to make life choices, Generosity, and Perception of corruption) as well as year and regional fixed effect. These variables are rescaling via a max-min normalization as done previously.
解释性变量包括6个关键指标( 人均GDP,社会支持,出生时的健康预期寿命,自由选择生活,慷慨大方和腐败感) 以及年度和区域固定效应。 如前所述,这些变量通过最大-最小归一化进行缩放。
I used the following Python libraries: scikit-learn and skater.
我使用了以下Python库: scikit-learn和skater 。
结果 (Results)
a. Modeling of the national average happiness
一个。 全国平均幸福度建模
I applied the most frequently used Machine Learning algorithms to predict Life Ladder based on the explanatory variables. To compare their performance in terms of prediction, I performed a 5-fold cross-validation and compared the mean Root Mean Square Error (RMSE).
我根据解释变量应用了最常用的机器学习算法来预测“ 人生阶梯” 。 为了比较它们在预测方面的性能,我进行了5次交叉验证,并比较了均方根误差(RMSE) 。
The results represented in the graph below suggest that tree-based methods exhibit better results. More especially, Extra Trees algorithm presents the lowest RMSE.
下图所示的结果表明基于树的方法显示出更好的结果。 更特别地, Extra Trees算法呈现最低的RMSE 。
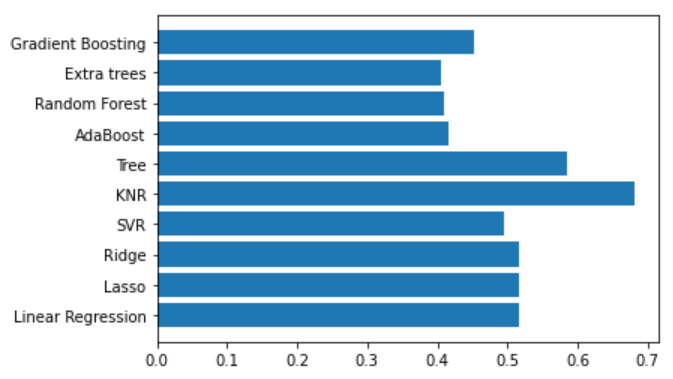
b. Comparison of the relative importance of variables
b。 变量相对重要性的比较
Extra-Trees is a well-known algorithm that trains multiple randomized decision trees and combines them by averaging their results. The graph briefly explains the underlying principle and the step of this algorithm.
Extra-Trees是一种众所周知的算法,它训练多个随机决策树并通过对它们的结果取平均来组合它们。 该图简要说明了该算法的基本原理和步骤。
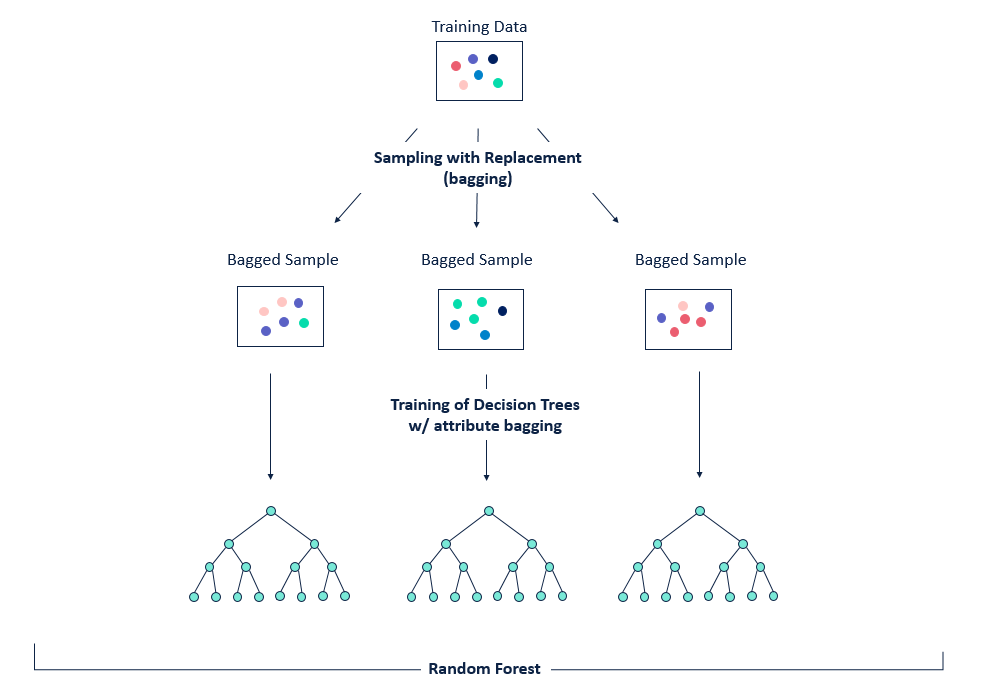
More information about forests of randomized trees is provided in sckit-learn documentation, here.
关于随机树木的森林中提供更多信息sckit学习文档, 在这里 。
Extra Trees model, as a tree-based model, provides an interpretation of its results through the importance of features. These are computed as the decrease in the impurity of each node where the feature is present, weighted by the probability of reaching this node.
额外的树木模型,基于树的模型,通过提供功能的重要性及其结果的解释。 将这些值计算为存在该特征的每个节点的杂质减少量,并按到达该节点的概率进行加权。
The graph below represents the feature importance of the variables as given by the model. Log GDP per capita, Social support and Healthy life expectancy at birth appears to be the most determinant factor of Life Ladder. This is not the case of the other models, namely Random Forest, AdaBoost and Gradient Boosting. These algorithms place Healthy life expectancy at birth before Log GDP per capita and Social support.
下图显示了模型给出的变量的特征重要性。 原木人均GDP , 社会支持和出生时健康的预期寿命似乎是决定人生阶梯的最重要因素。 其他模型( 随机森林,AdaBoost和梯度增强)则不是这种情况。 这些算法将出生时的健康预期寿命放在 人均GDP和社会支持之前。

