【深度学习】实验 — 动手实现 GPT【四】:代码实现Transformer、代码实现GPT模型、训练大型语言模型(LLM)
- 在 Transformer 块中连接注意力层和线性层
- 代码实现Transformer 块
- 代码实现GPT模型
- 文本生成
- 训练模型
- 计算训练集和验证集的损失
- 训练大型语言模型(LLM)
- 测试模型
- 尝试使用 Huggingface 上的预训练模型
在 Transformer 块中连接注意力层和线性层
- 在本节中,我们将前面介绍的概念组合成一个所谓的 Transformer 块。
- 一个 Transformer 块将上一章的因果多头注意力模块与线性层和前馈神经网络结合起来,我们在前面章节中已实现过这些部分。
- 此外,Transformer 块还使用了 dropout 和短路连接。
代码实现Transformer 块
class TransformerBlock(nn.Module):def __init__(self, cfg):super().__init__()self.att = MultiHeadAttention(d_in=cfg["emb_dim"],d_out=cfg["emb_dim"],context_length=cfg["context_length"],num_heads=cfg["n_heads"],dropout=cfg["drop_rate"],qkv_bias=cfg["qkv_bias"])self.ff = FeedForward(cfg)self.norm1 = LayerNorm(cfg["emb_dim"])self.norm2 = LayerNorm(cfg["emb_dim"])self.drop_shortcut = nn.Dropout(cfg["drop_rate"])def forward(self, x):"""参数:x: torch.Tensor输入张量,形状为 (batch_size, num_tokens, emb_size)返回:torch.Tensor输出张量,形状为 (batch_size, num_tokens, emb_size)步骤:1. 对输入张量应用层归一化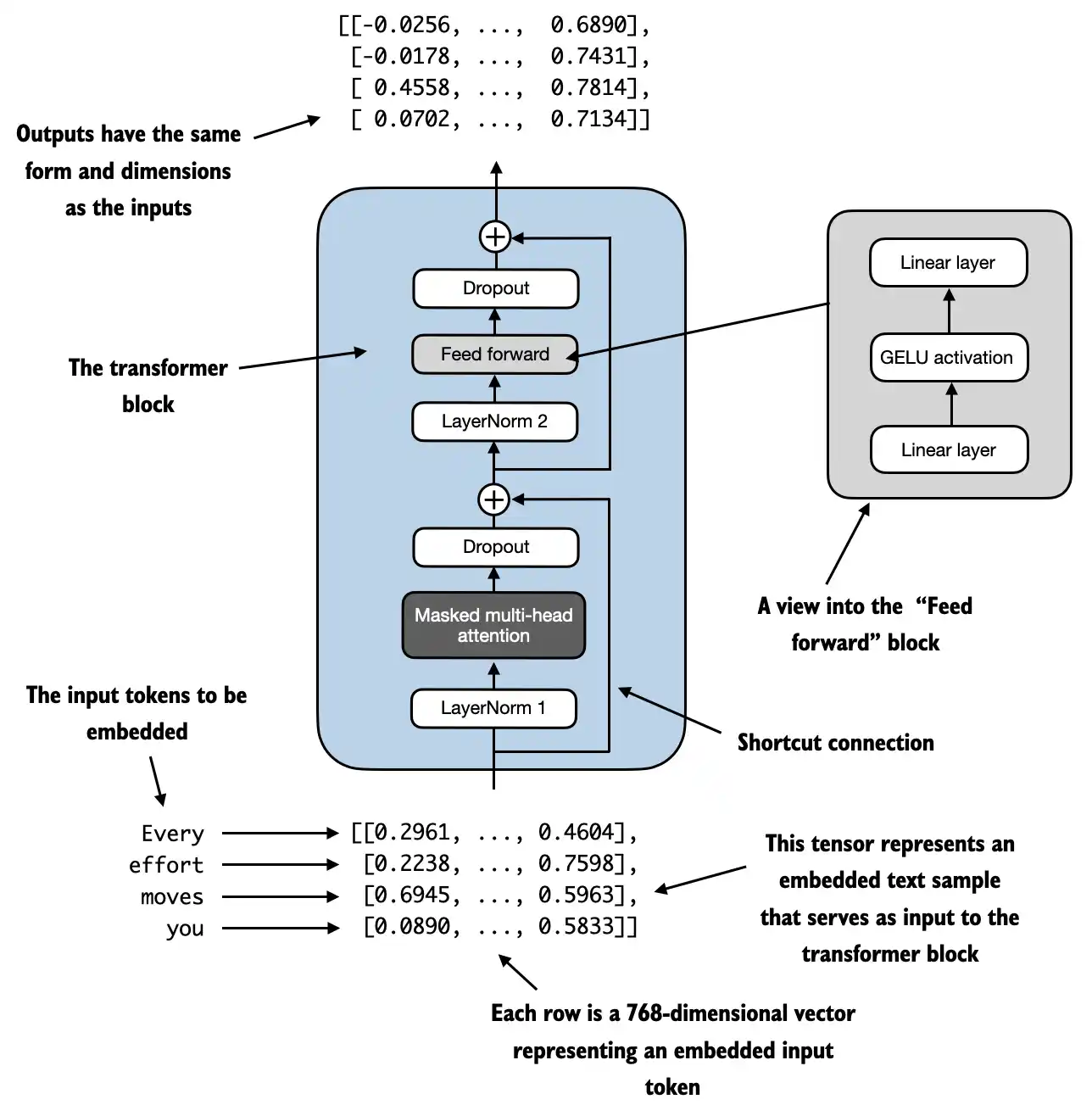
。2. 应用带有 dropout 的多头注意力块。3. 将原始输入加回(残差连接)。4. 对输出张量应用层归一化。5. 应用带有 dropout 的前馈网络块。6. 将原始输入加回(残差连接)。7. 返回输出张量。"""# complete this section (6/10)# Step 1: 对输入进行层归一化norm_x = self.norm1(x)# Step 2: 使用多头注意力机制,进行注意力计算,并应用 dropoutatt_out = self.att(norm_x)att_out = self.drop_shortcut(att_out)# Step 3: 残差连接,将输入 x 添加到注意力输出x = x + att_out# Step 4: 对残差连接后的输出再次进行层归一化norm_x = self.norm2(x)# Step 5: 使用前馈网络进行处理,并应用 dropoutff_out = self.ff(norm_x)ff_out = self.drop_shortcut(ff_out)# Step 6: 第二个残差连接,将步骤 3 的结果添加到前馈网络输出x = x + ff_out# Step 7: 返回最终输出return x
- 假设我们有 2 个输入样本,每个样本包含 6 个词元,其中每个词元是一个 768 维的嵌入向量;那么这个 Transformer 块将应用自注意力,随后是线性层,以产生相似大小的输出。
- 您可以将输出视为我们在前一章讨论的上下文向量的增强版本。
x = torch.rand(2, 4, 768) # Shape: [batch_size, num_tokens, emb_dim]
block = TransformerBlock(GPT_CONFIG_124M)
output = block(x)print("Input shape:", x.shape)
print("Output shape:", output.shape)
输出
Input shape: torch.Size([2, 4, 768])
Output shape: torch.Size([2, 4, 768])
代码实现GPT模型
- 我们快完成了:现在让我们将 Transformer 块插入到本章一开始编写的架构中,以获得一个可用的 GPT 架构。
- 注意,Transformer 块会重复多次;在最小的 1.24 亿参数的 GPT-2 模型中,我们重复该块 12 次。
- 对应的代码实现,其中
cfg["n_layers"] = 12
:
class GPTModel(nn.Module):def __init__(self, cfg):super().__init__()self.tok_emb = nn.Embedding(cfg["vocab_size"], cfg["emb_dim"])self.pos_emb = nn.Embedding(cfg["context_length"], cfg["emb_dim"])self.drop_emb = nn.Dropout(cfg["drop_rate"])self.trf_blocks = nn.Sequential(*[TransformerBlock(cfg) for _ in range(cfg["n_layers"])])self.final_norm = LayerNorm(cfg["emb_dim"])self.out_head = nn.Linear(cfg["emb_dim"], cfg["vocab_size"], bias=False)def forward(self, in_idx):"""args:in_idx: torch.TensorThe input tensor of shape (batch_size, num_tokens)returns:torch.TensorThe output tensor of shape (batch_size, num_tokens, vocab_size)Step:1. Embed the input tokens and add positional encodings2. Apply dropout to the embeddings3. Apply the transformer blocks4. Apply the final layer normalization5. Apply the output linear layer6. Return the logits"""# Step 