ALSO, ARE RECOMMENDER SYSTEMS INFLUENCING OUR TASTE??
此外,推荐系统是否影响我们的口味?
An excerpt on creating a movie recommender system similar to the OTT platforms.
有关创建类似于OTT平台的电影推荐系统的摘录。
INTRODUCTION
介绍
Formally Defining,A Recommender System is a system that seeks to predict or filter preferences according to the user’s preferences. The demand for a good recommender system is soaring, especially with then onset of Covid-19 induced lock down,forcing everyone to stay home and watch movies of their favourite genre,actor,director….you get it right.This is where a recommender system plays an important role in providing the user, content he is more likely to watch, rather than the user searching for something that interests him,which would mess with the user experience.
正式定义,推荐系统是一种试图根据用户的偏好来预测或过滤偏好的系统。 对好的推荐器系统的需求猛增,尤其是在Covid-19引发锁定之后,迫使每个人呆在家里观看自己喜欢的类型,演员,导演的电影……您就对了。这就是推荐器的地方系统在提供用户更可能观看的内容而不是用户搜索他感兴趣的内容方面起着重要作用,而这会干扰用户体验。
The essence of a recommender system lies in its recommendation engine.There are Two types of Recommendation engine:
推荐系统的本质在于其推荐引擎。推荐引擎有两种类型:
Content-based filtering engine: It provides recommendations by matching the description of the movie and a user profile, generated by the interests provided by the user.It has an explicit understanding of the recommendation.You might have observed it in some apps,where you are asked questions about your preferences as soon as you signup.This is what it’s for.
基于内容的过滤引擎:它通过匹配电影的描述和由用户提供的兴趣产生的用户个人资料来提供推荐。它对推荐具有清晰的了解。您可能已经在某些应用中观察到了该推荐,在您注册后被问到有关您的偏好的问题。这就是它的用途。
Collaborative filtering engine: It is a method of making automatic predictions about the interests of a user by collecting preferences or taste information based on the activity of current user along with many other users with similar activity(collaborating).The underlying assumption of the collaborative filtering approach is that if a person A has the same opinion as a person B on an issue, A is more likely to have B’s opinion on a different issue than that of a randomly chosen person.It need not have any explicit understanding of the recommendation.You might have observed in one of your OTT platforms when you open a particular movie, An array of movies under the heading “people who watched this movie also watched”.This is what it uses.
协作过滤引擎:这是一种通过根据当前用户以及许多其他具有类似活动(协作)的用户的活动收集偏好或品味信息来自动预测用户兴趣的方法。方法是,如果一个人A在某个问题上与人B拥有相同的观点,那么与随机选择的人相比,A在一个不同的问题上更有可能拥有B的观点,它不需要对该建议有任何明确的理解。当您打开特定电影时,您可能已经在一个OTT平台中观察到过,标题为“看过这部电影的人也看过”的一系列电影。这就是它的用途。
Equipped with this basics,Lets dive into creating a movie recommender system using collaborative filtering.
配备了这些基础知识后,我们将深入研究使用协作过滤创建电影推荐系统。
We start by Importing required libraries. We will be using Scikit-surprise which contains the SVD(Singular Value Decomposition).SVD allows us to extract and untangle information,which is really helpful in creating a recommender system.
我们首先导入所需的库。 我们将使用包含SVD(奇异值分解)的Scikit-surprise。SVD允许我们提取和解开信息,这对于创建推荐系统非常有帮助。
This topic involves a lot of statistical data analysis.resources to know more about scikit surprise,SVD:
本主题涉及大量统计数据分析。了解更多关于scikit Surprise,SVD的资源:
First thing one must do before creating a model is observe the data. This gives us a lot of insight on the type of data it is, and what we could use to gain the maximum from it.
创建模型之前,必须做的第一件事就是观察数据。 这使我们对数据的类型以及可以用来从中获得最大收益的数据有很多了解。
As we observe the data, we see that timestamp is a redundant column and it is best to remove it.
当我们观察数据时,我们看到时间戳是多余的列,最好将其删除。
It is always a good practice to check for NaNs in your dataset,luckily we don’t have any.
最好在您的数据集中检查NaN,幸运的是我们没有。
现在是该模型的主要部分, 探索性数据分析 (Now comes the Main Part of this model, Exploratory Data Analysis)
To start,We look for the Number of movies and users in the dataset.
首先,我们在数据集中寻找电影和用户数。
Now we find Sparsity of the data. Sparsity tells us the percentage of movies missing rating by the users. i.e Not all users rate a movie, It tells us the percentage of missing values by the total values.Sparsity for this data is 98%. Usually the lower the sparsity,the better.But in the case of Collaborative Filtering, below 99% is manageable.
