vue取数据第一个数据
A lot.
很多。
I landed my first job as a Data Scientist at the beginning of August, and like any new job, there’s a lot of information to take in at once.
我于8月初找到了数据科学家的第一份工作,并且像任何新工作一样,一次有很多信息需要接受。
By documenting and sharing my own thoughts, hopefully those that are aspiring to work as a Data Scientist (or in anything data-related) can find this helpful in the future. Of course, each company and workplace is different, but I’d like to think that these tips can be useful to many people in general.
通过记录和分享我自己的想法,希望那些希望成为数据科学家(或从事与数据相关的工作)的人将来能对您有所帮助。 当然,每个公司和工作场所都是不同的,但是我想这些技巧通常对许多人有用。
遇见尽可能多的人 (Meet as many people as possible)
This applies to a lot of other roles, but I feel like this is particularly important when working with data.
这也适用于许多其他角色,但是我觉得这在处理数据时特别重要。
The more people you know, the easier it is for you to do your job.
您认识的人越多,就越容易完成工作。
There’s no better time to meet people than at the start where you have the excuse of introducing yourself. By expanding your reach within the company, there’s more potential for you to find the data that you might need for analysis in the future.
没有比在开始时介绍自己的借口更好的时间与人见面了。 通过扩大公司的业务范围,您就有更多的潜力来查找将来可能需要进行分析的数据。