1: 对输入 token 进行嵌入并添加位置编码batch_size, num_tokens = in_idx.size()token_embeddings = self.tok_emb(in_idx) # 形状 (batch_size, num_tokens, emb_dim)# 位置编码positions = torch.arange(0, num_tokens, device=in_idx.device).unsqueeze(0) # (1, num_tokens)position_embeddings = self.pos_emb(positions) # (1, num_tokens, emb_dim)# 将 token 嵌入和位置编码相加x = token_embeddings + position_embeddings# Step 2: 对嵌入应用 dropoutx = self.drop_emb(x)# Step 3: 通过 Transformer 块x = self.trf_blocks(x)# Step 4: 应用最后的层归一化x = self.final_norm(x)# Step 5: 应用输出线性层,得到词汇表大小的输出logits = self.out_head(x)# Step 6: 返回 logitsreturn logits
- 使用 1.24 亿参数模型的配置,我们现在可以如下实例化这个带有随机初始权重的 GPT 模型:
model = GPTModel(GPT_CONFIG_124M)import tiktoken
tokenizer = tiktoken.get_encoding("gpt2")
batch = []
txt1 = "Every effort moves you"
txt2 = "Every day holds a"
batch.append(torch.tensor(tokenizer.encode(txt1)))
batch.append(torch.tensor(tokenizer.encode(txt2)))
batch = torch.stack(batch, dim=0)out = model(batch)
print("Input batch:\n", batch)
print("\nOutput shape:", out.shape)
print(out)
输出
Input batch:tensor([[6109, 3626, 6100, 345],[6109, 1110, 6622, 257]])Output shape: torch.Size([2, 4, 50257])
tensor([[[-0.5235, -0.3854, 0.4782, ..., 0.1847, -0.4556, 0.6479],[-0.0586, -0.2504, 1.1969, ..., -0.5739, -0.4729, 0.3889],[-0.2640, -0.0780, -0.2919, ..., -0.2544, -0.4883, -0.6277],[ 0.1339, 0.2805, 0.3406, ..., 0.3529, 0.2728, 0.4377]],[[-0.5772, -0.5613, 0.2101, ..., 0.3271, -0.9243, 0.8179],[ 0.3581, 0.6702, 0.9333, ..., 0.1118, 0.0250, 0.1287],[-0.2741, -0.7146, 0.1639, ..., -0.0092, 0.5911, -0.0957],[ 0.2862, -0.6868, 0.4364, ..., 0.3579, 0.6057, 0.3257]]],grad_fn=<UnsafeViewBackward0>)
- 我们将在下一章训练此模型。
- 不过,关于其规模的一个简要说明:我们之前称其为 1.24 亿参数的模型;我们可以通过以下方式再次确认该参数数量:
total_params = sum(p.numel() for p in model.parameters())
print(f"Total number of parameters: {total_params:,}")
输出
Total number of parameters: 163,009,536
- 如上所见,这个模型实际上有 1.63 亿参数,而不是 1.24 亿参数;为什么会这样?
- 在原始的 GPT-2 论文中,研究人员应用了权重共享,即重用了词元嵌入层 (
tok_emb
) 作为输出层,这意味着设置self.out_head.weight = self.tok_emb.weight
。 - 词元嵌入层将 50,257 维的独热编码输入词元投影到 768 维的嵌入表示。
- 输出层将 768 维嵌入投影回 50,257 维的表示,以便我们可以将这些表示转换回单词(有关更多信息,请参见下一节)。
- 因此,嵌入层和输出层的权重参数数量相同,正如我们可以从其权重矩阵的形状中看到的那样。
print("Token embedding layer shape:", model.tok_emb.weight.shape)
print("Output layer shape:", model.out_head.weight.shape)
输出
Token embedding layer shape: torch.Size([50257, 768])
Output layer shape: torch.Size([50257, 768])
- 在原始的 GPT-2 论文中,研究人员将词元嵌入矩阵重用于输出矩阵。
- 相应地,如果我们减去输出层的参数数量,就会得到一个 1.24 亿参数的模型:
total_params_gpt2 = total_params - sum(p.numel() for p in model.out_head.parameters())
print(f"Number of trainable parameters considering weight tying: {total_params_gpt2:,}")
输出
Number of trainable parameters considering weight tying: 124,412,160
- 实际中,我发现不使用权重共享更容易训练模型,这也是我们在这里没有实现它的原因。
- 不过,当我们在第 5 章加载预训练权重时,会再次回顾并应用这个权重共享的概念。
- 最后,我们可以按如下方式计算模型的内存需求,这将是一个有用的参考点:
# Calculate the total size in bytes (assuming float32, 4 bytes per parameter)
total_size_bytes = total_params * 4# Convert to megabytes
