鸽子 迷信
鸽子回避系统 (Pigeon Avoidance System)
Disclaimer: You are reading Part 1 that gives an overview of the project. Part 2 describes the technical setup and data collection. Part 3 is about how to train the Pigeon Recognition Model and run it on Raspberry Pi.
免责声明:您正在阅读第1部分,该部分概述了该项目。 第2部分 介绍了技术设置和数据收集。 第3部分 介绍如何训练Pigeon识别模型并在Raspberry Pi上运行它。
Imagine a beautiful summer morning. The sun is shining through the leaves, the birds are singing happy songs, and everything is full of cheerful colors. You make your first cup of coffee and step on the balcony to enjoy the peace and harmony that only Sunday mornings can provide. Suddenly something disrupts you from the contemplation of the beauty of terrestrial life. In my world, it is the pigeon poop that I find on my balcony almost every day. I am a busy woman. I like enjoying my morning coffee in peace, but what I do not like — to clean the balcony from pigeon’s feces.
想象一个美丽的夏日早晨。 阳光穿过树叶,鸟儿唱着快乐的歌,一切都充满了欢快的色彩。 您冲泡第一杯咖啡,踏上阳台,享受只有周日早晨才能提供的和平与和谐。 突然间,某些事情干扰了您对地球生命之美的沉思。 在我的世界中,几乎每天我都会在阳台上发现鸽子便便。 我是忙碌的女人。 我喜欢安宁地享受早晨的咖啡,但是我不喜欢这样-清洁鸽子粪便上的阳台。
This morning I decided to start a war against the pigeons and Artificial Intelligence is the right weapon for this.
今天早上,我决定发动一场与鸽子的战争,而人工智能是解决这一问题的正确武器。
Now I set the emotional part aside and jump straight to the business of engineering, wiring, modeling, and soldering.
现在,我将情感部分搁置一旁,直接进入工程,布线,建模和焊接业务。
问题陈述 (Problem statement)
Pigeons regularly visit my balcony and left certain traces there. I want to get rid of pigeons without harming them, but by delivering a clear message that they are not welcomed here.
鸽子定期拜访我的阳台,并在那留下一些痕迹。 我想摆脱鸽子而不伤害他们,但是要传达一个明确的信息,那就是这里不欢迎他们。
解决方法 (Solution approach)
I have built a Pigeon Avoidance System that detects pigeons and shoos them away. The initial hardware setup includes:
我建立了一个避免鸽子的系统,该系统可以检测并驱赶鸽子。 初始硬件设置包括:
Raspberry Pi. I used Raspberry Pi 4 Model B 8GB RAM
Raspberry Pi 。 我使用了Raspberry Pi 4 Model B 8GB RAM
Camera module for Raspberry
覆盆子相机模组
Two motion sensors
两个运动传感器
Stepping motor
步进马达
Software stack:
软件堆栈:
- Python for the automation 自动化的Python
- Keras for the Deep Learning part 深度学习部分的Keras
- PHP, JavaScript, HTML for data labelling solution PHP,JavaScript,HTML数据标签解决方案
架构概述 (Architecture overview)
The overall solution works in the following way. Whenever a pigeon lands on the balcony, the motion sensor detects a light change. It triggers the Main Pipeline, which in return activates the camera. The camera takes a picture and stores it on the Raspberry. The Main Pipeline sends the picture to the Pigeon Recognition Model that calculates the probability of a pigeon being on the picture and returns back the class. If a pigeon was detected, the Main Pipeline activates the stepping motor that shoos pigeon away by lifting up a stick with ribbons. Sounds pretty straightforward, though effective.
