梯度下降法优化目标函数
Nowadays we can learn about domains that were usually reserved for academic communities. From Artificial Intelligence to Quantum Physics, we can browse an enormous amount of information available on the Internet and benefit from it.
如今,我们可以了解通常为学术团体保留的领域。 从人工智能到量子物理学 ,我们可以浏览互联网上大量的信息并从中受益。
However, the availability of information has some drawbacks. We need to be aware of a huge amount of unverified sources, full of factual errors (it’s a topic for the whole different discussion). What’s more, we can get used to getting answers with ease by googling it. As a result, we often take them for granted and use them without a better understanding.
但是,信息的可用性有一些缺点。 我们需要意识到大量未经验证的来源,充满事实错误(这是整个不同讨论的主题)。 而且,我们可以通过谷歌搜索来轻松地轻松获得答案。 结果,我们经常认为它们是理所当然的,并在没有更好的理解的情况下使用它们。
The process of discovering things on our own is an important part of learning. Let’s take part in such an experiment and calculate derivatives behind Gradient Descent algorithm for a Linear Regression.
独自发现事物的过程是学习的重要组成部分。 让我们参加这样的实验,并为线性回归计算梯度下降算法背后的导数。
一点介绍 (A little bit of introduction)
Linear Regression is a statistical method that can be used to model the relationship between variables [1, 2]. It’s described by a line equation:
线性回归是一种统计方法,可用于对变量之间的关系进行建模[1、2]。 它由线方程描述:

We have two parameters Θ₀ and Θ₁ and a variable x. Having data points we can find optimal parameters to fit the line to our data set.
我们有两个参数Θ₀和Θ₁和a 变量x 。 有了数据点,我们可以找到最佳参数以使线适合我们的数据集。
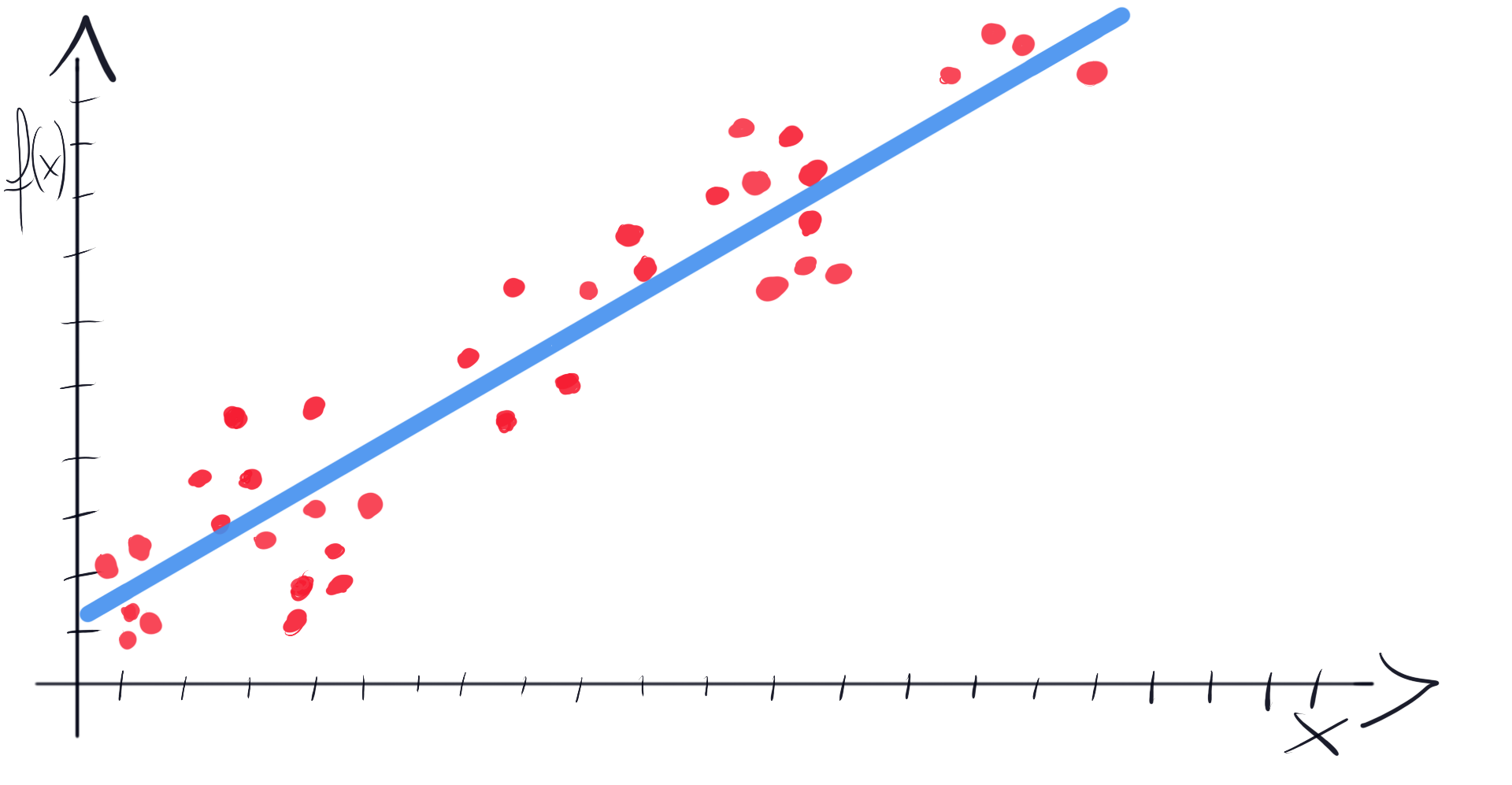
Ok, now the Gradient Descent [2, 3]. It is an iterative algorithm that is widely used in Machine Learning (in many different flavors). We can use it to automatically find optimal parameters of our line.
好的,现在是梯度下降[2,3]。 它是一种迭代算法,已在机器学习中广泛使用(有许多不同的风格)。 我们可以使用它来自动找到生产线的最佳参数。
To do this, we need to optimize an objective function defined by this formula:
为此,我们需要优化由以下公式定义的目标函数:
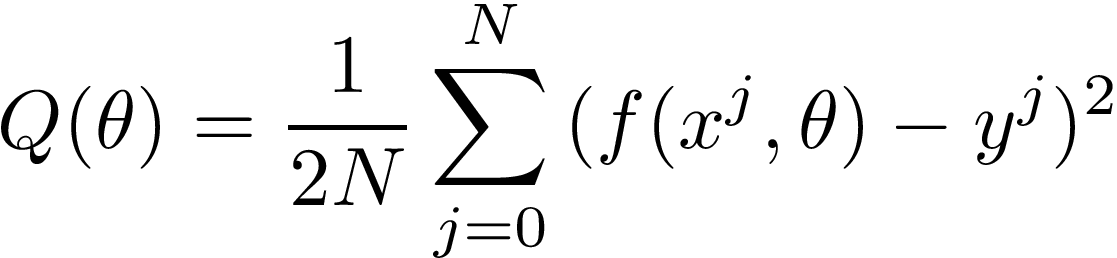
In this function, we iterate over each point (xʲ, yʲ) from our data set. Then we calculate the value of a function f for xʲ, and current theta parameters (Θ₀, Θ₁). We take a result and subtract yʲ. Finally, we square it and add it to the sum.