c. Analysis of the marginal impact of the variables on happiness
C。 变量对幸福的边际影响分析
It is also possible to visualize the marginal impact of each of these 3 variables on the model predictions by plotting partial dependencies. The graphs below show a slight upward trend. This means that an increase of one variable among Log GDP per capita, Social support and Healthy life expectancy at birth, while holding other variables in the model constant, leads to an increase of happiness.
通过绘制部分相关性,还可以可视化这三个变量中的每一个对模型预测的边际影响。 下图显示了轻微的上升趋势。 这意味着,在人均GDP不变, 社会支持和健康出生时预期寿命之间增加一个变量 模型常量中的其他变量会导致幸福感增加。

The analysis can be taken one step further by visualizing the marginal impact of two variables on the model predictions. The 3D graph below is consistent with what we could have expected: the higher one’s wealth and social support are, the happier he is!
通过可视化两个变量对模型预测的边际影响,可以进一步进行分析。 下面的3D图与我们可以预期的一致:财富和社会支持越高,他就越快乐!

On the whole, the analysis conducted so far not only confirms the insights we have seen by manipulating the data but also allows us to quantify them. Money and health with the social support of our loved ones are the pillars of happiness. They are the factors that drive people to evaluate their lives positively.
总体而言,到目前为止进行的分析不仅可以通过处理数据来确认我们已经看到的见解,还可以量化这些见解。 金钱和健康在我们亲人的社会支持下是幸福的Struts。 它们是驱使人们积极评价自己生活的因素。
Nevertheless, these observations are made on a worldwide scale. We still need to explore happiness dynamics from a country perspective to make new discoveries.
然而,这些观察是在全球范围内进行的。 我们仍然需要从国家的角度探索幸福的动态,以发现新的发现。
d。 按地区分析幸福动态 (d. Analysis of happiness dynamics by region)
The analysis becomes even more interesting when considering the country level by geographical region. In particular, there is a significant gap between developed countries, emerging countries, and developing countries. These countries do not value things in the same way. Let’s take a look!
当按地理区域考虑国家/地区级别时,分析变得更加有趣。 特别是, 发达国家,新兴国家和发展中国家之间存在巨大差距。 这些国家不以相同的方式重视事物。 让我们来看看!
North America
北美
The results for North American countries are the most surprising and radically different from the rest: Log GDP per capita lags behind other factors. Its importance barely represents half of the importance of Freedom to make life choices. Healthy life expectancy at birth, Social support, and Perception of corruption come after with a relatively similar importance in the model prediction of happiness.
北美国家的结果是最令人惊讶的,并且与其他国家截然不同: 人均原木GDP落后于其他因素。 它的重要性仅代表自由做出生活选择的重要性的一半。 随之而来的是出生时的健康预期寿命,社会支持和腐败感 在幸福的模型预测中具有相对相似的重要性。

