回归分析假设
The Linear Regression is the simplest non-trivial relationship. The biggest mistake one can make is to perform a regression analysis that violates one of its assumptions! So, it is important to consider these assumptions before applying regression analysis on the dataset.
线性回归是最简单的非平凡关系。 一个人可能犯的最大错误是进行违反其假设之一的回归分析! 因此,在对数据集进行回归分析之前,必须考虑这些假设。
This article focuses both on the assumptions and measures to fix them in case the dataset violates it.
本文着重于假设和纠正假设的方法,以防数据集违反假设。
Linearity: The specified model must represent a linear relationship.
线性:指定的模型必须表示线性关系。
This is the simplest assumption to deal with as it signifies that the relationship between dependent and independent variable is linear wherein independent variable is multiplied by its coefficient to obtain dependent variable.
这是要处理的最简单假设,因为它表示因变量和自变量之间的关系是线性的,其中将自变量乘以其系数即可获得因变量。
Y=β0+β1X1+…+βkXk+ε
Y =β0 +β1X1 + ... +βKXK +ε
It is quite easy to verify this assumption as plotting independent variable against dependent variable on a scatterplot gives us insights whether the pattern formed can be represented through a line or not. However, applying linear regression on data would not be appropriate if a line can’t fit the data. In the latter case, one can perform non-linear regression, logarithmic or exponential transformation on the dataset to convert it into a linear relationship.
验证这一假设非常容易,因为在散点图上绘制自变量与因变量的关系使我们洞悉所形成的模式是否可以通过线条表示。 但是,如果一条线无法拟合数据,则对数据进行线性回归将是不合适的。 在后一种情况下,可以对数据集执行非线性回归,对数或指数变换,以将其转换为线性关系。
2. No endogeneity of regressors: The independent variables shouldn’t be correlated with the error term.
2. 回归变量无内生性:自变量不应与误差项相关。
This refers to the prohibition of link between the independent variable and the error term. Mathematically, it can be expressed in the following way.
这是指禁止自变量与错误项之间的链接。 在数学上,它可以用以下方式表示。
𝜎 𝑥,𝜀 =0:∀𝑥,𝜀
𝜎 𝜀,𝜀 = 0:∀𝑥,𝜀
As we know that independent variables involved in the model are somewhat correlated. The incorrect exclusion of one or more independent variable that could be relevant for the model gives us the omitted variable bias. This excluded variable ultimately gets reflected in the error term resulting in the covariance between the independent variable and the error term as non zero.
众所周知,模型中涉及的自变量有些相关。 错误地排除可能与模型相关的一个或多个自变量会给我们省略变量偏差。 该排除的变量最终反映在误差项中,导致自变量和误差项之间的协方差为非零。
The only way to deal with this assumption is to try different variables for the model so as to ensure that relevant variables are very well conisdered in the model.
处理此假设的唯一方法是为模型尝试不同的变量,以确保在模型中很好地考虑了相关变量。
3. Normality and Homoscedasticity: The variance of the errors should be consistent across observations.
3. 正态性和同方性:误差的方差在所有观测值之间应保持一致。
This assumption states that the error term is normally distributed and an expected value (mean) is zero. It is important to note that normal distribution of the term is only required for making inferences.
该假设表明误差项为正态分布,期望值(均值)为零。 重要的是要注意,仅在进行推断时才需要该术语的正态分布。
𝜀 ~𝑁 (0,𝜎2)
𝜀〜𝑁(0,𝜎2)
As far as homoscedasticity is concerned, it simply means variance of all error terms related to independent variables is equal to each other. However, below is an example of a dataset with different variance of the error terms. The regression performed on this dataset would have a better result for smaller values of independent and dependent variables.
