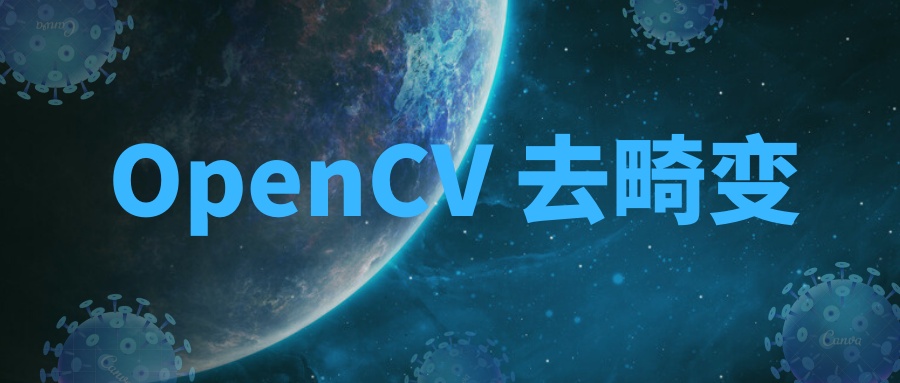
在opencv中,有关图像或像素点(角点)去畸变的函数有cv::undistort(),cv::getOptimalNewCameraMatrix(),cv::initUndistortRectifyMap(),remap(),cv::undistortPoints()。其中undistort可以直接对图像去畸变,getOptimalNewCameraMatrix、initUndistortRectifyMap和remap配合也可以对图像去畸变,他们之间有相同之处,又有各自特性,而undistortPoints是只针对像素点去畸变。下面就使用方法及细节进行梳理,并给出代码示例,以便加深印象。
1. 函数功能及参数介绍
1.1 cv::getOptimalNewCameraMatrix()
函数参数说明:
Mat cv::getOptimalNewCameraMatrix
( InputArray cameraMatrix, // 相机内参矩阵InputArray distCoeffs, // 相机畸变参数Size imageSize, // 图像尺寸double alpha, // 缩放比例Size newImgSize = Size(), // 校正后的图像尺寸Rect * validPixROI = 0, // 输出感兴趣区域设置bool centerPrincipalPoint = false // 可选标志
)
这个函数的功能是,"Return the new camera matrix based on the free scaling parameter",即根据根据比例因子返回相应的新的相机内参矩阵。
这里的"比例因子"就是函数的第四个参数(alpha),这个参数的范围是(0, 1)。调节alpha的值能够控制得到的新矩阵中的fx和fy的大小。
当alpha=1的时候,原图像中的所有像素能够得到保留,因此这个时候得到的矫正后的图像是带黑框的,如后面图1所示。
当alpha=0时,得到的图像是不带黑色边框的,相对于原图像,此时的图像损失了部分像素,如后面图2所示。alpha的值控制着具体损失多少像素。
alpha
和newImageSize
是两个互不干扰的参数,alpha
只管是否对图像进行裁剪,而newImageSize
只负责把图像进行缩放,这二者都会对返回的新的相机参数造成影响.
