詹森不等式
背景 (Background)
In Kaggle’s M5 Forecasting — Accuracy competition, the square root transformation ruined many of my team’s forecasts and led to a selective patching effort in the eleventh hour. Although it turned out well, we were reminded that “reconstitution bias” can plague predictions on the original scale, even with common transformations such as the square root.
在Kaggle的“ M5预测-准确性”竞赛中 ,平方根转换破坏了我团队的许多预测,并在第11小时进行了选择性修补工作。 尽管结果很好 ,但我们仍被提醒,“重构偏见”会困扰原始规模的预测,即使采用平方根之类的常见转换也是如此。
平方根变换 (The square root transformation)
For Poisson data, the rationale of the square root is that it is a variance-stabilizing transformation; in theory, the square root of the values are distributed approximately normal with constant variance and a mean that is the square root of the original mean. It is an approximation, and as Wikipedia puts it, one in which the “convergence to normality (as [the original mean] increases) is far faster than the untransformed variable.”
对于Poisson数据 ,平方根的基本原理是它是方差稳定的变换; 从理论上讲,值的平方根近似分布,且具有恒定方差,且均值是原始均值的平方根。 正如Wikipedia所说 ,这是一种近似,其中“ 归一化的收敛性(随着(原始均值)的增加)比未转换的变量快得多。 ”
Imagine you decide to take square roots in a count data scenario, feeling good reassured that the convergence to normality is “fast.” You then model the mean of square-root transformed data and then get predictions on the square root scale. At some point, especially in a forecasting scenario, you’ll have to get back to the original scale. That probably entails squaring the model-estimated means. The M5 competition served as a reminder that this approach can and will break down.
想象一下,您决定在计数数据方案中求平方根,并确信向正态的收敛是“快速的”。 然后,您可以对平方根转换后的数据的均值建模,然后获得平方根尺度的预测。 在某些时候,尤其是在预测情况下,您必须回到原始比例。 这可能需要对模型估计的均方进行平方。 M5竞赛提醒我们,这种方法可能并且将会失败。
詹森差距 (The Jensen Gap)
Jensen’s Inequality states that for convex functions, the function evaluated at the expectation is less than or equal to the expectation of the function, i.e., g(E[Y]) ≤ E[g(Y)]. The inequality is flipped for concave functions.
Jensen不等式指出,对于凸函数,按期望评估的函数小于或等于该函数的期望,即g(E [Y])≤E [g(Y)]。 对于凹函数,不等式被翻转。
Similarly, the Jensen Gap is defined as the difference E[g(Y)]-g(E[Y]), which is positive for convex functions g. (As an aside, notice that when g(x) is the square function, the Jensen Gap is the Variance of Y, which had better be non-negative!)
类似地, 詹森差距定义为差E [ g ( Y )]- g (E [ Y ]),对于凸函数g为正。 (顺便说一句, 请注意,当g ( x )是平方函数时,Jensen Gap 是 Y的方差,最好是非负的!)
When considering g(x) as the square function and the square root of Y as the random variable, the Jensen Gap becomes E[Y]-E[sqrt(Y)]². Since that quantity is positive, our reconstituted mean will be biased downward. To learn more about the magnitude of the gap, we turn to the Taylor expansion.
当将g ( x )作为平方函数并将Y的 平方根作为随机变量时,Jensen Gap变为E [ Y ] -E [sqrt( Y )]²。 由于该数量为正,因此我们重构的均值将向下偏向。 要了解有关差距大小的更多信息,我们转向泰勒展开。
泰勒展开至近似偏差 (Taylor expansion to approximate bias)
To the Mathematics StackExchange prompt “Expected Value of Square Root of Poisson Random Variable,” contributor Hernan Gonzalez explains the Taylor expansion of a random variable about its mean, as shown in the screenshot below.
