物种分布模型
Species distribution models (SDM; for review and definition see, e.g., Peterson et al., 2011) are a dominant paradigm to quantify the relationship between environmental dynamics and several manifestations of species biogeography. These statistical approaches pushed an emerging body of research describing the global distribution of species, addressing niche-based questions, supporting biodiversity conservation and ecosystem-based management, as well as infering the likely anthropogenic pressures leading to population turnover and extinction.
物种分布模型(SDM;有关审查和定义,请参见例如Peterson等,2011)是量化环境动态与物种生物地理学几种表现之间关系的主要范例。 这些统计方法推动了一个新兴的研究机构,它描述了物种的全球分布,解决了基于生态位的问题,支持生物多样性保护和基于生态系统的管理,并推断出可能导致人口流动和灭绝的人为压力。
Spatial autocorrelation (SA) is a common challenge while modelling the distribution and abundance of species. This phenomenon, likely present in most ecological datasets, denotes the situation where the values of variables sampled at nearby locations are not independent due to correlation with values at nearby locations (i.e., the value of a predictor variable at a given site can be partially predicted by the values at neighbouring sites).
在对物种的分布和丰富度进行建模时,空间自相关(SA)是一个普遍的挑战。 这种现象很可能出现在大多数生态数据集中,表示这样一种情况:由于与附近位置的值相关,因此在附近位置采样的变量的值不是独立的(即,可以对给定位置的预测变量的值进行部分预测)根据相邻站点的值)。
Accounting for SA has not received much attention in applied SDM studies, however, when present, it may result in poorly specified models and inappropriate spatial inference and prediction. Recent studies proposed to incorporate SA into the actual models while predicting distributions (coined ‘spatial models’; Dormann, 2007), however, this approach does not allow to transfer models to new independent data (e.g., temporal and spatial transferability).