1.2 cv::initUndistortRectifyMap()
函数参数说明:
void cv::initUndistortRectifyMap
( InputArray cameraMatrix, // 原相机内参矩阵InputArray distCoeffs, // 原相机畸变参数InputArray R, // 可选的修正变换矩阵 InputArray newCameraMatrix, // 新相机内参矩阵Size size, // 去畸变后图像的尺寸int m1type, // 第一个输出的映射(map1)的类型,CV_32FC1 or CV_16SC2OutputArray map1, // 第一个输出映射OutputArray map2 // 第二个输出映射
)
这个函数用于计算原始图像和矫正图像之间的转换关系,将结果以映射的形式表达,映射关系存储在map1和map2中。
在单目相机例子中,newCameraMatrix
可以用cv::getOptimalNewCameraMatrix来计算,或者直接与cameraMatrix
相等。在双目相机例子中,newCameraMatrix
一般是用cv::stereoRectify
计算而来的(这里不做讨论)。
1.3 cv::remap()
void cv::remap
( InputArray src, // 原始图像OutputArray dst, // 矫正图像 InputArray map1, // 第一个映射 InputArray map2, // 第二个映射 int interpolation, // 插值方式int borderMode=BORDER_CONSTANT, // 边界模式 const Scalar& borderValue=Scalar() // 边界颜色,默认Scalar()黑色
)
函数功能:把原始图像中某位置的像素映射到矫正后的图像指定位置。
这里的map1和map2就是上面cv::initUndistortRectifyMap()计算出来的结果。
1.4 cv::undistort()
void cv::undistort
( InputArray src, // 原始图像OutputArray dst, // 矫正图像InputArray cameraMatrix, // 原相机内参矩阵InputArray distCoeffs, // 相机畸变参数InputArray newCameraMatrix = noArray() // 新相机内参矩阵
)
函数功能:直接对图像进行畸变矫正。
其内部调用了initUndistortRectifyMap和remap函数。
1.5 cv::undistortPoints()
void cv::undistortPoints
( InputArray src, // 原始像素点矩阵 1xN or Nx1 (CV_32FC2 or CV_64FC2).OutputArray dst, // 矫正像素点矩阵InputArray cameraMatrix, // 原相机内参矩阵InputArray distCoeffs, // 相机畸变参数InputArray R = noArray(), // 可选的修正变换矩阵InputArray P = noArray() // 新的相机矩阵
)
函数功能:只对图像中的某些点做畸变矫正。
2. 示例代码
2.1 使用 getOptimalNewCameraMatrix + initUndistortRectifyMap + remap 矫正图像
//
// Created by jiang on 2020/4/29.
//
#include <iostream>
#include <opencv2/opencv.hpp>using namespace std;int main()
{const cv::Mat K = ( cv::Mat_<double> ( 3,3 ) << 931.73, 0.0, 480.0, 0.0, 933.16, 302.0, 0.0, 0.0, 1.0 );const cv::Mat D = ( cv::Mat_<double> ( 5,1 ) << -1.7165e-1, 1.968259e-1, 0.0, 0.0, -3.639514e-1 );const string str = "/home/jiang/4_learn/WeChatCode/ImageUndistort/data/";const int nImage = 5;const int ImgWidth = 960;const int ImgHeight = 640;cv::Mat map1, map2;cv::Size imageSize(ImgWidth, ImgHeight);const double alpha = 1;cv::Mat NewCameraMatrix = getOptimalNewCameraMatrix(K, D, imageSize, alpha, imageSize, 