Llama3-Tutorial之XTuner微调Llama3图片理解多模态
基于 Llama3-8B-Instruct 和 XTuner 团队预训练好的 Image Projector 微调自己的多模态图文理解模型 LLaVA。
参考: https://github.com/SmartFlowAI/Llama3-Tutorial
1. 环境、模型、数据准备
1.1 配置环境
使用如下指令便可以安装好一个 python=3.10 pytorch=2.1.2+cu121 的基础环境。
conda create -n llama3 python=3.10
conda activate llama3
conda install pytorch==2.1.2 torchvision==0.16.2 torchaudio==2.1.2 pytorch-cuda=12.1 -c pytorch -c nvidia
接下来我们安装 XTuner。
cd ~
git clone -b v0.1.18 https://github.com/InternLM/XTuner
cd XTuner
pip install -e .[all]
如果已经配置好了环境,在这里也可以选择直接执行 conda activate llama3
以进入环境。
最后我们 clone 本教程仓库。
cd ~
git clone https://github.com/SmartFlowAI/Llama3-Tutorial
1.2 模型准备
1.2.1 准备 Llama3 权重
在微调开始前,我们首先来准备 Llama3-8B-Instruct 模型权重。
-
InternStudio
mkdir -p ~/model
cd ~/model
ln -s /root/share/new_models/meta-llama/Meta-Llama-3-8B-Instruct .
-
非 InternStudio
我们选择从 OpenXLab 上下载 Meta-Llama-3-8B-Instruct 的权重。
mkdir -p ~/model
cd ~/model
git lfs install
