实验人员考评指标
In the first part of my series on experimental design Thinking About Experimental Design, we covered the foundations of an experiment: the goals, the conditions, and the metrics. In this post, we will move away from the initial experimental set up to begin understanding baseline metrics and the nuances of picking appropriate conversion metrics and rates.
在我的实验设计系列的第一部分中,“ 思考实验设计”中 ,我们介绍了实验的基础:目标,条件和度量。 在这篇文章中,我们将脱离最初的实验设置,开始了解基线指标以及选择合适的转化指标和费率的细微差别。
介绍 (Introduction)
I introduced the goals of an experiment a business context, using the example of a lemonade stand: measuring the difference in outcomes (# of cups sold) under controlled conditions (color of cup) during comparable time-frames. In the example, I failed to mention a critical element of any experiment — a hypothesis, a preliminary presumption based on limited evidence. Strictly speaking, the goal of an experiment is to validate or refute ideas put forth by a hypothesis. A reasonable hypothesis for our lemonade stand example might be that “the color of the lemonade cup affects the number of cups sold.”
我以柠檬水摊位为例,介绍了在商业环境中进行实验的目标:在可比较的时间范围内,在受控条件 (杯子的颜色)下测量结果 (所售杯子的数量)的差异 。 在示例中,我没有提到任何实验的关键要素- 假设 ,即基于有限证据的初步假设。 严格来说,实验的目的是验证或驳斥假设提出的想法。 我们的柠檬水摊位示例的合理假设可能是“柠檬水杯的颜色会影响所售杯子的数量”。
从基线开始 (Starting from a Baseline)
If we’re just starting our lemonade stand (or other small business/business line), can you see a problem with immediately designing this particular experiment (or any experiment) to validate a hypothesis? Market research aside, we don’t have any reliable (backed by hard-data) predictions on what to expect for our number of cups sold!
如果我们只是开始我们的柠檬水摊位(或其他小型企业/业务线),您是否会立即设计此特定实验(或任何实验)以验证假设是否存在问题? 除了市场研究,我们对售出的杯子数量还没有可靠的预测(有硬数据支持)!
If we have poor sales the first two weeks with red cups, followed by boosted sales in the next two weeks with blue cups, I wouldn’t be comfortable concluding that blue cups are superior. The sudden change in sales may be due to the fact that people didn’t know about our stand in its opening weeks, and only recently discovered our stand. Remember, the goal of an experiment is to understand the effects of incremental changes. When we introduce a big change (ie. starting our stand or completely revamping our storefront), we introduce instability into our existing business, which will mask and/or distort the effect of our experimental conditions.
如果我们在头两周使用红色杯子的销售情况不佳,然后在接下来的两周使用蓝色杯子的销售量有所增长,那么我不能肯定地说蓝色杯子是上乘的。 销售量的突然变化可能是由于人们在开放的几周内并不了解我们的展位,而只是在最近才发现了我们的展位。 请记住, 实验的目的是了解增量变化的影响。 当我们进行重大更改时(即启动我们的展位或完全改造店面),我们会将不稳定因素引入现有业务,这将掩盖和/或扭曲我们的实验条件的影响。
Before conducting an experiment it’s important to establish a stable baseline that will be used the judge the effects our incremental changes.
在进行实验之前,重要的是要建立一个稳定的基线,该基线将用于判断我们的增量变化的影响。
To make our example more concrete, let’s say we’ve been running our lemonade stand for 1.5 years. We’re relatively well-known in the community, but we’ve run out of ideas on how we can continue growing our business. After prioritizing our business goals and brainstorming relevant conversion metrics and rates*, we’ve decided to analyze the conversion metric:
为了使我们的示例更具体,假设我们已经运行柠檬水摊位1.5年了。 我们在社区中相对知名,但是关于如何继续发展业务的想法已经耗尽。 在确定了业务目标的优先级并集体讨论了相关的转化指标和转化率*之后,我们决定分析转化指标:
# of cups sold / people who stop by our stand.
售出的杯子数/站在我们展位旁的人。
I have generated some dummy data representing our monthly sales for the past year. The relative stability of our business with respect to the conversion rate. Now, when we introduce our experimental condition (changing the color of the cup), we have a reliably predictable baseline conversion rate (3.62% average) with which we can compare our new outcome.
我已经生成了一些虚拟数据,这些数据代表了过去一年的每月销售额。 我们的业务相对于转换率的相对稳定性。 现在,当我们介绍实验条件(改变杯子的颜色)时,我们有了可靠的可预测的基线转化率(平均3.62%),可以与我们比较新的结果。
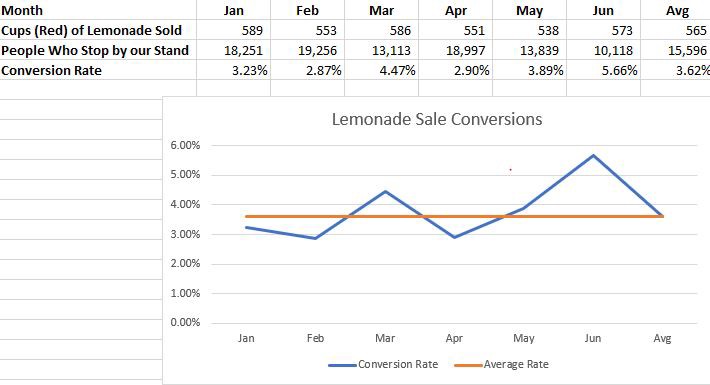
考虑转化率的变化 (Thinking about changes in our conversion rate)
At this point, it is easy to forget that our target metric is a conversion rate and begin brainstorming incremental changes that increase # of cups sold. The use of a conversion rate instead of an absolute value requires us to expand our focus from a single metric, to the relationship between two related metrics — from a focus on scale to a focus on scale & efficiency.
