上凸包和下凸包
I recently came across the article titled High-dimensional data clustering by using local affine/convex hulls by HakanCevikalp in Pattern Recognition Letters. It proposes a novel algorithm to cluster high-dimensional data using local affine/convex hulls. I was inspired by their method of using convex hulls for clustering. I wanted to give a try at implementing my own simple clustering approach using convex hulls. So, in this article, I will walk you through my implementation of my clustering approach using convex hulls. Before we get into coding, let’s see what a convex hull is.
我最近在“ 模式识别字母”中碰到了一篇文章,标题为HakanCevikalp 使用本地仿射/凸包来进行高维数据聚类 。 提出了一种使用局部仿射/凸包对高维数据进行聚类的新算法。 他们使用凸包进行聚类的方法给我启发。 我想尝试使用凸包实现我自己的简单聚类方法。 因此,在本文中,我将引导您完成使用凸包的聚类方法的实现。 在进行编码之前,让我们看看什么是凸包。
凸包 (Convex Hull)
According to Wikipedia, a convex hull is defined as follows.
根据维基百科 ,凸包的定义如下。
In geometry, the convex hull or convex envelope or convex closure of a shape is the smallest convex set that contains it.
在几何中,形状的凸包或凸包络或凸包是包含该形状的最小凸集。
Let us consider an example of a simple analogy. Assume that there are a few nails hammered half-way into a plank of wood as shown in Figure 1. You take a rubber band, stretch it to enclose the nails and let it go. It will fit around the outermost nails (shown in blue) and take a shape that minimizes its length. The area enclosed by the rubber band is called the convex hull of the set of nails.
让我们考虑一个简单类比的例子。 如图1所示,假设有一些钉子被钉在一块木板上。将橡皮筋拉开,将其拉紧以包住钉子,然后松开。 它将适合最外面的钉子(以蓝色显示),并具有使长度最小化的形状。 橡皮筋包围的区域称为钉组的凸包 。
This convex hull (shown in Figure 1) in 2-dimensional space will be a convex polygon where all its interior angles are less than 180°. If it is in a 3-dimensional or higher-dimensional space, the convex hull will be a polyhedron.
这个在二维空间中的凸包(如图1所示)将是一个凸多边形 ,其所有内角均小于180°。 如果在3维或更高维空间中,则凸包将是多面体 。
There are several algorithms that can determine the convex hull of a given set of points. Some famous algorithms are the gift wrapping algorithm and the Graham scan algorithm.
有几种算法可以确定给定点集的凸包。 一些著名的算法是礼品包装算法和Graham扫描算法 。
Since a convex hull encloses a set of points, it can act as a cluster boundary, allowing us to determine points within a cluster. Hence, we can make use of convex hulls and perform clustering. Let’s get into the code.
由于凸包包围着一组点,因此它可以充当群集边界,从而使我们能够确定群集中的点。 因此,我们可以利用凸包并执行聚类。 让我们进入代码。
一个简单的例子 (A Simple Example)
I will be using Python for this example. Before getting started, we need the following Python libraries.
我将在此示例中使用Python。 在开始之前,我们需要以下Python库。
sklearn
numpy
matplotlib
mpl_toolkits
itertools
scipy
quadprog
数据集 (Dataset)
To create our sample dataset, I will be using sci-kit learn library’s make blobs function. I will make 3 clusters.
为了创建示例数据集,我将使用sci-kit学习库的make blobs函数。 我将制作3个群集。
import numpy as np
from sklearn.datasets import make_blobscenters = [[0, 1, 0], [1.5, 1.5, 1], [1, 1, 1]]
stds = [0.13, 0.12, 0.12]X, labels_true = make_blobs(n_samples=1000, centers=centers, cluster_std=stds, random_state=0)
point_indices = np.arange(1000)
Since this is a dataset of points with 3 dimensions, I will be drawing a 3D plot to show our ground truth clusters. Figure 2 denotes the scatter plot of the dataset with coloured clusters.