git clone https://code.openxlab.org.cn/MrCat/Llama-3-8B-Instruct.git Meta-Llama-3-8B-Instruct
1.2.2 准备 Visual Encoder 权重
我们接下来准备 Llava 所需要的 openai/clip-vit-large-patch14-336,权重,即 Visual Encoder 权重。
-
InternStudio
mkdir -p ~/model
cd ~/model
ln -s /root/share/new_models/openai/clip-vit-large-patch14-336 .
-
非 InternStudio
可以访问 https://huggingface.co/openai/clip-vit-large-patch14-336 以进行下载。
1.2.3 准备 Image Projector 权重
然后我们准备 Llava 将要用到的 Image Projector 部分权重。
-
InternStudio
mkdir -p ~/model
cd ~/model
ln -s /root/share/new_models/xtuner/llama3-llava-iter_2181.pth .
-
非 InternStudio
相关权重可以访问:https://huggingface.co/xtuner/llava-llama-3-8b 以及 https://huggingface.co/xtuner/llava-llama-3-8b-v1_1 。(已经过微调,并非 Pretrain 阶段的 Image Projector)
1.3 数据准备
我们按照 https://github.com/InternLM/Tutorial/blob/camp2/xtuner/llava/xtuner_llava.md 中的教程来准备微调数据。为了让大家可以快速上手,我们选择了使用过拟合的方式快速实现。
可以执行以下代码:
cd ~
git clone https://github.com/InternLM/tutorial -b camp2
python ~/tutorial/xtuner/llava/llava_data/repeat.py \