在此函数中,我们迭代数据集中的每个点(xʲ,yʲ) 。 然后我们计算一个函数f x的值,和当前THETA参数(Θ₀,Θ₁)。 我们得到一个结果并减去yʲ 。 最后,我们将其平方并加到总和上。
Then in the Gradient Descent formula (which updates Θ₀ and Θ₁ in each iteration), we can find these mysterious derivatives on the right side of equations:
然后,在“梯度下降”公式(每次迭代中更新Θ₀和Θ₁ )中,我们可以在等式右边找到这些神秘的导数:
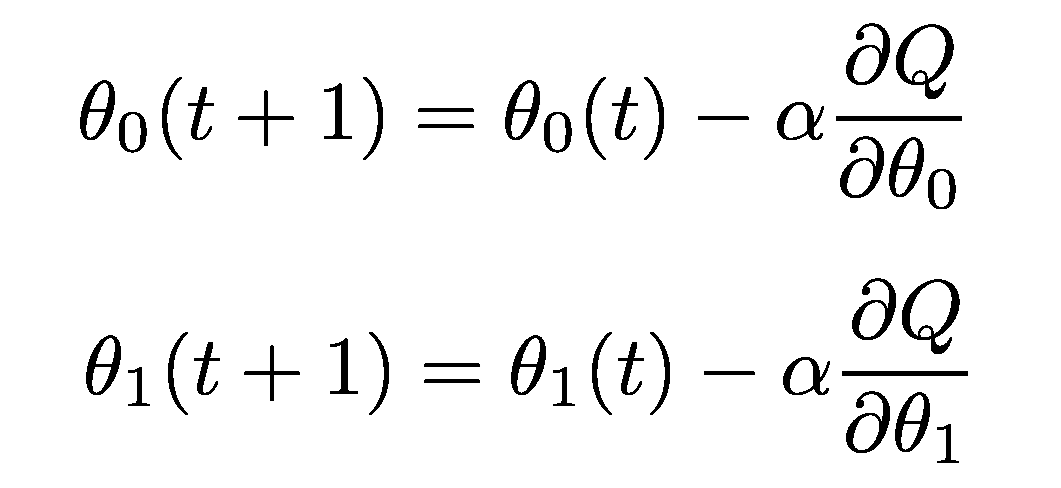
These are derivatives of the objective function Q(Θ). There are two parameters, so we need to calculate two derivatives, one for each Θ. Let’s move on and calculate them in 3 simple steps.
这些是目标函数Q(Θ)的导数。 有两个参数,因此我们需要计算两个导数,每个Θ一个 。 让我们继续并通过3个简单的步骤计算它们。
步骤1.链式规则 (Step 1. Chain Rule)
Our objective function is a composite function. We can think of it as it has an “outer” function and an “inner” function [1]. To calculate a derivative of a composite function we’ll follow a chain rule:
我们的目标函数是一个复合函数 。 我们可以认为它具有“外部”功能和“内部”功能[1]。 要计算复合函数的导数,我们将遵循一条链规则:

In our case, the “outer” part is about raising everything inside the brackets (“inner function”) to the second power. According to the rule we need to multiply the “outer function” derivative by the derivative of an “inner function”. It looks like this:
在我们的案例中, “外部”部分是关于将方括号内的所有内容( “内部功能” )提升至第二幂。 根据规则,我们需要将“外部函数”导数乘以“内部函数”的导数。 看起来像这样:

步骤2.功率规则 (Step 2. Power Rule)
The next step is calculating a derivative of a power function [1]. Let’s recall a derivative power rule formula:
下一步是计算幂函数的导数[1]。 让我们回想一下微分幂规则公式:

Our “outer function” is simply an expression raised to the second power. So we put 2 before the whole formula and leave the rest as it (2 -1 = 1, and expression raised to the first power is simply that expression).
我们的“外部功能”只是表达为第二力量的表达。 因此,我们将2放在整个公式的前面,其余部分保留为原来的值( 2 -1 = 1 ,升到第一幂的表达式就是该表达式)。
After the second step we have:
第二步之后,我们有:

We still need to calculate a derivative of an “inner function” (right side of the formula). Let’s move to the third step.
我们仍然需要计算“内部函数”的导数(公式的右侧)。 让我们转到第三步。
步骤3.常数的导数 (Step 3. The derivative of a constant)
The last rule is the simplest one. It is used to determine a derivative of a constant:
最后一条规则是最简单的规则。 用于确定常数的导数:
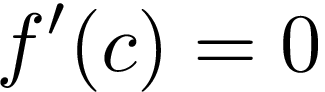
As a constant means, no changes, derivative of a constant is equal to zero [1]. For example f’(4) = 0.
作为常数,没有变化,常数的导数等于零[1]。 例如f'(4)= 0 。
Having all three rules in mind let’s break the “inner function” down:
考虑到所有三个规则,让我们分解一下“内部功能” :

The tricky part of our Gradient Descent objective function is that x is not a variable. x and y are constants that come from data set points. As we look for optimal parameters of our line, Θ₀ and Θ₁ are variables. That’s why we calculate two derivatives, one with respect to Θ₀ and one with respect to Θ₁.
梯度下降目标函数的棘手部分是x不是变量。 x和y是来自数据设置点的常数。 当我们寻找线的最佳参数时, Θ₀和Θ₁是变量。 这就是为什么我们计算两个导数,一个关于Θ₀ ,一个关于Θ₁。
Let’s start by calculating the derivative with respect to Θ₀. It means that Θ₁ will be treated as a constant.
让我们开始计算关于Θ₀的导数。 这意味着Θ₁将被视为常数。

You can see that constant parts were set to zero. What happened to Θ₀? As it’s a variable raised to the first power (a¹=a), we applied the power rule. It resulted in Θ₀ raised to the power of zero. When we raise a number to the power of zero, it’s equal to 1 (a⁰=1). And that’s it! Our derivative with respect to Θ₀ is equal to 1.
您会看到常量部分设置为零。 Θ₀怎么了? 由于它是一个提高到第一幂( a¹= a )的变量,因此我们应用了幂规则。 结果导致Θ₀提高到零的幂。 当我们将数字提高到零的幂时,它等于1( a⁰= 1 )。 就是这样! 关于Θ₀的导数等于1。
Finally, we have the whole derivative with respect to Θ₀:
最后,我们有了关于Θ₀的整个导数:

Now it’s time to calculate a derivative with respect to Θ₁. It means that we treat Θ₀ as a constant.
现在是时候来计算相对于Θ₁衍生物。 这意味着我们将Θ₀视为常数。

By analogy to the previous example, Θ₁ was treated as a variable raised to the first power. Then we applied a power rule which reduced Θ₁ to 1. However Θ₁ is multiplied by x, so we end up with derivative equal to x.
与前面的示例类似,将θ₁视为提高到第一幂的变量。 然后我们应用了一个幂规则,将Θ₁减小到1。但是Θ乘以x ,因此最终得到的导数等于x。
The final form of the derivative with respect to Θ₁ looks like this:
关于Θ₁的导数的最终形式如下:

完整的梯度下降配方 (Complete Gradient Descent recipe)
We calculated the derivatives needed by the Gradient Descent algorithm! Let’s put them where they belong:
我们计算了梯度下降算法所需的导数! 让我们将它们放在它们所属的位置:
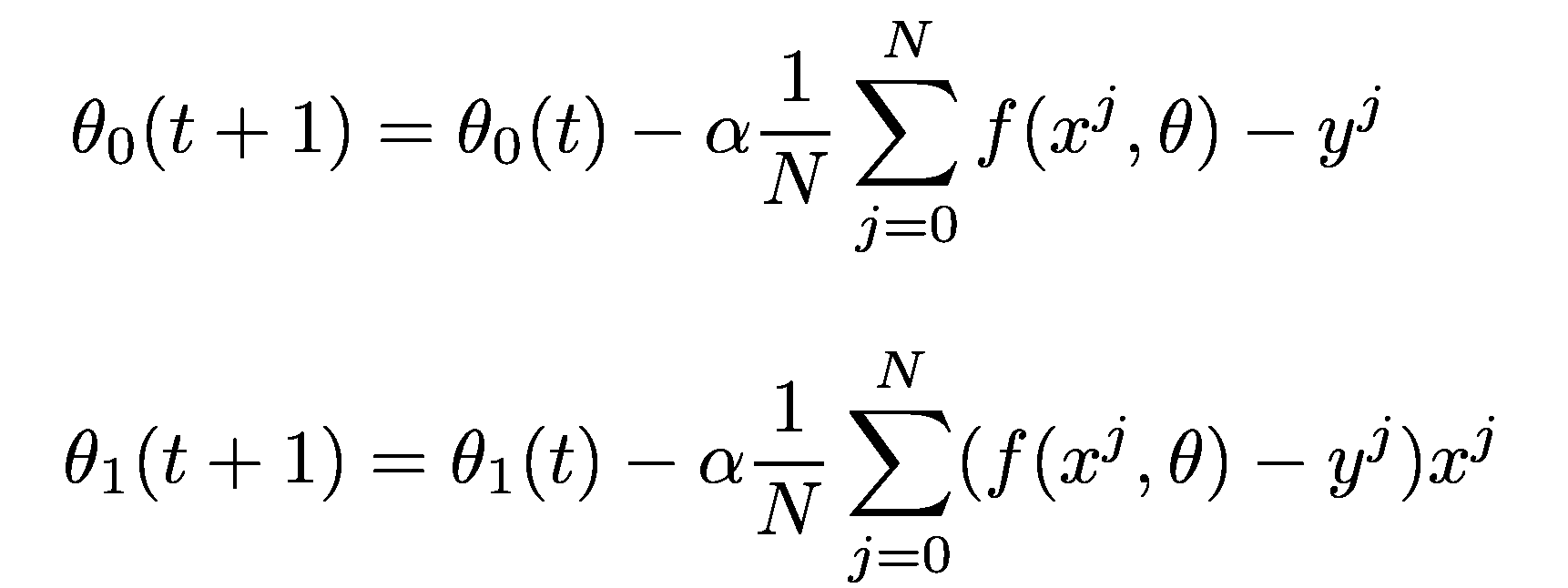
By doing this exercise we get a deeper understanding of formula origins. We don’t take it as a magic incantation we found in the old book, but instead, we actively go through the process of analyzing it. We break down the method to smaller pieces and we realize that we can finish calculations by ourselves and put it all together.
通过执行此练习,我们对公式的起源有了更深入的了解。 我们不把它当作在旧书中发现的魔咒,而是积极地进行了分析。 我们将该方法分解为较小的部分,我们意识到我们可以自己完成计算并将其组合在一起。
From time to time grab a pen and paper and solve a problem. You can find an equation or method you already successfully use and try to gain this deeper insight by decomposing it. It will give you a lot of satisfaction and spark your creativity.
时不时地拿笔和纸解决问题。 您可以找到已经成功使用的方程式或方法,并尝试通过分解来获得更深入的了解。 它将给您带来极大的满足感并激发您的创造力。
参考书目: (Bibliography:)
K.A Stroud, Dexter J. Booth, Engineering Mathematics, ISBN: 978–0831133276.
KA Stroud,Dexter J. Booth, 工程数学 ,ISBN:978–0831133276。
Joel Grus, Data Science from Scratch, 2nd Edition, ISBN: 978–1492041139
Joel Grus, Scratch的数据科学,第二版 ,ISBN:978–1492041139
Josh Patterson, Adam Gibson, Deep Learning, ISBN: 978–1491914250
Josh Patterson,Adam Gibson, 深度学习 ,ISBN:978–1491914250
翻译自: https://towardsdatascience.com/how-to-differentiate-gradient-descent-objective-function-in-3-simple-steps-b9d58567d387
梯度下降法优化目标函数
本文来自互联网用户投稿,该文观点仅代表作者本人,不代表本站立场。本站仅提供信息存储空间服务,不拥有所有权,不承担相关法律责任。如若转载,请注明出处:http://www.mzph.cn/news/388076.shtml
如若内容造成侵权/违法违规/事实不符,请联系多彩编程网进行投诉反馈email:809451989@qq.com,一经查实,立即删除!