mardown 标题带数字
统计 (Statistics)
I have read a few stories on Medium about writing advice, and there were some of them which, along with other tips, suggested that putting numbers in your story’s title will increase the number of views, as people tend to be more attracted by such headlines, and therefore, more people will click on your story.
我已经阅读了有关“撰写建议”的“媒体”上的一些故事,其中有一些故事以及其他技巧建议将数字放在故事标题中会增加观看次数,因为人们通常会被此类标题吸引,因此会有更多人点击您的故事。
It seems interesting that people are attracted by such headlines. But I don’t like to take things for granted. I want to convince myself that this fact is actually true.
人们被这样的头条新闻吸引似乎很有趣。 但是我不喜欢把事情当作理所当然。 我想说服自己,这个事实确实是真的。
So, what I have been thinking? Let’s use Statistics to check if this thing is actually true. But Statistics is useless without data. I first need to obtain some data about Medium articles and use that to do hypothesis testing. Therefore, I used Python and Beautiful Soup to scrape data about a random set of 6K+ Medium articles from 7 different publications. This dataset can be found on Kaggle. If you want to see how I scraped this data, I have an article about that here:
所以,我一直在想什么? 让我们使用统计信息来检查这件事是否真的正确。 但是,如果没有数据,统计信息将无用。 我首先需要获取有关中型文章的一些数据,并使用这些数据进行假设检验。 因此,我使用Python和Beautiful Soup来刮取来自7个不同出版物的6K +中型随机文章集的数据。 该数据集可以在Kaggle上找到。 如果您想了解如何抓取这些数据,请在此处发表有关此内容的文章:
What we are going to do now is to split this dataset into 2 groups (or samples): one that has numbers in headlines and one without numbers. Then, we will do a hypothesis test on the expected value for the number of claps in these 2 groups. We use the number of claps as a measure of “how successful” a story is, although a more logical variable for our scenario would be the number of views as it is the one that is more directly affected by our choice for the title. People typically click on a story because of the preview that they see (including headline and image), and then after they read the story, they decide whether to clap or not. But, because the number of views is not publicly shown on Medium, we use the number of claps as it should be highly correlated with views (the more the views, the more likely is that someone would clap).
现在,我们要做的就是将此数据集分为2组(或样本):一组在标题上有数字,而另一组没有数字。 然后,我们将对这两组的拍手数量的期望值进行假设检验。 我们使用拍手次数来衡量故事的“成功”程度,尽管对于我们的场景而言,更合乎逻辑的变量是观看次数,因为观看次数会直接受到我们对标题的选择的影响。 人们通常会因为看到的预览(包括标题和图像)而点击故事,然后在阅读故事后决定是否拍手。 但是,由于视图的数量未在“媒体”上公开显示,因此我们使用拍手的数量,因为它应该与视图高度相关(视图越多,有人拍手的可能性就越大)。
If you are not familiar with hypothesis testing, here is an article you can read:
如果您不熟悉假设检验,则可以阅读以下文章:
That being said, we will consider the following model:
话虽如此,我们将考虑以下模型:
Sample 1: Articles with numbers in headlines
示例1:标题中带有数字的文章
We will model the number of claps inside this group as n i.i.d. (independent and identically distributed) random variables: X₁, X₂, …, Xₙ with expected value µ₁ and variance σ₁², both of which are finite.
我们将这个组中拍手的数量建模为n iid(独立且分布均匀)的随机变量:X 1,X 2,…,X 1,其期望值为μ1,方差为σ2,这两个都是有限的。
Sample 2: Articles without numbers in headlines
示例2:标题中没有数字的文章
We will model the number of claps inside this group as m i.i.d. random variables: Y₁, Y₂, …, Yₘ with expected value µ₂ and variance σ₂², both of which are finite.
我们会为m IID随机变量本组内拍手的次数型号:Y 1 Y 2,...,Yₘ与期望值μ₂和方差σ₂²,这两者都是有限的。
We formulate the null hypothesis as “articles with numbers in headlines bring no improvement over articles that have no numbers in headlines”, and the alternative hypothesis as “articles with numbers in headlines are more successful compared to articles without numbers in headlines”.
我们将无效假设表述为“标题中带有数字的文章与标题中没有数字的文章没有任何改进”,替代假设为“标题中带有数字的文章比标题中没有数字的文章更成功”。
Mathematically this means:
从数学上讲,这意味着:
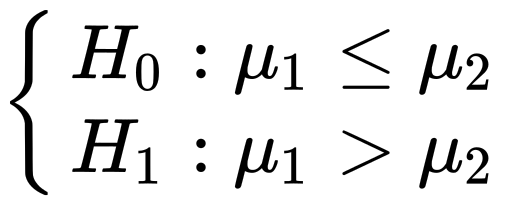
We will consider the following test statistic:
我们将考虑以下测试统计信息:
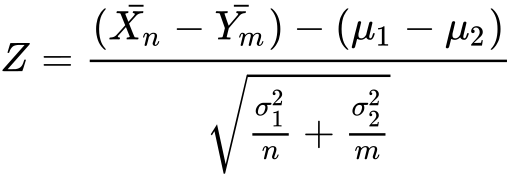
Where Xn bar and Ym bar are the averages of sample 1, respectively sample 2.