total_size_mb = total_size_bytes / (1024 * 1024)print(f"Total size of the model: {total_size_mb:.2f} MB")
输出
Total size of the model: 621.83 MB
文本生成
- 像我们上面实现的 GPT 模型这样的 LLM 是逐词生成文本的。
- 以下
generate_text_simple
函数实现了贪婪解码,这是一种简单且快速的文本生成方法。 - 在贪婪解码中,每一步模型选择概率最高的词(或词元)作为下一个输出(最高的对数值对应于最高的概率,因此理论上我们甚至不需要显式计算 softmax 函数)。
- 在下一章中,我们将实现一个更高级的
generate_text
函数。 - 下图展示了 GPT 模型在给定输入上下文时如何生成下一个词元。
def generate_text_simple(model, idx, max_new_tokens, context_size):# idx is (batch, n_tokens) array of indices in the current contextfor _ in range(max_new_tokens):# Crop current context if it exceeds the supported context size# E.g., if LLM supports only 5 tokens, and the context size is 10# then only the last 5 tokens are used as contextidx_cond = idx[:, -context_size:]# Get the predictionswith torch.no_grad():logits = model(idx_cond)# Focus only on the last time step# (batch, n_tokens, vocab_size) becomes (batch, vocab_size)logits = logits[:, -1, :]# Apply softmax to get probabilitiesprobas = torch.softmax(logits, dim=-1) # (batch, vocab_size)# Get the idx of the vocab entry with the highest probability valueidx_next = torch.argmax(probas, dim=-1, keepdim=True) # (batch, 1)# Append sampled index to the running sequenceidx = torch.cat((idx, idx_next), dim=1) # (batch, n_tokens+1)return idx
- 上述
generate_text_simple
实现了一个迭代过程,每次生成一个词元。 - 让我们准备一个输入示例:
start_context = "Hello, I am"encoded = tokenizer.encode(start_context)
print("encoded:", encoded)encoded_tensor = torch.tensor(encoded).unsqueeze(0)
print("encoded_tensor.shape:", encoded_tensor.shape)
输出
encoded: [15496, 11, 314, 716]
encoded_tensor.shape: torch.Size([1, 4])
model.eval() # disable dropoutout = generate_text_simple(model=model,idx=encoded_tensor,max_new_tokens=6,context_size=GPT_CONFIG_124M["context_length"]
)print("Output:", out)
print("Output length:", len(out[0]))
输出
Output: tensor([[15496, 11, 314, 716, 19947, 28507, 10354, 32672, 21128, 10944]])
Output length: 10
- 移除批量维度并转换回文本:
decoded_text = tokenizer.decode(out.squeeze(0).tolist())
print(decoded_text)
输出
Hello, I am Rodgers swung':illin modeling derived
训练模型
计算训练集和验证集的损失
-
我们使用相对较小的数据集来训练 LLM(实际上只有一个短篇故事)。
-
原因如下:
- 您可以在没有 GPU 的笔记本电脑上在几分钟内运行代码示例。
- 训练相对快速完成(几分钟而非几周),这对教学目的很有帮助。
- 我们使用公共领域的文本,可以包含在此 GitHub 仓库中而不会违反使用权或增加仓库大小。
-
例如,Llama 2 7B 的训练需要在 A100 GPU 上使用 184,320 小时,处理 2 万亿个词元。
- 截至撰写本文时,AWS 上 8xA100 云服务器的每小时成本约为 30 美元。
- 因此,通过粗略计算,训练这个 LLM 的成本约为 184,320 / 8 * 30 美元 = 690,000 美元。
import os
import urllib.requestfile_path = "the-verdict.txt"
url = "https://raw.githubusercontent.com/rasbt/LLMs-from-scratch/main/ch02/01_main-chapter-code/the-verdict.txt"if not os.path.exists(file_path):with urllib.request.urlopen(url) as response:text_data = response.read().decode('utf-8')with open(file_path, "w", encoding="utf-8") as file:file.write(text_data)
else:with open(file_path, "r", encoding="utf-8") as file:text_data = file.read()
total_characters = len(text_data)
