现实世界 机器学习
In my previous posts I covered analytical subjects from a scientific point of view, rather than an applied real world problem. For this reason, this article aims at approaching an analytical idea from a managerial point of view, rather than going through the hard math. Let’s start with the “why questions”.
在我以前的文章中,我从科学的角度介绍了分析主题,而不是实际的现实问题。 出于这个原因,本文旨在从管理的观点出发,而不是通过严格的数学方法来分析一种想法。 让我们从“为什么问题”开始。
While some executives feared employees will not be as efficient as they are at the office, others were not quite convinced that processes, precisely planned and designed for on site work, could also be followed from home. Well, these concerns turned out to be wrong. As the crisis around Covid-19 has proved it, working from home is feasible even on a large scale and will likely be the new normal.
尽管一些高管担心员工的效率不如办公室,但另一些高管则不太相信为现场工作精心计划和设计的流程也可以在家中进行。 好吧,这些担心被证明是错误的。 正如围绕Covid-19的危机所证明的那样,即使大规模进行在家工作也是可行的,并且很可能成为新的常态 。
Many may have returned to their previous working environment, but still communication within the company has changed sustainably — do not expect this to return to the pre-Covid-time! We could observe that communicating about the virus and its impact on all of us, is essential in order to “create clarity, build resilience and catalyse positive change” among the employees. To be more concrete, sound communication is not only a task related to Corona virus, but an ongoing necessity to assure the companies’ success — and no, I am not only talking about the top-down company news.
许多人可能已经回到了以前的工作环境,但是公司内部的沟通仍然发生了可持续的变化-不要指望这种情况能回到Covid之前的时代! 我们可以观察到,就员工之间的“ 创建清晰性,增强适应力和促进积极变化 ”而言,就该病毒及其对我们所有人的影响进行交流至关重要。 更具体地说,声音交流不仅是与Corona病毒有关的任务,而且是确保公司成功的持续必要条件-不,我不仅在谈论自上而下的公司新闻。
This all might sound a bit esoteric, so let me address the following agenda in more detail — we will even cover a breeze of unsupervised machine learning to get the most of our data 🚀 — this is going to be real fun!
Ť 他的一切听起来有点深奥,所以让我更详细地讨论了以下议程-我们甚至将覆盖无监督机器的微风学习,以获得我们的大多数数据🚀 -这将是真正的乐趣!
This article will be split into two sections:
本文将分为两部分:
Rationale: Why is analysing communication data so important?
理由:为什么分析通讯数据如此重要?
How-To: Means to analyse communcation data?
方法:分析通讯数据的方法?
The second part is covered through a quantitative approach that does some basic data exploration based on Microsoft Office 365 data.
第二部分通过定量方法进行介绍,该方法基于Microsoft Office 365数据进行一些基本数据探究。
基本原理 (Rationale)
First things first, let’s throw around a couple more buzz words. Digital Transformation and Big Data are not new and have been floating around for a couple of years now. Triggered by new ways of analysing data, the strong industry pressure to move towards a more digital business model as well as the need to gain and retain a competitive edge, these buzz words seem to remain inherent to sustainable corporate success.
首先,让我们再说几个时髦的词。 数字转型和大数据并不是什么新鲜事物,并且已经流行了两年。 在新的数据分析方法,行业向更数字化的商业模式转变的强大压力以及获得和保持竞争优势的需求的推动下,这些时髦的话似乎仍然是可持续企业成功所固有的。
For this reason we will have a closer look at the results of previous studies and surveys carried out by several of the most industry acknowledged consulting companies.
因此,我们将更仔细地研究由一些最受业界认可的咨询公司进行的先前研究和调查的结果。
What is communication related data?
什么是与通信相关的数据?
Communication data is data that captures interaction between individuals or groups, that can be e.g. graphs (directed and undirected), aggregations of data that may represent which application or service was used or simply the amount of time spent or number of messages sent by a user. There is of course no way to move further towards analysing text data or other private and person related details — remember privacy and GDPR folks!
通信数据是捕获个人或组之间的交互的数据,例如可以是图形(有向图和无向图),可以表示使用了哪个应用程序或服务的数据集合,或者只是用户花费的时间量或发送的消息数。 当然,没有办法进一步分析文本数据或其他与私人和个人相关的详细信息 - 记住隐私和GDPR人士 !