This is especially true if the data is not well-managed. Even if your team has a clean and dedicated data warehouse, there’s bound to be a moment where you’ll need something but not be able to find it without the help of someone more familiar with the data than you are.
如果数据管理不当,尤其如此。 即使您的团队有干净整洁的数据仓库,也一定会有一会儿您需要一些东西,但是如果没有比您更熟悉数据的人的帮助,便无法找到它们。
定期记笔记 (Take notes regularly)
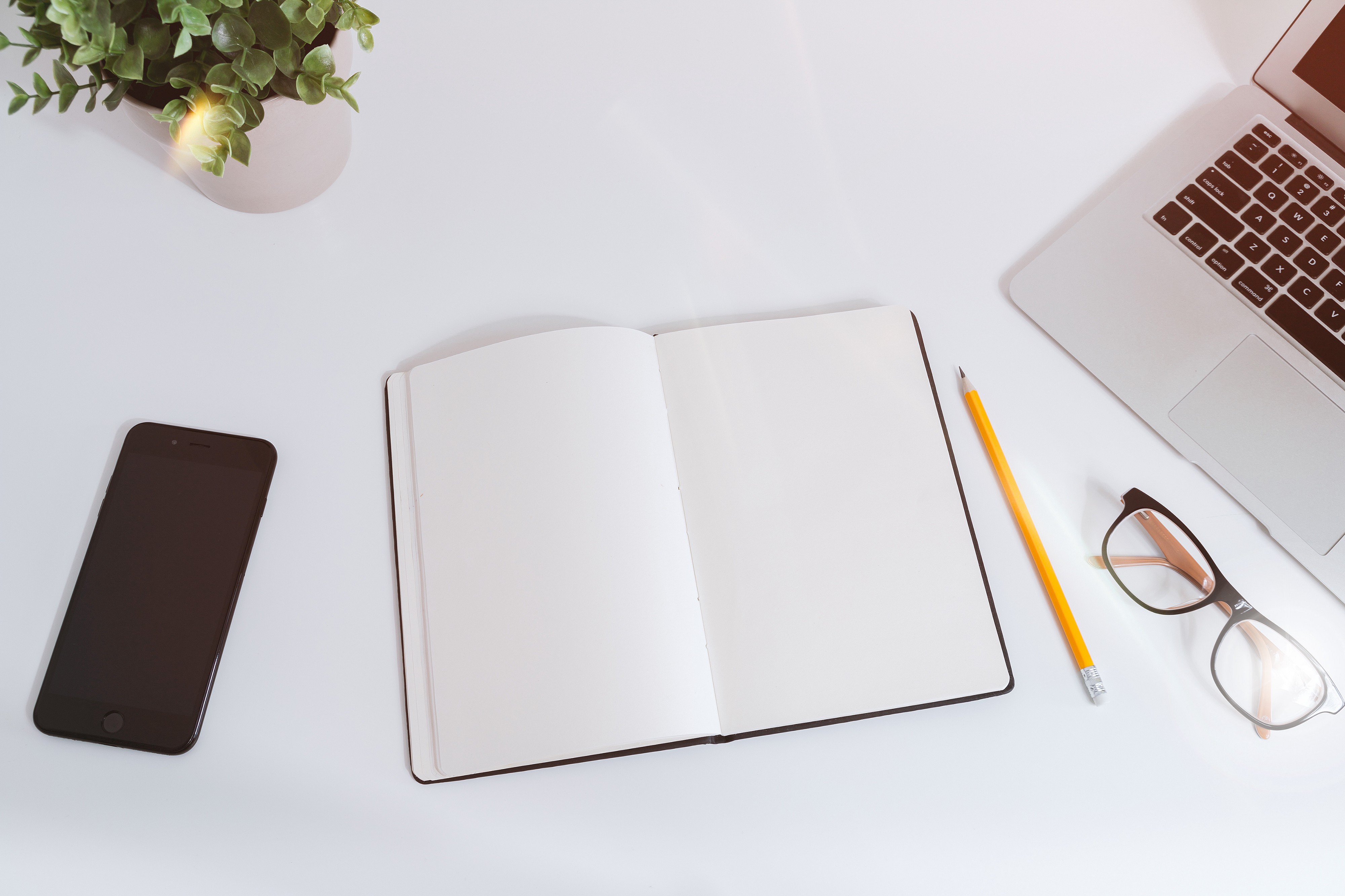
Personally, I think this is a habit that’s worth having throughout your career.
就个人而言,我认为这是一个在整个职业生涯中都值得拥有的习惯。
By regularly taking notes, you’ll have something to refer back to in the future if you forget something — and at the beginning, you will end up forgetting things.
通过定期记笔记,如果您忘记了某些内容,将来您将有一些需要参考的地方–开始时,您最终会忘记一些东西。
Developing this habit early means that you won’t have to awkwardly ask for something in the future when you know you should have remembered it by then.
早日养成这种习惯,意味着当您知道届时应该已经记住它时,您将来就不必笨拙地要求一些东西。
It’s also a good way to keep track of what people are currently doing or using (e.g. what data do they use etc.) and lets you document the location of things that might potentially be useful to you in the future.