0);initUndistortRectifyMap(K, D, cv::Mat(), NewCameraMatrix, imageSize, CV_16SC2, map1, map2);for(int i=0; i<nImage; i++){string InputPath = str + to_string(i) + ".png";cv::Mat RawImage = cv::imread(InputPath);cv::imshow("RawImage", RawImage);cv::Mat UndistortImage;remap(RawImage, UndistortImage, map1, map2, cv::INTER_LINEAR);cv::imshow("UndistortImage", UndistortImage);string OutputPath = str + to_string(i) + "_un" + ".png";cv::imwrite(OutputPath, UndistortImage);cv::waitKey(0);}return 0;
}
当alpha=1时,所有像素均保留,但存在黑色边框,矫正后的图像如图1所示。
当alpha=0时,损失最多的像素,没有黑色边框,矫正后的图像如图2所示。
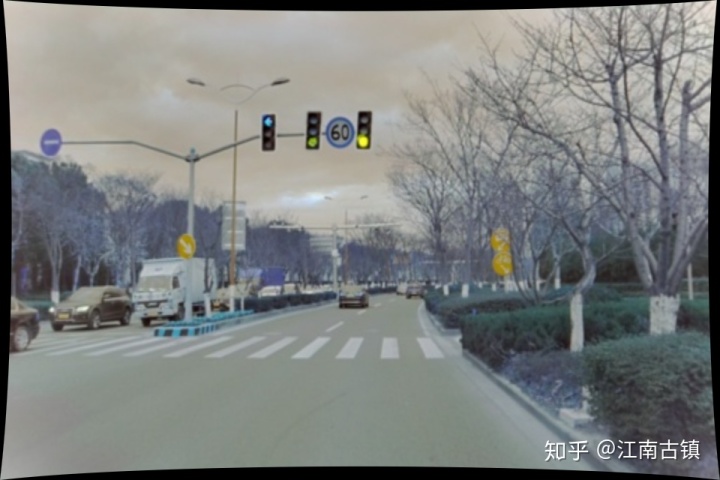
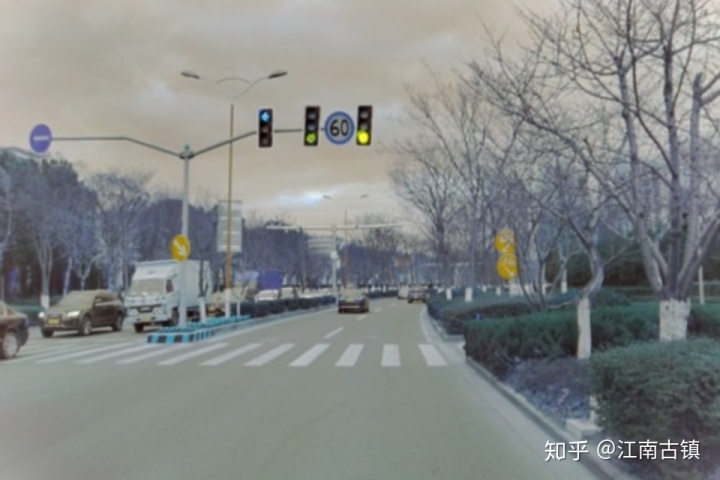
2.2 使用 undistort 矫正图像
//
// Created by jiang on 2020/4/29.
//
#include <iostream>
#include <opencv2/opencv.hpp>using namespace std;int main()
{const cv::Mat K = ( cv::Mat_<double> ( 3,3 ) << 931.73, 0.0, 480.0, 0.0, 933.16, 302.0, 0.0, 0.0, 1.0 );const cv::Mat D = ( cv::Mat_<double> ( 5,1 ) << -1.7165e-1, 1.968259e-1, 0.0, 0.0, -3.639514e-1 );const string str = "/home/jiang/4_learn/WeChatCode/ImageUndistort/data/";const int nImage = 5;const int ImgWidth = 960;const int ImgHeight = 640;cv::Mat map1, map2;cv::Size imageSize(ImgWidth, ImgHeight);const double alpha = 1;cv::Mat NewCameraMatrix = getOptimalNewCameraMatrix(K, D, imageSize, alpha, imageSize, 0);for(int i=0; i<nImage; i++){string InputPath = str + to_string(i) + ".png";cv::Mat RawImage = cv::imread(InputPath);cv::imshow("RawImage", RawImage);cv::Mat UndistortImage;cv::undistort(RawImage, UndistortImage, K, D, K);
// cv::undistort(RawImage, UndistortImage, K, D, NewCameraMatrix);cv::imshow("UndistortImage", UndistortImage);string OutputPath = str + to_string(i) + "_un2" + ".png";cv::imwrite(OutputPath, UndistortImage);cv::waitKey(0);}return 0;
}
如果undistort函数的最后一个参数使用原相机内参,那么得到的结果就是上面图2的结果,相当于alpha=0的情况。
如果undistort函数的最后一个参数使用getOptimalNewCameraMatrix计算出来的新矩阵,那么得到损失像素后的图像,当alpha=1时,就得到上面图1的结果。
如果像我示例程序一样,有多个图片需要矫正,那么推荐使用2.1的方法,因为initUndistortRectifyMap函数只需要计算一次就行,不需要每次循环都计算,可以像我程序中一样,将initUndistortRectifyMap放在循环外面。而使用2.2的方法,因为undistort函数内部调用了initUndistortRectifyMap和remap,所以相当于你n张图像计算了n次initUndistortRectifyMap,这会大大降低效率,增加程序耗时。
2.3 使用 undistortPoints 矫正角点
方法一:
//
// Created by jiang on 2020/4/29.