由于这是3维点的数据集,因此我将绘制3D图以显示我们的地面真相群集。 图2表示带有彩色簇的数据集的散点图。
import matplotlib.pyplot as plt
from mpl_toolkits.mplot3d import Axes3Dx = X[:,0]
y = X[:,1]
z = X[:,2]
# Creating figure
fig = plt.figure(figsize = (15, 10))
ax = plt.axes(projection ="3d")
# Add gridlines
ax.grid(b = True, color ='grey',
linestyle ='-.', linewidth = 0.3,
alpha = 0.2)
mycolours = ["red", "green", "blue"]# Creating color map
col = [mycolours[i] for i in labels_true]# Creating plot
sctt = ax.scatter3D(x, y, z, c = col, marker ='o')plt.title("3D scatter plot of the data\n")
ax.set_xlabel('X-axis', fontweight ='bold')
ax.set_ylabel('Y-axis', fontweight ='bold')
ax.set_zlabel('Z-axis', fontweight ='bold')
# show plot
plt.draw()
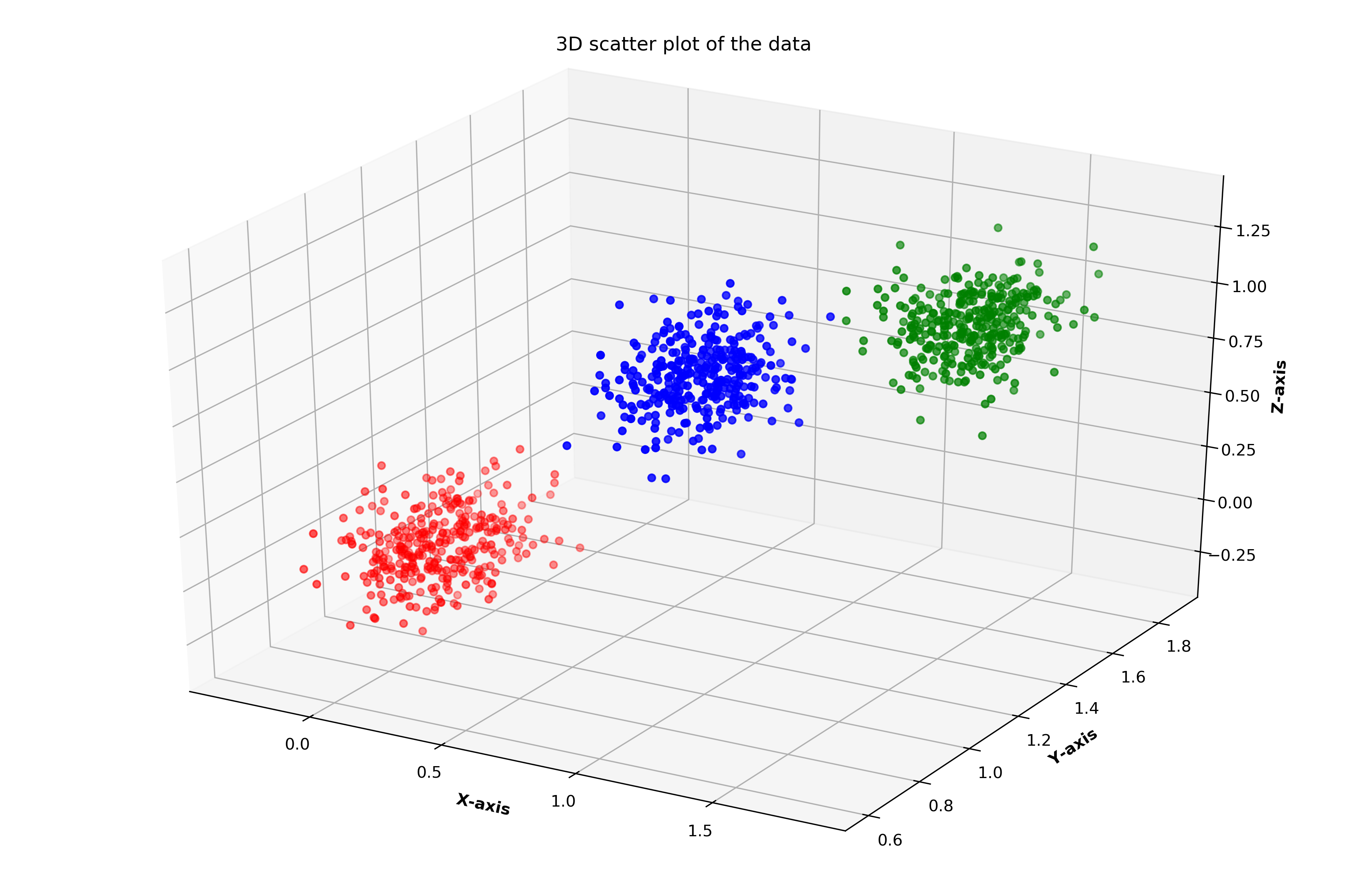
获取初始聚类 (Obtaining an Initial Clustering)
First, we need to break our dataset into 2 parts. One part will be used as seeds to obtain an initial clustering using K-means. The points in the other part will be assigned to clusters based on the initial clustering.
