PCL库学习
c_cpp_properties.json
{"configurations": [{"name": "Linux","includePath": ["${workspaceFolder}/**","/usr/include","/usr/local/include"],"defines": [],"compilerPath": "/usr/bin/gcc","cStandard": "c11","cppStandard": "c++17","intelliSenseMode": "clang-x64"// "compileCommands": "${workspaceFolder}/build/compile_commands.json"}],"version": 4
}
CMakeLists.txt
cmake_minimum_required(VERSION 2.6)
project(pcl_demo)find_package(PCL 1.14 REQUIRED)include_directories(${PCL_INCLUDE_DIRS})
link_directories(${PCL_LIBRARY_DIRS})
add_definitions(${PCL_DEFINITIONS})add_executable(pcl_read pcl_read.cpp)target_link_libraries (pcl_read ${PCL_LIBRARIES})
# install(TARGETS pcl_read RUNTIME DESTINATION bin)
点云数据类型
- pcl::PointXYZ:最简单也可能是最常用到的点类型;它只储存了3Dxyz的信息。
- pcl::PointXYZI:除上面XYZ内容外还包含了一个描述点亮度(intensity)的字段。当想要获取传感器返回的亮度高于一定级别的点时非常有用。还有与此相似的其他两种标准的点数据类型:一是pcl::nterestPoint,它有一个字段储存强度(strength);二是pcl::PointWithRange;它有一个字段储存距离(视点到采样点)。 pcl::PointXYZRGBA:这种点类型储存3D信息,也储存颜色(RGB=Red,Green, Blue)和透明度(A=Alpha)。
- pcl::PointXYZRGB:这种点类型与前面的点类型相似,但是它没有透明度字段。
- pcl::Normal:这是最常用的点类型,表示曲面上给定点处的法线以及测量的曲率。
- pcl::PointNormal:这种点类型跟前一个点类型一样;它包含了给定点所在曲面法线以及曲率信息,但是它也包含了点的3Dxyz坐标。这种点类型的变异类型是PointXYZRGBNormal和 PointXYZINormal,正如名字所提示,它们包含了颜色(前者)和亮度(后者)。
公共字段
- header:这个字段是pcl::PCLHeader类型,指定了点云的获取时间。
- points:这个字段是std::vector<PointT,…>类型,它是储存所有点的容器。vector定 义中的PointT对应于类的模板参数,即点的类型。
- width:这个字段指定了点云组织成一种图像时的宽度,否则它包含的是云中点的数量。
- height:这个字段指定了点云组织成一种图像时的高度,否则它总是1。
- is_dense:这个字段指定了点云中是否有无效值(无穷大或NaN值)。
- sensor_origin_:这个字段是Eigen::Vector4f类型,并且定义了传感器根据相对于原点 的平移所得到的位姿。
- sensor_orientation_:这个字段是Eigen::Quaternionf类型,并且定义了传感器旋转所 得到的位姿。
创建并保存点云
方式1
#include <iostream>
#include <pcl/io/pcd_io.h>