//
#include <iostream>
#include <opencv2/opencv.hpp>using namespace std;const cv::Mat K = ( cv::Mat_<double> ( 3,3 ) << 931.73, 0.0, 480.0, 0.0, 933.16, 302.0, 0.0, 0.0, 1.0 );
const cv::Mat D = ( cv::Mat_<double> ( 5,1 ) << -1.7165e-1, 1.968259e-1, 0.0, 0.0, -3.639514e-1 );void UndistortKeyPoints(vector<cv::Point2f> &points);int main()
{const string str = "/home/jiang/4_learn/WeChatCode/ImageUndistort/data/";const int nImage = 5;const int MAX_CNT = 150;const int MIN_DIST = 30;for(int i=0; i<nImage; i++){string InputPath = str + to_string(i) + ".png";cv::Mat RawImage = cv::imread(InputPath);vector<cv::Point2f> pts;cv::Mat RawImage_Gray;cv::cvtColor(RawImage, RawImage_Gray, CV_RGB2GRAY);cv::goodFeaturesToTrack(RawImage_Gray, pts, MAX_CNT, 0.01, MIN_DIST);for(auto& pt:pts)circle(RawImage, pt, 2, cv::Scalar(255, 0, 0), 2);cv::imshow("pts", RawImage);UndistortKeyPoints(pts);cv::Mat UndistortImage;cv::undistort(RawImage, UndistortImage, K, D, K);for(auto& pt:pts)circle(UndistortImage, pt, 2, cv::Scalar(0, 0, 255), 2);cv::imshow("pts_un", UndistortImage);string OutputPath = str + to_string(i) + "_pts_un" + ".png";cv::imwrite(OutputPath, UndistortImage);cv::waitKey(0);}return 0;
}void UndistortKeyPoints(vector<cv::Point2f> &points)
{if(D.at<float>(0)==0.0) // 图像矫正过return;// N为提取的特征点数量,将N个特征点保存在N*2的mat中uint N = points.size();cv::Mat mat(N,2,CV_32F);for(int i=0; i<N; i++){mat.at<float>(i,0)=points[i].x;mat.at<float>(i,1)=points[i].y;}// 调整mat的通道为2,矩阵的行列形状不变mat=mat.reshape(2);cv::undistortPoints(mat, mat, K, D, cv::Mat(), K);mat=mat.reshape(1);// 存储校正后的特征点for(int i=0; i<N; i++){cv::Point2f kp = points[i];kp.x=mat.at<float>(i,0);kp.y=mat.at<float>(i,1);points[i] = kp;}
}
方法二:
//
// Created by jiang on 2020/4/29.
//
#include <iostream>
#include <opencv2/opencv.hpp>using namespace std;const cv::Mat K = ( cv::Mat_<double> ( 3,3 ) << 931.73, 0.0, 480.0, 0.0, 933.16, 302.0, 0.0, 0.0, 1.0 );
const cv::Mat D = ( cv::Mat_<double> ( 5,1 ) << -1.7165e-1, 1.968259e-1, 0.0, 0.0, -3.639514e-1 );void UndistortKeyPoints(vector<cv::Point2f> &points, cv::Mat &newCamMatrix);int main()