其中Xn bar和Ym bar是样本1的平均值,分别是样本2的平均值。
Because the sample sizes are pretty large and due to the Central Limit Theorem, the probability distribution of our test statistic Z can be approximated very well by a standard normal distribution, and the true variances σ₁², σ₂² should be very close to the estimated variances from our data. So, when we compute the test statistic, we can just substitute the estimated variances for σ₁², σ₂².
由于样本量非常大并且由于中心极限定理,所以我们的测试统计量Z的概率分布可以通过标准正态分布很好地近似,并且真实方差σ₁²,σ²²应该非常接近于估计的方差我们的数据。 因此,当我们计算检验统计量时,我们可以仅将估计方差替换为σ₁²,σ²²。
But, what about µ₁ - µ₂? By assuming H₀ to be true, it follows that µ₁ - µ₂ ≤ 0. And we choose µ₁ - µ₂ = 0 as this value is the worst-case scenario for the probability of type I error (we don’t want to underestimate the error).
但是,μ₁-μ2呢? 通过假设H₀为真,可得出µ₁-µ²≤0。我们选择µ₁-µ² = 0,因为该值是I型错误概率的最坏情况(我们不想低估该错误) )。
Now, let’s run some Python code. We start by importing the required packages and defining a utility function: like(x, pattern)
. This function is used to match regular expressions in pandas data frames; x
is the column, and pattern
is a regular expression. I named this function after SQL’s LIKE operator as it is meant to do something similar, but for pandas data frames.
现在,让我们运行一些Python代码。 我们首先导入所需的包并定义一个实用程序函数: like(x, pattern)
。 该函数用于匹配熊猫数据帧中的正则表达式。 x
是列,而pattern
是正则表达式。 我将此函数命名为SQL的LIKE运算符,因为它的意思是做类似的事情,但适用于熊猫数据帧。
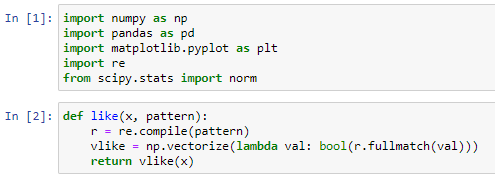
After that, we read the CSV file into a pandas data frame:
之后,我们将CSV文件读入pandas数据框中:
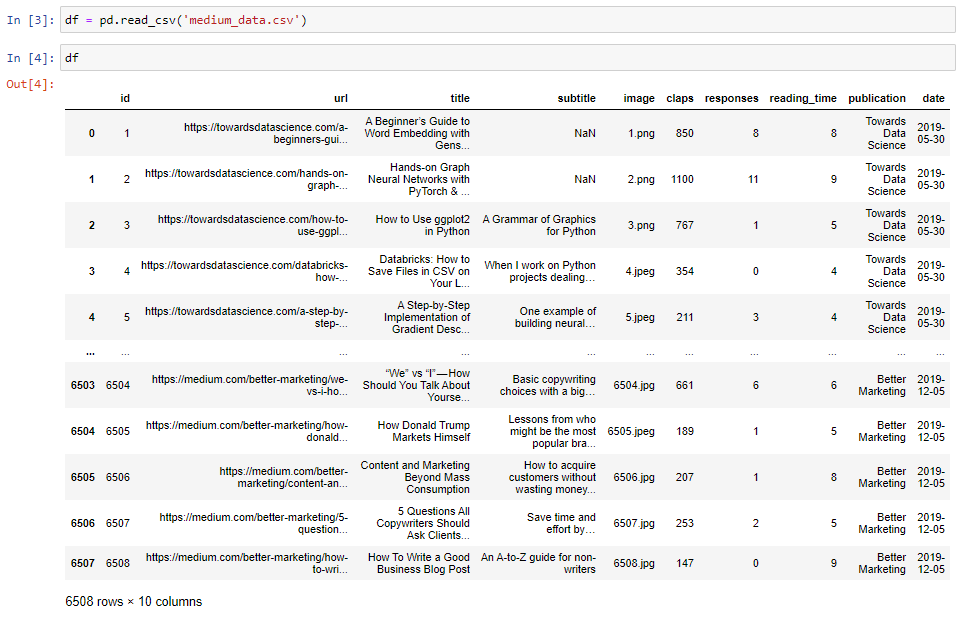
We make sure we don’t have missing values in the “title” or “claps” columns:
我们确保在“标题”或“拍子”列中没有缺失的值:
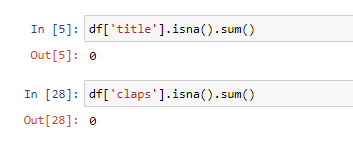
