ai前沿公司
In 1950, Alan Turing developed the Turing Test as a test of a machine’s ability to display human-like intelligent behavior. In his prolific paper, he posed the following questions:
1950年,阿兰图灵开发的图灵测试作为一台机器的显示类似人类的智能行为能力的考验。 在他的论文中 ,他提出了以下问题:
“Can machines think?”
“机器可以思考吗?”
“Are there imaginable digital computers which would do well in the imitation game?”
“有没有可以想象的数字计算机在模仿游戏中表现出色?”
In most applications of AI, a model is created to imitate the judgment of humans and implement it at scale, be it autonomous vehicles, text summarization, image recognition, or product recommendation.
在大多数AI应用中,都会创建一个模型来模仿人类的判断并大规模实施,例如自动驾驶汽车,文本摘要,图像识别或产品推荐。
By the nature of imitation, a computer is only able to replicate what humans have done, based on previous data. This doesn’t leave room for genuine creativity, which relies on innovation, not imitation.
根据模仿的性质,计算机只能根据以前的数据来复制人类所做的事情。 这就没有真正的创造力的余地,真正的创造力是依靠创新而不是模仿。
But more recently, computer-generated creations have started to push the boundaries between imitation and innovation across various mediums.
但是最近,计算机生成的作品已经开始跨各种媒介推动模仿与创新之间的界限。
The question arises: can a computer be creative? Can it be taught to innovate on its own and generate original outputs? And can it do this in a way that makes it indistinguishable from human creativity?
随之而来的问题是:计算机能否具有创造力? 可以教它自己进行创新并产生原始输出吗? 并能以使其与人类创造力没有区别的方式做到这一点吗?
Here, a few developments at the intersection of art and AI that can help us to answer those questions.
在这里,艺术和人工智能的交汇处可以帮助我们回答这些问题。
1.埃德蒙·德·贝拉米 (1. Edmond de Belamy)
In October 2018, Christie’s Auction House in New York sold a computer-generated portrait of Edmond de Belamy, created in the style of 19th-century European portraiture.
2018年10月,纽约佳士得拍卖行出售了计算机生成的埃德蒙·德·贝拉米的肖像,该肖像以19世纪欧洲肖像画的风格创作。
The piece sold for $432,500, more than 40 times its original estimate.
这件作品以432,500美元的价格售出,是其原始估价的40倍以上。
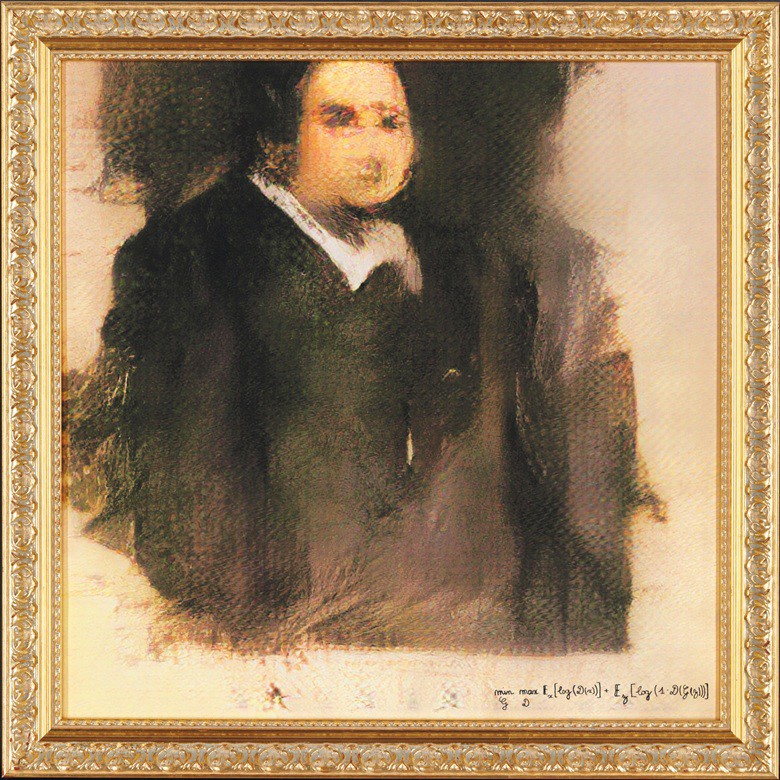
The painting (or, as art aficionados may prefer, print) is part of a collection of portraits of the fictional Belamy family, created by the French collective Obvious, which aims to explore the interface of AI with art.
这幅画(或者像艺术爱好者可能喜欢的那样,是印刷品)是虚构的Belamy家族肖像集的一部分,该肖像集是由法国集体组织Obvious创建的,旨在探索AI与艺术的界面。
As well as the seemingly unfinished, blurry and featureless portrait of Edmond Belamy himself, almost as eye-catching is the mathematical formula, in place of a signature, in the bottom right corner.
就像埃德蒙·贝拉米(Edmond Belamy)本人的看似未完成的,模糊的和毫无特色的肖像一样,右下角的数学公式几乎代替了签名,引人注目。
This formula is the loss function used by the Generative Adversarial Network (GAN) to create the portrait. This raises interesting questions about the authorship of such pieces of art. Are they truly the result of the mathematical formula, or the human who originally developed it?
此公式是Genversative Adversarial Network (GAN)用于创建肖像的损失函数 。 这就提出了有关这些艺术品的作者的有趣问题。 它们是真正的数学公式的结果,还是真正开发数学公式的人?
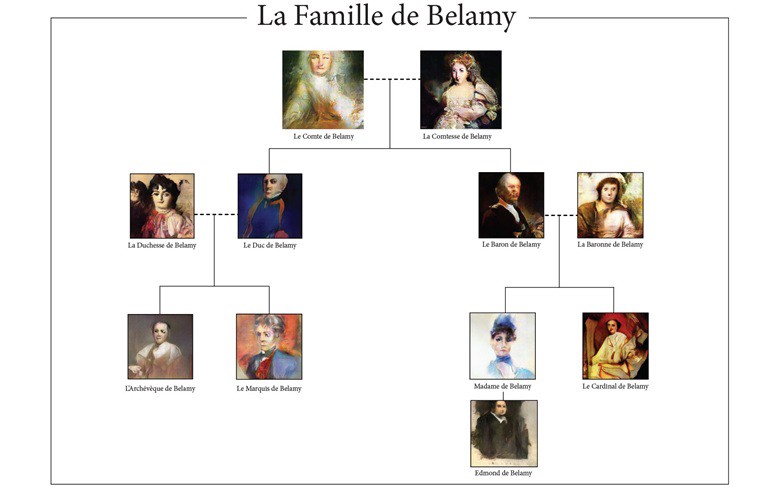
GANs are a deep learning framework containing two competing (hence the name “adversarial”) neural networks, with the aim of creating new datasets that statistically mimic the original training data.