{const string str = "/home/jiang/4_learn/WeChatCode/ImageUndistort/data/";const int nImage = 5;const int ImgWidth = 960;const int ImgHeight = 640;const int MAX_CNT = 150;const int MIN_DIST = 30;cv::Mat map1, map2;cv::Size imageSize(ImgWidth, ImgHeight);const double alpha = 1;cv::Mat NewCameraMatrix = getOptimalNewCameraMatrix(K, D, imageSize, alpha, imageSize, 0);initUndistortRectifyMap(K, D, cv::Mat(), NewCameraMatrix, imageSize, CV_16SC2, map1, map2);for(int i=0; i<nImage; i++){string InputPath = str + to_string(i) + ".png";cv::Mat RawImage = cv::imread(InputPath);cv::Mat UndistortImage;cv::remap(RawImage, UndistortImage, map1, map2, cv::INTER_LINEAR);vector<cv::Point2f> pts;cv::Mat RawImage_Gray;cv::cvtColor(RawImage, RawImage_Gray, CV_RGB2GRAY);cv::goodFeaturesToTrack(RawImage_Gray, pts, MAX_CNT, 0.01, MIN_DIST);for(auto& pt:pts)circle(RawImage, pt, 2, cv::Scalar(255, 0, 0), 2);cv::imshow("pts", RawImage);UndistortKeyPoints(pts, NewCameraMatrix);for(auto& pt:pts)circle(UndistortImage, pt, 2, cv::Scalar(0, 0, 255), 2);cv::imshow("pts_un", UndistortImage);string OutputPath = str + to_string(i) + "_pts_un" + ".png";cv::imwrite(OutputPath, UndistortImage);cv::waitKey(0);}return 0;
}void UndistortKeyPoints(vector<cv::Point2f> &points, cv::Mat &newCamMatrix)
{if(D.at<float>(0)==0.0) // 图像矫正过return;// N为提取的特征点数量,将N个特征点保存在N*2的mat中uint N = points.size();cv::Mat mat(N,2,CV_32F);for(int i=0; i<N; i++){mat.at<float>(i,0)=points[i].x;mat.at<float>(i,1)=points[i].y;}// 调整mat的通道为2,矩阵的行列形状不变mat=mat.reshape(2);cv::undistortPoints(mat, mat, K, D, cv::Mat(), newCamMatrix);mat=mat.reshape(1);// 存储校正后的特征点for(int i=0; i<N; i++){cv::Point2f kp = points[i];kp.x=mat.at<float>(i,0);kp.y=mat.at<float>(i,1);points[i] = kp;}
}
先使用cv::goodFeaturesToTrack函数在图像中提取一些FAST角点,如图3所示。
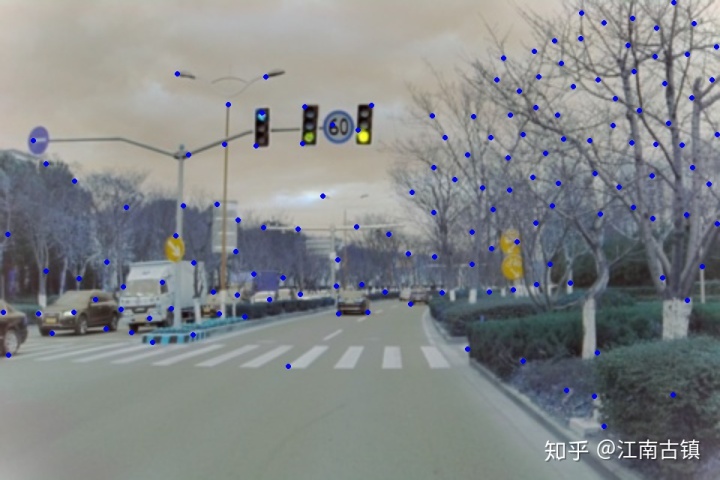
然后对这些角点去畸变,注意,我们矫正后的点是不能直接打印在原图像上的,所以我们需要先对图像去畸变(这里的两种方法就是为了应对两种不同的图像矫正),然后将矫正后的点打印在矫正后的图像上。矫正结果如图3所示。
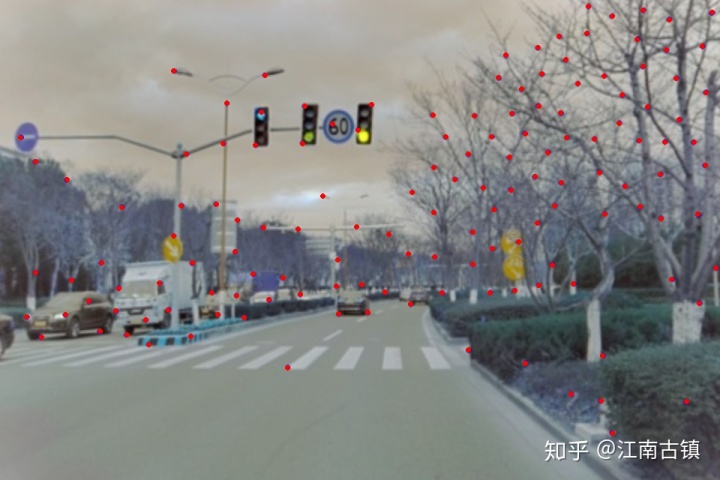
注意,undistortPoints和undistort函数都有newCameraMatrix参数,这两个函数要使用相同的newCameraMatrix参数,这样才能将去畸变的点和去畸变的图像对应起来,这里的newCameraMatrix使用原相机内参。
2.4 undistortPoints 矫正BoundingBox
//
// Created by jiang on 2020/4/29.