total_tokens = len(tokenizer.encode(text_data))print("Characters:", total_characters)
print("Tokens:", total_tokens)
输出
Characters: 20479
Tokens: 5145
- 接下来,我们将数据集划分为训练集和验证集,并使用数据加载器为 LLM 训练准备批次数据。
- 出于可视化的目的,下面的图示假设
max_length=6
,但对于训练加载器,我们将max_length
设置为 LLM 支持的上下文长度。 - 为简化表示,下图仅显示输入词元:
- 由于我们训练 LLM 预测文本中的下一个词,目标与这些输入相同,只是目标向右偏移了一个位置。
GPT_CONFIG_124M = {"vocab_size": 50257, # Vocabulary size"context_length": 256, # Shortened context length (orig: 1024)"emb_dim": 768, # Embedding dimension"n_heads": 12, # Number of attention heads"n_layers": 12, # Number of layers"drop_rate": 0.1, # Dropout rate"qkv_bias": False # Query-key-value bias
}
# Train/validation ratio
train_ratio = 0.90
split_idx = int(train_ratio * len(text_data))
train_data = text_data[:split_idx]
val_data = text_data[split_idx:]train_loader = create_dataloader_v1(train_data,batch_size=2,max_length=GPT_CONFIG_124M["context_length"],stride=GPT_CONFIG_124M["context_length"],drop_last=True,shuffle=True,num_workers=0
)val_loader = create_dataloader_v1(val_data,batch_size=2,max_length=GPT_CONFIG_124M["context_length"],stride=GPT_CONFIG_124M["context_length"],drop_last=False,shuffle=False,num_workers=0
)
# Sanity checkif total_tokens * (train_ratio) < GPT_CONFIG_124M["context_length"]:print("Not enough tokens for the training loader. ""Try to lower the `GPT_CONFIG_124M['context_length']` or ""increase the `training_ratio`")if total_tokens * (1-train_ratio) < GPT_CONFIG_124M["context_length"]:print("Not enough tokens for the validation loader. ""Try to lower the `GPT_CONFIG_124M['context_length']` or ""decrease the `training_ratio`")
- 可选的检查步骤,以确认数据是否正确加载:
print("Train loader:")
for x, y in train_loader:print(x.shape, y.shape)print("\nValidation loader:")
for x, y in val_loader:print(x.shape, y.shape)
输出
Train loader:
torch.Size([2, 256]) torch.Size([2, 256])
torch.Size([2, 256]) torch.Size([2, 256])
torch.Size([2, 256]) torch.Size([2, 256])
torch.Size([2, 256]) torch.Size([2, 256])
torch.Size([2, 256]) torch.Size([2, 256])
torch.Size([2, 256]) torch.Size([2, 256])
torch.Size([2, 256]) torch.Size([2, 256])
torch.Size([2, 256]) torch.Size([2, 256])
torch.Size([2, 256]) torch.Size([2, 256])Validation loader:
torch.Size([2, 256]) torch.Size([2, 256])
- 另一个可选的检查步骤,用于确认词元大小是否在预期范围内:
train_tokens = 0
for input_batch, target_batch in train_loader:train_tokens += input_batch.numel()val_tokens = 0
for input_batch, target_batch in val_loader:val_tokens += input_batch.numel()print("Training tokens:", train_tokens)
print("Validation tokens:", val_tokens)
print("All tokens:", train_tokens + val_tokens)
输出
Training tokens: 4608
Validation tokens: 512
All tokens: 5120
- 接下来,我们实现一个实用函数来计算给定批次的交叉熵损失。
- 另外,我们实现第二个实用函数,用于计算数据加载器中用户指定批次数的损失。