在应用SDM研究中,对SA的会计处理并未引起太多关注,但是,如果存在SA会计,可能会导致指定的模型不正确以及空间推断和预测不适当。 最近的研究提出在预测分布的同时将SA合并到实际模型中(硬币化的“空间模型”; Dormann,2007年),但是,这种方法不允许将模型转移到新的独立数据中(例如,时间和空间转移性)。
I propose a straightforward approach to reduce the effect of SA in SDM (see also Boavida et al., 2016 for more details). I use a simple example bellow focused on a brown algae species capable of producing marine forests and a set of environmental predictors known to largely explain its distribution.
我提出了一种直接的方法来减少SA在SDM中的影响(更多信息,另请参阅Boavida等人,2016)。 我用一个简单的例子来说明波纹管,它着重于能够生产海洋森林的褐藻物种,以及一组已知的环境预测因子,可以在很大程度上解释其分布。
Get the R code: Reducing spatial autocorrelationhttps://github.com/jorgeassis/spatialAutocorrelation
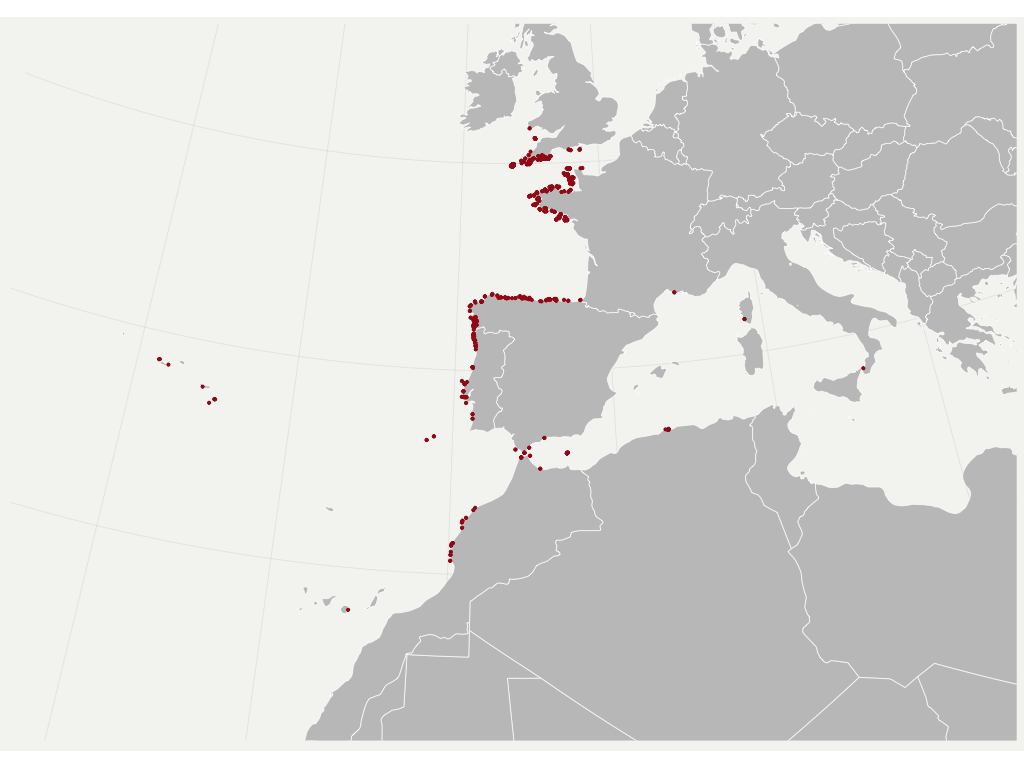
1. A correlogram is produced to assess the correlation of each variable predictor within a range of geographic distances.
1.产生相关图以评估地理距离范围内每个变量预测变量的相关性。
2. For each distance class, a linear model tests the effect of correlation with geographic distance. This finds the minimum non-significant autocorrelated distance.
2.对于每个距离类别,线性模型测试与地理距离的相关性影响。 这将找到最小的不重要的自相关距离。
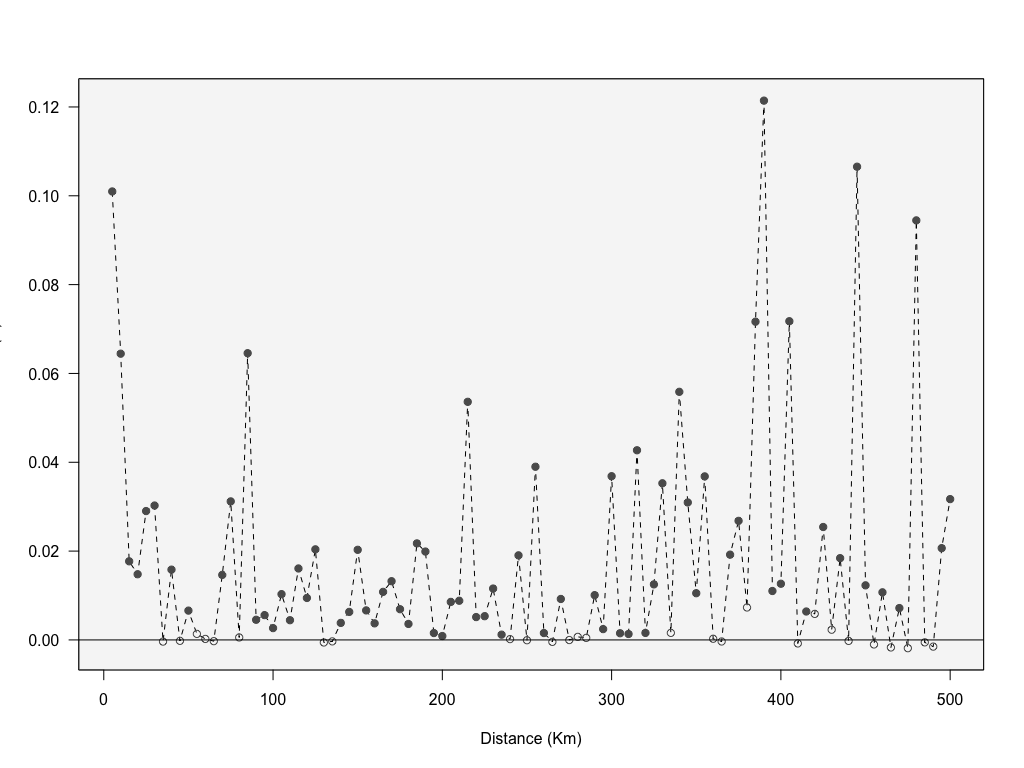
3. The average of the minimum non-significant distances found per variable is used to prune the occurrence records, by leaving only one record within such distance.