#include <pcl/point_types.h>int main()
{pcl::PointCloud<pcl::PointXYZ> cloud;// Fill in the cloud datacloud.width = 5;cloud.height = 1;cloud.is_dense = false;cloud.resize(cloud.width * cloud.height);for (auto &point : cloud){point.x = 1024 * rand() / (RAND_MAX + 1.0f);point.y = 1024 * rand() / (RAND_MAX + 1.0f);point.z = 1024 * rand() / (RAND_MAX + 1.0f);}pcl::io::savePCDFileASCII("test_pcd.pcd", cloud);std::cerr << "Saved " << cloud.size() << " data points to test_pcd.pcd." << std::endl;for (const auto &point : cloud)std::cerr << " " << point.x << " " << point.y << " " << point.z << std::endl;return 0;
}
/*************
Saved 5 data points to test_pcd.pcd.0.352222 -0.151883 -0.106395-0.397406 -0.473106 0.292602-0.731898 0.667105 0.441304-0.734766 0.854581 -0.0361733-0.4607 -0.277468 -0.916762
***************/
方式2
#include <iostream>
#include <pcl/io/pcd_io.h>
#include <pcl/point_types.h>
#include <pcl/common/common_headers.h>
#include <pcl/visualization/pcl_visualizer.h>
#include <pcl/console/parse.h>int main(int argc, char **argv)
{pcl::PointCloud<pcl::PointXYZ>::Ptr basic_cloud_ptr(new pcl::PointCloud<pcl::PointXYZ>);pcl::PointCloud<pcl::PointXYZRGB>::Ptr point_cloud_ptr(new pcl::PointCloud<pcl::PointXYZRGB>);uint8_t r(255), g(15), b(15);for(float z(-1.0); z < 1.0; z += 0.05){for(float angle(0.0); angle <= 360.0; angle += 5.0){pcl::PointXYZ basic_point;basic_point.x = 0.5 * cosf(pcl::deg2rad(angle));basic_point.y = sinf(pcl::deg2rad(angle));basic_point.z = z;basic_cloud_ptr->points.push_back(basic_point);pcl::PointXYZRGB point;point.x = basic_point.x;point.y = basic_point.y;point.z = basic_point.z;uint32_t rgb = (static_cast<uint32_t>(r) << 16 | static_cast<uint32_t>(g) << 8 |static_cast<uint32_t>(b));point.rgb = *reinterpret_cast<float *> (&rgb);point_cloud_ptr->points.push_back(point); }if(z<0.0){r -= 12;g += 12;}else{g -= 12;b += 12;}}basic_cloud_ptr->width = (int)basic_cloud_ptr->points.size();basic_cloud_ptr->height = 1;basic_cloud_ptr->is_dense = false;pcl::io::savePCDFileASCII("test_pcd_basic_2.pcd", *basic_cloud_ptr);for(const auto &point:basic_cloud_ptr->points)std::cerr<<" "<<point.x<<" "<<point.y<<" "<<point.z<<std::endl;point_cloud_ptr->width = (int)point_cloud_ptr->points.size();point_cloud_ptr->height = 1;point_cloud_ptr->is_dense = false;pcl::io::savePCDFileASCII("test_pcd_point_2.pcd", *point_cloud_ptr);for(const auto &point:point_cloud_ptr->points)std::cerr<<" "<<point.x<<" "<<point.y<<" "<<point.z<<std::endl;return 0;
}
test_pcd.pcd
# .PCD v.7 - Point Cloud Data file format
VERSION .7 # 版本号
FIELDS x y z # 指定一个点可以有的每一个维度和字段的名字
SIZE 4 4 4 # 用字节数指定每一个维度的大小。例如:
TYPE F F F # 用一个字符指定每一个维度的类型 int uint folat
COUNT 1 1 1 1 # 指定每一个维度包含的元素数目
WIDTH 5 # 像图像一样的有序结构,有5行和1列,
HEIGHT 1 # 这样该数据集中共有5*1=5个点
VIEWPOINT 0 0 0 1 0 0 0 # 指定数据集中点云的获取视点 视点信息被指定为平移(txtytz)+四元数(qwqxqyqz)
POINTS 5 # 指定点云中点的总数。从0.7版本开始,该字段就有点多余了
DATA ascii # 指定存储点云数据的数据类型。支持两种数据类型:ascii和二进制
0.35222197 -0.15188313 -0.10639524
-0.3974061 -0.47310591 0.29260206
-0.73189831 0.66710472 0.44130373
-0.73476553 0.85458088 -0.036173344
-0.46070004 -0.2774682 -0.91676188
读取点云
#include <iostream>
#include <pcl/io/pcd_io.h>
#include <pcl/point_types.h>int main(int argc, char ** argv)