在数学StackExchange提示“ 泊松随机变量平方根的期望值 ”中, 贡献者Hernan Gonzalez解释了随机变量的泰勒展开式及其均值,如下面的屏幕快照所示。
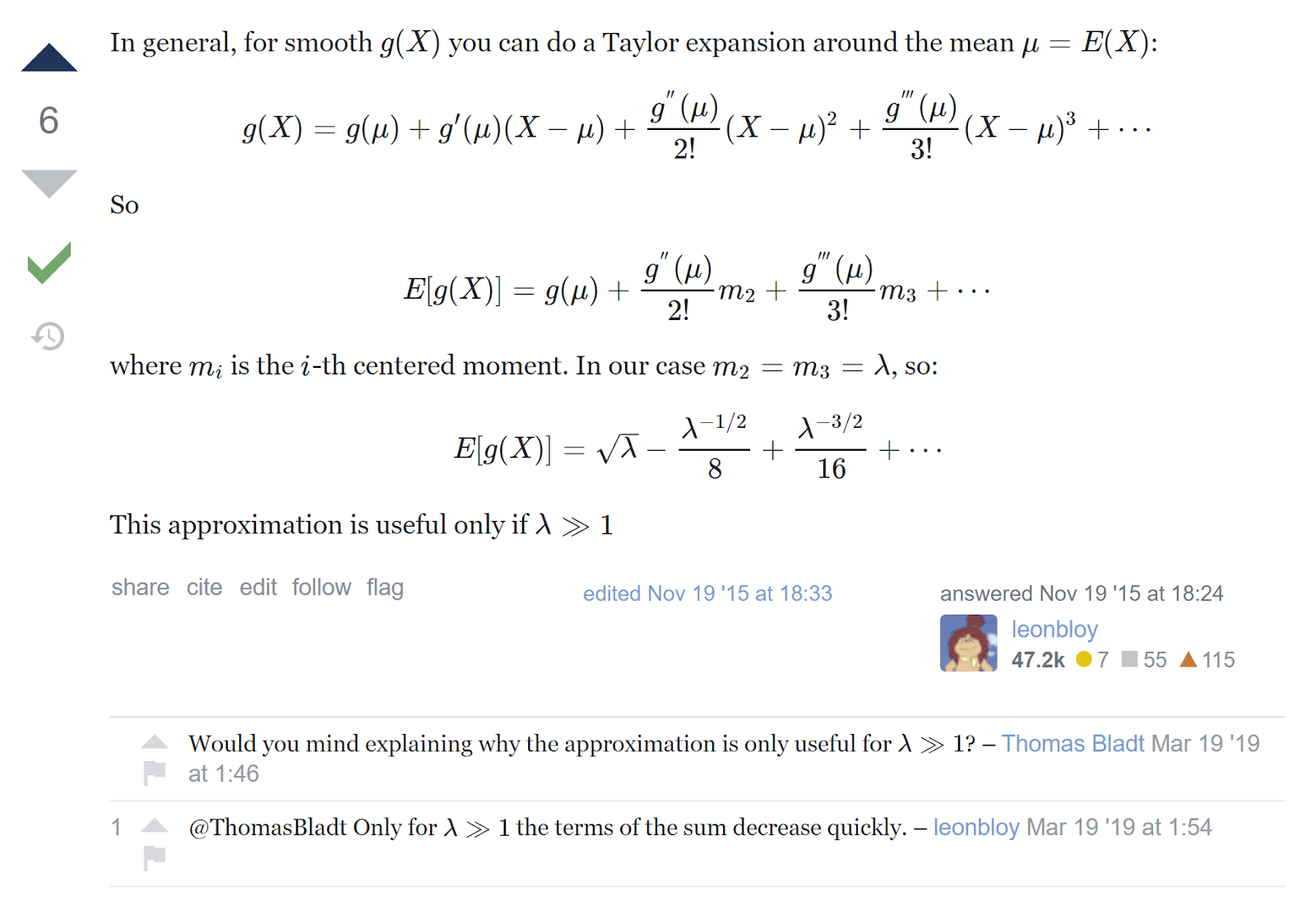
Note that the expansion needs at least a few central moments of the original distribution. For the Poisson, the first three are just the mean parameter.
请注意,展开至少需要原始分布的几个中心时刻。 对于泊松而言,前三个只是均值参数。
Ignoring that the mean estimator is also a random variable, we can run the expectation above through the inverse transformation, i.e., square it, to get an idea of the bias on the original scale for any Poisson mean value (the algebra isn’t here but it’s computed in line 34 of the demonstration code.) Similarly, with properties of the square root of the random variable, it’s straightforward to analyze g(x) = x ^2 in the same way. That opens up the possibility of bias correction, an interesting proposition, albeit one with assumptions and complexities of its own.
忽略均值估计器也是一个随机变量,我们可以通过逆变换在上面运行期望值,即对它求平方,以了解任何泊松均值在原始比例上的偏差(代数不在此处但是,它是在演示代码的第34行中计算出来的 。)类似地,由于具有随机变量的平方根的属性,因此以相同的方式分析g (x)= x ^ 2很简单。 这开辟了偏差校正的可能性,这是一个有趣的主张,尽管它有其自身的假设和复杂性。
近似分解 (Approximation breakdown)
Near the end of his answer, Gonzalez mentions that the approximation “is only useful if” the mean of the original Poisson is quite a bit bigger than 1, clarifying in the comments that this is needed so that “the terms of the sum decrease quickly.” That follows from the mean being raised to negative powers after the original term.