3.每个变量找到的最小非有效距离的平均值用于修剪出现记录,方法是在该距离内仅保留一个记录。
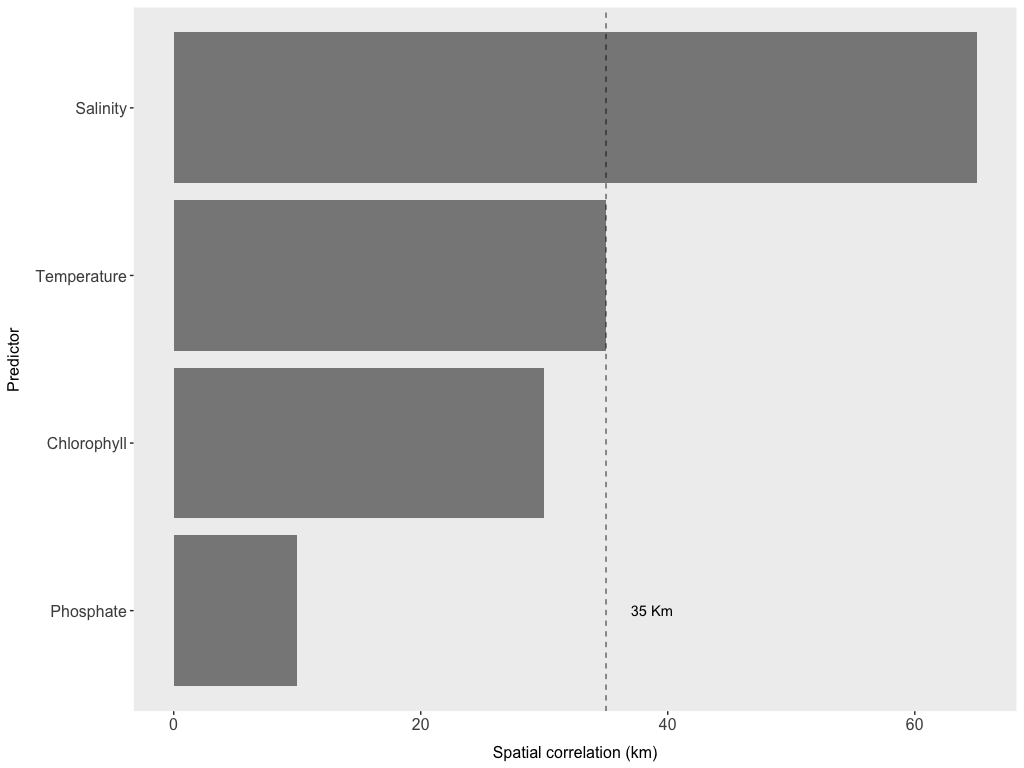
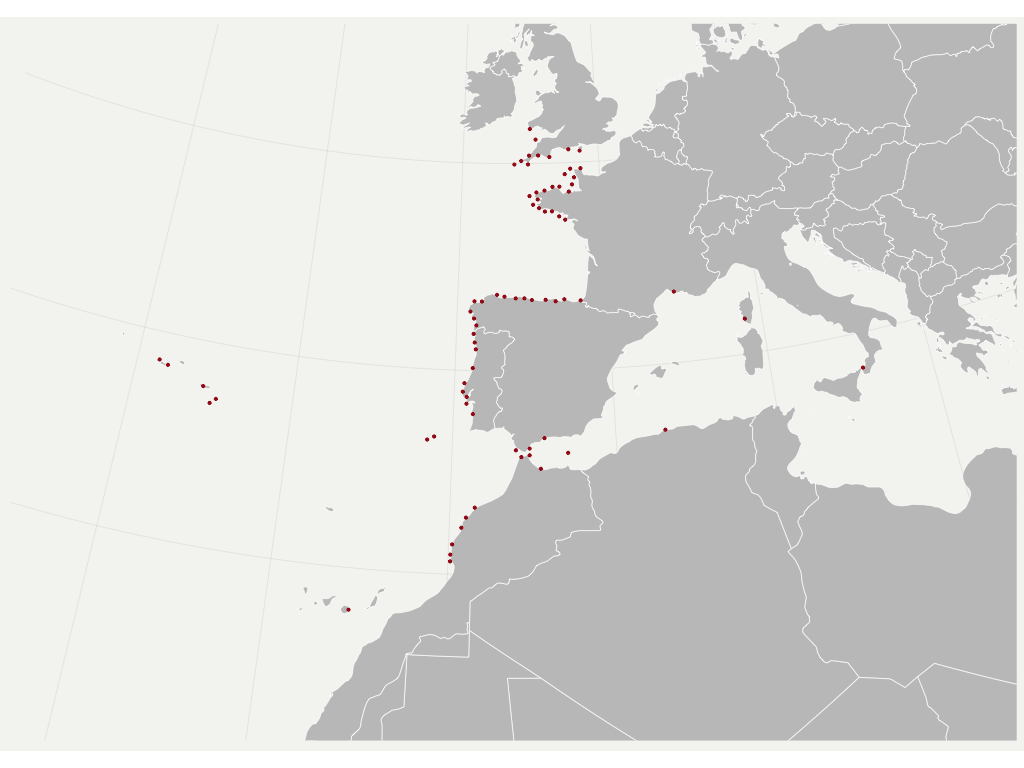
Get the R code: Reducing spatial autocorrelationhttps://github.com/jorgeassis/spatialAutocorrelation
引用文献 (Literature cited)
C. F. Dormann (2007) Effects of incorporating spatial autocorrelation into the analysis of species distribution data. Global Ecology and Biogeography. 16, 129–138.
CF Dormann(2007)将空间自相关纳入物种分布数据分析的影响。 全球生态与生物地理。 16、129–138。
Peterson, A.T., Soberon, J., Pearson, R.G., Anderson, R.P., Martinez-Meyer, E., Nakamura, M. &Araujo, M.B. (2011) Ecological niches and geographic distributions. Monographs in Population Biology, 314 pp. Vol. 49. Princeton University Press, Princeton.
Peterson,AT,Soberon,J.,Pearson,RG,Anderson,RP,Martinez-Meyer,E.,Nakamura,M.&Araujo,MB(2011)生态位和地理分布。 专着《人口生物学》 ,第314页,第一卷。 49.普林斯顿大学出版社,普林斯顿。
Boavida, J., Assis, J., Silva, I. et al. (2016) Overlooked habitat of a vulnerable gorgonian revealed in the Mediterranean and Eastern Atlantic by ecological niche modelling. Scientific Reports. 6, 36460.
Boavida,J.,Assis,J.,Silva,I。 等。 (2016)通过生态位建模在地中海和东大西洋发现了易受攻击的高哥人的栖息地。 科学报告。 6,36460。
翻译自: https://medium.com/themarinedatascientist/reducing-spatial-autocorrelation-in-species-distribution-models-fe84d4269cee
物种分布模型
本文来自互联网用户投稿,该文观点仅代表作者本人,不代表本站立场。本站仅提供信息存储空间服务,不拥有所有权,不承担相关法律责任。如若转载,请注明出处:http://www.mzph.cn/news/389047.shtml
如若内容造成侵权/违法违规/事实不符,请联系多彩编程网进行投诉反馈email:809451989@qq.com,一经查实,立即删除!