{pcl::PointCloud<pcl::PointXYZ>::Ptr cloud(new pcl::PointCloud<pcl::PointXYZ>);if(pcl::io::loadPCDFile<pcl::PointXYZ>("/home/ghigher/CPuls_Project/test01/build/test_pcd.pcd", *cloud) == -1){PCL_ERROR("Couldn`t read file test_pcd.pcd \n");return -1;}std::cout<<"Load " << cloud->width * cloud->height << "data points from test_pcd.pcd width the following fields:" <<std::endl;for(size_t i = 0; i<cloud->points.size(); ++i){std::cout << " "<< cloud->points[i].x << " "<< cloud->points[i].y <<" "<< cloud->points[i].z << std::endl;}return 0;
}
/*********************************
Load 5data points from test_pcd.pcd width the following fields:0.352222 -0.151883 -0.106395-0.397406 -0.473106 0.292602-0.731898 0.667105 0.441304-0.734766 0.854581 -0.0361733-0.4607 -0.277468 -0.916762
*********************************/
显示点云
#include <pcl/visualization/cloud_viewer.h>
#include <iostream>
#include <pcl/io/io.h>
#include <pcl/io/pcd_io.h>int main()
{pcl::PointCloud<pcl::PointXYZRGB>::Ptr cloud(new pcl::PointCloud<pcl::PointXYZRGB>);pcl::io::loadPCDFile("/home/ghigher/CPuls_Project/test01/build/test_pcd_point_2.pcd", *cloud);//创建CloudViewer点云显示对象pcl::visualization::CloudViewer viewer("Cloud Viewer");viewer.showCloud(cloud);while(!viewer.wasStopped()){}return 0;
}
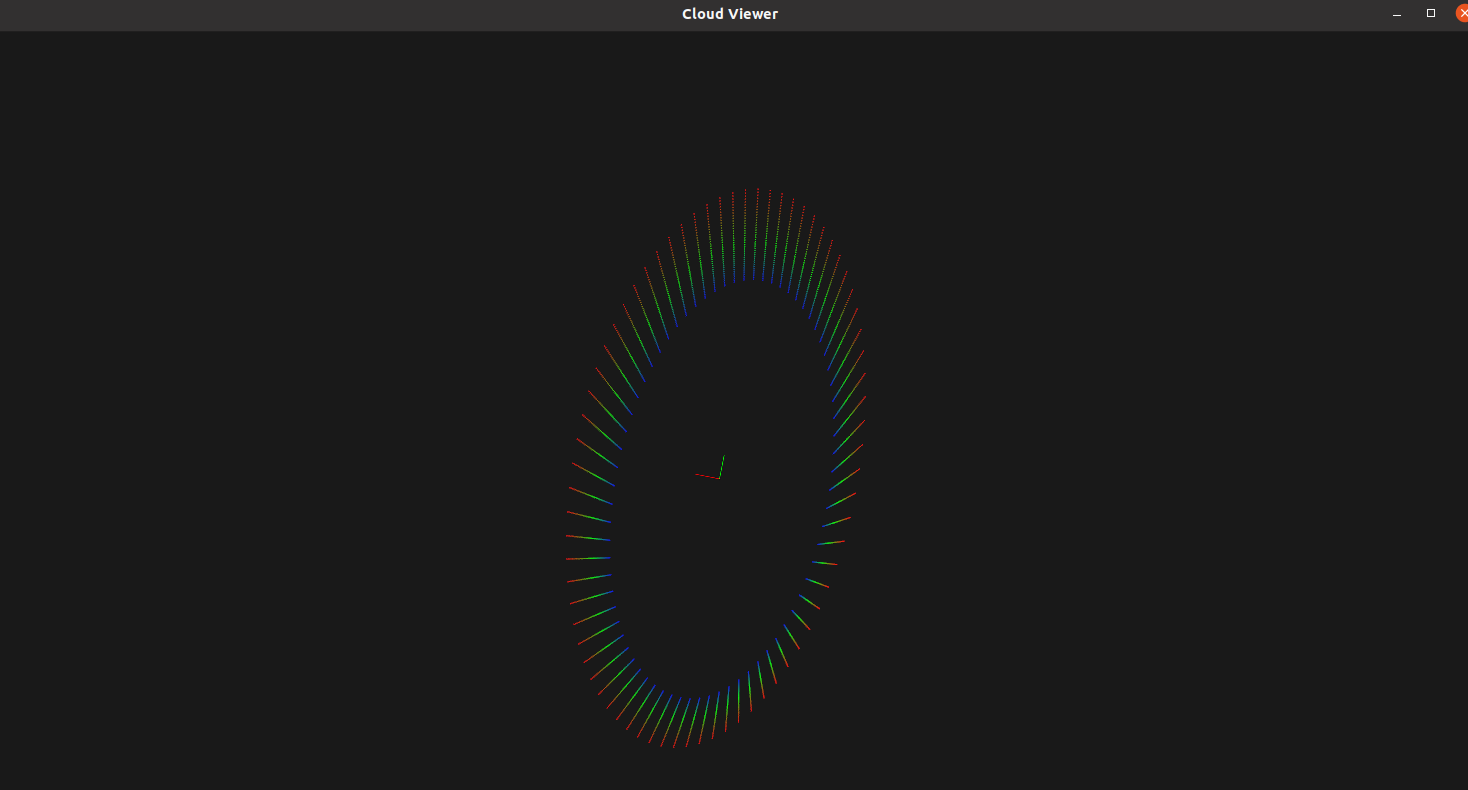
KDTree
#include <pcl/point_cloud.h> //点类型定义头文件
#include <pcl/kdtree/kdtree_flann.h> //kdtree类定义头文件#include <iostream>
#include <vector>
#include <ctime>int
main (int argc, char** argv)
{srand (time (NULL)); //用系统时间初始化随机种子//创建一个PointCloud<pcl::PointXYZ>pcl::PointCloud<pcl::PointXYZ>::Ptr cloud (new pcl::PointCloud<pcl::PointXYZ>);// 随机点云生成cloud->width = 1000; //此处点云数量cloud->height = 1; //表示点云为无序点云cloud->points.resize (cloud->width * cloud->height);for (size_t i = 0; i < cloud->points.size (); ++i) //循环填充点云数据{cloud->points[i].x = 1024.0f * rand() / (RAND_MAX + 1.0f); // // 产生数值为0-1024的浮点数cloud->points[i].y = 1024.0f * rand () / (RAND_MAX + 1.0f);cloud->points[i].z = 1024.0f * rand () / (RAND_MAX + 1.0f);}//创建KdTreeFLANN对象,并把创建的点云设置为输入,创建一个searchPoint变量作为查询点pcl::KdTreeFLANN<pcl::PointXYZ> kdtree; // pcl::KdTreeFLANN<PointT, Dist>::setInputCloud (const PointCloudConstPtr &cloud, const IndicesConstPtr &indices)//设置搜索空间kdtree.setInputCloud (cloud);//设置查询点并赋随机值pcl::PointXYZ searchPoint;searchPoint.x = 1024.0f * rand () / (RAND_MAX + 1.0f);searchPoint.y = 1024.0f * rand () / (RAND_MAX + 1.0f);searchPoint.z = 1024.0f * rand () / (RAND_MAX + 1.0f);// K 临近搜索//创建一个整数(设置为10)和两个向量来存储搜索到的K近邻,两个向量中,一个存储搜索到查询点近邻的索引,另一个存储对应近邻的距离平方int K = 10;std::vector<int> pointIdxNKNSearch(K); //存储查询点近邻索引std::vector<float> pointNKNSquaredDistance(K); //存储近邻点对应距离平方//打印相关信息std::cout << "K nearest neighbor search at (" << searchPoint.x << " " << searchPoint.y << " " << searchPoint.z<< ") with K=" << K << std::endl;if ( kdtree.nearestKSearch (searchPoint, K, pointIdxNKNSearch, pointNKNSquaredDistance) > 0 ) //执行K近邻搜索{//打印所有近邻坐标for (size_t i = 0; i < pointIdxNKNSearch.size (); ++i)std::cout << " " << cloud->points[ pointIdxNKNSearch[i] ].x << " " << cloud->points[ pointIdxNKNSearch[i] ].y << " " << cloud->points[ pointIdxNKNSearch[i] ].z << " (squared distance: " << pointNKNSquaredDistance[i] << ")" << std::endl;}// 半径 R内近邻搜索方法std::vector<int> pointIdxRadiusSearch; //存储近邻索引std::vector<float> pointRadiusSquaredDistance; //存储近邻对应距离的平方float radius = 256.0f * rand () / (RAND_MAX + 1.0f); //随机的生成某一半径//打印输出std::cout << "Neighbors within radius search at (" << searchPoint.x << " " << searchPoint.y << " " << searchPoint.z<< ") with radius=" << radius << std::endl;// 假设我们的kdtree返回了大于0个近邻。