GAN是一个深度学习框架,其中包含两个相互竞争的(因此称为“对抗性”)神经网络,目的是创建新的数据集,以统计学方式模拟原始训练数据。
The first, known as the discriminator, is fed a training set of data (in this case images) and aims to learn to discriminate this data from synthetically generated data. To create the Belamy family, Obvious trained the discriminator on 15,000 portraits produced between the 14th and 20th centuries.
第一个被称为鉴别器,被提供训练数据集(在这种情况下为图像),目的是学习从合成生成的数据中区分该数据。 为了创建Belamy家族,Obvious在14至20世纪间制作的15,000张肖像上对鉴别器进行了培训。
The second, the generator, creates an output, trying to fool the discriminator into incorrectly identifying it as part of the original data. As such, the final output is newly created data, similar enough to the original that the discriminator cannot tell it has been synthetically created.
第二个函数是生成器,它创建一个输出,试图欺骗鉴别器,将其错误地标识为原始数据的一部分。 这样,最终的输出是新创建的数据,与原始数据足够相似,因此鉴别器无法告知它是综合创建的。
Edmond de Belamy may be proof of at least one thing: that people are willing to pay for fine art developed by AI.
埃德蒙·德·贝拉米(Edmond de Belamy)至少可以证明一件事:人们愿意为AI开发的美术付出代价。
But the question remains whether Obvious successfully imitated human creativity. Considering the purpose of a GAN is to replicate its training data, it might be a stretch to argue that their outputs are truly innovative.
但是问题仍然存在,就是“显而易见”是否成功地模仿了人类的创造力。 考虑到GAN的目的是复制其训练数据,因此可能会争辩说其输出是真正的创新。
2.艾坎 (2. AICAN)
On 13th February 2019 a four-week exhibit, Faceless Portraits Transcending Time, at the HG Contemporary art gallery in Chelsea, New York, contained prints of artwork produced entirely by AICAN, an algorithm designed and written by Ahmed Elgammal, Director of the Art & AI Lab at Rutgers University. According to Elgammal,
2019年2月13日,在纽约切尔西HG当代美术馆的为期四周的展览《 无脸肖像超越时间》 ( Farless Portraits Transcending Time)包含了由AICAN (艺术和艺术总监艾哈迈德· 埃尔加马尔 ( Ahmed Elgammal)设计和编写的算法)完全由AICAN制作的艺术品印刷品罗格斯大学AI实验室。 根据Elgammal ,
AICAN [is] a program that could be thought of as a nearly autonomous artist that has learned existing styles and aesthetics and can generate innovate images of its own.
AICAN是一个程序,可以被认为是一个近乎自主的艺术家,已经学习了现有的样式和美学,并且可以生成自己的创新图像。
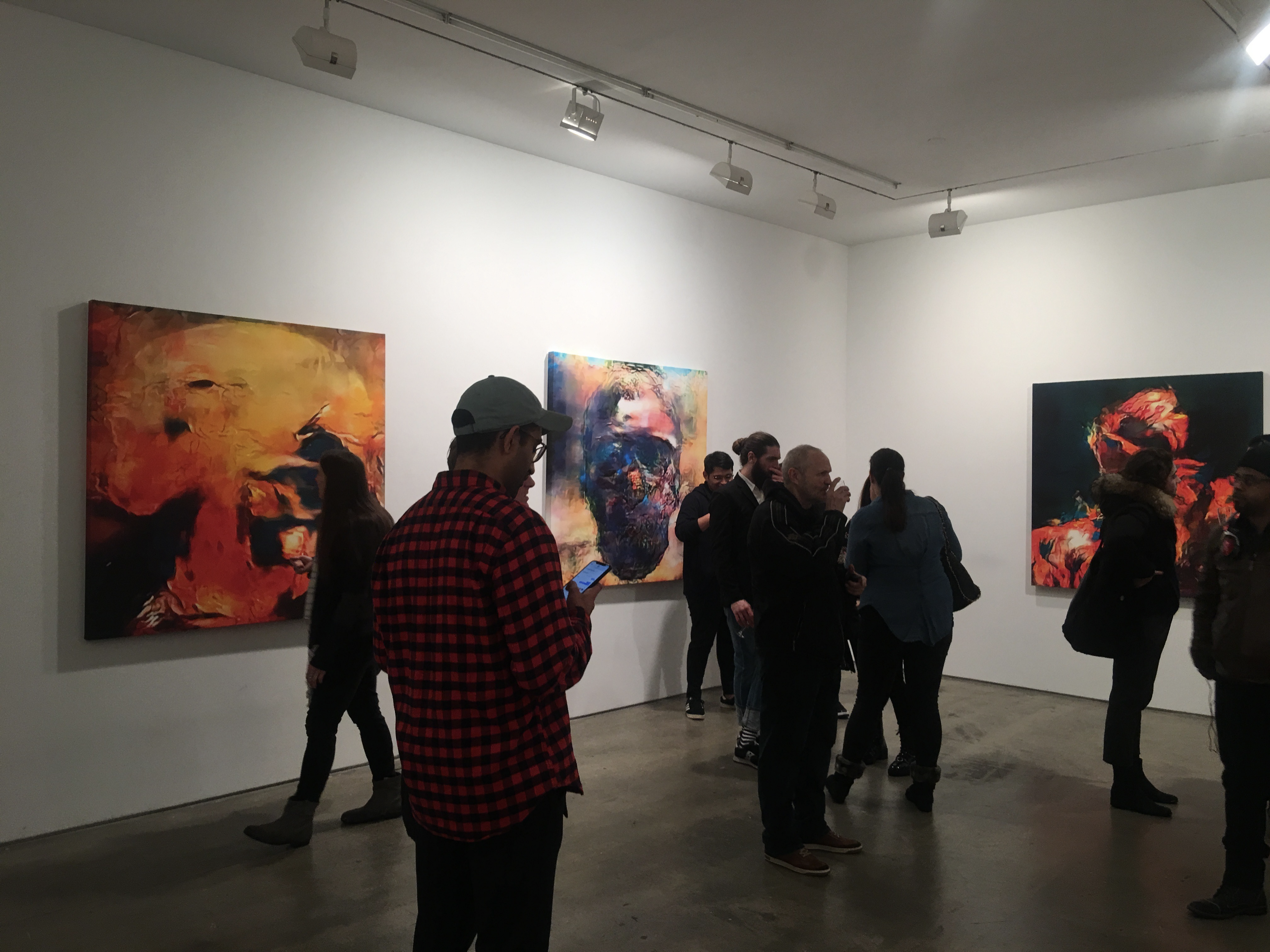
Instead of GANs, AICAN uses what Elgammal has called a “creative adversarial network” (CAN). These diverge from GANs by adding an element that penalizes the model for work that too closely matches a given established style.