def calc_loss_batch(input_batch, target_batch, model, device):"""args:input_batch: torch.TensorThe input tensor of shape (batch_size, num_tokens)target_batch: torch.TensorThe target tensor of shape (batch_size, num_tokens)model: nn.ModuleThe transformer modeldevice: strThe device typereturns:torch.TensorThe loss valueStep:1. Move the input and target batch to the device2. Forward pass the model3. Compute the cross-entropy loss4. Return the loss"""# Step 1: 将输入和目标批次移动到设备上input_batch, target_batch = input_batch.to(device), target_batch.to(device)# Step 2: 前向传播计算 logitslogits = model(input_batch) # logits 的形状: (batch_size, num_tokens, vocab_size)# complete this section (8/10) tips: use function torch.nn.functional.cross_entropyimport torch.nn.functional as F# Step 3: 计算交叉熵损失# 需要将 logits 变成形状 (batch_size * num_tokens, vocab_size)# 将 target_batch 变成 (batch_size * num_tokens)loss = F.cross_entropy(logits.view(-1, logits.size(-1)), # 展平成形状 (batch_size * num_tokens, vocab_size)target_batch.view(-1) # 展平成形状 (batch_size * num_tokens))# Step 4: 返回损失return lossdef calc_loss_loader(data_loader, model, device, num_batches=None):total_loss = 0.if len(data_loader) == 0:return float("nan")elif num_batches is None:num_batches = len(data_loader)else:# Reduce the number of batches to match the total number of batches in the data loader# if num_batches exceeds the number of batches in the data loadernum_batches = min(num_batches, len(data_loader))for i, (input_batch, target_batch) in enumerate(data_loader):if i < num_batches:loss = calc_loss_batch(input_batch, target_batch, model, device)total_loss += loss.item()else:breakreturn total_loss / num_batches
- 如果您有支持 CUDA 的 GPU 设备,无需更改代码,LLM 将在 GPU 上进行训练。
- 通过
device
设置,我们可以确保数据加载到与 LLM 模型相同的设备上。
device = torch.device("cuda" if torch.cuda.is_available() else "cpu")
model.to(device) # no assignment model = model.to(device) necessary for nn.Module classeswith torch.no_grad(): # Disable gradient tracking for efficiency because we are not training, yettrain_loss = calc_loss_loader(train_loader, model, device)val_loss = calc_loss_loader(val_loader, model, device)print("Training loss:", train_loss)
print("Validation loss:", val_loss)
输出
Training loss: 10.980463345845541
Validation loss: 10.958412170410156
训练大型语言模型(LLM)
- 最后,我们实现 LLM 的训练代码。
from tqdm import tqdmdef train_model_simple(model, train_loader, val_loader, optimizer, device, num_epochs,eval_freq, eval_iter, start_context, tokenizer):# Initialize lists to track losses and tokens seentrain_losses, val_losses, track_tokens_seen = [], [], []tokens_seen, global_step = 0, -1# Main training loopfor epoch in tqdm(range(num_epochs)):model.train() # Set model to training modefor input_batch, target_batch in train_loader:"""implement forward pass and backward pass1. Zero the gradients of the optimizer2. Calculate the loss for the batch (tips: base on func calc_loss_loader)3. Calculate the gradients of the loss4. Update the model weights using the optimizer"""# complete this section (9/10)###################tokens_seen += input_batch.numel()global_step += 1# Optional evaluation stepif global_step % eval_freq == 0:train_loss, val_loss = evaluate_model(model, train_loader, val_loader, device, eval_iter)train_losses.append(train_loss)val_losses.append(val_loss)track_tokens_seen.append(tokens_seen)logging.info(f"Ep {epoch+1} (Step {global_step:06d}): "f"Train loss {train_loss:.3f}, Val loss {val_loss:.3f}")# Print a sample text after each epochgenerate_and_print_sample(model, tokenizer, device, start_context)return train_losses, val_losses, track_tokens_seendef evaluate_model(model, train_loader, val_loader, device, eval_iter):model.eval()with torch.no_grad():train_loss = calc_loss_loader(train_loader, model, device, num_batches=eval_iter)val_loss = calc_loss_loader(val_loader, model, device, num_batches=eval_iter)model.train()return train_loss, val_lossdef text_to_token_ids(text, tokenizer):encoded = tokenizer.encode(text, allowed_special={'<|endoftext|>'})encoded_tensor = torch.tensor(encoded).unsqueeze(0) # add batch dimensionreturn encoded_tensordef token_ids_to_text(token_ids, tokenizer):flat = token_ids.squeeze(0) # remove batch dimensionreturn tokenizer.decode(flat.tolist())def generate_and_print_sample(model, tokenizer, device, start_context):model.eval()context_size = model.pos_emb.weight.shape[0]encoded = text_to_token_ids(start_context, tokenizer).to(device)with torch.no_grad():token_ids = generate_text_simple(model=model, idx=encoded,max_new_tokens=50, context_size=context_size)decoded_text = token_ids_to_text(token_ids, tokenizer)print(decoded_text.replace("\n", " ")) # Compact print formatmodel.train()
- 现在,让我们使用上面定义的训练函数来训练 LLM:
model = GPTModel(GPT_CONFIG_124M)
model.to(device)
optimizer = torch.optim.AdamW(model.parameters(), lr=0.0004, weight_decay=0.1)num_epochs = 10
train_losses, val_losses, tokens_seen = train_model_simple(model, train_loader, val_loader, optimizer, device,num_epochs=num_epochs, eval_freq=5, eval_iter=5,start_context="Every effort moves you", tokenizer=tokenizer
)
输出
0%| | 0/10 [00:00<?, ?it/s]2024-10-31 00:53:15 -INFO: Ep 1 (Step 000000): Train loss 11.004, Val loss 11.026
2024-10-31 00:53:15 -INFO: Ep 1 (Step 000005): Train loss 10.990, Val loss 11.02610%|█ | 1/10 [00:00<00:08, 1.01it/s]2024-10-31 00:53:16 -INFO: Ep 2 (Step 000010): Train loss 10.985, Val loss 11.026
Every effort moves youOTH greedy Strauss repairrawdownloadcloneembedreportprintconnected ka googleLife Militiathreadicks� selfie studying22 renamed authenticationunin Mead actively crossings Explicit Hospitalbd charsSuddenly Containerva grandparents WORLD HuffPostUh drilled Certified cancel Celtic Suffolk Speed where EskEduc Phase << Barton Contin STEMtheneum Shame
2024-10-31 00:53:16 -INFO: Ep 2 (Step 000015): Train loss 10.986, Val loss 11.02620%|██ | 2/10 [00:01<00:07, 1.05it/s]2024-10-31 00:53:17 -INFO: Ep 3 (Step 000020): Train loss 11.001, Val loss 11.026
Every effort moves youOTH greedy Strauss repairrawdownloadcloneembedreportprintconnected ka googleLife Militiathreadicks� selfie studying22 renamed authenticationunin Mead actively crossings Explicit Hospitalbd charsSuddenly Containerva grandparents WORLD HuffPostUh drilled Certified cancel Celtic Suffolk Speed where EskEduc Phase << Barton Contin STEMtheneum Shame