首先,我们需要将数据集分为两部分。 一部分将用作种子,以使用K均值获得初始聚类。 另一部分中的点将根据初始聚类分配给聚类。
from sklearn.model_selection import train_test_splitX_seeds, X_rest, y_seeds, y_rest, id_seeds, id_rest = train_test_split(X, labels_true, point_indices, test_size=0.33, random_state=42)
Now we perform K-means clustering on the seed points.
现在我们对种子点执行K-均值聚类。
from sklearn.cluster import KMeanskmeans = KMeans(n_clusters=3, random_state=9).fit(X_seeds)
initial_result = kmeans.labels_
Since the resulting labels may not be the same as the ground truth labels, we have to map the two sets of labels. For this, we can use the following function.
由于生成的标签可能与地面真相标签不同,因此我们必须映射两组标签。 为此,我们可以使用以下功能。
from itertools import permutations# Source: https://stackoverflow.com/questions/11683785/how-can-i-match-up-cluster-labels-to-my-ground-truth-labels-in-matlabdef remap_labels(pred_labels, true_labels): pred_labels, true_labels = np.array(pred_labels), np.array(true_labels)
assert pred_labels.ndim == 1 == true_labels.ndim
assert len(pred_labels) == len(true_labels)
cluster_names = np.unique(pred_labels)
accuracy = 0 perms = np.array(list(permutations(np.unique(true_labels)))) remapped_labels = true_labels for perm in perms: flipped_labels = np.zeros(len(true_labels))
for label_index, label in enumerate(cluster_names):