The partial dependence graphs of Log GDP per capita, Social support and Healthy life expectancy at birth show a growing trend towards flattening. This suggests that after a certain point, an increase in their value does not lead to a significant increase in happiness.
人均GDP的对数,社会支持和出生时的健康预期寿命的偏倚图显示出趋于扁平化的趋势。 这表明,在一定程度上,他们的价值增加并不会导致幸福感的显着增加。
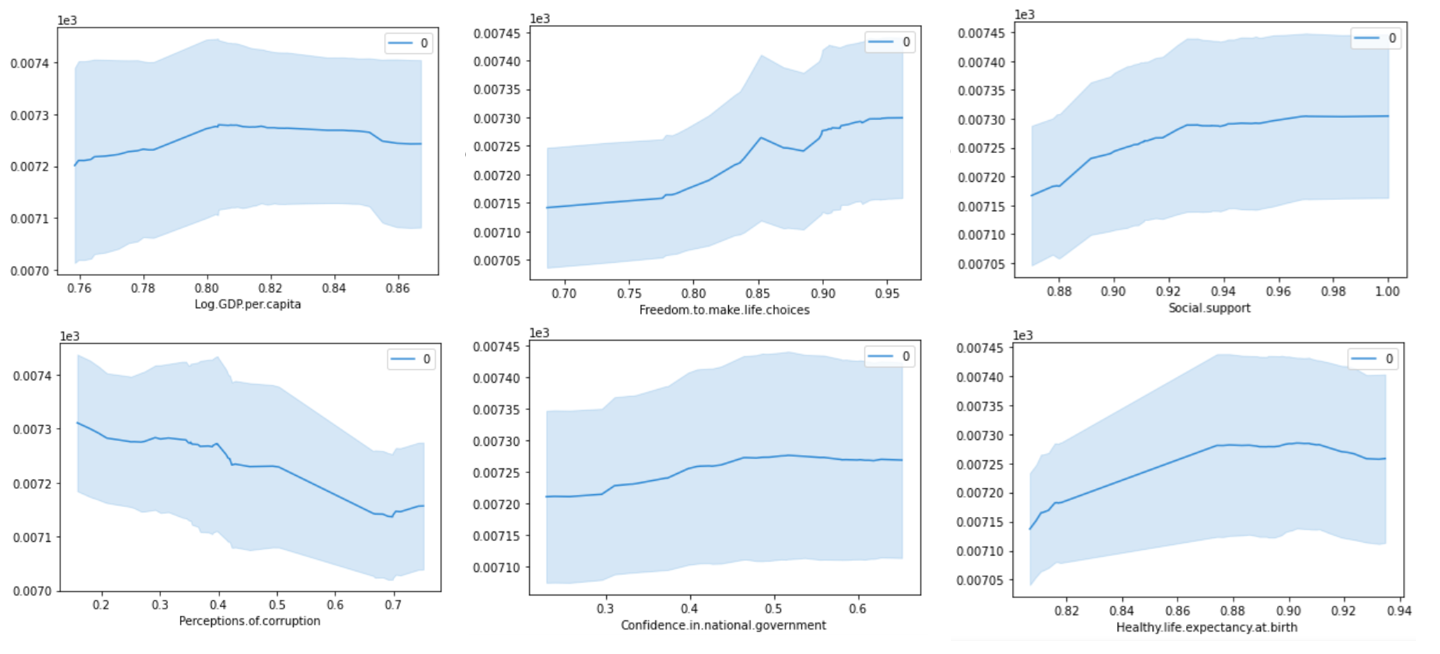
For Western European countries, Log GDP per capita and Perceptions of corruption play a similar role in determining people happiness. Again, Freedom to make life choices comes in the third position, just before Social support. On the contrary, Healthy life expectancy at birth lags behind other variables.
对于西欧国家来说, 人均GDP的对数和对腐败的看法在确定人们的幸福感方面起着相似的作用。 再者, 做出生活选择的自由排在第三位,就在社会支持之前。 相反, 出生时的健康预期寿命落后于其他变量。

As for North American countries, the partial dependence graph of Log GDP per capita shows a growing trend towards flattening which suggests that after a certain point, an increase of wealth does not lead to a significant increase of happiness.
对于北美国家而言, 人均GDP的对数偏倚关系图显示出趋于扁平化的趋势,这表明在一定点之后,财富的增加并不会导致幸福感的显着增加。
For emerging and developing countries, Log GDP per capita is, consistently and by far, the most important factor. The variable that comes next depends on the region, usually Social support or Healthy life expectancy at birth. In the case of South Asian countries, Social support is almost as important as Log GDP per capita.
对于新兴国家和发展中国家, 人均GDP一直是迄今为止的最重要因素。 接下来的变量取决于地区,通常是社会支持或出生时的健康预期寿命。 在南亚国家, 社会支持几乎与人均GDP一样重要。

Even more surprisingly is the partial dependence on the Log GDP per capita which has a bell shape. This would suggest that, after a certain level of wealth, an increase in one’s wealth would cause a degradation of his happiness!
更令人惊讶的是,部分依赖于人均GDP呈钟形。 这表明,在一定水平的财富之后,一个人的财富增加会导致他的幸福感下降!