AICAN代替了GAN,使用Elgammal所谓的“创意对抗网络”(CAN)。 这些元素与GAN的区别在于,添加了对模型进行惩罚的元素,这些元素对与给定既定样式过于接近的工作进行了惩罚。
Psychologist Colin Martindale hypothesizes that artists will try to increase the appeal of their work by diverging from existing artistic styles. CANs do just that: allowing a model to introduce novelty so that AICAN can diverge from existing styles.
心理学家科林·马丁代尔(Colin Martindale) 假设 ,艺术家将试图通过与现有的艺术风格有所不同来增加其作品的吸引力。 CAN就是这样做的:允许模型引入新颖性,以便AICAN可以与现有样式有所不同。
AICAN is trained on over 80,000 images of Western art over the last 5 centuries but does not focus on a specific artistic style. As well as the images themselves, the algorithm is also fed the names of the pieces, so that the output is an image along with a title, all created by AICAN.
在过去的5个世纪中,AICAN接受了80,000幅西方艺术图像的培训,但并未专注于特定的艺术风格。 除了图像本身之外,算法还获得了片段的名称,因此输出是一幅图像以及一个标题,均由AICAN创建。
More often than not, these pieces are more abstract, which Elgammal believes is because AICAN uses the most recent trends in art history, such as abstract art, to understand how best to diverge from existing styles.
这些作品通常更多地是抽象的,Elgammal 认为这是因为AICAN利用艺术史上的最新趋势(例如抽象艺术)来了解如何最好地与现有样式区分开。
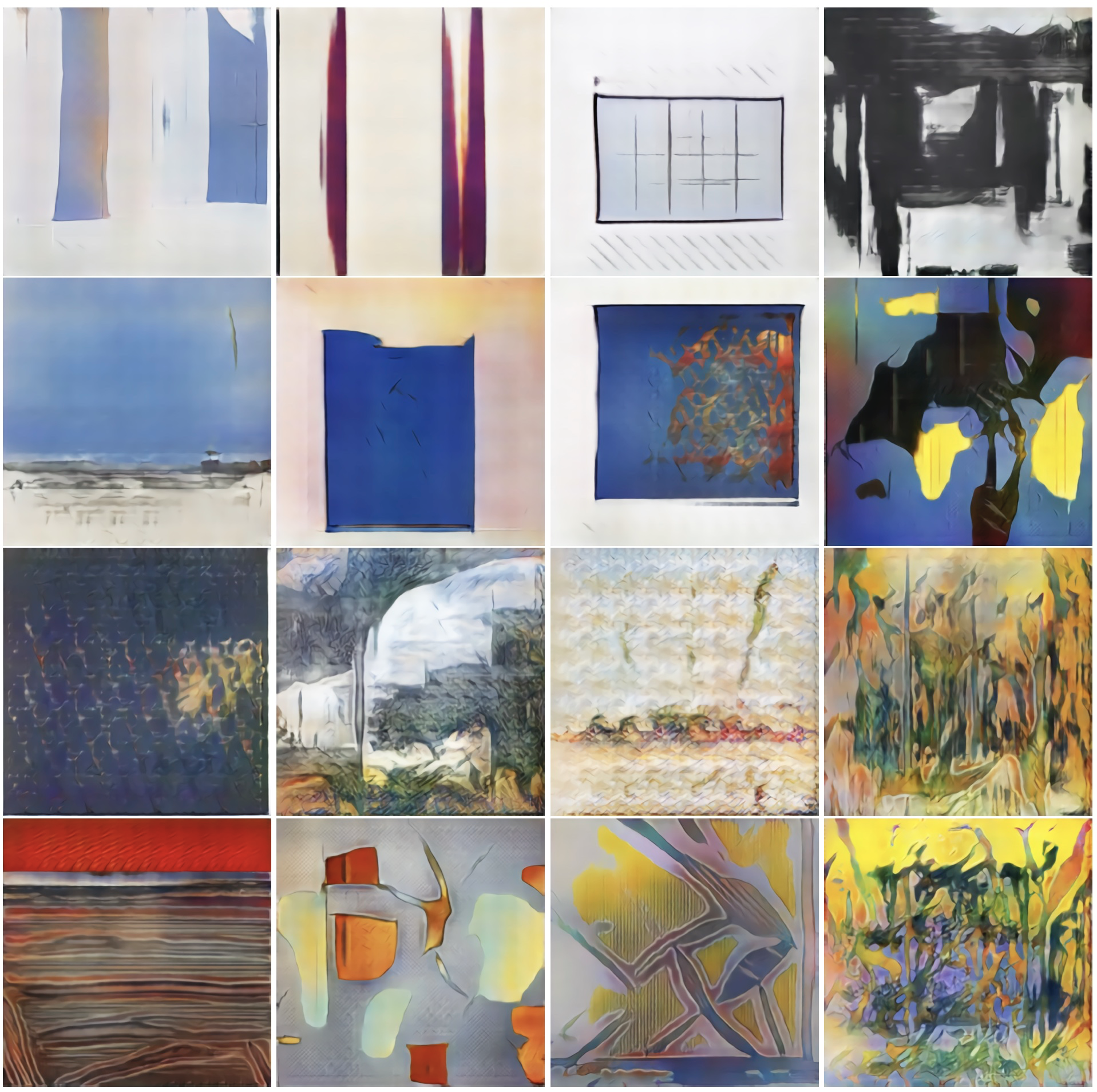
In the paper introducing CANs, two experiments were conducted on humans to ascertain whether or not they could distinguish between human and computer-generated images. Each experiment, which received 10 distinct responses, measured that humans incorrectly labeled the CAN images as produced by humans 53% and 75% of the time, respectively. This is compared to 35% and 65% for GANs.
在介绍CAN的论文中 ,对人体进行了两个实验,以确定它们是否可以区分人和计算机生成的图像。 每个实验收到10个不同的响应,测量出人类分别错误地标记了人类在53%和75%的时间内所产生的CAN图像。 相比之下,GAN分别为35%和65%。
CANs may be more successful than GANs at imitating humans. Perhaps we can finally argue that CANs succeed where GANs failed. They don’t just try to replicate a dataset—the penalty term might actually allow them to innovate.