2024-10-31 00:53:17 -INFO: Ep 3 (Step 000025): Train loss 10.984, Val loss 11.02630%|███ | 3/10 [00:02<00:06, 1.06it/s]2024-10-31 00:53:18 -INFO: Ep 4 (Step 000030): Train loss 11.004, Val loss 11.026
Every effort moves youOTH greedy Strauss repairrawdownloadcloneembedreportprintconnected ka googleLife Militiathreadicks� selfie studying22 renamed authenticationunin Mead actively crossings Explicit Hospitalbd charsSuddenly Containerva grandparents WORLD HuffPostUh drilled Certified cancel Celtic Suffolk Speed where EskEduc Phase << Barton Contin STEMtheneum Shame
2024-10-31 00:53:18 -INFO: Ep 4 (Step 000035): Train loss 10.990, Val loss 11.02640%|████ | 4/10 [00:03<00:05, 1.06it/s]2024-10-31 00:53:19 -INFO: Ep 5 (Step 000040): Train loss 10.998, Val loss 11.026
Every effort moves youOTH greedy Strauss repairrawdownloadcloneembedreportprintconnected ka googleLife Militiathreadicks� selfie studying22 renamed authenticationunin Mead actively crossings Explicit Hospitalbd charsSuddenly Containerva grandparents WORLD HuffPostUh drilled Certified cancel Celtic Suffolk Speed where EskEduc Phase << Barton Contin STEMtheneum Shame50%|█████ | 5/10 [00:04<00:04, 1.14it/s]2024-10-31 00:53:20 -INFO: Ep 6 (Step 000045): Train loss 11.001, Val loss 11.026
Every effort moves youOTH greedy Strauss repairrawdownloadcloneembedreportprintconnected ka googleLife Militiathreadicks� selfie studying22 renamed authenticationunin Mead actively crossings Explicit Hospitalbd charsSuddenly Containerva grandparents WORLD HuffPostUh drilled Certified cancel Celtic Suffolk Speed where EskEduc Phase << Barton Contin STEMtheneum Shame
2024-10-31 00:53:20 -INFO: Ep 6 (Step 000050): Train loss 10.982, Val loss 11.02660%|██████ | 6/10 [00:05<00:03, 1.11it/s]2024-10-31 00:53:21 -INFO: Ep 7 (Step 000055): Train loss 11.002, Val loss 11.026
Every effort moves youOTH greedy Strauss repairrawdownloadcloneembedreportprintconnected ka googleLife Militiathreadicks� selfie studying22 renamed authenticationunin Mead actively crossings Explicit Hospitalbd charsSuddenly Containerva grandparents WORLD HuffPostUh drilled Certified cancel Celtic Suffolk Speed where EskEduc Phase << Barton Contin STEMtheneum Shame
2024-10-31 00:53:21 -INFO: Ep 7 (Step 000060): Train loss 10.989, Val loss 11.02670%|███████ | 7/10 [00:06<00:02, 1.08it/s]2024-10-31 00:53:22 -INFO: Ep 8 (Step 000065): Train loss 10.980, Val loss 11.026
Every effort moves youOTH greedy Strauss repairrawdownloadcloneembedreportprintconnected ka googleLife Militiathreadicks� selfie studying22 renamed authenticationunin Mead actively crossings Explicit Hospitalbd charsSuddenly Containerva grandparents WORLD HuffPostUh drilled Certified cancel Celtic Suffolk Speed where EskEduc Phase << Barton Contin STEMtheneum Shame
2024-10-31 00:53:22 -INFO: Ep 8 (Step 000070): Train loss 10.999, Val loss 11.02680%|████████ | 8/10 [00:07<00:01, 1.08it/s]2024-10-31 00:53:22 -INFO: Ep 9 (Step 000075): Train loss 10.993, Val loss 11.026