现在我们发现数据的稀疏性。 稀疏度告诉我们用户缺少电影评分的百分比。 即,并非所有用户都对电影进行评分,它告诉我们缺失值占总值的百分比。此数据的稀疏度为98%。 通常,稀疏度越低越好。但是在协作过滤的情况下,低于99%是可以控制的。
Sparsity(%) = (No of Missing Values/(Total Values))*100
稀疏度(%)=(遗漏值/(总值))* 100
Now we try to visualize ratings distribution.
现在,我们尝试可视化收视率分布。
Most of the ratings are between 3–5 and the range of the ratings are from 0.5 to 5.
大多数评级介于3-5之间,评级范围介于0.5到5之间。
FEATURE ENGINEERING
特征工程
Now comes The next essential part of the system, Feature Engineering.I always believe that Feature Engineering as Important as building a model, as It allows the model to better understand and converge better.
现在是系统的下一个基本部分,即要素工程。我一直认为要素工程对于构建模型同样重要,因为它可以使模型更好地理解和融合。
Here We are Reducing the Dimensions by removing the redundant data like Movies with less than 3 ratings or user who rated less than 3 movies, as it is difficult to recommend something with such less data to analyse.
在这里,我们正在通过删除冗余数据(例如评级低于3的电影或评级低于3的用户的电影)来减少尺寸,因为很难推荐具有此类数据的数据来进行分析。
Now lets start creating the Model,
现在开始创建模型,
Creating a Surprise Dataset for training using the Reader class that we imported and provide the expected scale of rating,which we found out during our exploratory data analysis.You can add that to your data using the dataset import.
使用我们导入的Reader类创建一个用于训练的Surprise Dataset,并提供我们在探索性数据分析中发现的预期的评分等级。您可以使用数据集导入将其添加到数据中。
Now as we are using our whole train set for training,we create an antiset which consists of all the data without the reviews on which we can test.
现在,当我们使用整个训练集进行训练时,我们将创建一个包含所有数据的antiset,而没有可以测试的评论。
We create our SVD, which untangles the information for us to complete the recommender model.
我们创建了SVD,它为我们整理了信息,以完成推荐模型。
We then evaluate our model with the metrics Root Mean Square Error and Mean Absolute Error as they provide the average over the epoch of the absolute values of difference between the recommendation and the actual observation.
然后,我们使用度量均方根误差和均值绝对误差来评估我们的模型,因为它们提供了建议与实际观察值之间的绝对差值的平均值。
Predicting
预测
预测为我们提供了用户ID为1的电影ID。 (The prediction gives us a movie id for user id 1.)
This finishes our recommender system’s job.
这样就完成了推荐系统的工作。