这也是跟踪人们当前在做什么或正在使用的好方法(例如,他们使用什么数据等),并让您记录将来可能对您有用的事物的位置。
Speaking of note-taking, I’d recommend using Notion. It’s served me well during my student days for documenting my own projects and ideas, and has transitioned easily over to my working career.
说到笔记,我建议使用Notion 。 在学生时期记录自己的项目和想法对我很有帮助,并且可以轻松地过渡到我的工作生涯。
提前集思广益 (Brainstorm ideas ahead of time)
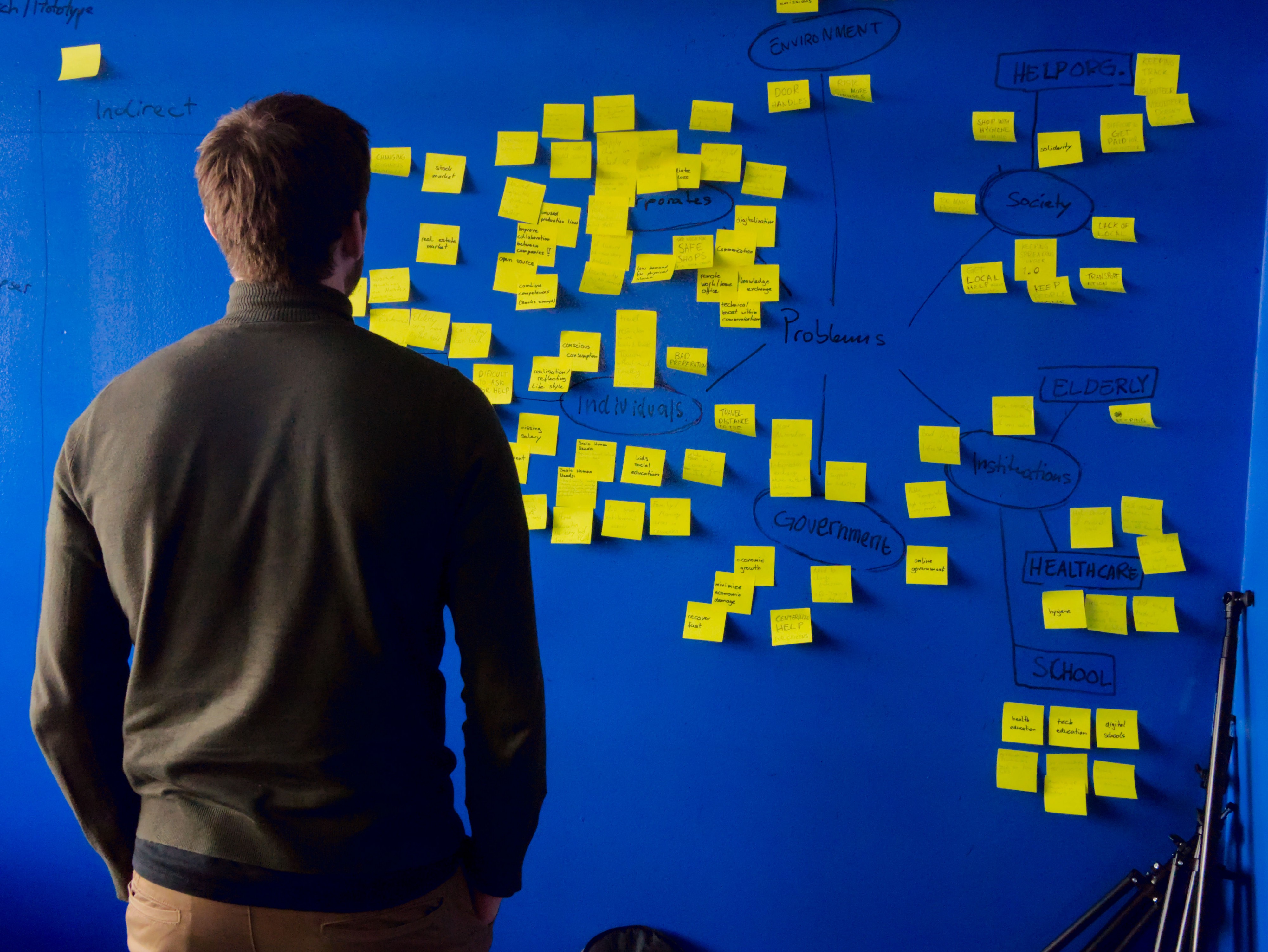
This follows on from the previous section: start jotting down ideas as you’re getting more familiar with the data — even if they might seem unreasonable for now.
这是从上一节开始的:随着对数据的熟悉程度的增加,开始记下想法,即使目前看来这些想法并不合理。
There have been times where I’ve had an idea about solving a particular problem but then forget about it later because I didn’t write it down. If you’re finally tasked to solve that same problem, you’d have to spend time coming up with the same idea again!
有时候我对解决一个特定的问题有个主意,但是后来我忘了,因为我没有写下来。 如果您最终被要求解决相同的问题,那么您将不得不花费时间再次提出相同的想法!
Documenting your ideas also lets you improve on them over time as you become more familiar with everything. When someone presents to you a new problem to solve, you might already have a good idea on how to solve it, thus making your job easier in the long run.