在模仿人类方面,CAN可能比GAN更成功。 也许我们最终可以争辩说,CAN可以在GAN失败的地方成功。 他们不仅尝试复制数据集,而且惩罚性条款实际上可能允许他们进行创新。
3.音乐智力 (3. Musical intelligence)
In 1981, David Cope, a music professor at the University of California, began what he called “Experiments in Musical Intelligence” (EMI, pronounced “Emmy”).
1981年,加利福尼亚大学的音乐教授David Cope开始了他所谓的“ 音乐智能实验 ”(EMI,发音为“ Emmy”)。
According to Cope, he began these experiments as the result of composer’s block; he wanted a program that understood his overall style of music and could provide him with the next note or measure. However, he found that he had very little information about his own style and instead,
根据库普的说法,他是由于作曲家的阻挠而开始进行这些实验的。 他需要一个程序来理解他的音乐整体风格,并为他提供下一个音符或小节 。 但是,他发现自己对自己风格的了解很少,相反,
I began creating computer programs which composed complete works in the styles of various classical composers, about which I felt I knew something more concrete.
我开始创建计算机程序,这些程序以各种古典作曲家的风格构成完整的作品,对此我感到我知道更具体的东西。
So, Cope began writing EMI in Lisp, a functional programming language created in the mid-1900s. He developed it on three key principles:
因此,Cope开始用Lisp(一种在1900年代中期创建的功能性编程语言)编写EMI。 他根据三个关键原则进行了开发:
- Deconstruction — analyzing the music and separating it into parts 解构—分析音乐并将其分成几部分
- Signatures — identifying commonalities for a given composer and retaining the parts that signify their style 签名-识别给定作曲家的共性并保留表示其风格的部分
- Compatibility — recombining the pieces into a new piece 兼容性—将片段重组为新片段
After seven years of work Cope finally finished a version of EMI to imitate the style of Johann Sebastian Bach and, in a single day, it was able to compose 5,000 works in Bach’s style. Of these, Cope selected a few which were performed in Santa Cruz without informing the audience that they were not authentic works of Bach.
经过7年的工作,Cope最终完成了EMI的版本,以模仿Johann Sebastian Bach的风格,并且一天之内就可以创作出5,000幅Bach风格的作品 。 其中,科普选择了一些在圣克鲁斯(Santa Cruz)演出的作品,而没有告知观众他们不是巴赫的真实作品。
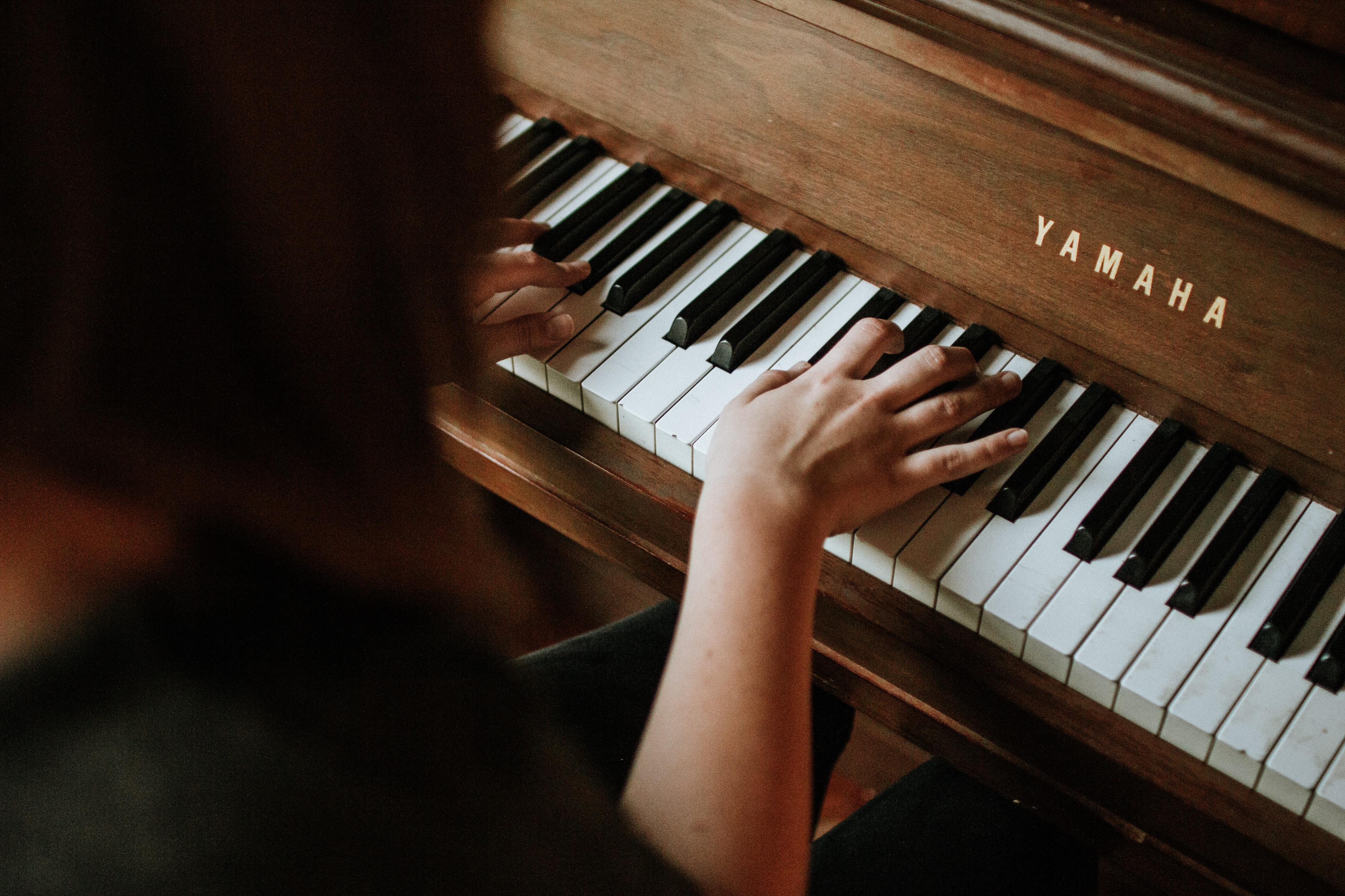
After praising the wonderful performance, the audience was told that these were created by a computer, and a significant proportion of the audience, and the wider music community, reacted with anger.