在这一点上,很容易忘记我们的目标指标是转化率,并开始集思广益,以增加销售杯数的增量变化。 使用转换率而非绝对值要求我们将重点从单一指标扩展到两个相关指标之间的关系-从关注规模到关注规模和效率。
To increase our conversion rate, we must develop a strategy to increase the number of cups sold at a greater rate than we increase the number of people who stop by our stand. Altering the color or design of the cup may be an interesting business initiative; this is assuming that we believe people stop by our stand mostly for the lemonade and our beautiful stand and not due to the color of the cup.
为了提高转化率,我们必须制定一项战略,以比增加增加在我们展台前停留的人数更多的速度增加杯子的销售数量。 改变杯子的颜色或设计可能是一个有趣的商业尝试; 这是假设我们相信人们主要是为了柠檬水和漂亮的立场而停下来,而不是因为杯子的颜色。
From a business perspective, we often read about using data and experiments to deliver actionable insights. In addition to our baseline rate, it is important to set reasonable target rates under each of our experimental conditions; we do not want to be taking action on any small change in conversion rates. Setting a target conversion rate is as much an art as it is a science, and can be based on a combination of past data and intuitive business sense. In our lemonade example, we might say that we will switch the color of our cups moving forward if our conversion rate is 4.12% over the next couple of months, an increase of .5%.
从业务角度来看,我们经常阅读有关使用数据和实验来提供可行见解的信息。 除了我们的基准速率外,在每个实验条件下设定合理的目标速率也很重要; 我们不希望对转化率的任何小变化采取行动。 设定目标转化率既是一门艺术,也是一门科学,并且可以基于过去的数据和直观的商业意识相结合。 在我们的柠檬水示例中,我们可以说,如果未来几个月我们的转化率为4.12%(增加0.5%),我们将改变杯子的颜色。
结论: (Conclusion:)
To summarize what we have accomplished in our lemonade example:
总结一下我们在柠檬水示例中所取得的成就:
We defined our business goal: increase cups sold
我们确定了我们的业务目标: 增加杯子销量
2. We defined our conversion metric and conversion rate: cups sold & cups sold / foot traffic
2.我们定义了转化指标和转化率: 售出杯数和售出杯数/人流量
3. We developed a controlled incremental change that will (hypothetically) affect our outcome
3.我们开发了受控的增量更改,该更改将(假设地)影响我们的结果
4. We established a stable baseline for comparison.
4.我们建立了稳定的基线进行比较。
If our conversion metric achieves the target goal, we can conduct business under the new conditions moving forward right? Well, not quite.
如果我们的转化指标实现了目标,那么我们可以在新的条件下开展业务吗? 好吧,不完全是。
So far, you may have realized that I have not introduced any statistics in our experiment! At this point, it may not be entirely clear why we need statistics to validate our hypothesis and complete our experiment. Nonetheless, when dealing with such uncertainty, we want a way of quantifying our decision-making process; we use statistics quantify the strength of our experimental evidence. In the next article of this series, I will begin introducing ideas of basic statistical concepts and test statistics as they apply to our experimental design.
到目前为止,您可能已经意识到我没有在实验中引入任何统计信息! 在这一点上,可能还不清楚,为什么我们需要统计数据来验证我们的假设并完成我们的实验。 但是,在处理此类不确定性时,我们需要一种量化决策过程的方法。 我们使用统计数据来量化我们的实验证据的强度。 在本系列的下一篇文章中,我将开始介绍适用于我们的实验设计的基本统计概念和测试统计概念。
[1]: Monika Wahi. (2020). The Data Science of Experimental Design. LinkedIn Learning. https://www.linkedin.com/learning/the-data-science-of-experimental-design
[1]:莫妮卡·瓦希(Monika Wahi)。 (2020)。 实验设计的数据科学。 LinkedIn学习。 https://www.linkedin.com/learning/the-data-science-of-experimental-design
[2]: Icons & Images. Pexels: https://www.pexels.com/
[2]: 图标和图像。 Pexels: https ://www.pexels.com/
翻译自: https://towardsdatascience.com/understanding-experiment-metrics-ecb0d759f743
实验人员考评指标
本文来自互联网用户投稿,该文观点仅代表作者本人,不代表本站立场。本站仅提供信息存储空间服务,不拥有所有权,不承担相关法律责任。如若转载,请注明出处:http://www.mzph.cn/news/392414.shtml
如若内容造成侵权/违法违规/事实不符,请联系多彩编程网进行投诉反馈email:809451989@qq.com,一经查实,立即删除!