记录您的想法还可以使您随着时间的流逝对它们的熟悉程度不断提高。 当有人向您提出要解决的新问题时,您可能已经对如何解决有个好主意,从长远来看,这使您的工作变得更轻松。
不要过于复杂 (Don’t overcomplicate things)
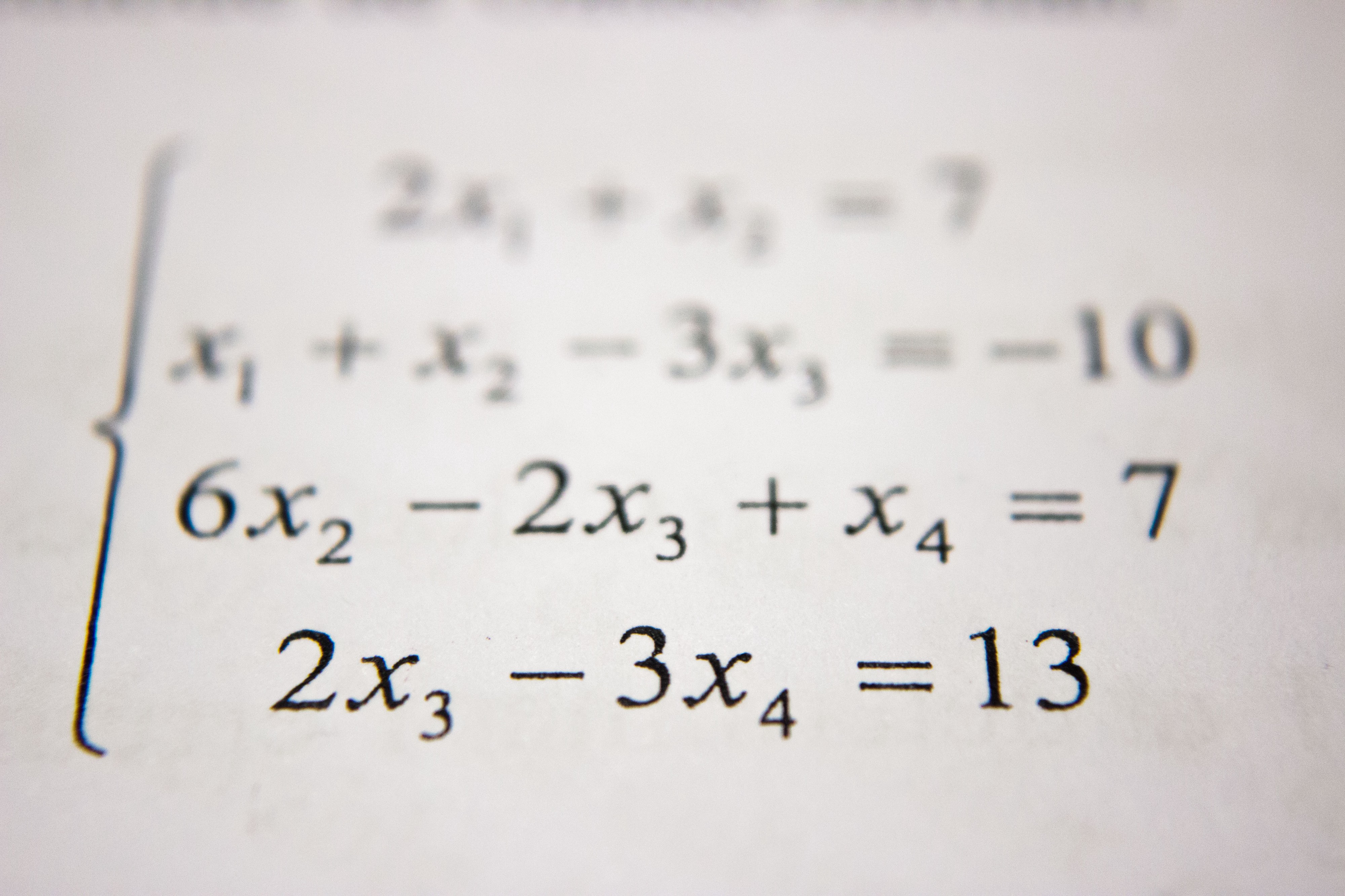
With the hype surrounding machine learning these days, it’s quite easy to fall into the trap of overcomplicating a problem that could be solved with a simple linear or logistic regression.
如今随着围绕机器学习的炒作,很容易陷入使问题复杂化的陷阱,而该问题可以通过简单的线性或逻辑回归来解决。
In some cases, the required infrastructure for a complex machine learning pipeline might not even be available.