//
#include <iostream>
#include <opencv2/opencv.hpp>using namespace std;const cv::Mat K = ( cv::Mat_<double> ( 3,3 ) << 931.73, 0.0, 480.0, 0.0, 933.16, 302.0, 0.0, 0.0, 1.0 );
const cv::Mat D = ( cv::Mat_<double> ( 5,1 ) << -1.7165e-1, 1.968259e-1, 0.0, 0.0, -3.639514e-1 );void UndistortBbox(cv::Rect &rect, cv::Mat &newCamMatrix);int main()
{const string str = "/home/jiang/4_learn/WeChatCode/ImageUndistort/data/";const int nImage = 5;const int ImgWidth = 960;const int ImgHeight = 640;cv::Mat map1, map2;cv::Size imageSize(ImgWidth, ImgHeight);const double alpha = 1;cv::Mat NewCameraMatrix = getOptimalNewCameraMatrix(K, D, imageSize, alpha, imageSize, 0);initUndistortRectifyMap(K, D, cv::Mat(), NewCameraMatrix, imageSize, CV_16SC2, map1, map2);cv::Rect Bbox{338, 141, 23, 57};for(int i=0; i<nImage; i++){string InputPath = str + to_string(i) + ".png";cv::Mat RawImage = cv::imread(InputPath);cv::Mat UndistortImage;cv::remap(RawImage, UndistortImage, map1, map2, cv::INTER_LINEAR);
// cv::undistort(RawImage, UndistortImage, K, D, K);cv::rectangle(RawImage, Bbox, cv::Scalar(255, 0, 0), 2, 1);cv::imshow("RawImage", RawImage);string OutputPath1 = str + to_string(i) + "_Bbox" + ".png";cv::imwrite(OutputPath1, RawImage);UndistortBbox(Bbox, NewCameraMatrix);cv::rectangle(UndistortImage, Bbox, cv::Scalar(0, 0, 255), 2, 1);cv::imshow("UndistortImage", UndistortImage);string OutputPath2 = str + to_string(i) + "_Bbox_un" + ".png";cv::imwrite(OutputPath2, UndistortImage);cv::waitKey(0);}return 0;
}void UndistortBbox(cv::Rect &rect, cv::Mat &newCamMatrix)