Digital Transformation
数字化转型
I asked Google what is essential to a successful digital transformation and came up with a good collections of statements that seem convincing and based on experiences rather than opinions:
我问Google对成功进行数字化转型的必要条件,并提出了一系列令人信服的陈述,这些陈述似乎是有说服力的,是基于经验而非观点的:
McKinsey: “Our research points to a set of factors that might improve the chances of a transformation succeeding covering five categories:
麦肯锡 :“我们的研究指出了一系列因素,这些因素可能会提高成功转型的机会,涉及五个方面:
having the right, digital-savvy leaders in place
聘请合适的,精通数字的领导者
building capabilities for the workforce of the future
为未来的劳动力建设能力
empowering people to work in new ways
赋予人们以新方式工作的权力
giving day-to-day tools a digital upgrade
为日常工具提供数字升级
communicating frequently via traditional and digital methods”
通过传统和数字方式进行频繁交流”
Gartner’s opinion on the necessity of Communication Leaders in the light of digital transformation:
Gartner从数字化转型的角度对传播领导者的必要性的看法:
“[..] regularly discussing the company’s digital business narrative with employees can more than double their digital dexterity. Communicators can drive this effort by leveraging their storytelling ability.”
“ [..]定期与员工讨论公司的数字业务叙述,可以使他们的数字灵活性提高一倍以上。 沟通者可以利用他们的 讲故事能力 来推动这一努力 。 ”
“Only 17% of organizations discuss narratives consistently,” says Earl. “A successful digital business narrative
“ 只有17%的组织一直在讨论叙事 ,”厄尔说。 “成功的数字业务叙事
highlights the importance of digital dexterity and
强调数字灵巧的重要性,
builds a consistent, shared understanding of what digital business transformation means and how individual employees should contribute.”
对数字业务转型的含义以及每个员工应如何贡献建立了一致的共识。”
Big Data
大数据
Communication data is data your company may already have — or could obtain easily! Deloitte evaluated types of data through assigning value and volume/complexity. We may determine internal communication data to be “Targeted data” for which:
通信数据是您的公司可能已经拥有的数据,或者可以轻松获得! 德勤通过分配值和数量/复杂性来评估数据类型 。 我们可能将内部通信数据确定为“目标数据”,其目标是:
there are few sources
资料来源很少
often difficult to collect, but
通常很难收集,但是
containing insights highly relevant to the business problem
包含 与业务问题高度相关的见解

PwC is very clear on the complexity of communication information — whether it is internal or external:
普华永道非常清楚通信信息的复杂性,无论是内部的还是外部的:
“As with the growth of email, the proliferation of other digital communications and service channels mean that whether your audience is business or consumer facing — they are undoubtedly hit with a barrage of information, much of which is ignored simply due to the sheer volume.”
“随着电子邮件的增长,其他数字通信和服务渠道的泛滥意味着您的受众是面向企业还是面向消费者–毫无疑问,他们受到大量信息的冲击,其中的许多信息由于数量庞大而被忽略 。 ”
如何? (How-To?)
Let’s proceed with the analytical part, this is where things become tangible, finally.
让我们继续分析部分 ,最后这是东西变得有形的地方。
As outlined under Deloitte’s opinion on big data, there are very few sources that allow obtaining communication data. With regard to my data source, I have used data originated from an application called OfficeExpert which allows its users to collect meta-data-like communication data within the Microsoft Office environment. More specifically, I focused on communication data gathered from Microsoft Teams (Messages to individuals and in groups) and Exchange in order to identify “Communication Leaders”.
正如德勤对大数据的看法所概述的那样,很少有资源可以获取通信数据。 关于我的数据源,我使用了来自名为OfficeExpert的应用程序的数据,该应用程序允许其用户在Microsoft Office环境中收集类似元数据的通信数据。 进一步来说, 我专注于从Microsoft团队(发给个人和小组的消息)和Exchange收集的通信数据,以便确定“通信负责人”。
First, I checked on what data was actually collected per user in order to get a bit of a feeling what further analysis I could do. Anonymous user data is visualised in different ways e.g.:
首先,我检查了每个用户实际上收集了哪些数据,以便对我可以做进一步的分析有所了解。 匿名用户数据以不同方式可视化,例如:


After having clicked through the tabs I felt that I would even be more interested in the raw data collected by the tool. In order to stay consistent with my first impression, I went through the underlying data which is the basis for the above visualisations and is can be accessed through a SQL client. With a bit of SQL understanding this works without any issues.