Then, we create 2 new data frames (numbers/no-numbers) using the like()
function defined earlier:
然后,我们使用前面定义的like()
函数创建2个新的数据帧(数字/无数字):

These 2 new data frames are shown below:
这两个新数据帧如下所示:
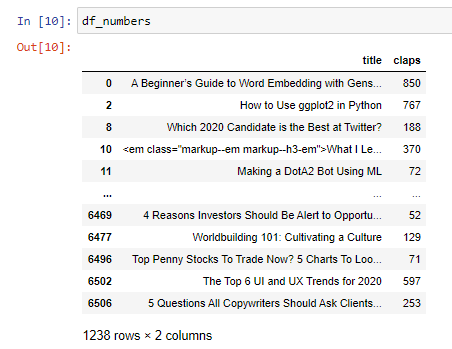
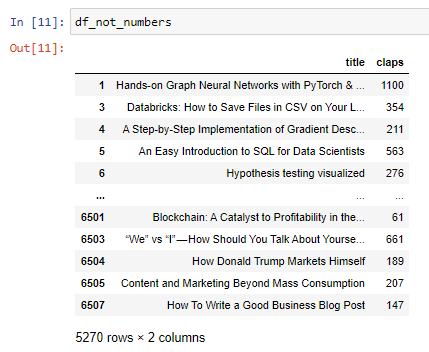
After that, we compute the quantities that we need for the test statistic:
之后,我们计算测试统计所需的数量:
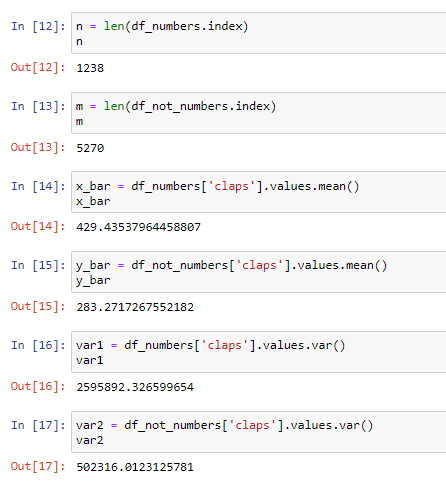
Now, we compute the test statistic and the p-value. In our case, because we’re doing a one-sided test, the p-value is the area to the right of our test statistic under a standard gaussian:
现在,我们计算检验统计量和p值。 在我们的例子中,因为我们正在进行单面测试,所以p值是标准高斯下测试统计量右侧的面积:
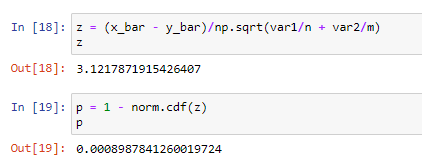
And we got a p-value much smaller than the usual threshold of 0.05. That’s good news, we can reject the null hypothesis very confidently.
而且我们得到的p值比通常的阈值0.05小得多。 这是个好消息,我们可以非常有信心地拒绝原假设。
For a significance level of α = 0.001, it follows that p ≈ 0.0009 < α, and therefore we reject the null hypothesis and accept the alternative. In plain English, this means: “We are 99.9% confident that stories with numbers in their headlines are expected to have more claps than stories without numbers in headlines”.
对于显着性水平α= 0.001,可以得出p≈0.0009 <α,因此我们拒绝零假设并接受替代假设。 用简单的英语来说,这意味着:“ 我们相信99.9%的人相信标题中带有数字的故事比标题中没有数字的故事更有拍手声 ”。
You can find the Jupyter notebook on Kaggle.
您可以在Kaggle上找到Jupyter笔记本。
Thanks for reading!
谢谢阅读!
翻译自: https://medium.com/towards-artificial-intelligence/are-stories-with-numbers-in-headlines-more-successful-b925cae2f6b4
mardown 标题带数字
本文来自互联网用户投稿,该文观点仅代表作者本人,不代表本站立场。本站仅提供信息存储空间服务,不拥有所有权,不承担相关法律责任。如若转载,请注明出处:http://www.mzph.cn/news/388645.shtml
如若内容造成侵权/违法违规/事实不符,请联系多彩编程网进行投诉反馈email:809451989@qq.com,一经查实,立即删除!