就同质性而言,它仅表示与自变量相关的所有误差项的方差彼此相等。 但是,以下是误差项的方差不同的数据集的示例。 对于较小的自变量和因变量,对该数据集执行的回归将具有更好的结果。
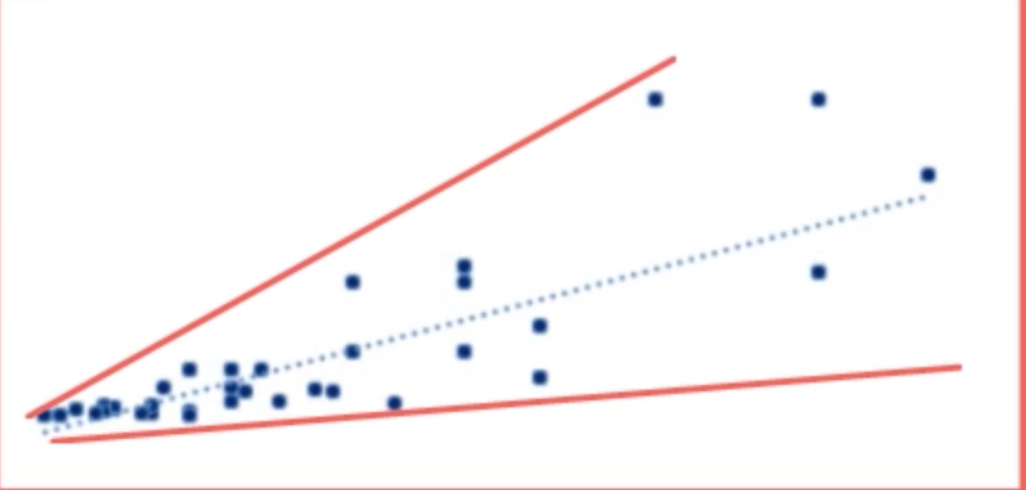
The way forward to validate this assumption is to look for omitted variable bias, outliers and perform log transformation.
验证该假设的方法是寻找遗漏的变量偏差,离群值并执行对数转换。
4. No Autocorrelation: No identifiable relationship should exist between the values of the error term
4. 无自相关:误差项的值之间不应存在可识别的关系
This assumption is the least favorite of all as it is hard to fix. Mathematically, it is represented in the following way.
该假设是所有假设中最不喜欢的,因为它很难解决。 在数学上,它以以下方式表示。
𝜎 𝜀𝑖𝜀𝑗=0:∀𝑖 ≠𝑗
𝜎 𝜀𝑖𝜀𝑗 = 0:∀𝑖≠𝑗
It is assumed that error terms are un-correlated. A common way to identify this is Durbin-Watson test which is provided in the regression summary table. If the value is less than one or more than three, it indicates autocorrelation. If the value is 2, there is no autocorrelation. It is better to avoid linear regression when there is autocorrelation.
假定误差项是不相关的。 识别此问题的常用方法是回归汇总表中提供的Durbin-Watson检验。 如果该值小于一或大于三,则表示自相关。 如果值为2,则不存在自相关。 自相关时最好避免线性回归。
5. No Multicollinearity: No predictor variable should be perfectly (or almost perfectly) explained by the other predictors.
5.没有多重共线性:其他预测变量不能完美(或几乎完美)地解释预测变量。
It is observed when two or more variables have high correlation. The logic behind this assumption is that if two variables have high collinearity, there is no point of representing both the variables in the model .
当两个或多个变量具有高相关性时可以观察到。 该假设背后的逻辑是,如果两个变量具有较高的共线性,则没有必要在模型中表示两个变量。
𝜌 𝑥𝑖𝑥𝑗 ≉1:∀𝑖,𝑗; 𝑖 ≠𝑗
≉1:∀𝑖,𝑗; 𝑖≠𝑗
It is easy to validate this assumption by dropping one of the variable or transforming them into one.
通过删除变量之一或将其转换为一个变量可以很容易地验证这一假设。
Criticisms/suggestions are really welcome 🙂.
批评/建议真的很受欢迎🙂。
翻译自: https://medium.com/swlh/simplest-guide-to-regression-analysis-assumptions-1a51d9ed69ae
回归分析假设
本文来自互联网用户投稿,该文观点仅代表作者本人,不代表本站立场。本站仅提供信息存储空间服务,不拥有所有权,不承担相关法律责任。如若转载,请注明出处:http://www.mzph.cn/news/389902.shtml
如若内容造成侵权/违法违规/事实不符,请联系多彩编程网进行投诉反馈email:809451989@qq.com,一经查实,立即删除!