那么它将打印出在我们"searchPoint"附近的10个最近的邻居并把它们存到先前创立的向量中。if ( kdtree.radiusSearch (searchPoint, radius, pointIdxRadiusSearch, pointRadiusSquaredDistance) > 0 ) //执行半径R内近邻搜索方法{for (size_t i = 0; i < pointIdxRadiusSearch.size (); ++i)std::cout << " " << cloud->points[ pointIdxRadiusSearch[i] ].x << " " << cloud->points[ pointIdxRadiusSearch[i] ].y << " " << cloud->points[ pointIdxRadiusSearch[i] ].z << " (squared distance: " << pointRadiusSquaredDistance[i] << ")" << std::endl;}return 0;
}
/**************************
K nearest neighbor search at (763.71 771.792 499.333) with K=10743.497 707.763 466.9 (squared distance: 5560.2)743.305 814.092 581.355 (squared distance: 8933.23)831.035 753.543 578.683 (squared distance: 11162.2)706.388 734.124 580.101 (squared distance: 11228.1)811.653 670.148 524.748 (squared distance: 13276)819.709 753.288 599.12 (squared distance: 13435.7)852.38 697.685 530.509 (squared distance: 14326.1)817.204 665.183 481.782 (squared distance: 14535)694 796.036 396.447 (squared distance: 16032.8)788.24 794.644 621.827 (squared distance: 16128.8)
Neighbors within radius search at (763.71 771.792 499.333) with radius=118.164743.497 707.763 466.9 (squared distance: 5560.2)743.305 814.092 581.355 (squared distance: 8933.23)831.035 753.543 578.683 (squared distance: 11162.2)706.388 734.124 580.101 (squared distance: 11228.1)811.653 670.148 524.748 (squared distance: 13276)819.709 753.288 599.12 (squared distance: 13435.7)
**************************/
ROS中使用
PCL接口
- std_msgs::Header:通常是每一个ROS消息的一部分。它包含消息发送时间、序列号和坐标系名称等信息。等价于pcl::Headertype。
- sensor_msgs::PointCloud2:用来转换pcl::PointCloud类型