flipped_labels[pred_labels == label] = perm[label_index] testAcc = np.sum(flipped_labels == true_labels) / len(true_labels) if testAcc > accuracy:
accuracy = testAcc
remapped_labels = flipped_labels return accuracy, remapped_labels
We can get the accuracy and the mapped initial labels from the above function.
我们可以从上面的函数中获得准确性和映射的初始标签。
intial_accuracy, remapped_initial_result = remap_labels(initial_result, y_seeds)
Figure 3 denotes the initial clustering of the seed points.
图3表示种子点的初始聚类。
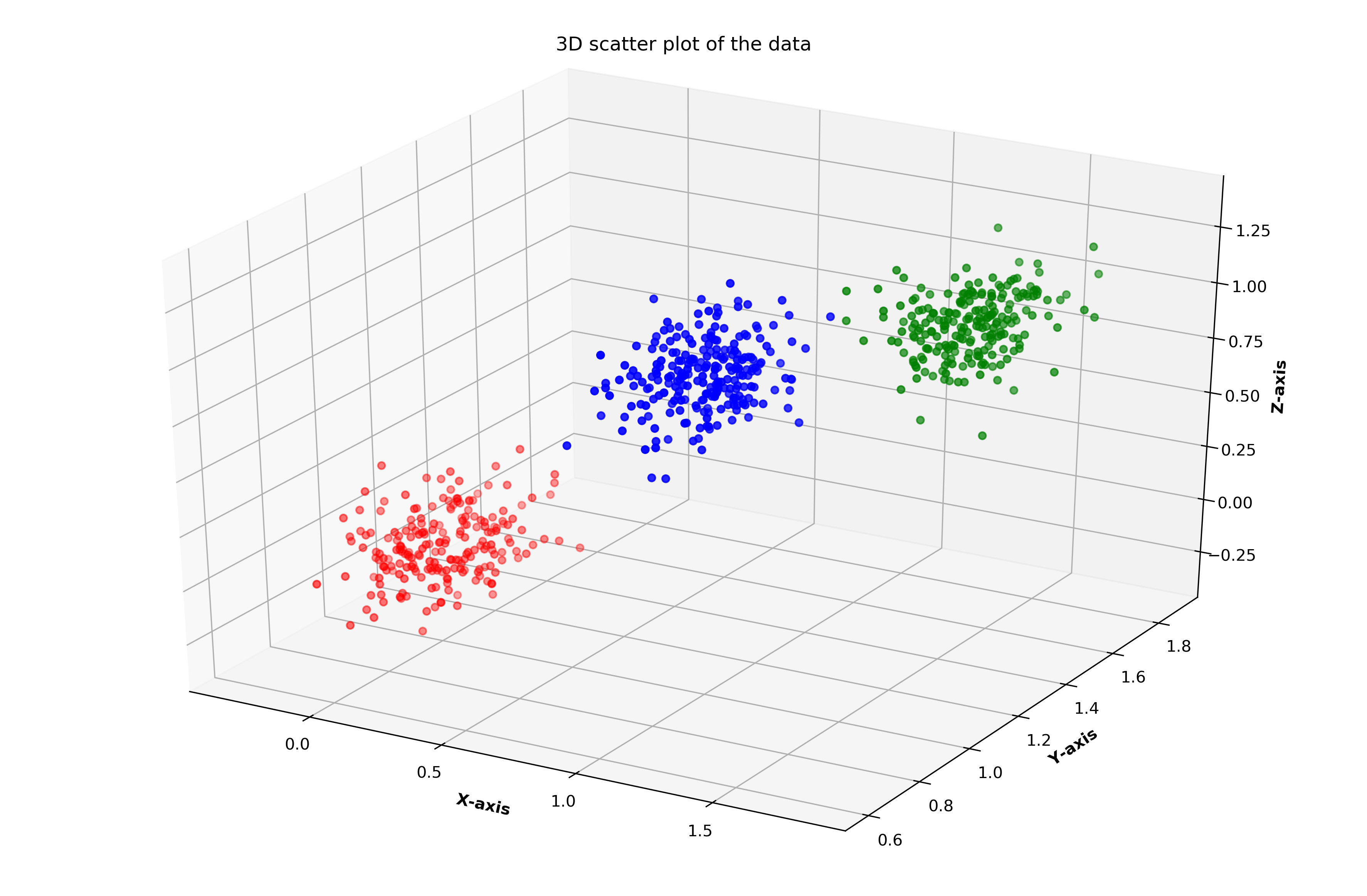
获取初始聚类的凸包 (Get Convex Hulls of the Initial Clustering)
Once we have obtained an initial clustering, we can get the convex hulls for each cluster. First, we have to get the indices of each data point in the clusters.
一旦获得初始聚类,就可以获取每个聚类的凸包。 首先,我们必须获取群集中每个数据点的索引。
# Get the idices of the data points belonging to each cluster
indices = {}for i in range(len(id_seeds)):
if int(remapped_initial_result[i]) not in indices:
indices[int(remapped_initial_result[i])] = [i]
else:
indices[int(remapped_initial_result[i])].append(i)
Now we can obtain the convex hulls from each cluster.
现在我们可以从每个聚类中获得凸包。
from scipy.spatial import ConvexHull# Get convex hulls for each cluster
hulls = {}for i in indices:
hull = ConvexHull(X_seeds[indices[i]])
hulls[i] = hull
Figure 4 denotes the convex hulls representing each of the 3 clusters.