在赞扬了精彩的表演之后,听众被告知这是由计算机制作的,很大比例的听众以及更广泛的音乐界对此感到愤怒。
In particular, Professor Steve Larson from the University of Oregon proposed to Cope a challenge. In October 1997 Larson’s wife, the pianist Winifred Kerner performed three pieces of music in front of hundreds of students in the University of Oregon’s concert hall. One was composed by Bach, one by Larson and one by EMI.
俄勒冈大学的史蒂夫·拉森教授特别提出了应对挑战的建议。 1997年10月,拉尔森的妻子,钢琴家Winifred Kerner在俄勒冈大学音乐厅的数百名学生面前演奏了三首音乐。 其中一位由巴赫组成,一位由拉尔森(Larson)组成,另一位由EMI(EMI)组成。
At the end of the concert, the audience was asked to guess which piece was by which composer. To Larson’s dismay, the audience thought EMI’s piece was composed by Bach, Bach’s piece by Larson and Larson’s piece by EMI.
音乐会结束时,要求观众猜测是哪个作曲家创作的。 令拉森沮丧的是,观众们认为EMI的作品是巴赫的作品,巴赫的作品是拉森的作品,拉森的作品是EMI的作品。
This is possibly one of the most successful stories of a computer imitating human creativity. (Have a listen to some of the pieces and you will be hardpressed to notice any difference between EMI and a human composer.) However, what makes EMI great at imitation is also what makes it bad at innovation. Just like GANs, they are imitating to the detriment of innovation.
这可能是计算机模仿人类创造力最成功的故事之一。 ( 听一些文章,您将很难注意到EMI和人类作曲家之间的任何区别。)但是,使EMI在模仿方面表现出色的原因也在于在创新方面不利的原因。 就像GAN一样,它们在模仿创新。
4.伪装肖像 (4. POEMPORTRAITS)
In 2016, artist and designer Es Devlin met with Hans-Ulrich Obrist, Artistic Director of the Serpentine Galleries in London, to discuss what original and creative ideas they could come up with for the Serpentine Gala in 2017. Devlin decided to collaborate with Google Art & Culture Lab and Ross Goodwin to create POEMPORTRAITS.
2016年 ,艺术家兼设计师Es Devlin与伦敦蛇形画廊艺术总监Hans-Ulrich Obrist会面,讨论他们在2017年的蛇形艺术晚会上可以提出哪些原创和创意。Devlin决定与Google Art合作与文化实验室和罗斯古德温一起创建POEMPORTRAITS 。
POEMPORTRAITS asks users to donate a word, then uses the word to write a poem. This poem is then overlaid onto a selfie taken by the user.
POEMPORTRAITS要求用户捐赠一个单词,然后使用该单词写一首诗 。 然后将这首诗覆盖在用户拍摄的自拍上。
According to Devlin,
据德夫林说,
“the resulting poems can be surprisingly poignant, and at other times nonsensical.”
“由此产生的诗词可能令人惊讶地凄美,有时甚至是荒谬的。”
These poems are then added to an ever-growing collective poem, containing all POEMPORTRAITS’ generated poems.
然后将这些诗歌添加到不断增长的集体诗歌中,其中包含所有POEMPORTRAITS生成的诗歌。
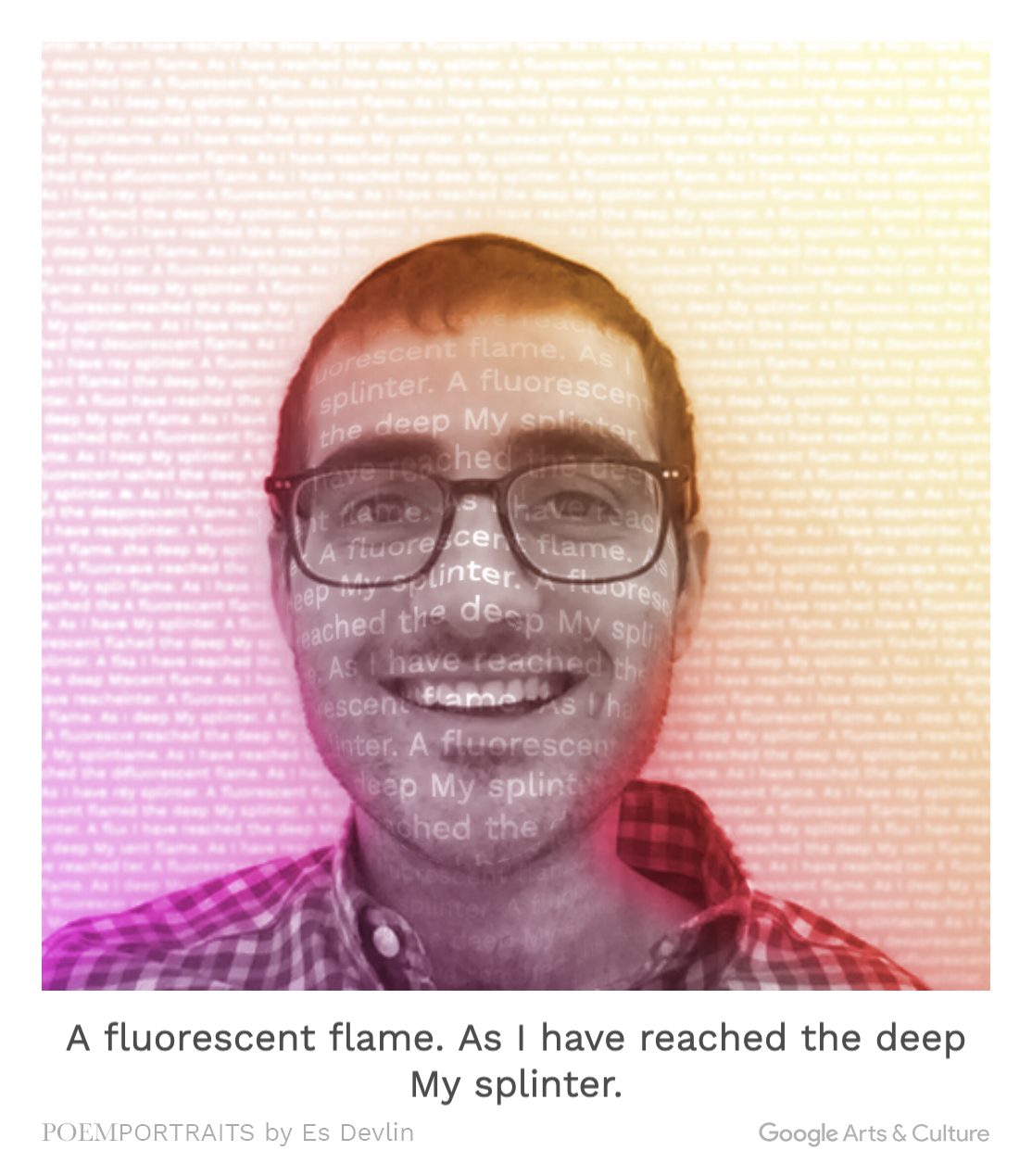
I tried it myself, donating the word ‘fluorescent’. You can see my POEMPORTRAIT above.