在某些情况下,复杂的机器学习管道所需的基础架构甚至可能不可用。
Most data science problems are statistical ones that require you to think more like a statistician than a machine learning engineer.
大多数数据科学问题都是统计问题,需要您像统计学家一样思考而不是机器学习工程师。
That means starting with the usual: What does the distribution of the data look like? What sort of model would best fit this kind of distribution? And if so, does the data satisfy the statistical assumptions of the model? Do I need to remove any data if it doesn’t satisfy my assumptions? (e.g. multicollinearity).
这意味着从通常的情况开始:数据的分布是什么样的? 哪种模型最适合这种分布? 如果是这样,数据是否满足模型的统计假设? 如果数据不符合我的假设,是否需要删除? (例如多重共线性)。
From here, if it seems reasonable, a machine learning algorithm and/or pipeline could be considered. However, the more complicated the solution becomes, the harder it is to explain and justify your results to the decision makers. Try explaining how neural networks work to a non-mathematical audience, and you’ll find that it’s a very difficult thing to do.
从这里开始,如果看起来合理,则可以考虑使用机器学习算法和/或管道。 但是,解决方案越复杂,就很难向决策者解释和证明您的结果。 尝试向非数学对象解释神经网络的工作原理,您会发现这是一件非常困难的事情。
If it provides actionable insight and the evidence can be communicated clearly to the audience, then I think that’s a job well done.