- pcl_msgs::PointIndices:这个消息类型储存了一个点云中点的索引,等价PCL类型是pcl::PointIndices。
- pcl_msgs::PolygonMesh:这个消息类型保存了描绘网格、顶点和多边形的信息,等价PCL类型是pcl::PolygonMesh。
- pcl_msgs::Vertices:这个消息类型将一组顶点的索引保存在一个数组中,例如描述一个多边形。等价PCL类型是pcl::Vertices。
- pcl_msgs::ModelCoefficients:这个消息类型储存了一个模型的不同系数,例如描述一 个平面需要的四个参数。等价的PCL类型是pcl:ModelCoefficients。
void toROSMsg(const pcl::PointCloud<T> &, sensor_msgs::PointCloud2 &);
void fromROSMsg(const sensor_msgs::PointCloud2 &, pcl::PointCloud<T> &);
void moveFromROSMsg(sensor_msgs::PointCloud2 &, pcl::PointCloud<T> &);
创建并发布
依赖
message_filters roscpp rosbag rospy sensor_msgs std_msgs pcl_ros pcl_conversions message_generation
#include <iostream>
#include <pcl/point_types.h>
#include <pcl_conversions/pcl_conversions.h>
#include <ros/ros.h>
#include <sensor_msgs/PointCloud2.h>int main(int argc, char **argv)
{ros::init(argc, argv, "point_cloud_node");ros::NodeHandle nh;ros::Publisher pub = nh.advertise<sensor_msgs::PointCloud2>("pointcloud_pub_topic", 1000);ros::Rate rate(3);unsigned int num_points = 4;pcl::PointCloud<pcl::PointXYZRGB> cloud;cloud.points.resize(num_points);sensor_msgs::PointCloud2 output_msg;while(ros::ok()){output_msg.header.stamp = ros::Time::now();for(int i=0; i<num_points; i++){cloud.points[i].x = i;cloud.points[i].y = i;cloud.points[i].z = i;cloud.points[i].r = 0;cloud.points[i].g = 255;cloud.points[i].b = 0;}pcl::toROSMsg(cloud, output_msg);output_msg.header.frame_id = "point_cloud_frame_id";pub.publish(output_msg);rate.sleep();}ros::spin();return 0;}
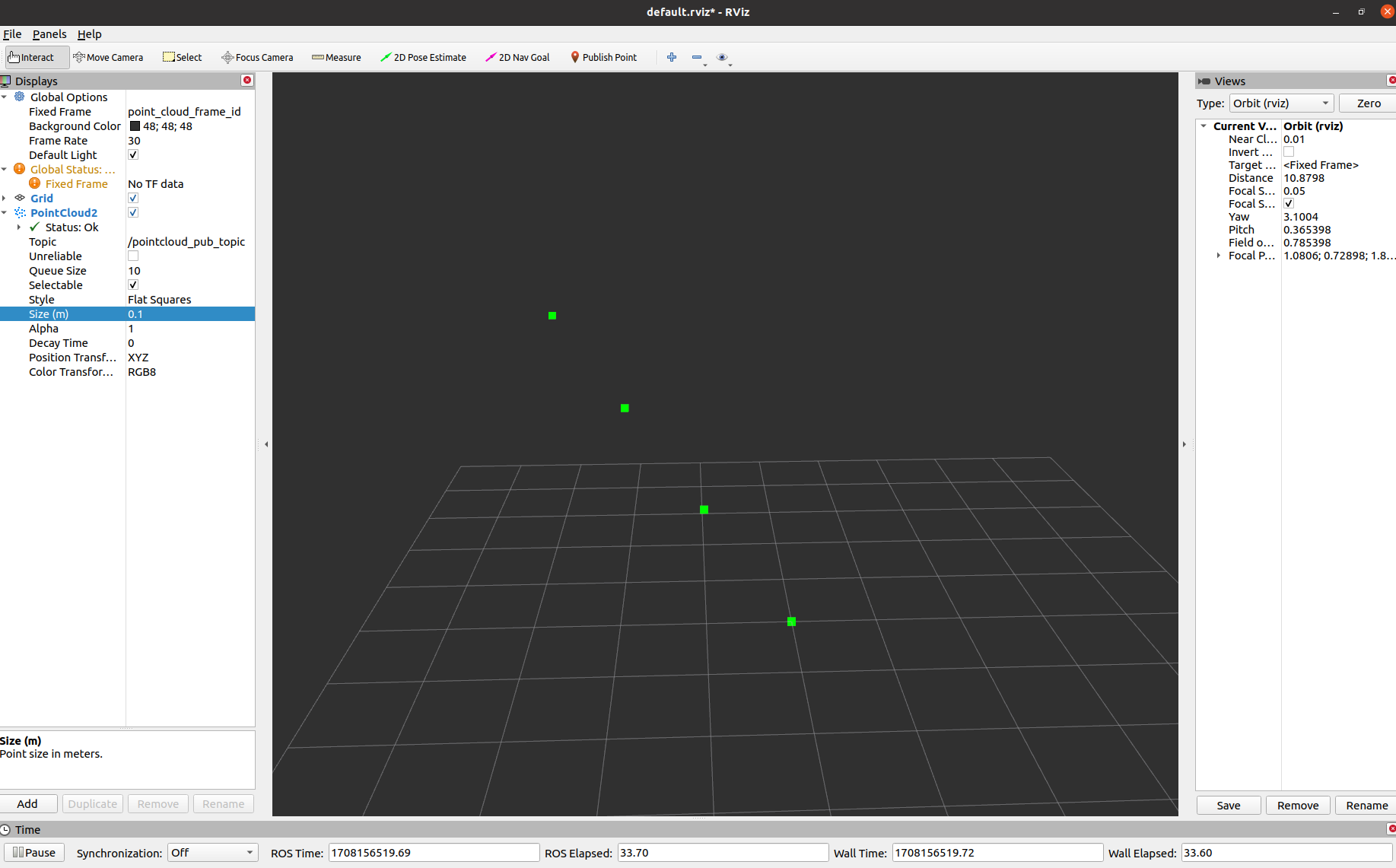
接收并进行位姿转换
#include <ros/ros.h>
#include <sensor_msgs/PointCloud2.h>
#include <pcl/point_types.h>
#include <pcl_conversions/pcl_conversions.h>
#include <pcl/common/transforms.h>