单击选项卡后,我感到我对该工具收集的原始数据会更加感兴趣。 为了与第一印象保持一致,我仔细研究了基础数据,这些数据是上述可视化的基础,可以通过SQL客户端进行访问。 对SQL有一点了解,这可以正常工作。
My first impression is, that there are quite a few users that are considerably more involved in communications than others. Through another functionality in the tool, I could draw a graph of users that are related to each other in terms of how intensively they communicate. A company undirected graph can be observed in the tool and looks like this (users are nodes, any type of connection is an edge):
我的第一印象是,有相当多的用户比其他用户参与通信。 通过该工具中的另一个功能, 我可以绘制一张用户图表,这些图表根据他们的交流强度而彼此相关 。 可以在工具中观察到公司无向图,它看起来像这样(用户是节点,任何类型的连接都是边):

Clearly there are very well connected users at the bottom of the visualisation, these do have a variety of connections to other users and many tiny rectangles as well. The rectangles describe channels. Microsoft Teams allows to create channels and Teams in order to orchestrate and facilitate communication to several users.
显然,在可视化的底部有连接良好的用户,这些用户与其他用户的连接确实多种多样,并且还有许多小矩形。 矩形描述通道。 Microsoft团队允许创建渠道和团队,以编排并促进与多个用户的通信。
As a data scientist you will now probably have a hard time resisting to think of this even further. Is there a way to cluster users? Naturally I would expect a group of highly active users, medium and less involved users. Addressing the Communication leaders in the company is essential — I am referring to Gartner (Digital Transformation) once again.
作为数据科学家,您现在可能会很难拒绝进一步考虑。 有没有一种群集用户的方法? 当然,我希望一群活跃的用户,中度和较少参与的用户。 与公司的沟通负责人打交道是至关重要的–我再一次指的是Gartner (数字化转型)。
For this purpose I will use a method of unsupervised learning — Kmeans clustering. The purpose of kmeans clustering is to divide our data in k segments that can be differentiated from each other.
为此,我将使用一种无监督学习的方法-Kmeans聚类。 kmeans聚类的目的是将我们的数据分为可彼此区分的k个段。
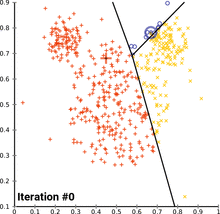
This heuristic algorithm is very straightforward, as soon as the number k cluster centers is determined, the algorithm iterates over the dataset and re-calculates the cluster centers for every iteration, hence the crucial part is to determine the number clusters the data is separated into. According to the next chart, more than two to three clusters will not provide a sufficiently large cluster size.
此启发式算法非常简单,一旦确定了k个聚类中心,该算法就会对数据集进行迭代,并针对每次迭代重新计算聚类中心,因此关键部分是确定将数据分为多少个聚类。 根据下一张图表,超过两到三个群集将无法提供足够大的群集大小。

The possible ways to analyse this data are numerous. According to the above described basic algorithm, we may already be able to identify three key users that share certain similarities in a way that allows us gather them together in a cluster. Further, there are 24 users that are quite active as well, not specified as “Communication Leader”, but still very relevant to the overall flow of information within and outside of the company.
分析此数据的可能方法很多。 根据上述基本算法,我们可能已经能够识别三个具有某些相似性的关键用户,从而使我们可以将他们聚集在一起。 此外,还有24个用户也非常活跃,没有指定为“通信主管”,但仍然与公司内部和外部的总体信息流相关。

# Clustering counts| label| user ||-----:|-----:|| 0 | 107 | # Low involvement with other users and groups| 1 | 24 | # Medium involvement with other users and groups| 2 | 3 | # Communication leaders
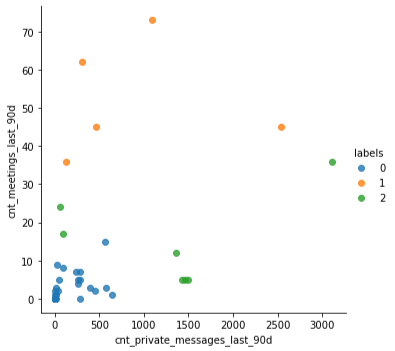
When using a variety of predictors we come to the result that there 3 clusters is a proper number to separate the data points. If we compared our final result to just a lower number of predicting dimensions, cluster sizes would be considerably larger (image to the left).