如果它提供了可行的见解并且可以将证据清楚地传达给听众,那么我认为这是一项出色的工作。
不要为解决一切感到压力 (Don’t feel pressured to solve everything)
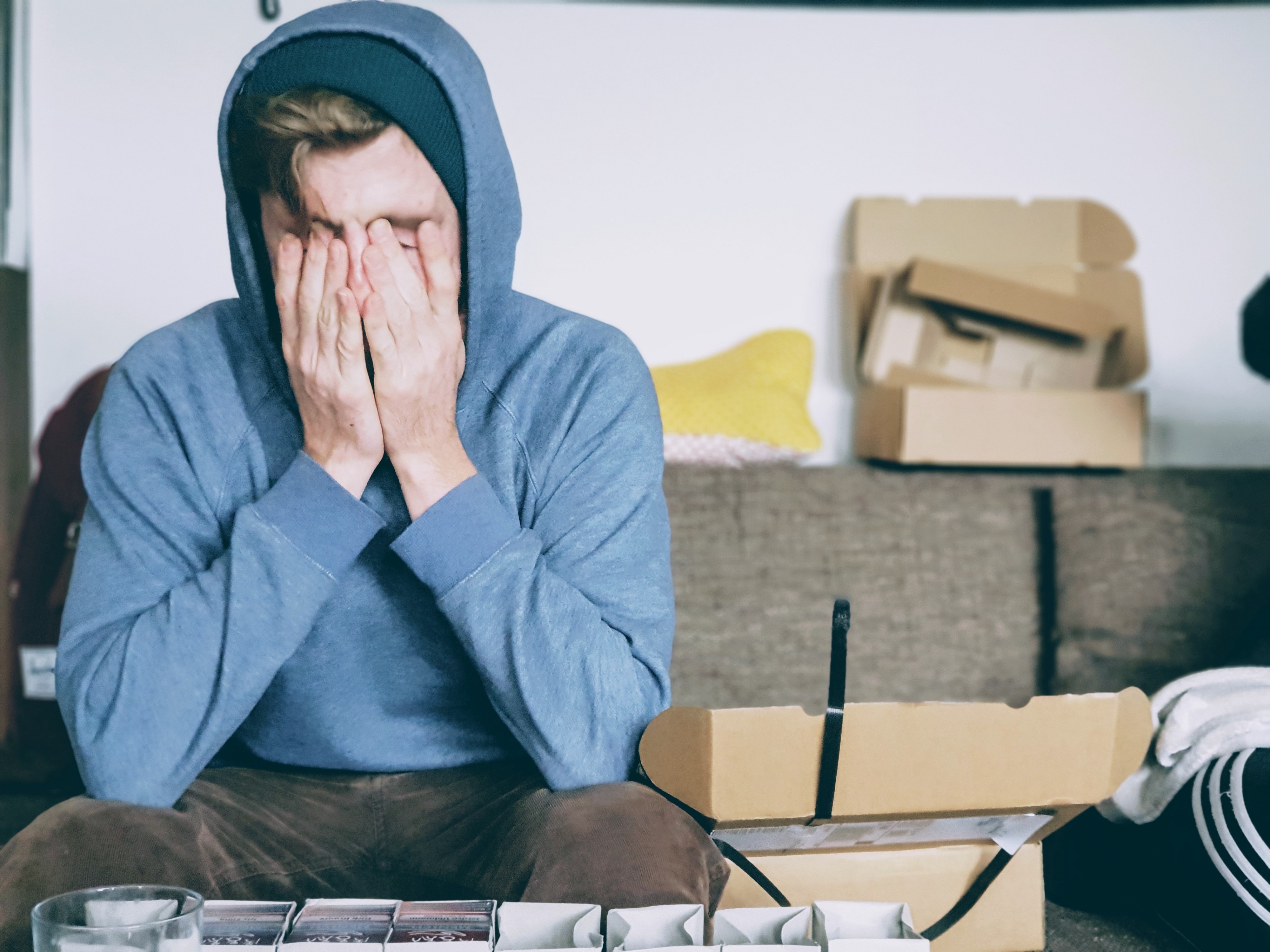
Although we’re hired to solve problems, there will always be times where it simply isn’t possible to go any further. It could be due to a lack of (usable) data, or that the solution takes too long to implement.
尽管我们被雇用来解决问题,但总有一些时候根本无法进一步解决问题。 可能是由于缺少(可用)数据,或者解决方案实施时间过长。
Whatever the reason is, it’s sometimes better to put it in the backburner and move on to something that can be solved. Most of the time, completing a single task is better than not completing any tasks at all.
不管是什么原因,有时最好将其放回炉中,然后继续进行可以解决的问题。 在大多数情况下,完成一项任务比根本不完成任何任务要好。
最后-犯错误并从中学到快乐! (And lastly — make mistakes and have fun learning!)
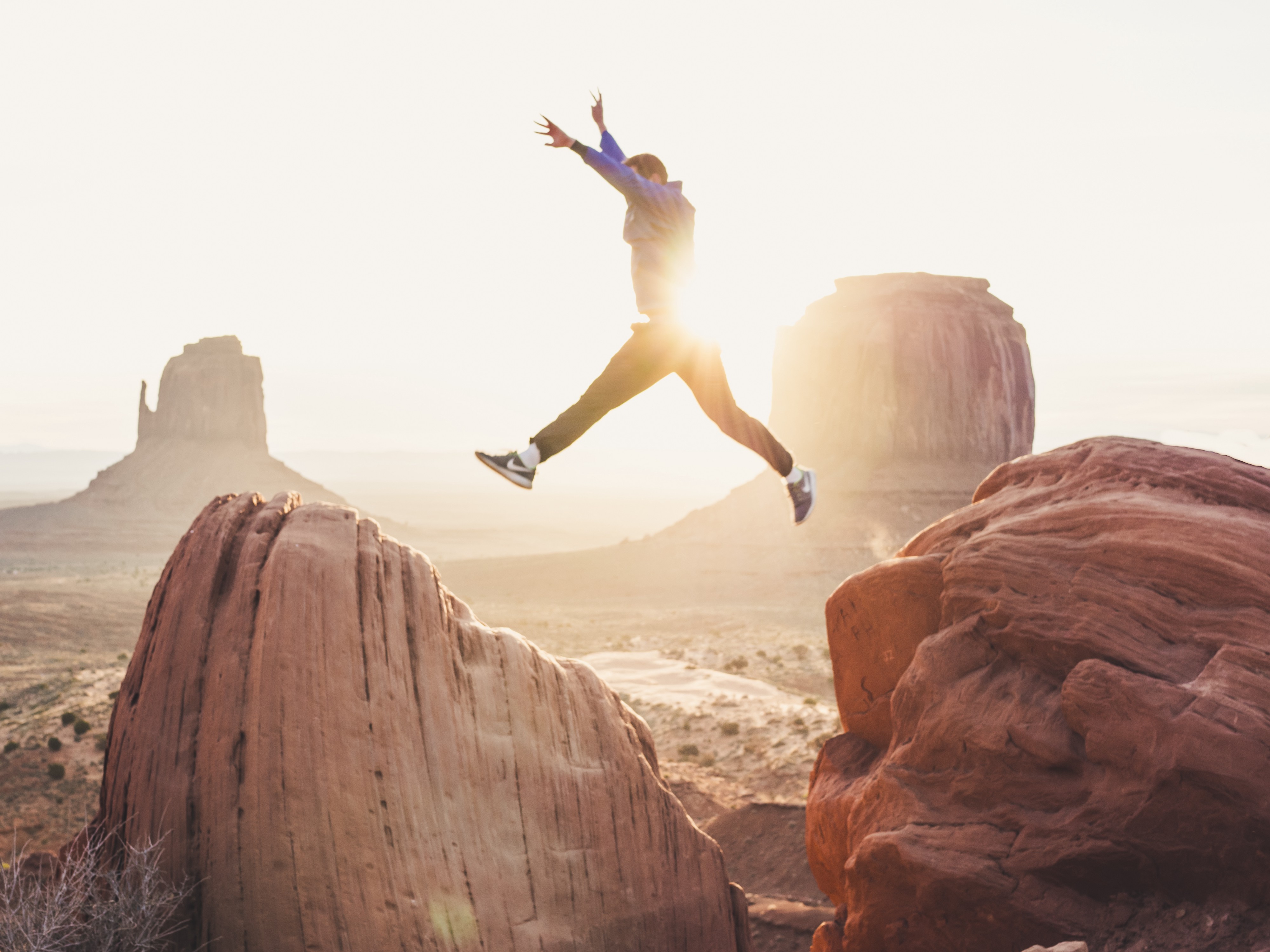
Imposter syndrome is real, and it can sometimes feel a bit overwhelming when expectations are high.
冒名顶替综合症是真实的,当期望值很高时,有时会感到有些不知所措。
Don’t be afraid to make mistakes, especially at the beginning of your career. Instead, focus on making fewer mistakes over time. It’s only natural that as you progress, fewer and fewer mistakes will be tolerated, so make the most of it at the beginning where you have an excuse to.