我自己尝试过,捐赠了“荧光灯”一词。 您可以在上方看到我的POEMPORTRAIT。
Before he collaborated with Google and Devlin, Goodwin had been experimenting with text generation. His code is available on GitHub and includes two pre-trained LSTM (Long Short-Term Memory) models for poem generation, which were used as a base for POEMPORTRAIT.
在与Google和Devlin合作之前, Goodwin一直在尝试生成文本。 他的代码可在GitHub上获得 ,其中包括两个用于诗生成的经过预先训练的LSTM( 长短期记忆 )模型,这些模型被用作POEMPORTRAIT的基础。
An LSTM is a type of recurrent neural network (RNN) that determines which word connections should be persisted further into a text to ensure the model understands the association between words.
LSTM是一种递归神经网络 (RNN),它确定哪些单词连接应进一步保留到文本中,以确保模型能够理解单词之间的关联。
For example, in the sentence “The car was great, so I decided to buy it,” the model will learn that the word ‘it’ refers to the word ‘car. This is a step beyond earlier models which only considered relations between words within a given distance of each other.
例如,在句子“汽车很棒,所以我决定购买它”中 ,模型将得知单词“ it”是指单词“ car”。 这是较早的模型的一个步骤,该模型仅考虑彼此之间给定距离内的单词之间的关系。
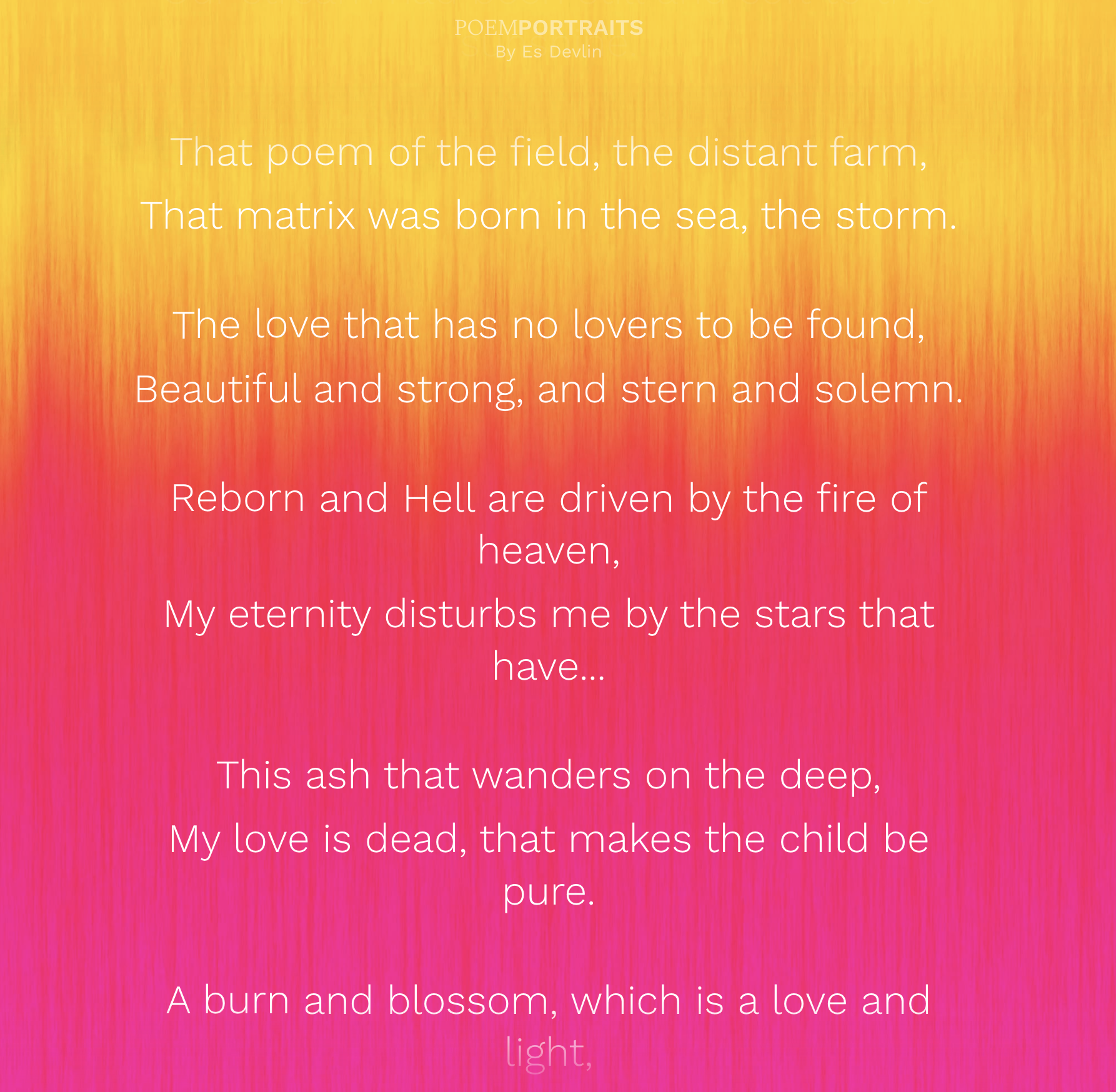
For POEMPORTRAIT, the LSTM model was trained on over 25 million words, written by 19th-century poets, to build a statistical model that essentially predicts the next word given a word or set of words. Hence, the donated word acts as a seed to which words are added, producing prose in the style of 19th-century poetry.
对于POEMPORTRAIT,LSTM模型接受了19世纪诗人撰写的超过2500万个单词的训练,从而建立了一个统计模型,该模型本质上可以预测给定一个单词或一组单词的下一个单词。 因此,捐赠的单词充当添加单词的种子,从而产生了19世纪诗歌风格的散文。
Unfortunately, there have not been any experiments on humans to qualitatively measure the effectiveness of POEMPORTRAITS at imitating human poets.