当使用各种预测变量时,我们得出的结果是,有3个聚类是适当的数目来分隔数据点。 如果我们将最终结果与较少的预测维数进行比较,则聚类大小将大得多 (左图)。
Back at the model that explained 3 extraordinary users, that showed different using patterns than the majority of other users, I could learn the following from this tiny group of users:
回到解释3个非常规用户的模型,该模型显示出与大多数其他用户不同的使用模式,我可以从这一小部分用户中学到以下知识:
- they have the highest involvement in internal channels/groups 他们对内部渠道/群体的参与度最高
- they are among the top users being responsible for channels/groups 他们是负责渠道/组的主要用户之一
- they contribute a lot of time to external communication (that could mean partners or customers) 他们为外部交流贡献了很多时间(这可能意味着合作伙伴或客户)
- The tend to have fewer direct messages, they are rather involved in the groups where they post and interact 趋向于减少直接消息,他们宁愿参与发布和交互的小组
Of course this is just the tip of the iceberg — but nonetheless, this should have given a basic idea of what could be possible and how the treasure of company owned data could be used.
当然,这只是冰山一角,但尽管如此,这应该已经给出了一个基本的思路,即可能发生的事情以及如何利用公司拥有的数据的宝藏。
Other ideas towards I could imagine heading to:
我能想到的其他想法可能会导致:
- Label users according to their position in the company. Through tweaking and fitting a classification model, it may be possible to identify users that show a behaviour that is more related to another label than actually specified (e.g. a data analyst may be even more similar to a team leader than compared to other analysts) 根据用户在公司中的职位为用户贴标签。 通过调整和拟合分类模型,有可能识别出显示出与实际指定的标签相比与另一个标签更相关的行为的用户(例如,与其他分析员相比,数据分析员与团队领导者的相似性更高)
- Can we answer the question, whether a project is likely to be more successful than another based on the team members behave? With regard to this communication topic, does communication contribute to the project’s success? 我们能否回答这个问题,根据团队成员的表现,一个项目是否可能比另一个项目更成功? 关于此交流主题,交流是否有助于项目的成功?
These are just some very generic ideas, however, no matter what we would like to investigate next, further analytics require spending more time on additional data exploration. Visualisations are a good starting point and often allow (visually) identifying essential attributes (features) with regard to the models.