Social support is also the second most important variable of countries in Central and Eastern Europe, Sub-Saharan Africa, Commonwealth of Independent States, but not always with the same relative proportion, as shown in the graphs below.
社会支持也是中欧,东欧,撒哈拉以南非洲,独立国家联合体国家的第二重要变量,但并非总是相对比例相同,如下图所示。


On the contrary, countries in the Latin America and Caribean, Middle East and North Africa East, East and Southeast Asia ‘value’ more Healthy life expectancy at birth than Social support, as suggested in the graph below.
相反,如下图所示,拉丁美洲和加勒比海地区,中东和北非地区的东,东和东南亚国家“重视” 出生时的健康预期寿命而不是社会支持 。



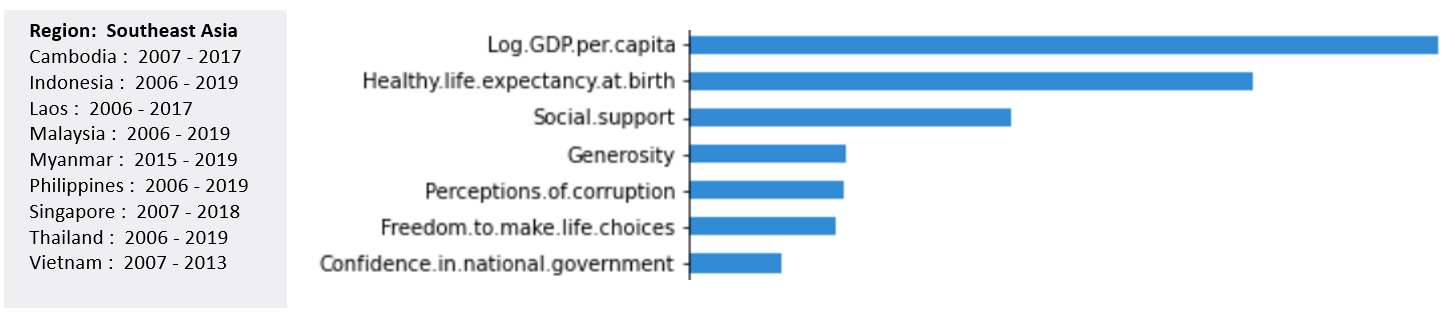
How to explain these differences between countries?
如何解释国家之间的这些差异?
Some might say it’s all about countries' culture and values but actually it is a tricky question that needs a deeper study. Nevertheless, the analysis undertaken already gives us an idea of what people in a country value the most and what policies should be introduced to improve their living conditions.
有人可能会说这全都与国家的文化和价值观有关,但实际上这是一个棘手的问题,需要深入研究。 尽管如此,进行的分析已经使我们了解了一个国家的人们最看重什么,应该采取什么政策来改善他们的生活条件。
局限性 (Limitations)
Finally, I would like to point out 3 main limitations of the approaches presented above:
最后,我想指出上述方法的三个主要局限性:
Omitted variables: Although intuitively relevant given the topic, the variables used to explain happiness might not be enough. Their correlation might actually reflect some other “unobserved” factor that is not included in the analysis.
省略的变量:尽管给定主题在直观上相关,但用于解释幸福的变量可能还不够。 它们之间的相关性实际上可能反映了分析中未包括的其他“不可观察”因素。
Reverse causality: There is also a risk of bidirectional causality. This means that happiness can cause changes in one or more explanatory variables. For instance, are people happy because they are healthy, or is their sentiment of happiness that makes them feel healthy? Are people happy because they are wealthy or is the feeling of happiness that makes them more dynamic and ambitious in their professional lives?
反向因果关系:还存在双向因果关系的风险。 这意味着幸福会导致一个或多个解释变量发生变化。 例如,人们是因为健康而快乐,还是使自己感到健康的幸福感? 人们是因为自己有钱而幸福,还是因为幸福感使他们的职业生活更加充满活力和野心?
Mismeasurement: Many variables analyzed are subjective and represent more people perceptions than reality. For example, people from a country with a long political tradition of democracy might feel corruption more strongly while it remains weaker and less widespread than in other countries. Moreover, choosing GDP per capita and Healthy life expectancy at birth to represent respectively people wealth and health.
错误计量:分析的许多变量都是主观的,代表的是人们对现实的认识多于现实。 例如,来自一个有着悠久民主政治传统的国家的人们可能会比其他国家更强烈地感受到腐败,而它仍然比其他国家更弱小和普遍。 此外,选择人均GDP和出生时的健康预期寿命分别代表人民的财富和健康。
翻译自: https://towardsdatascience.com/understanding-happiness-dynamics-with-machine-learning-part-2-4df36e52486
机器人的动力学和动力学联系
本文来自互联网用户投稿,该文观点仅代表作者本人,不代表本站立场。本站仅提供信息存储空间服务,不拥有所有权,不承担相关法律责任。如若转载,请注明出处:http://www.mzph.cn/news/391011.shtml
如若内容造成侵权/违法违规/事实不符,请联系多彩编程网进行投诉反馈email:809451989@qq.com,一经查实,立即删除!