图4表示分别代表3个群集的凸包。
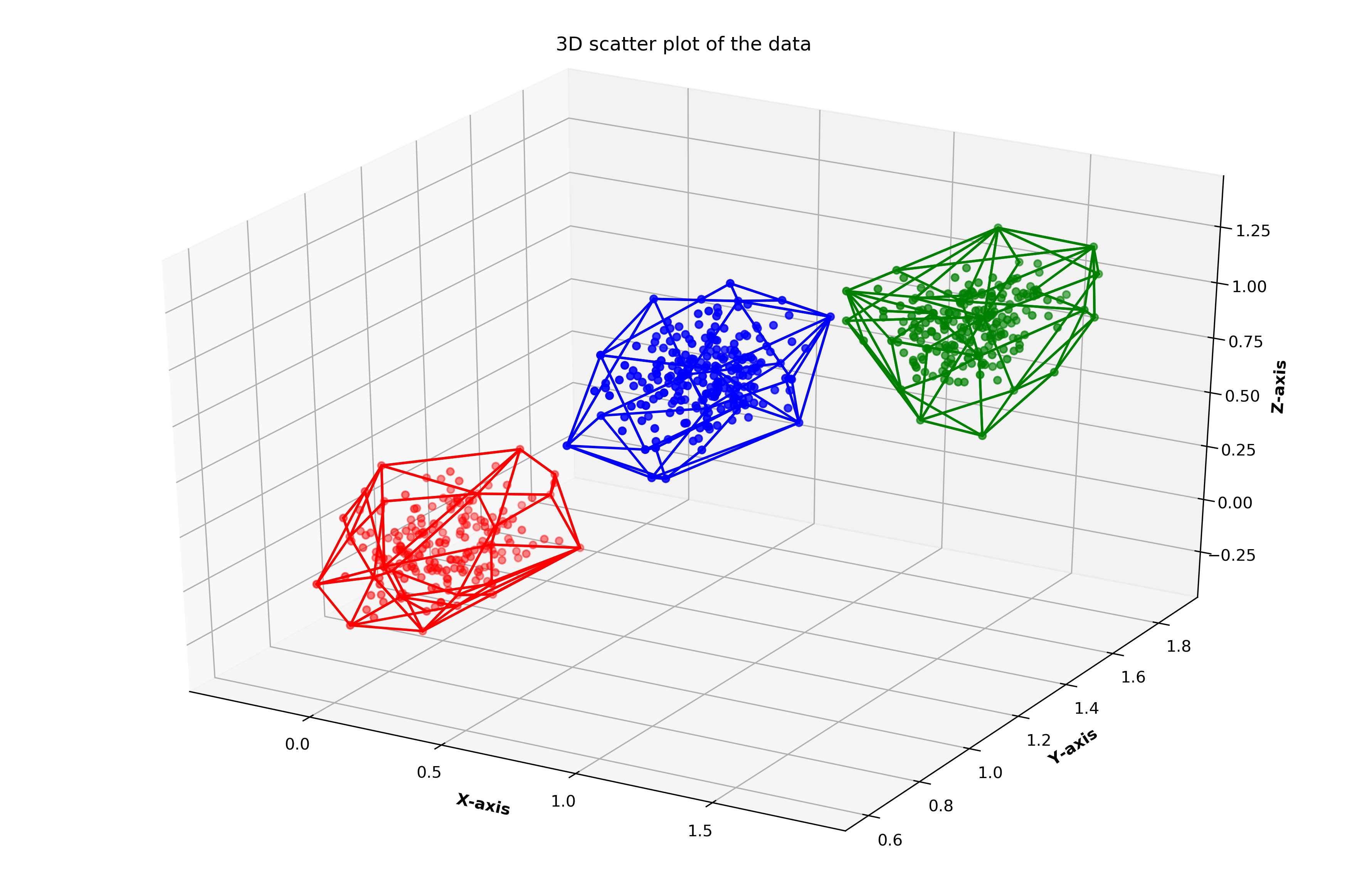
将剩余点分配给最接近的凸包的群集 (Assign Remaining Points to the Cluster of the Closest Convex Hull)
Now that we have the convex hulls of the initial clusters, we can assign the remaining points to the cluster of the closest convex hull. First, we have to get the projection of the data point on to a convex hull. To do so, we can use the following function.
现在我们有了初始聚类的凸包,我们可以将其余点分配给最接近的凸包的聚类。 首先,我们必须将数据点投影到凸包上。 为此,我们可以使用以下功能。
from quadprog import solve_qp# Source: https://stackoverflow.com/questions/42248202/find-the-projection-of-a-point-on-the-convex-hull-with-scipydef proj2hull(z, equations): G = np.eye(len(z), dtype=float)
a = np.array(z, dtype=float)
C = np.array(-equations[:, :-1], dtype=float)
b = np.array(equations[:, -1], dtype=float) x, f, xu, itr, lag, act = solve_qp(G, a, C.T, b, meq=0, factorized=True) return x
The problem of finding the projection of a point on a convex hull can be solved using quadratic programming. The above function makes use of the quadprog
module. You can install the quadprog
module using conda
or pip
.
