计算机科学必读书籍
Product categorization/product classification is the organization of products into their respective departments or categories. As well, a large part of the process is the design of the product taxonomy as a whole.
产品分类/产品分类是将产品组织到各自部门或类别中。 同样,整个过程的很大一部分是整个产品分类的设计。
Product categorization was initially a text classification task that analyzed the product’s title to choose the appropriate category. However, numerous methods have been developed which take into account the product title, description, images, and other available metadata. The following papers on product categorization represent essential reading in the field and offer novel approaches to product classification tasks.
产品分类最初是一个文本分类任务,用于分析产品标题以选择适当的类别。 但是,已经开发出许多方法来考虑产品标题,描述,图像和其他可用的元数据。 以下有关产品分类的论文代表了该领域的重要阅读内容,并为产品分类任务提供了新颖的方法。
1.不要分类,翻译 (1. Don’t Classify, Translate)
In this paper, researchers from the National University of Singapore and the Rakuten Institute of Technology propose and explain a novel machine translation approach to product categorization. The experiment uses the Rakuten Data Challenge and Rakuten Ichiba datasets. Their method translates or converts a product’s description into a sequence of tokens which represent a root-to-leaf path to the correct category. Using this method, they are also able to propose meaningful new paths in the taxonomy.
在本文中,新加坡国立大学和乐天技术学院的研究人员提出并解释了一种新颖的机器翻译方法来进行产品分类。 该实验使用了Rakuten Data Challenge和Rakuten Ichiba数据集。 他们的方法将产品的描述转换或转换为一系列标记,这些标记代表从根到叶的正确类别路径。 使用这种方法,他们还能够在分类法中提出有意义的新路径。
The researchers state that their method outperforms many of the existing classification algorithms commonly used in machine learning today.
研究人员指出,他们的方法优于当今机器学习中常用的许多现有分类算法。
Published/Last Updated — Dec. 14, 2018
发布/最新更新— 2018年12月14日
Authors and Contributors — Maggie Yundi Li (National University of Singapore), Stanley Kok (National University of Singapore), and Liling Tan (Rakuten Institute of Technology)
作者和撰稿人:李Mag(新加坡国立大学),斯坦利·科克(新加坡国立大学)和谭丽玲(乐天技术学院)
Read Now
现在读
2.使用神经注意模型对日本商品名称进行大规模分类 (2. Large-Scale Categorization of Japanese Product Titles Using Neural Attention Models)
The authors of this paper propose attention convolutional neural network (ACNN) models over baseline convolutional neural network (CNN) models and gradient boosted tree (GBT) classifiers. The study uses Japanese product titles taken from Rakuten Ichiba as training data. Using this data, the authors compare the performance of the three methods (ACNN, CNN, and GBT) for large-scale product categorization. While differences in accuracy can be less than 5%, even minor improvements in accuracy can result in millions of additional correct categorizations.
本文的作者提出了关注卷积神经网络(ACNN)模型,而不是基线卷积神经网络(CNN)模型和梯度提升树(GBT)分类器。 该研究使用从Rakuten Ichiba获得的日语产品标题作为培训数据。 利用这些数据,作者比较了三种方法(ACNN,CNN和GBT)用于大规模产品分类的性能。 尽管精度差异可以小于5%,但即使精度略有提高,也可以导致数百万种其他正确的分类。
Lastly, the authors explain how an ensemble of ACNN and GBT models can further minimize false categorizations.