不幸的是,还没有关于人类的实验来定性地评估POEMPORTRAITS在模仿人类诗人方面的有效性。
It is clear that these are not just a random string of words, but follow (at least loosely) a set of language rules learned by the LSTM models. However, one can argue that poetry (and the same argument can be made for painting and music) is the culmination of human emotion.
显然,这些不仅仅是单词的随机字符串,而且(至少是宽松地)遵循LSTM模型学习的一组语言规则。 但是,人们可以辩称,诗歌(绘画和音乐也可以提出同样的论点)是人类情感的高潮。
5.互动图形 (5. Interactive graphics)
A group of researchers from NVIDIA released a paper in 2018 detailing Video-to-Video Synthesis, a process whereby a model generates a new video based on a training video or set of training videos.
NVIDIA(英伟达)的一组研究人员于2018年发表了一篇论文 ,详细介绍了Video-to-Video Synthesis ,该过程是模型根据训练视频或一组训练视频生成新视频的过程。
As well as making their work publicly available on their GitHub repo, an physical, interactive prototype was showcased at the NeurIPS conference in Montreal, Canada. This prototype was a simple driving simulator, in a world where the graphics had been designed entirely by a machine learning model.
除了在GitHub存储库上公开提供其工作外, 还在加拿大蒙特利尔的NeurIPS会议上展示了一个物理,交互式原型。 这个原型是一个简单的驾驶模拟器,在这个世界中,图形完全是由机器学习模型设计的。
To build this prototype they first took training data from an open-source dataset created for the training of autonomous vehicles. This dataset was then segmented into different objects (trees, cars, road, etc.) and a GAN was trained on these segments so that it could generate its own versions of these objects.
为了构建此原型,他们首先从用于训练自动驾驶汽车的开源数据集中获取了训练数据。 然后将此数据集分割为不同的对象(树木,汽车,道路等),并在这些段上对GAN进行了训练,以便可以生成自己的这些对象版本。
Using a standard game engine, Unreal Engine 4, they created a framework for their graphical world. Then, the GAN generated objects for each category of item in real-time as needed.
他们使用标准游戏引擎Unreal Engine 4创建了用于图形世界的框架。 然后,GAN根据需要实时为每个类别的项目生成对象。
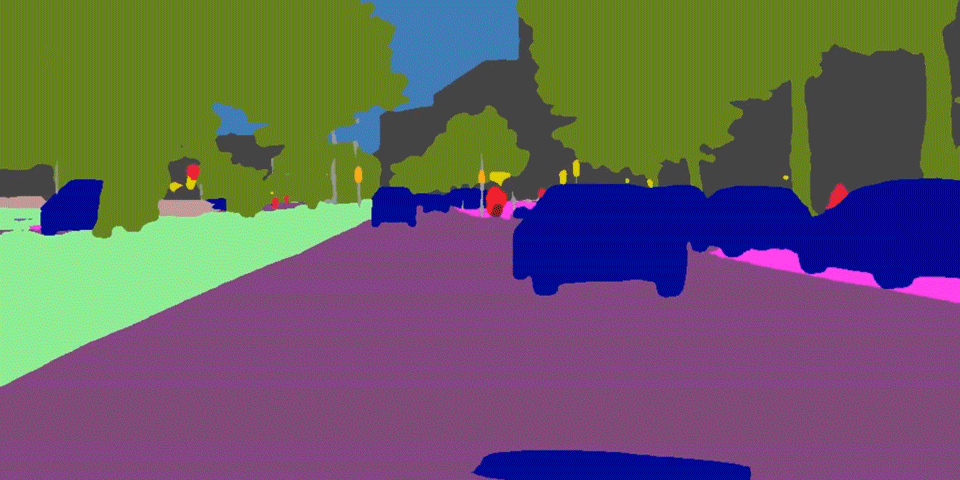
In some sense, this may seem similar to any other computer-generated image created by a GAN (or CAN). We saw two examples of these earlier in this article.
从某种意义上讲,这似乎与GAN(或CAN)创建的任何其他计算机生成的图像相似。 在本文前面的部分中,我们看到了两个示例。
However, the researchers realized that regenerating the entire world for each frame led to inconsistencies. Although a tree would appear in the same position in each frame, the image of the tree itself would change as it was being regenerated by the model.
但是,研究人员意识到,为每个帧重新生成整个世界会导致不一致。 尽管一棵树将在每个帧中出现在相同的位置,但是由于模型正在重新生成,树本身的图像将发生变化。
To solve this, the researchers added a short term memory to the model, ensuring that the objects remained somewhat consistent between frames.
为了解决这个问题,研究人员在模型中添加了一个短期记忆,以确保对象在帧之间保持一定的一致性。
Unlike all our previous example, video games may have a slightly different goal. The models don’t have to innovate in the same way an artist innovates when they create a new piece, and, generally speaking, there doesn’t need to be any emotion behind the output.
与我们之前的所有示例不同,视频游戏的目标可能会稍有不同。 这些模型不必像艺术家创作新作品时那样进行创新,通常来说,输出后不需要任何情感。
Instead, gamers will want models to depict a realistic-looking world for them to play in. However, in this case, the model was extremely computationally expensive and the demo only ran at 25 frames per second. As well as this, despite being in 2K the images display the characteristic blurriness of GAN generated images.
取而代之的是,游戏玩家希望模型能够描绘出逼真的世界供他们玩。但是,在这种情况下,模型的计算量非常大,因此演示仅以每秒25帧的速度运行。 不仅如此,尽管图像大小为2K,但仍显示GAN生成图像的特征模糊。
Unfortunately, according to Bryan Catanzaro, NVIDIA’s Vice Chairman of Applied Deep Learning, it will likely be decades before AI-produced graphics are used in consumer games.
不幸的是, 根据 NVIDIA应用深度学习副主席Bryan Catanzaro所说,在消费者游戏中使用AI生产的图形可能要几十年了。
AI is starting to contribute to all areas of the art world, as we can see from the examples above. However, the question remains as to whether these innovations are truly—well, innovative.
从上面的示例可以看出,人工智能开始为艺术世界的所有领域做出贡献。 但是,问题仍然在于这些创新是否是真正的,很好的创新。
Are these models effective imitators?
这些模型是有效的模仿者吗?
We saw in several cases, including AICAN and EMI that computers can generate outputs that fool humans. However, especially for painting, this may be limited to particular styles.
我们在包括AICAN和EMI在内的几种情况下看到,计算机可以产生愚弄人类的输出。 但是,尤其是对于绘画,这可能仅限于特定样式。
The outputs of generative models (GANs and CANs) generally do not create solid and well-defined lines, meaning images are often blurry. This can be effective for certain styles (say, abstract art) but not for others (say, portraiture).