-i ~/tutorial/xtuner/llava/llava_data/unique_data.json \
-o ~/tutorial/xtuner/llava/llava_data/repeated_data.json \
-n 200
2. 微调过程
2.1 训练启动
我们已经为大家准备好了可以一键启动的配置文件,主要是修改好了模型路径、对话模板以及数据路径。
我们使用如下指令以启动训练:
xtuner train ~/Llama3-Tutorial/configs/llama3-llava/llava_llama3_8b_instruct_qlora_clip_vit_large_p14_336_lora_e1_finetune.py --work-dir ~/llama3_llava_pth --deepspeed deepspeed_zero2
训练过程所需显存约为44447 MiB,在单卡 A100 上训练所需时间为30分钟。
如果是30%的A100资源(24GB显存),使用如下命令启动训练:
xtuner train ~/Llama3-Tutorial/configs/llama3-llava/llava_llama3_8b_instruct_qlora_clip_vit_large_p14_336_lora_e1_finetune.py --work-dir ~/llama3_llava_pth --deepspeed deepspeed_zero2_offload
训练耗时约4.5h:024-05-07 11:21:12到2024-05-07 15:54:06。
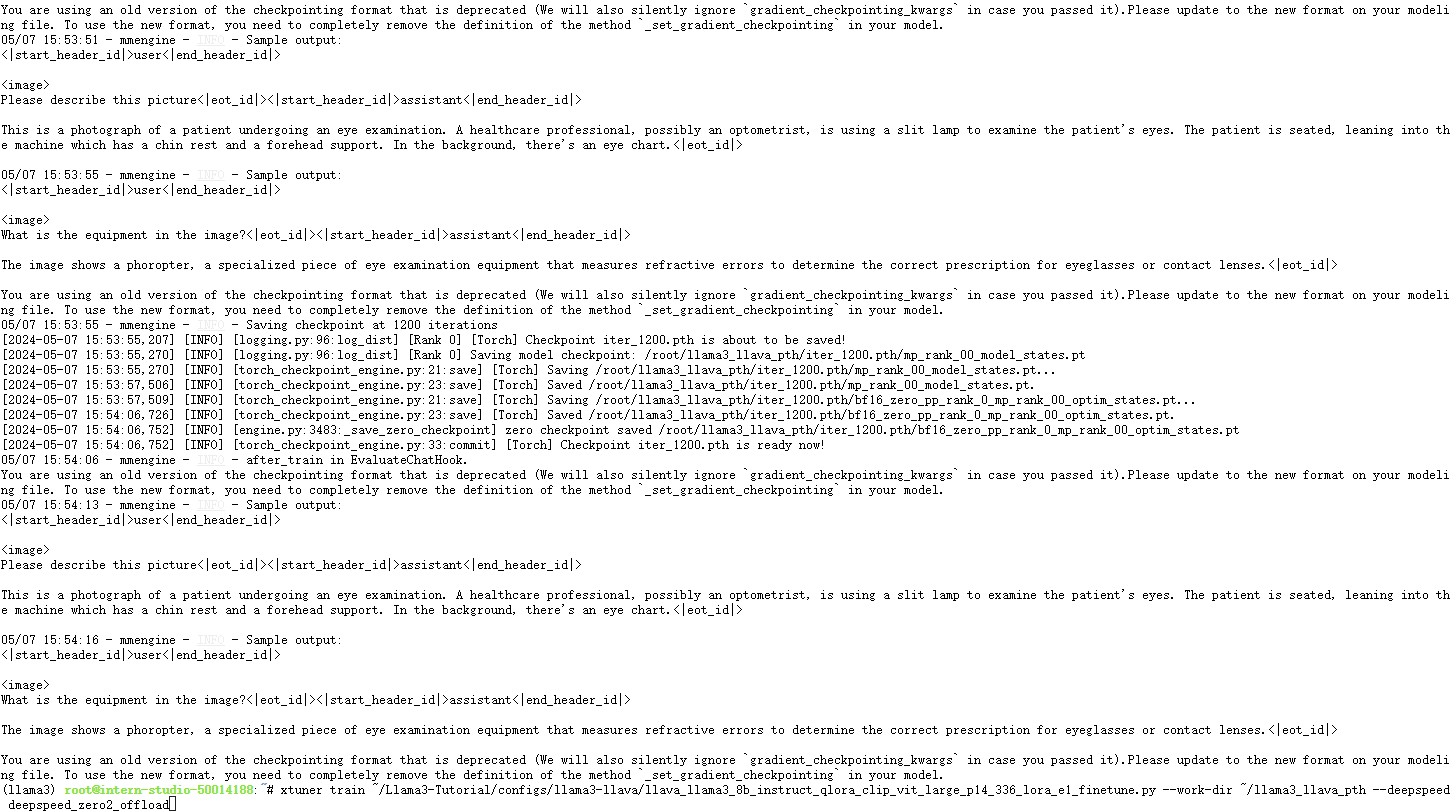
在训练好之后,我们将原始image projector和我们微调得到的image projector都转换为 HuggingFace 格式,为了下面的效果体验做准备。
注意:
训练过程时间较长,建议使用开发机webide进行操作,或者使用vscode ssh连接环境后,结合tmux工具进行。