查找点在凸包上的投影的问题可以使用二次编程解决。 上面的功能利用了quadprog
模块。 您可以安装quadprog
使用模块conda
或pip
。
conda install -c omnia quadprog
OR
pip install quadprog
I won’t go into details about how to solve this problem using quadratic programming. If you are interested, you can read more from here and here.
我不会详细介绍如何使用二次编程解决此问题。 如果您有兴趣,可以从这里和这里内容。
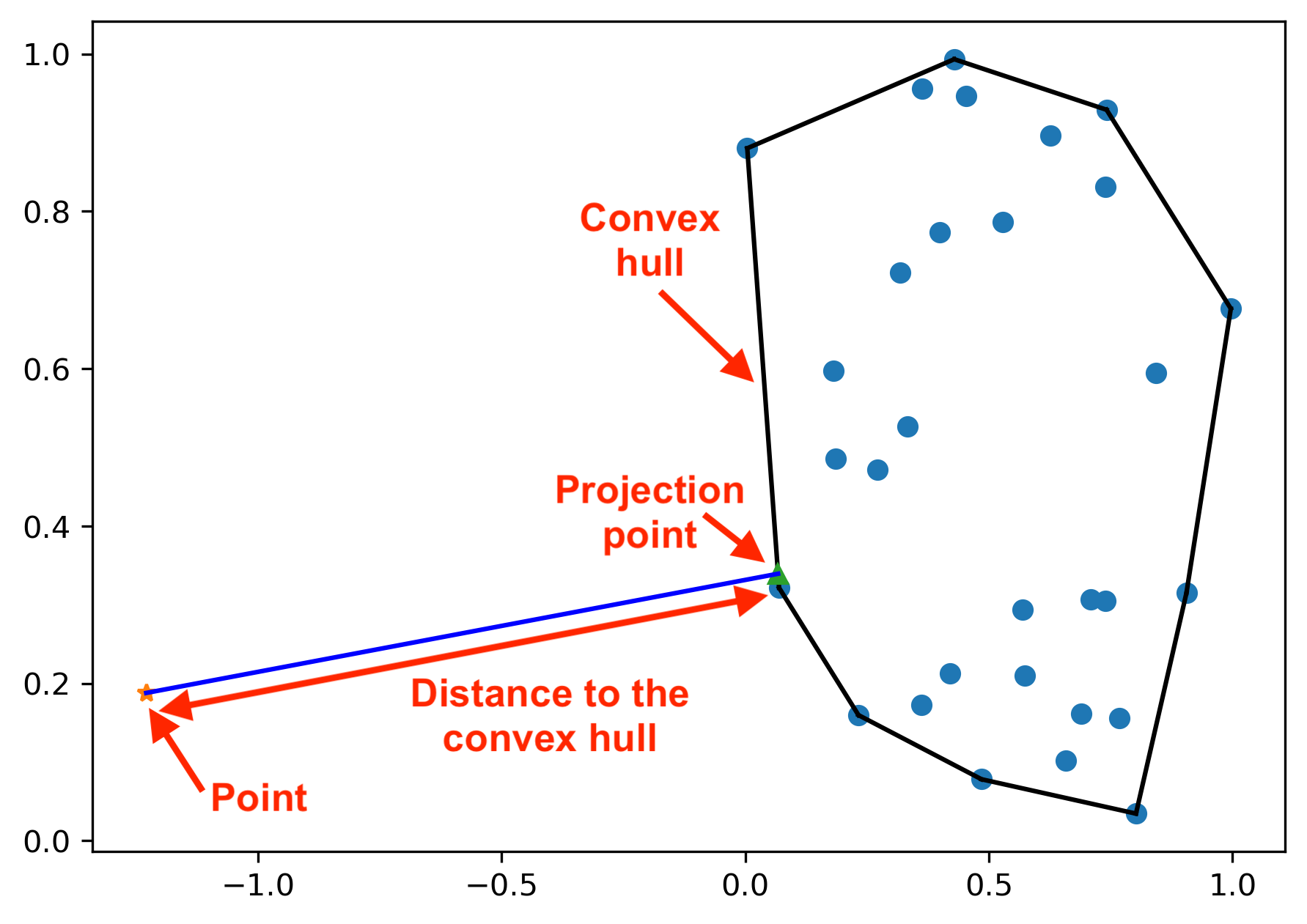
Once you have obtained the projection on the convex hull, you can calculate the distance from the point to the convex hull as shown in Figure 5. Based on this distance, now let’s assign the remaining data points to the cluster of the closest convex hull.