冈萨雷斯在回答接近尾声时提到,“ 仅当 ”原始泊松的均值比1大很多时,近似值“ 才有用 ”,并在注释中阐明了这一点是必要的,以便“ 总和的项Swift减少”。 。 ”这是因为原任期之后,均值被提升为负数。
In the M5 competition, mean sales for many items were substantially below one, and thus using the square root transformation was a recipe for poor performance. To get an idea of how this plays out in an actual sample, the next section will investigate this phenomenon via simulation.
在M5竞赛中,许多商品的平均销售额都大大低于1,因此使用平方根变换是降低性能的良方。 为了了解这种情况在实际样本中如何发挥作用,下一部分将通过仿真研究这种现象。
示范 (Demonstration)
In this section, we use the loess smoother to create models on both the original scale and the square root scale, and square the mean estimates of the latter. For simulated Poisson data with both a mean of 20 and a mean of 0.2, we plot the two sets of predictions and examine the bias. The code is under 50 lines and is available in Nousot’s Public Github repository.
在本节中,我们将使用黄土平滑器在原始比例和平方根比例上创建模型,并对后者的均值进行平方。 对于均值为20和均值为0.2的模拟Poisson数据,我们绘制了两组预测并检查了偏差。 该代码少于50行,可在Nousot的Public Github存储库中找到 。
当平均值是20 (When the mean is 20)
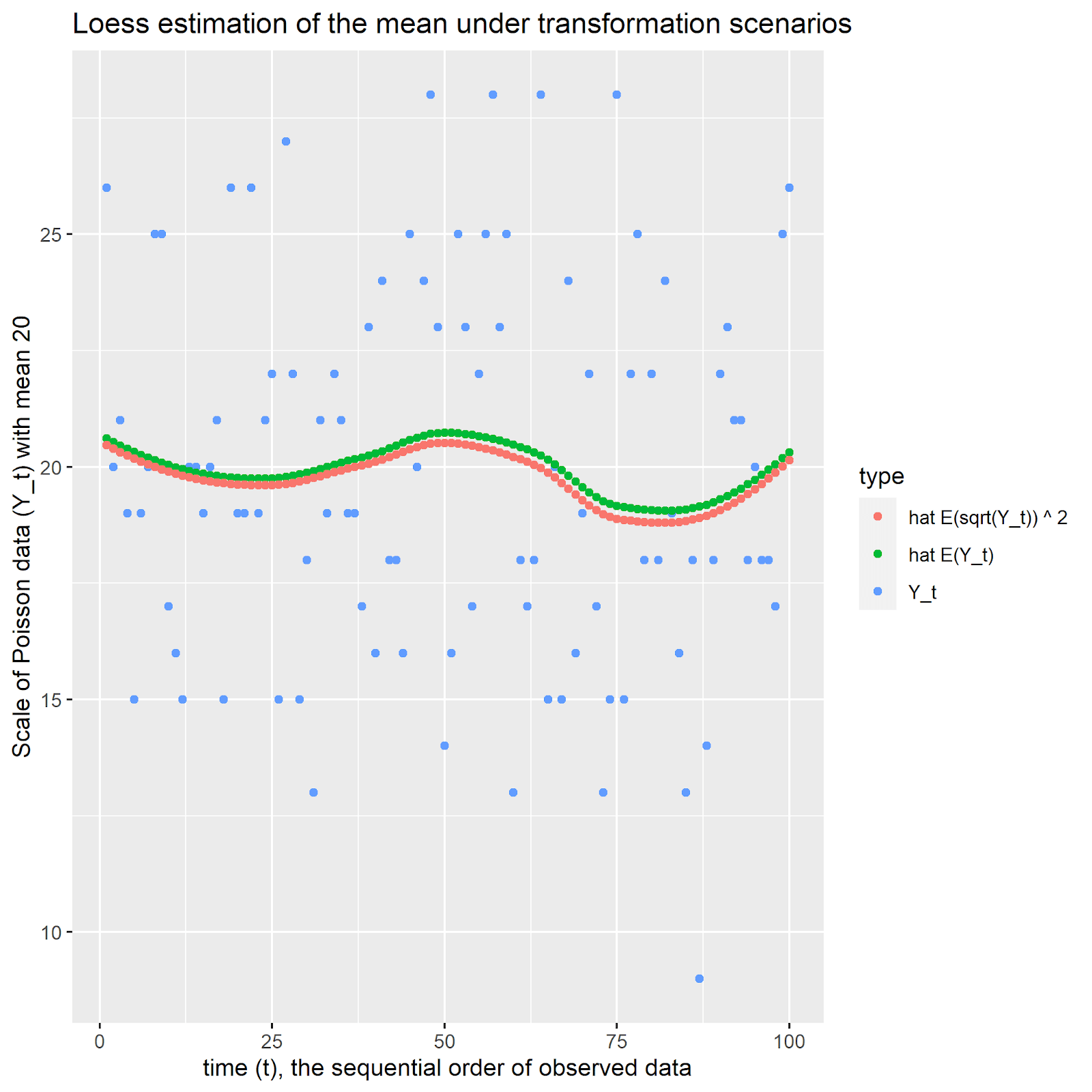
For the case where the mean of the Poisson random variable is 20, the retransformation bias is negative (as Jensen’s Inequality said it would be), but also relatively small. In the code, the first two terms of the Taylor expansion are computed and compared to the empirical bias on the square root scale. At -0.027 and -0.023, respectively, they are relatively close.