Now… lets discuss about something debatable.
现在...让我们讨论一些值得商bat的问题。
推荐系统是否正在影响我们在电影中的品味并控制我们? (Are Recommender Systems influencing our taste in movies and taking the control from us??)
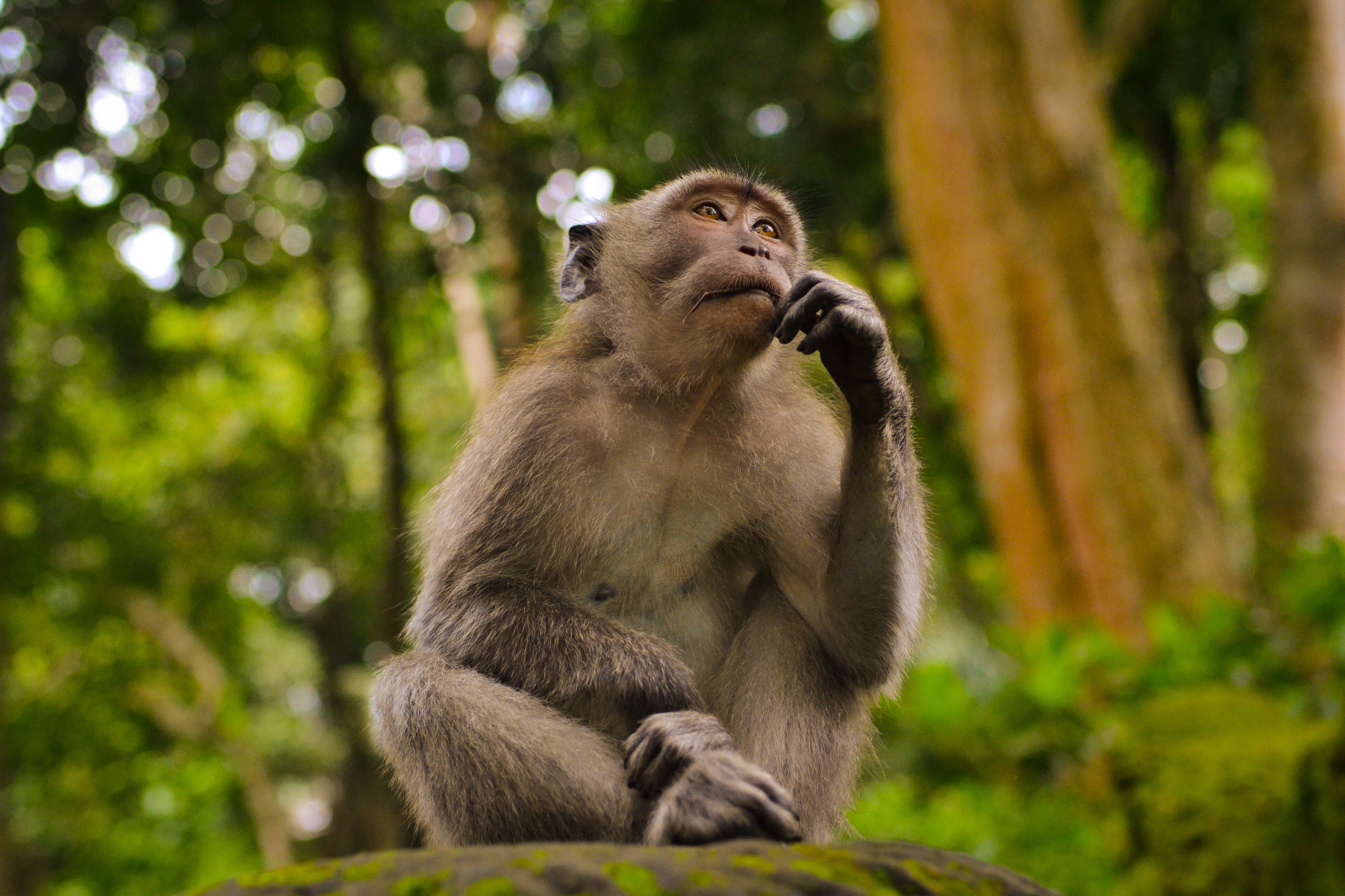
My Father who is no way related to computer Science asked me this one fine morning.He was going through his favourite video streaming service and made an observation that, He was seeing videos that are related to a few areas only. It made him feel that his choice is getting Influenced by it and was unable to come across something new.
我父亲与计算机科学毫无关系,今天上午好。我正在经历他最喜欢的视频流媒体服务,并观察到,他正在观看的视频仅涉及几个领域。 这让他感到自己的选择正在受到影响,无法遇到新的事物。
I explained this to him using my own words and understanding:
我用自己的语言和理解向他解释了这一点:
He has been watching the same videos over and over daily,Thus creating a profile that, he is interested in only in this particular topic of videos.That was the reason he was shown videos from that particular topic only.
他每天都在看相同的视频,因此创建了一个个人档案,他只对特定的视频主题感兴趣。这就是为什么他只看到该特定主题的视频。
But does it mean you have no control over it,
但这是否意味着您无法控制它,
The Answer is NO.
答案是否定的。
You still have your control, If you are not interested in a topic, but you were recommended by the engine, Just let the engine know that you are not interested. Yes, you have that option. Expand your viewing horizons for diverse content. A recommender system is there just to help you, not control you.It all finally depends on the viewer to watch or not.
您仍然可以控制自己,如果您对某个主题不感兴趣,但是引擎推荐您,只需让引擎知道您不感兴趣即可。 是的,您可以选择。 扩大您的观看范围,以获取各种内容。 推荐系统只是在帮助您而不是控制您,最终取决于观看者是否观看。
Lets share our views on this and spread some knowledge.Lets learn and grow as a community.. Because all we are left with is people,memories and knowledge.
让我们就此发表看法并传播一些知识。让我们作为一个社区学习和成长。因为我们所剩的就是人,记忆和知识。
Thank you.
谢谢。
翻译自: https://medium.com/swlh/recommending-a-movie-using-collaborative-filtering-6dab1b8f4472
本文来自互联网用户投稿,该文观点仅代表作者本人,不代表本站立场。本站仅提供信息存储空间服务,不拥有所有权,不承担相关法律责任。如若转载,请注明出处:http://www.mzph.cn/news/389669.shtml
如若内容造成侵权/违法违规/事实不符,请联系多彩编程网进行投诉反馈email:809451989@qq.com,一经查实,立即删除!