一旦获得了凸包的投影,就可以计算从点到凸包的距离,如图5所示。现在,基于该距离,我们将剩余的数据点分配给最近的凸包的群集。
I will consider the Euclidean distance from the data point to its projection on the convex hull. Then the data point will be assigned to the cluster with the convex hull having the shortest distance from that data point. If a point lies within the convex hull, then the distance will be 0.
我将考虑从数据点到其在凸包上的投影的欧几里得距离。 然后,将数据点分配给群集,其中凸包距该数据点的距离最短。 如果点位于凸包内,则距离将为0。
prediction = []for z1 in X_rest: min_cluster_distance = 100000
min_distance_point = ""
min_cluster_distance_hull = ""
for i in indices: p = proj2hull(z1, hulls[i].equations) dist = np.linalg.norm(z1-p) if dist < min_cluster_distance: min_cluster_distance = dist
min_distance_point = p
min_cluster_distance_hull = i prediction.append(min_cluster_distance_hull)prediction = np.array(prediction)
Figure 6 denotes the final clustering result.
图6表示最终的聚类结果。
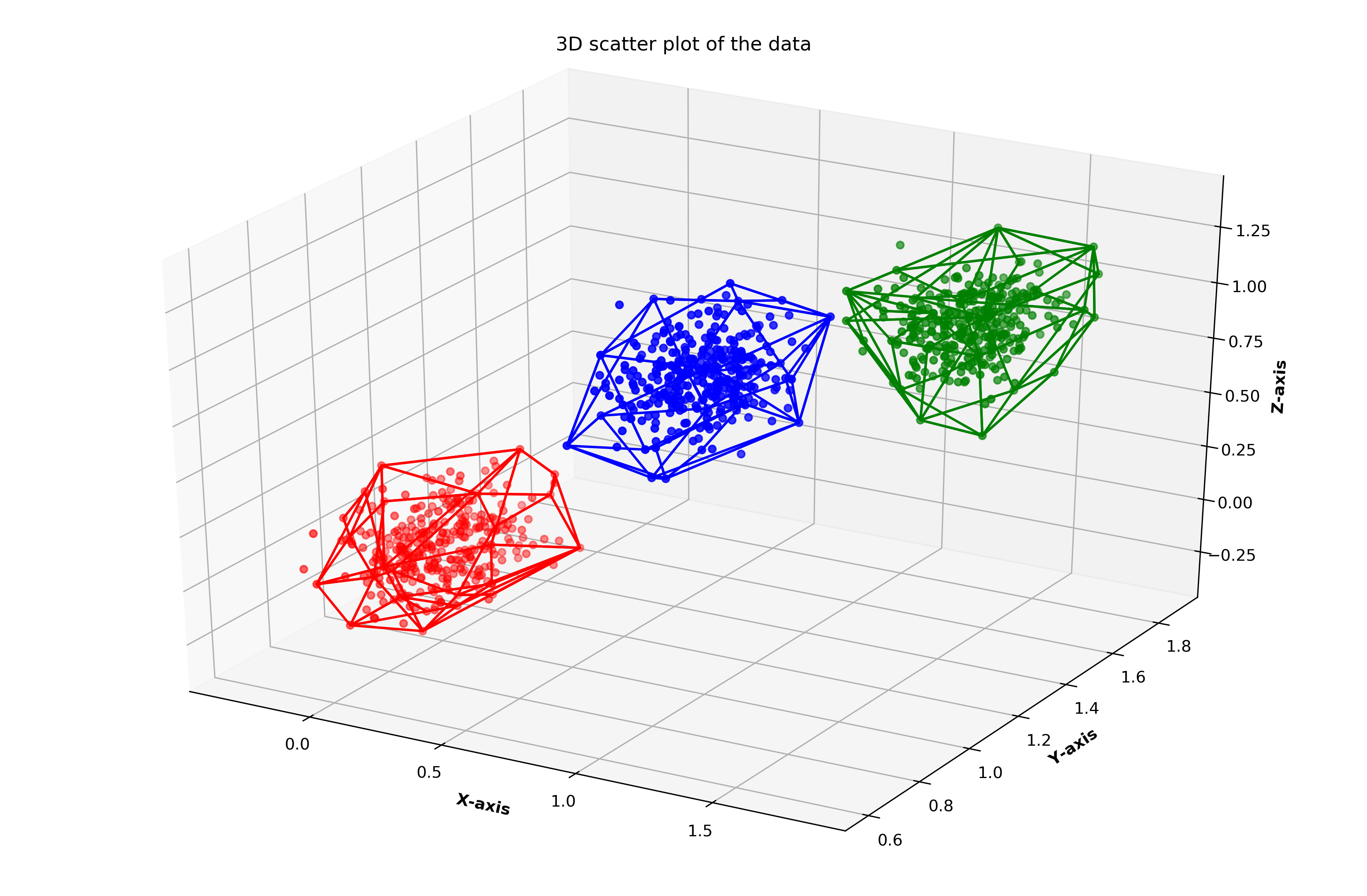
评估最终结果 (Evaluate the Final Result)
Let’s evaluate our result to see how accurate it is.
让我们评估我们的结果以查看其准确性。
from sklearn.metrics import accuracy_scoreY_pred = np.concatenate((remapped_initial_result, prediction))
Y_real = np.concatenate((y_seeds, y_rest))
print(accuracy_score(Y_real, Y_pred))
I got an accuracy of 1.0 (100%)! Awesome and exciting right? 😊
我的准确度是1.0(100%)! 太棒了,令人兴奋吧? 😊
If you want to know more about evaluating clustering results, you can check out my previous article Evaluating Clustering Results.
如果您想了解有关评估聚类结果的更多信息,可以查阅我之前的文章评估聚类结果 。
I have used a very simple dataset. You can try this method with more complex datasets and see what happens.