对于泊松随机变量的平均值为20的情况,重变换偏差为负(就像詹森的不等式所说的那样),但也相对较小。 在代码中,计算出泰勒展开的前两个项,并将其与平方根尺度上的经验偏差进行比较。 它们分别为-0.027和-0.023,相对接近。
当平均值为0.20时 (When the mean is 0.20)
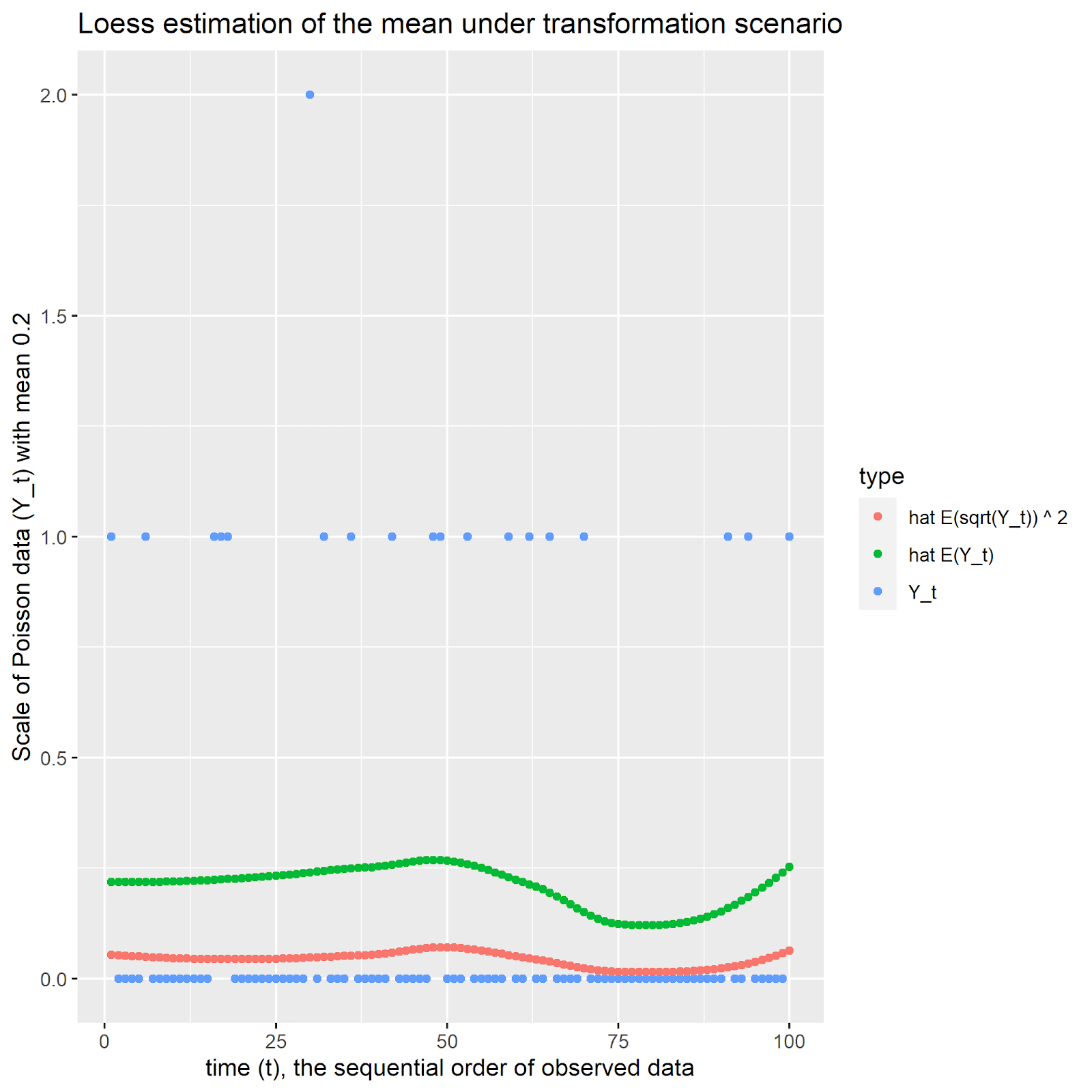
For the case where the mean of the Poisson random variable is 0.20, the picture is much different. While Jensen’s Inequality always holds, the Jensen Gap is now large in a relative sense. Furthermore, the Taylor approximation has completely broken down, with the first two bias terms summing to 0.419 while the empirical bias is -.251 (still on the square root scale).