生成模型(GAN和CAN)的输出通常不会创建实线和轮廓分明的线,这意味着图像通常很模糊。 这可能对某些样式(例如抽象艺术)有效,但对其他样式(例如肖像画)无效。
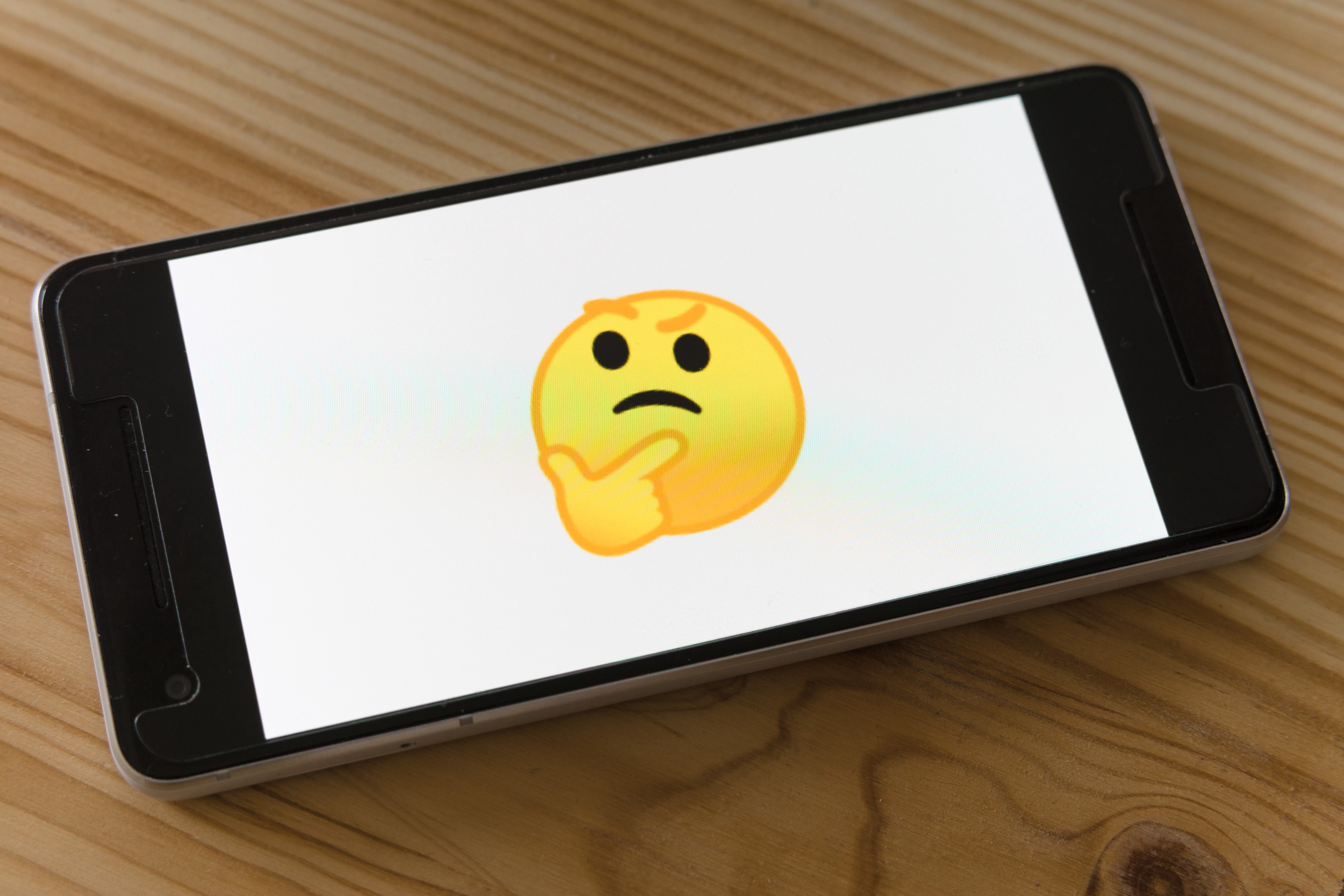
Are these models innovating?
这些模式在创新吗?
Innovation is a key characteristic of humans, but it is often hard to define. We clearly saw how CANs tried to add innovation by adapting GANs to penalize unoriginality, but one can still argue that the output is a culmination of whatever training data the model was fed.
创新是人类的关键特征,但通常很难定义。 我们清楚地看到了CAN如何通过调整GAN来惩罚原始性来尝试增加创新,但是仍然可以说输出是模型输入的任何训练数据的最终产物。
On the other hand, are humans ideas not the culmination of human past experiences, our own training data so to speak?
另一方面,人类的思想不是人类过去的经验的结晶吗?可以说我们自己的训练数据吗?
Finally, does art require human emotion?
最后,艺术需要人类的情感吗?
One thing is for certain, none of the pieces in the examples above were generated with any emotional intelligence. In mediums such as poetry and art, the story and emotion behind a piece, instilled by the author, is often what makes it resonate with others.
可以肯定的是,以上示例中的任何部分都不是由任何情商生成的。 在诗歌和艺术等媒介中,作者所灌输的作品背后的故事和情感常常使之与他人产生共鸣。
Without this emotional intelligence by the author, can a piece of art be truly appreciated by its audience?
如果没有作者的这种情感智慧,艺术品能不能真正受到观众的欣赏?
Perhaps the real question is, does it matter?
也许真正的问题是,这有关系吗?
In a world as subjective as the art world perhaps computers don’t have to definitively imitate or innovate but can find their own unique place alongside humans.
在像艺术世界这样的主观世界中,计算机不必一定要模仿或创新,而可以在人类旁边找到自己独特的地方。
If you enjoyed this, you might like another article I wrote, “Is AI Changing the Face of Modern Medicine?”.
如果喜欢这个,您可能会喜欢我写的另一篇文章,“ AI是否正在改变现代医学的面貌? ”。
翻译自: https://medium.com/swlh/is-fine-art-the-next-frontier-of-ai-64645f95bef8
ai前沿公司
本文来自互联网用户投稿,该文观点仅代表作者本人,不代表本站立场。本站仅提供信息存储空间服务,不拥有所有权,不承担相关法律责任。如若转载,请注明出处:http://www.mzph.cn/news/388651.shtml
如若内容造成侵权/违法违规/事实不符,请联系多彩编程网进行投诉反馈email:809451989@qq.com,一经查实,立即删除!