我使用了一个非常简单的数据集。 您可以对更复杂的数据集尝试此方法,然后看看会发生什么。
高维数据 (High-dimensional data)
I also tried to cluster a dataset with data points having 8 dimensions using my cluster hull method. You can find the jupyter notebook showing the code and results. The final results are as follows.
我还尝试使用我的群集包方法将数据集与8个维度的数据点群集在一起。 您可以找到显示代码和结果的jupyter笔记本 。 最终结果如下。
Accuracy of K-means method: 0.866
Accuracy of Convex Hull method: 0.867
There is a slight improvement in my convex hull method over K-means.
与K均值相比,我的凸包方法略有改进。
最后的想法 (Final Thoughts)
The article titled High-dimensional data clustering by using local affine/convex hulls by HakanCevikalp shows that the convex hull-based method they proposed avoids the “hole artefacts” problem (the sparse and irregular distributions in high-dimensional spaces can make the nearest-neighbour distances unreliable) and improves the accuracy of high-dimensional datasets over other state-of-the-art subspace clustering methods.
由HakanCevikalp撰写的使用局部仿射/凸包进行高维数据聚类的文章显示,他们提出的基于凸包的方法避免了“ Kong伪像 ”问题(高维空间中稀疏和不规则的分布可以使最近的邻居距离不可靠),并比其他最新的子空间聚类方法提高了高维数据集的准确性。
You can find the jupyter notebook containing the code used for this article.
您可以找到包含本文所用代码的jupyter笔记本 。
Hope this article was interesting and useful.
希望本文有趣而有用。
Cheers! 😃
干杯! 😃
翻译自: https://towardsdatascience.com/clustering-using-convex-hulls-fddafeaa963c
上凸包和下凸包
本文来自互联网用户投稿,该文观点仅代表作者本人,不代表本站立场。本站仅提供信息存储空间服务,不拥有所有权,不承担相关法律责任。如若转载,请注明出处:http://www.mzph.cn/news/389017.shtml
如若内容造成侵权/违法违规/事实不符,请联系多彩编程网进行投诉反馈email:809451989@qq.com,一经查实,立即删除!