最后,作者解释了ACNN和GBT模型的集成如何进一步减少错误分类。
Published/Last Updated — April, 2017 for EACL 2017
已发布/最新更新— 2017年4月,适用于EACL 2017
Authors and Contributors — From the Rakuten Institute of Technology: Yandi Xia, Aaron Levine, Pradipto Das Giuseppe Di Fabbrizio, Keiji Shinzato and Ankur Datta
作者和撰稿人—来自乐天技术学院:夏彦迪,亚伦·莱文,Pradipto Das Giuseppe Di Fabbrizio,京急新zato和安库·达塔
Read Now
现在读
3.地图集:电子商务服装产品分类的数据集和基准 (3. Atlas: A Dataset and Benchmark for Ecommerce Clothing Product Classification)
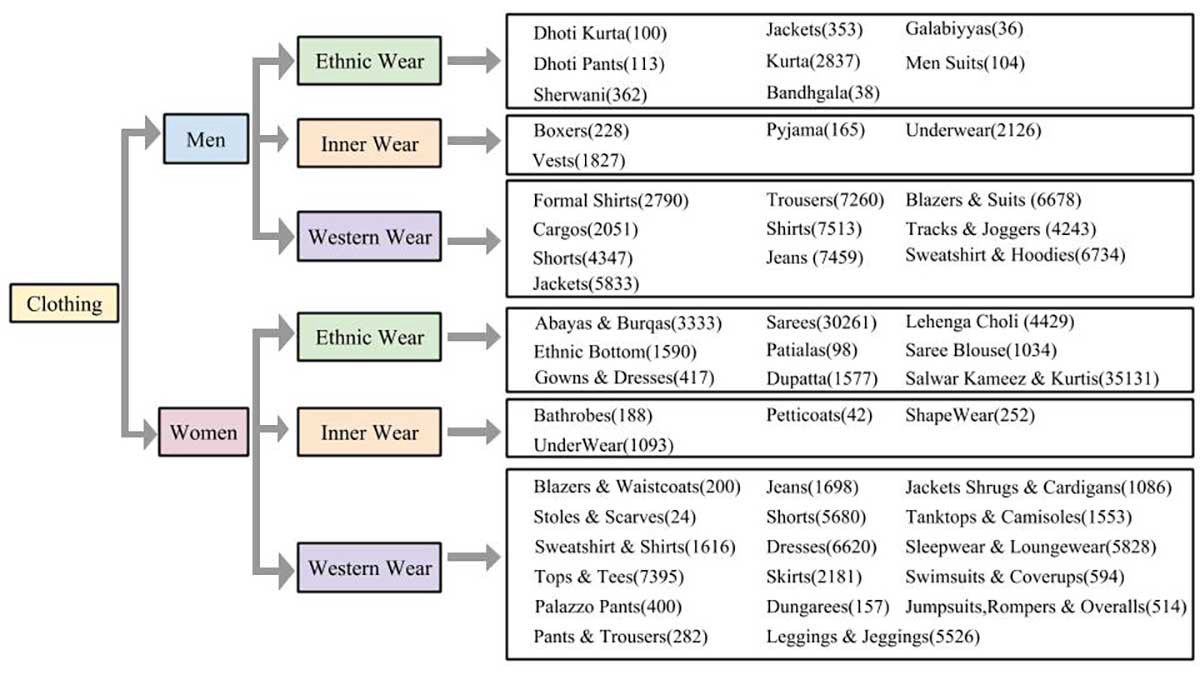
Researchers at the University of Colorado and Ericsson Research (Chennai, India) have created a large product dataset known as Atlas. In this paper, the team presents their dataset which includes over 186,000 images of clothing products along with their product titles. Furthermore, they introduce related work in the field that has influenced their study. Finally, they test their dataset using a Resnet34 classification model and a Seq to Seq model to categorize the products. The data is taken from Indian ecommerce stores, so some of the categories used may not be applicable to Western markets. However, the dataset has been open-sourced and is available on Github.
科罗拉多大学和爱立信研究公司(印度金奈)的研究人员创建了一个名为Atlas的大型产品数据集。 在本文中,研究小组展示了他们的数据集,其中包括超过186,000种服装产品的图像以及产品标题。 此外,他们介绍了影响他们的研究领域的相关工作。 最后,他们使用Resnet34分类模型和Seq to Seq模型对产品进行测试,以对产品进行分类。 数据来自印度的电子商务商店,因此使用的某些类别可能不适用于西方市场。 但是,该数据集已经开源,可以在Github上使用。
Published/Last Updated — Aug. 19, 2019
发布/最后更新— 2019年8月19日
Authors and Contributors — Venkatesh Umaashankar (Ericsson Research), Girish Shanmugam (Ericsson Research), and Aditi Prakash (University of Colorado)
作者和撰稿人— Venkatesh Umaashankar(爱立信研究中心),Girish Shanmugam(爱立信研究中心)和Aditi Prakash(科罗拉多大学)
Read Now
现在读
4.使用结构化和非结构化属性的大规模产品分类 (4. Large Scale Product Categorization using Structured and Unstructured Attributes)
In this study, a team at WalmartLabs compares hierarchical models to flat models for product categorization.