{cv::Mat mat(4, 2, CV_32F);mat.at<float>(0, 0) = rect.x;mat.at<float>(0, 1) = rect.y;mat.at<float>(1, 0) = rect.x + rect.width;mat.at<float>(1, 1) = rect.y;mat.at<float>(2, 0) = rect.x;mat.at<float>(2, 1) = rect.y + rect.height;mat.at<float>(3, 0) = rect.x + rect.width;mat.at<float>(3, 1) = rect.y + rect.height;mat = mat.reshape(2); // 2通道,行列不变cv::undistortPoints(mat, mat, K, D, cv::Mat(), newCamMatrix);mat = mat.reshape(1); // 单通道,行列不变double MaxX, MaxY;rect.x = min(mat.at<float>(0, 0), mat.at<float>(2, 0));MaxX = max(mat.at<float>(1, 0), mat.at<float>(3, 0));rect.y = min(mat.at<float>(0, 1), mat.at<float>(1, 1));MaxY = max(mat.at<float>(2, 1), mat.at<float>(3, 1));rect.width = MaxX - rect.x;rect.height = MaxY - rect.y;
}
这里我们手动画了一个Bbox{338,141,23,57}。然后用remap的方式矫正了图像。
在矫正BoundingBox也就是矫正BoundingBox的四个点。只是这里有一个细节要考虑,就是Bbox的四个角点在矫正后的大小不确定,因此要判断一下。矫正前的图像如图5所示,矫正后的图像如图6所示。
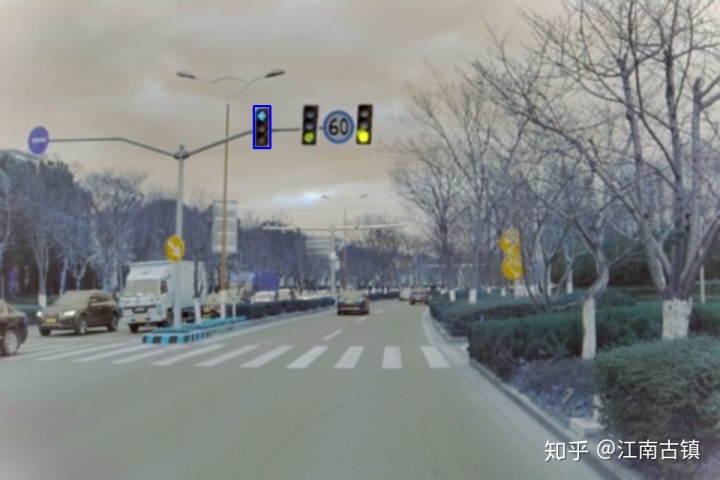
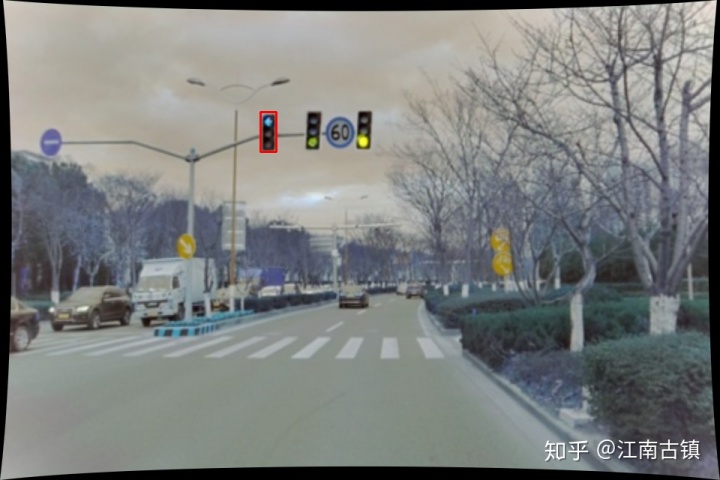
3. 总结与补充
关于图像、角点和Bbox的去畸变就介绍到这里,后续有更深入的研究在加以补充。本人才疏学浅,如文章中有错误,还请读者不吝赐教,吾当感激不尽。
文中用的opencv版本是3.3.0,CMakeLists如下:
cmake_minimum_required(VERSION 3.14)
project(ImageUndistort)set(CMAKE_CXX_STANDARD 11)set(OpenCV_DIR "/home/jiang/6_lib/install/opencv3.3.0/share/OpenCV")find_package(OpenCV REQUIRED)add_executable(undistort2_1 undistort2_1.cpp)
target_link_libraries(undistort2_1${OpenCV_LIBS}
)add_executable(undistort2_2 undistort2_2.cpp)
target_link_libraries(undistort2_2${OpenCV_LIBS})add_executable(undistort2_3_1 undistort2_3_1.cpp)
target_link_libraries(undistort2_3_1${OpenCV_LIBS})add_executable(undistort2_3_2 undistort2_3_2.cpp)
target_link_libraries(undistort2_3_2${OpenCV_LIBS})add_executable(undistort2_4 undistort2_4.cpp)
target_link_libraries(undistort2_4${OpenCV_LIBS})
完整的代码在这里:
https://github.com/JiangZhengok/WeChatCode/tree/master/ImageUndistortgithub.com