对于泊松随机变量的平均值为0.20的情况,图片有很大不同。 尽管詹森的不平等现象始终存在,但詹森差距现在相对来说还是很大的。 此外,泰勒近似已完全分解,前两个偏差项的总和为0.419,而经验偏差为-.251(仍在平方根刻度上)。
讨论区 (Discussion)
David Warton’s 2018 paper “Why You Cannot Transform Your Way Out of Trouble for Small Counts” demonstrates the hopelessness of getting to the standard assumptions for small-mean count data. For the sparse time series in M5, there was nothing to gain and a lot to lose by taking the square root. At the very least, we should have treated those series differently. (Regarding our use of the Kalman Filter, Otto Seiskari’s advice to tune via cross-validation when the model is misspecified is especially compelling).
戴维·沃顿(David Warton)在2018年发表的论文“ 为什么小数位数无法摆脱麻烦 ”,这说明了达到小数位数数据的标准假设的绝望。 对于M5中稀疏的时间序列,通过求平方根没有任何收益,也有很多损失。 至少,我们应该对这些系列进行不同的处理。 (关于我们对卡尔曼滤波器的使用,当模型指定不正确时, Otto Seiskari的建议通过交叉验证进行调谐特别引人注目)。
Warton’s paper has some harsh words for users of transformations in general. I still believe that if a transformation brings you closer to the standard assumptions, where your code runs faster and you enjoy nicer properties, then it’s worth considering. But there needs to be an honest exploration of properties of the transformation in the context of the data, and this does not come for free.
一般而言,沃顿的论文对转换的使用者来说有些苛刻的话。 我仍然相信,如果 转换使您更接近标准假设,即代码运行速度更快并且享受更好的属性,因此值得考虑。 但是需要在数据的上下文中诚实地探索转换的属性,而这并不是免费的。
Typically transformations (and their inverses) are either convex or concave, and thus Jensen’s Inequality will guarantee bias in the form of a Jensen Gap. If you’re wondering why you’ve never heard of it, it’s because it’s often written off as approximation error. According to Gao et al (2018),
通常,变换(及其逆变换)是凸的或凹的,因此Jensen的不等式将保证以Jensen Gap的形式出现偏差。 如果您想知道为什么从未听说过它,那是因为它经常被记为近似误差。 根据Gao等人(2018) ,
“Computing a hard-to-compute [expectation of a function] appears in theoretical estimates in a variety of scenarios from statistical mechanics to machine learning theory. A common approach to tackle this problem is to … show that the error, i.e., the Jensen gap, would be small enough for the application.”
从统计力学到机器学习理论,在各种情况下的理论估计中都出现了计算难以计算的[函数期望]。 解决此问题的常用方法是……表明误差(即詹森间隙)对于应用程序而言足够小。”
When using transformations, the work to understand the properties of inverse-transformation (in the context of the data) is worth it. It’s dangerous out there. Watch your step, and mind the Jensen Gap!
使用转换时,了解逆转换属性(在数据上下文中)的工作是值得的。 那里很危险。 注意您的脚步,并注意詹森差距!
翻译自: https://towardsdatascience.com/mind-the-jensen-gap-c54e0eb9e1b7
詹森不等式
本文来自互联网用户投稿,该文观点仅代表作者本人,不代表本站立场。本站仅提供信息存储空间服务,不拥有所有权,不承担相关法律责任。如若转载,请注明出处:http://www.mzph.cn/news/388668.shtml
如若内容造成侵权/违法违规/事实不符,请联系多彩编程网进行投诉反馈email:809451989@qq.com,一经查实,立即删除!