# 原始image projector转为HuggingFace格式
xtuner convert pth_to_hf ~/Llama3-Tutorial/configs/llama3-llava/llava_llama3_8b_instruct_qlora_clip_vit_large_p14_336_lora_e1_finetune.py \
~/model/llama3-llava-iter_2181.pth \
~/llama3_llava_pth/pretrain_iter_2181_hf
# 微调得到的image projector转为HuggingFace格式
xtuner convert pth_to_hf ~/Llama3-Tutorial/configs/llama3-llava/llava_llama3_8b_instruct_qlora_clip_vit_large_p14_336_lora_e1_finetune.py \
~/llama3_llava_pth/iter_1200.pth \
~/llama3_llava_pth/iter_1200_hf
# 合并后的数据如下
(llama3) root@intern-studio-50014188:~# ls -lrt ~/llama3_llava_pth/iter_1200_hf/
total 10
drwxr-xr-x 2 root root 4096 May 7 16:04 llm_adapter
drwxr-xr-x 2 root root 4096 May 7 16:04 visual_encoder_adapter
drwxr-xr-x 2 root root 4096 May 7 16:04 projector
-rw-r--r-- 1 root root 7724 May 7 16:04 xtuner_config.py
2.2 效果体验
使用下面的图片作为测试素材:
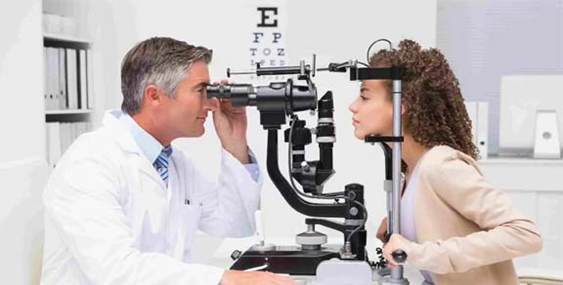
测试问题:
问题1:Describe this image. 问题2:What is the equipment in the image?
2.2.1 Pretrain模型
export MKL_SERVICE_FORCE_INTEL=1