在这项研究中,沃尔玛实验室的一个团队将层次模型与平面模型进行了比较,以进行产品分类。
The researchers employ deep-learning based models which extract features from each product to create a product signature. In the paper, the researchers describe a multi-LSTM and multi-CNN based approach to this extreme classification task. Furthermore, they present a novel way to use structured attributes. The team states that their methods can be scaled to take into account any number of product attributes during categorization.
研究人员采用了基于深度学习的模型,该模型从每个产品中提取功能以创建产品签名。 在论文中,研究人员描述了一种基于多LSTM和多CNN的方法来完成这种极端分类任务。 此外,它们提供了一种使用结构化属性的新颖方法。 该团队指出,他们的方法可以扩展,以在分类过程中考虑任何数量的产品属性。
Published/Last Updated — Mar. 1, 2019
已发布/最新更新— 2019年3月1日
Authors and Contributors — From WalmartLabs: Abhinandan Krishnan and Abilash Amarthaluri
作者和贡献者—来自沃尔玛实验室:Abhinandan Krishnan和Abilash Amarthaluri
Read Now
现在读
5.使用多模式融合模型进行多标签产品分类 (5. Multi-Label Product Categorization Using Multi-Modal Fusion Models)
In this paper, researchers from New York University and U.S. Bank investigate multi-modal approaches to categorize products on Amazon. Their approach utilizes multiple classifiers trained on each type of input data from the product listings. Using a dataset of 9.4 million Amazon products, they developed a tri-modal model for product classification based on product images, titles, and descriptions. Their tri-modal late fusion model retains an F1 score of 88.2%.
在本文中,来自纽约大学和美国银行的研究人员研究了多模式方法来对亚马逊上的产品进行分类。 他们的方法利用了针对产品列表中每种输入数据类型进行训练的多个分类器。 他们使用940万个Amazon产品的数据集,开发了一种基于产品图像,标题和描述的产品分类的三峰模型。 他们的三峰后期融合模型保留了88.2%的F1分数。
The findings of their study demonstrate that increasing the number of modalities could improve performance in multi-label product categorization.
他们研究的结果表明,增加模式数量可以改善多标签产品分类的性能。
Published/Last Updated — June 30, 2019
发布/最新更新— 2019年6月30日
Authors and Contributors — Pasawee Wirojwatanakul (New York University) and Artit Wangperawong (U.S. Bank)
作者和贡献者— Pasawee Wirojwatanakul(纽约大学)和Artit Wangperawong(美国银行)
Read Now
现在读
In the papers on product categorization above, the researchers trained their models on open datasets which included millions of products. However, if you are building a product categorization model for commercial use, many open datasets may not be available to you.
在上面有关产品分类的论文中,研究人员在包含数百万种产品的开放数据集上训练了他们的模型。 但是,如果您要构建用于商业用途的产品分类模型,则可能无法使用许多开放数据集。
Looking for training data for your product classification model? Check out this training data guide and these open datasets.
寻找针对您的产品分类模型的培训数据? 查阅本培训数据指南和这些开放的数据集 。
翻译自: https://medium.com/analytics-vidhya/5-must-read-papers-on-product-categorization-for-data-scientists-19c98421cef3
计算机科学必读书籍
本文来自互联网用户投稿,该文观点仅代表作者本人,不代表本站立场。本站仅提供信息存储空间服务,不拥有所有权,不承担相关法律责任。如若转载,请注明出处:http://www.mzph.cn/news/389410.shtml
如若内容造成侵权/违法违规/事实不符,请联系多彩编程网进行投诉反馈email:809451989@qq.com,一经查实,立即删除!