Every effort moves youOTH greedy Strauss repairrawdownloadcloneembedreportprintconnected ka googleLife Militiathreadicks� selfie studying22 renamed authenticationunin Mead actively crossings Explicit Hospitalbd charsSuddenly Containerva grandparents WORLD HuffPostUh drilled Certified cancel Celtic Suffolk Speed where EskEduc Phase << Barton Contin STEMtheneum Shame
2024-10-31 00:53:23 -INFO: Ep 9 (Step 000080): Train loss 10.989, Val loss 11.02690%|█████████ | 9/10 [00:08<00:00, 1.08it/s]2024-10-31 00:53:23 -INFO: Ep 10 (Step 000085): Train loss 10.990, Val loss 11.026
Every effort moves youOTH greedy Strauss repairrawdownloadcloneembedreportprintconnected ka googleLife Militiathreadicks� selfie studying22 renamed authenticationunin Mead actively crossings Explicit Hospitalbd charsSuddenly Containerva grandparents WORLD HuffPostUh drilled Certified cancel Celtic Suffolk Speed where EskEduc Phase << Barton Contin STEMtheneum Shame
100%|██████████| 10/10 [00:09<00:00, 1.10it/s]
Every effort moves youOTH greedy Strauss repairrawdownloadcloneembedreportprintconnected ka googleLife Militiathreadicks� selfie studying22 renamed authenticationunin Mead actively crossings Explicit Hospitalbd charsSuddenly Containerva grandparents WORLD HuffPostUh drilled Certified cancel Celtic Suffolk Speed where EskEduc Phase << Barton Contin STEMtheneum Shame
import matplotlib.pyplot as plt
from matplotlib.ticker import MaxNLocatordef plot_losses(epochs_seen, tokens_seen, train_losses, val_losses):fig, ax1 = plt.subplots(figsize=(5, 3))# Plot training and validation loss against epochsax1.plot(epochs_seen, train_losses, label="Training loss")ax1.plot(epochs_seen, val_losses, linestyle="-.", label="Validation loss")ax1.set_xlabel("Epochs")ax1.set_ylabel("Loss")ax1.legend(loc="upper right")ax1.xaxis.set_major_locator(MaxNLocator(integer=True)) # only show integer labels on x-axis# Create a second x-axis for tokens seenax2 = ax1.twiny() # Create a second x-axis that shares the same y-axisax2.plot(tokens_seen, train_losses, alpha=0) # Invisible plot for aligning ticksax2.set_xlabel("Tokens seen")fig.tight_layout() # Adjust layout to make roomplt.savefig("loss-plot.pdf")plt.show()epochs_tensor = torch.linspace(0, num_epochs, len(train_losses))
plot_losses(epochs_tensor, tokens_seen, train_losses, val_losses)
输出
测试模型
input_text = "I love XMU, "
generate_and_print_sample(model, tokenizer, device, input_text)
输出
I love XMU, vinyl cascade 1969 Alec POST noun Adds147UTF Influence checklistiring abstract Hive arrangement hundred PsyNet periphery sleeper $_encKevin magn wantEducationmeta scraping grou%%%%handled happ savingswornrub guards AerDDween regrettregate server cornerstone procedures downward王uben deer hellMPPHOTOS
尝试使用 Huggingface 上的预训练模型
from transformers import pipeline
generator = pipeline('text-generation', model='gpt2-large', device='cuda')