xtuner chat /root/model/Meta-Llama-3-8B-Instruct \
--visual-encoder /root/model/clip-vit-large-patch14-336 \
--llava /root/llama3_llava_pth/pretrain_iter_2181_hf \
--prompt-template llama3_chat \
--image /root/tutorial/xtuner/llava/llava_data/test_img/oph.jpg
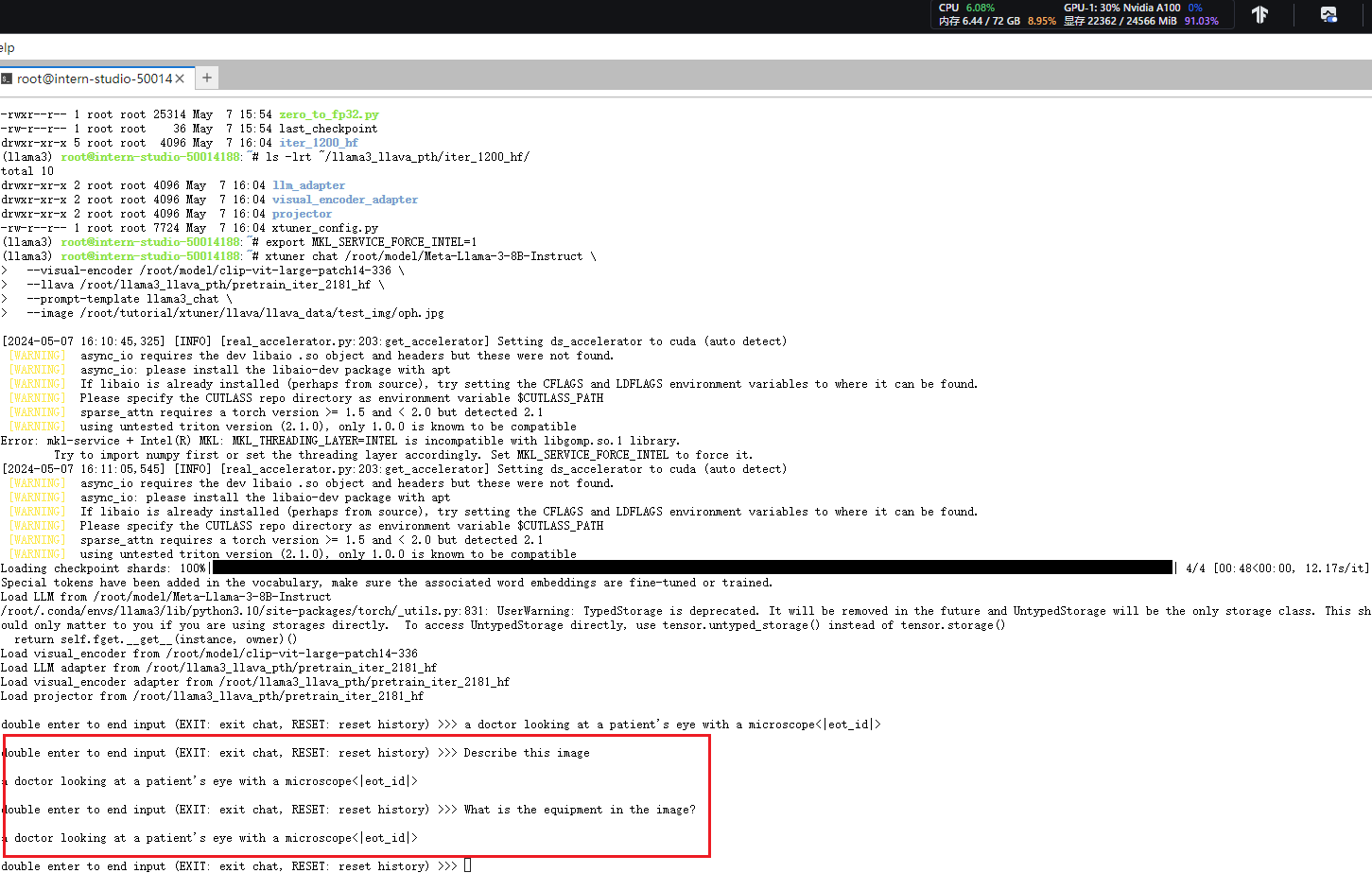
此时可以看到,Pretrain 模型只会为图片打标签,并不能回答问题。
2.2.2 Finetune后的模型
export MKL_SERVICE_FORCE_INTEL=1
xtuner chat /root/model/Meta-Llama-3-8B-Instruct \
--visual-encoder /root/model/clip-vit-large-patch14-336 \
--llava /root/llama3_llava_pth/iter_1200_hf \
--prompt-template llama3_chat \
--image /root/tutorial/xtuner/llava/llava_data/test_img/oph.jpg
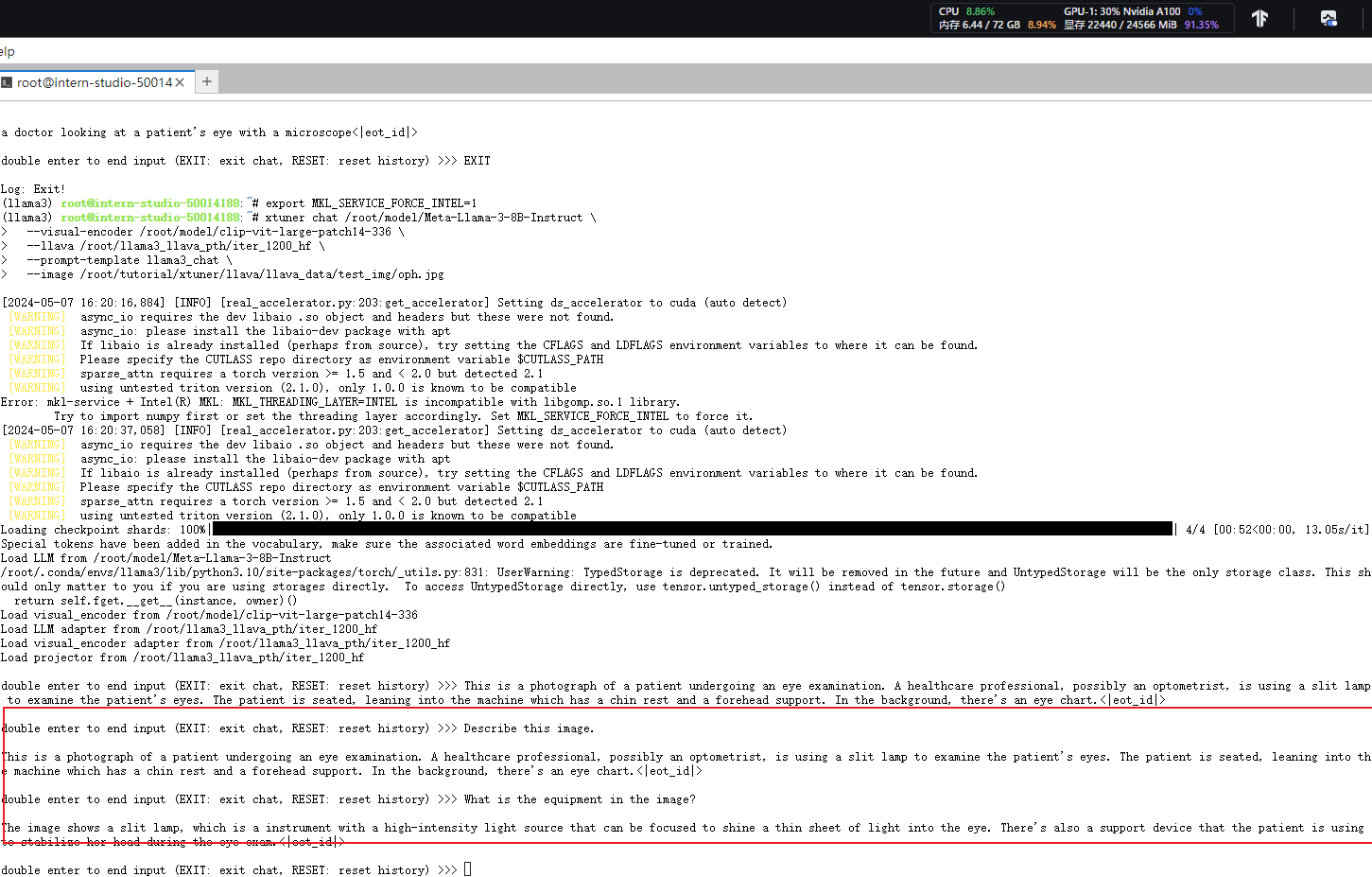
经过 Finetune 后,我们可以发现,模型已经可以根据图片回答我们的问题了。
备注:
遇到的下面问题不影响效果展示。
Error: mkl-service + Intel(R) MKL: MKL_THREADING_LAYER=INTEL is incompatible with libgomp.so.1 library.
Try to import numpy first or set the threading layer accordingly. Set MKL_SERVICE_FORCE_INTEL to force it.
本文由 mdnice 多平台发布