这些只是一些非常通用的想法,但是,无论我们接下来要调查什么,进一步的分析都需要花费更多的时间进行额外的数据探索。 可视化是一个很好的起点,并且通常允许(视觉上)识别模型的基本属性(特征)。
As an example, a simple pair plot might help us to identify useful relationships. Although quite hard to interpret at this stage, but the large variety of different scatter plots could enable better clustering algorithms. But again, these are just starting points.
例如,简单的对图可能有助于我们识别有用的关系。 尽管在这个阶段很难解释,但是各种各样的散点图可以实现更好的聚类算法。 但是,这些仅仅是起点 。
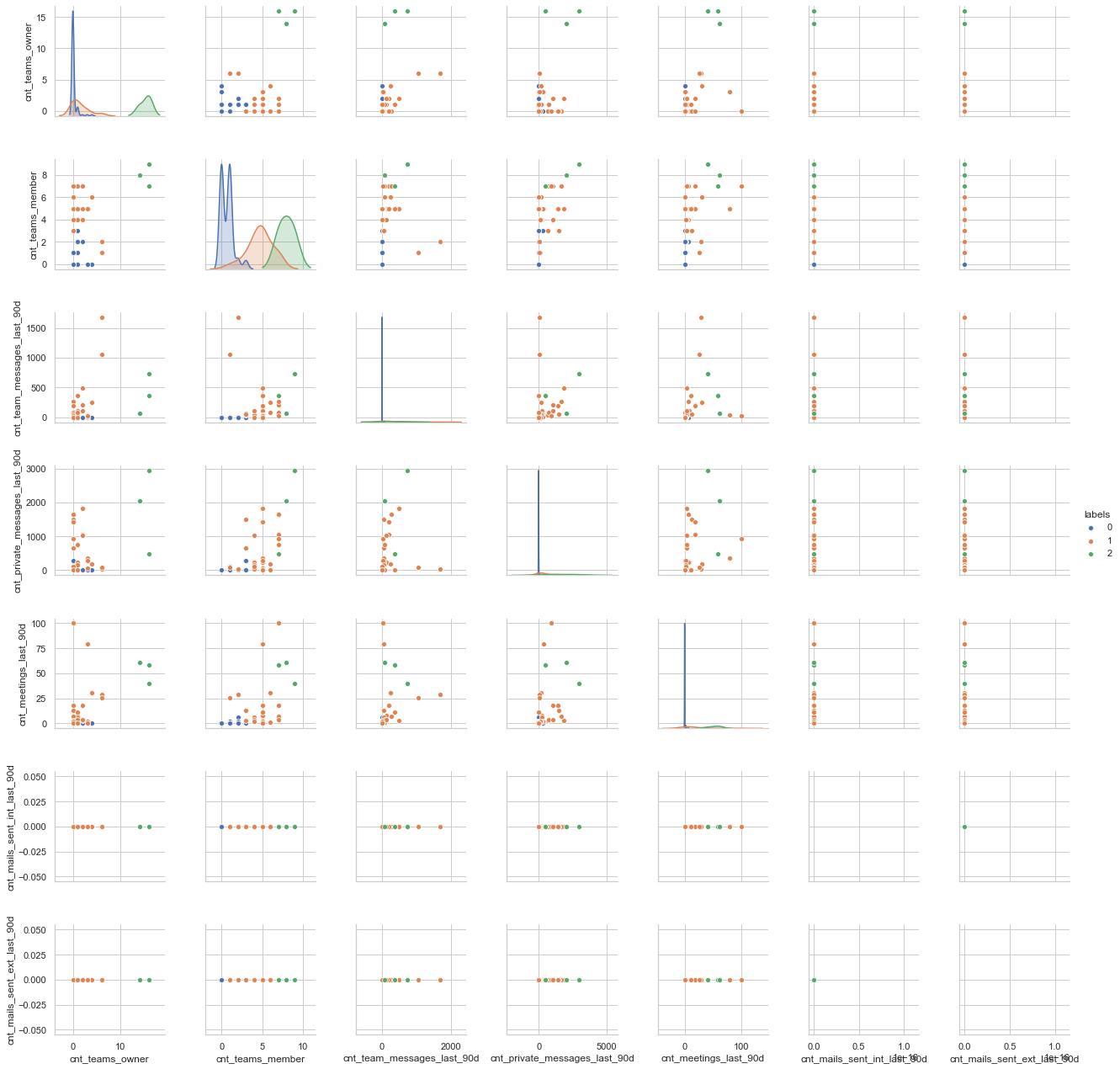
外卖 (Take aways)
There are many things we need to learn from this crisis. Especially in the corporate environment, the new normal of more frequent “working from home” days, more remote employees and the need of technical capabilities to successfully drive communication will remain and require the companies to adapt.
我们需要从这场危机中学到很多东西。 尤其是在公司环境中,新的常态将变得更加频繁,即“在家工作”的日子越来越多,远程员工越来越多,对成功推动沟通的技术能力的需求将仍然存在,并且需要公司适应 。
These changes resulting in new sources of data bear the potential to be a real gold mine for data scientists. Corporations must not oversee this opportunity and should aim at obtaining precious insights into their own structures in a way they have never considered before. Analytical tools are numerous, so it is up to the creativity of the data scientists to dive into world of corporate data.
这些变化产生了新的数据源,这有可能成为数据科学家真正的金矿。 公司绝不能忽视这个机会,而应该以从未有过的方式获取对自己的结构的宝贵见解。 分析工具多种多样,因此取决于数据科学家的创造力,以深入研究公司数据世界。
{see you next time}
{下次见}
Thanks to Volodymyr Hryshchenko for the title image and to panagenda for providing tools, visualizations and insights through OfficeExpert which allowed collecting and obtaining my dataset out of the Microsoft 365 environment.
感谢弗拉基米尔Hryshchenko为标题图像和panagenda通过OfficeExpert这使得收集和获取我的数据集了微软365环境提供工具,可视化和见解。
Charts are created through OfficeExpert and Seaborn library
通过OfficeExpert和Seaborn库创建图表
翻译自: https://towardsdatascience.com/an-introduction-to-corporate-communication-analysis-a-real-world-machine-learning-approach-bb82e5755bbc
现实世界 机器学习
本文来自互联网用户投稿,该文观点仅代表作者本人,不代表本站立场。本站仅提供信息存储空间服务,不拥有所有权,不承担相关法律责任。如若转载,请注明出处:http://www.mzph.cn/news/389946.shtml
如若内容造成侵权/违法违规/事实不符,请联系多彩编程网进行投诉反馈email:809451989@qq.com,一经查实,立即删除!