不要害怕犯错误,尤其是在您的职业生涯初期。 相反,应着重于随着时间的流逝减少错误。 很自然,随着您的进步,越来越少的错误会被容忍,因此在您有借口的一开始就充分利用它。
And finally —you might feel like you should know how to solve every problem and provide amazing insights at the beginning; however, now’s the perfect opportunity to learn more about the industry instead.
最后,您可能会觉得自己应该知道如何解决每个问题并在一开始就提供惊人的见解; 但是,现在是了解该行业的绝佳机会。
Take the time to explore how certain data science techniques could be applied to solving your own business problems. I’ve noticed that I’m more motivated to read and explore other potential solutions since I now have a good reason to. The biggest motivator for me though, is realising that after all these years of hard studying, I’m finally getting paid for it!
花时间探索如何将某些数据科学技术应用于解决您自己的业务问题。 我注意到,由于我现在有充分的理由,因此我更加有动力去阅读和探索其他潜在的解决方案。 但是,对我而言,最大的动力是意识到经过多年的努力学习,我终于为此获得了报酬!
翻译自: https://towardsdatascience.com/my-first-month-as-a-data-scientist-454b44aaef91
vue取数据第一个数据
本文来自互联网用户投稿,该文观点仅代表作者本人,不代表本站立场。本站仅提供信息存储空间服务,不拥有所有权,不承担相关法律责任。如若转载,请注明出处:http://www.mzph.cn/news/389341.shtml
如若内容造成侵权/违法违规/事实不符,请联系多彩编程网进行投诉反馈email:809451989@qq.com,一经查实,立即删除!