#include <pcl/visualization/cloud_viewer.h>
#include <pcl/filters/voxel_grid.h>class pcl_sub
{private:ros::NodeHandle nh;ros::Subscriber subCloud;ros::Publisher pubCloud;sensor_msgs::PointCloud2 msg;sensor_msgs::PointCloud2 adjust_msg;pcl::PointCloud<pcl::PointXYZRGB> adjust_pcl;public:pcl_sub():nh("~"){subCloud = nh.subscribe<sensor_msgs::PointCloud2>("/point_cloud_topic", 1, &pcl_sub::getcloud, this);pubCloud = nh.advertise<sensor_msgs::PointCloud2>("/adjust_msgs", 1);}void getcloud(const sensor_msgs::PointCloud2ConstPtr &laserCloudMsg){pcl::PointCloud<pcl::PointXYZRGB>::Ptr adjust_pcl_ptr(new pcl::PointCloud<pcl::PointXYZRGB>);//将ROS消息转换为PCL点云pcl::fromROSMsg(*laserCloudMsg, *adjust_pcl_ptr);//定义一个旋转矩阵 虽然为3D 实质上是4x4矩阵(旋转+平移)Eigen::Isometry3d T = Eigen::Isometry3d::Identity();Eigen::AngleAxisd rotationVector(M_PI/4, Eigen::Vector3d(0, 0, 1));Eigen::Matrix3d rotationMatrix = Eigen::Matrix3d::Identity();rotationMatrix = rotationVector.toRotationMatrix();//旋转部分赋值T.linear() = rotationMatrix;//平移部分赋值T.translation() = Eigen::Vector3d(0, 0, 0);//执行变换 保存结果pcl::PointCloud<pcl::PointXYZRGB>::Ptr transform_cloud(new pcl::PointCloud<pcl::PointXYZRGB>);pcl::transformPointCloud (*adjust_pcl_ptr, *transform_cloud, T.matrix());adjust_pcl = *transform_cloud;for(int i = 0; i<adjust_pcl.points.size();i++){adjust_pcl.points[i].r = 255;adjust_pcl.points[i].g = 0;adjust_pcl.points[i].b = 0;}//将PCL点云转换为ROS消息pcl::toROSMsg(adjust_pcl, adjust_msg);pubCloud.publish(adjust_msg);}~pcl_sub(){}
};int main(int argc, char **argv)
{ros::init(argc, argv, "point_cloud_transform");pcl_sub ps;ros::spin();return 0;
}
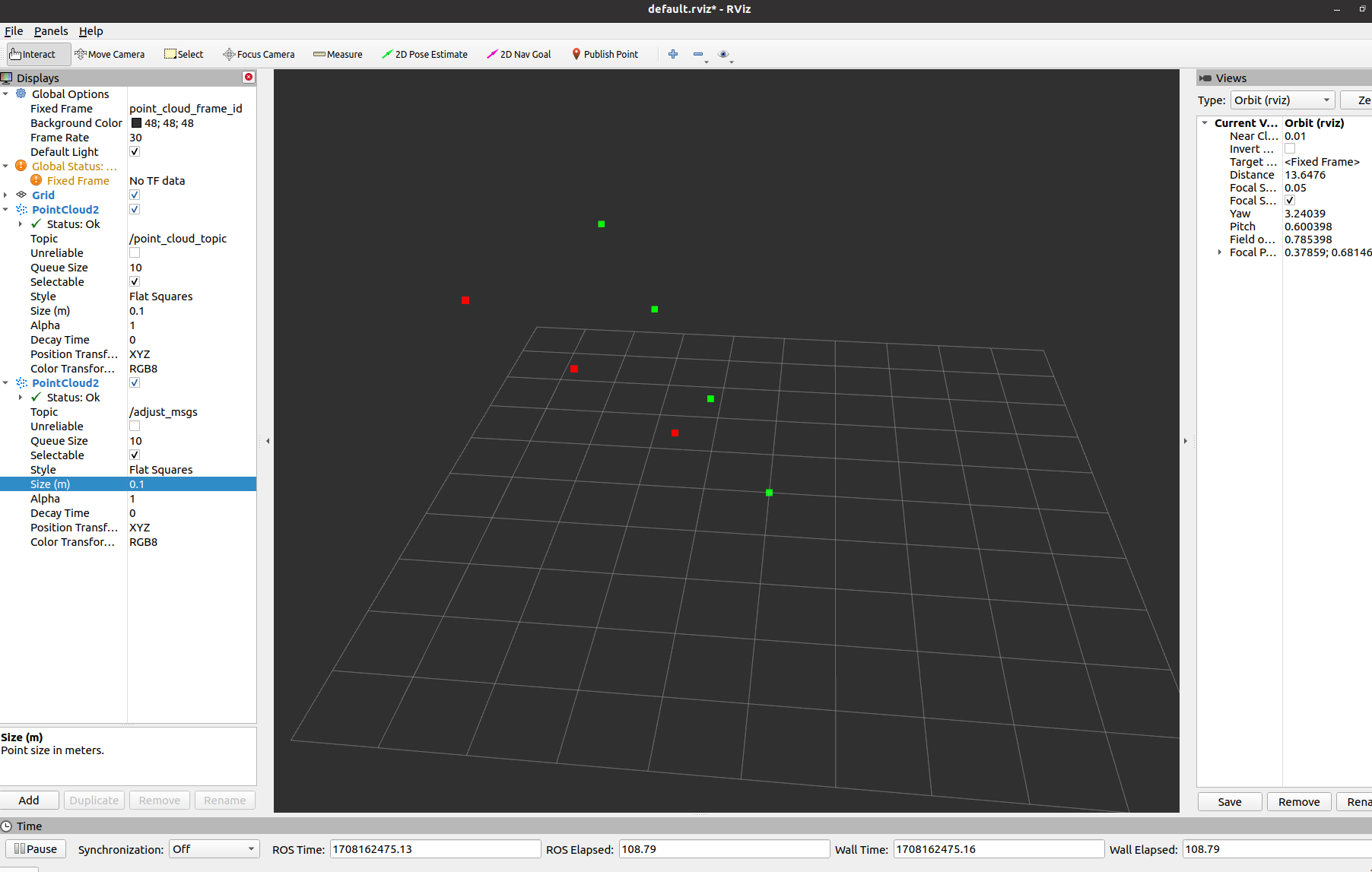
参考视频:https://space.bilibili.com/222855903