整体解决方案以以下方式工作。 每当鸽子降落在阳台上时,运动传感器就会检测到光线变化。 它触发了主管道,而主管道反过来又激活了摄像机。 相机拍摄照片并将其存储在Raspberry上。 主管道将图片发送到“鸽子识别模型”,该模型计算鸽子出现在图片上的概率,并返回该类。 如果检测到鸽子,则主管道会通过抬起带有色带的棍子来激活步进电机,以驱赶鸽子。 听起来很简单,虽然有效。

为什么您可能需要鸽子火种 (Why you might need a Pigeon Tinder)
There is one element on the architecture diagram that hasn’t been explained so far, the Pigeon Tinder. That is exactly the moment when people usually raising their eyebrows asking: “What the hell is the Pigeon Tinder?” Pigeon Tinder is an essential part of the overall solution. Apparently, in order to train the Pigeon Recognition Model, I need to feed labeled data into it. Pigeon Tinder is a web app hosted on the Raspberry that helps me to manually label the images. Initially, I wanted to implement a mobile app where the left swipe would label the picture as “Not a Pigeon” and the right swipe as “Pigeon”. However, shortly I realized that first, mobile app development is a bit of overkill for the Minimal Viable Product (MVP). Second, in reality, I need three classes, as whenever I drink coffee on the balcony I would produce a lot of motion and the Raspberry would go off by making hundreds of pictures and then trying to classify them. Therefore I introduced a class “Human” that causes a two minutes delay before the next picture is taken. My main hope is that pigeons are not impudent enough to get on the balcony while I am already there. Because of these reasons, I settled for a web app running on Apache HTTP Server installed on the Raspberry. That how the interface of the Pigeon Tinder looks like.
到目前为止,尚未解释的架构图上有一个要素。 这正是人们通常扬起眉毛问:“鸽子火种到底是什么?”的时刻。 Pigeon Tinder是整个解决方案的重要组成部分。 显然,为了训练Pigeon Recognition Model,我需要将标记的数据馈入其中。 Pigeon Tinder是Raspberry上托管的Web应用程序,可帮助我手动标记图像。 最初,我想实现一个移动应用程序,其中向左滑动将图片标记为“不是鸽子”,向右滑动将标签标记为“鸽子”。 但是,我很快意识到,首先,移动应用程序开发对于最小可行产品(MVP)有点过大。 其次,实际上,我需要三个课程,因为每当我在阳台上喝咖啡时,我都会产生很多动作,而Raspberry会通过制作数百张图片然后尝试对其进行分类而消失。 因此,我引入了“人类”类,该类在拍摄下一张照片之前会造成两分钟的延迟。 我的主要希望是,在我已经呆在阳台上的时候,鸽子还不够轻率。 由于这些原因,我决定在Raspberry上安装的Apache HTTP Server上运行一个Web应用程序。 鸽子火种的界面是什么样的。

As I prefer to tinder pigeons on my phone, the web page is adapted for mobile usage. The app is very simple. Whenever I assign a label to the image, it moves the picture into one of the folders: “pigeon”, “human”, or “nothing”. That is also a proper directory structure for future training. The Delete button temporarily moves the picture into a “trash” folder that is regularly emptied by the clean_directories.py script that is run by a cron job every night.
由于我更喜欢在手机上打鸽子,因此该网页适合移动应用。 该应用程序非常简单。 每当我为图像分配标签时,它就会将图片移动到以下文件夹之一:“鸽子”,“人”或“无”。 这也是将来培训的合适目录结构。 “删除”按钮将图片临时移动到“垃圾箱”文件夹中,该文件夹定期由cron作业每晚运行的clean_directories.py脚本清空。
By the way, all code, including training routine, inference, Pigeon Tinder, and Raspberry automation is available on GitHub.
顺便说一下,所有代码,包括训练例程,推理,Pigeon Tinder和Raspberry自动化,都可以在GitHub上获得 。
Even though I didn’t go for the original idea of the mobile app, I still kept the original name of the labeling module. I hope Tinder will not sue me for this, otherwise you will hear about me in the news.
即使我没有使用移动应用程序的原始想法,但仍保留了标签模块的原始名称。 我希望Tinder不会为此起诉我,否则您会在新闻中听到关于我的消息。
接下来是什么? (What is next?)
I am documenting this project in a few articles under the name “Pigeon Avoidance System”. Part 2: “How to set up data collection for the pigeon avoidance system” will explain how to access external Raspberry components like camera, motion sensor, and stepping engine. It will also provide details about the data collection and how to set up the overall system on the balcony. The Deep Learning part is described in: “How to use Deep Learning to shoo the pigeons from the balcony”. However, I would recommend reading the article about the data collection first. So stay updated and in the meantime, a sneak peek for Part 2: me soldering cables in my kitchen. I indeed need a lot of cables for this project.
我正在以“ Pigeon躲避系统”的名义在几篇文章中记录该项目。 第2部分:“ 如何为鸽子回避系统设置数据收集 ”将说明如何访问外部Raspberry组件,例如摄像头,运动传感器和步进引擎。 它还将提供有关数据收集以及如何在阳台上设置整个系统的详细信息。 深度学习部分在“ 如何使用深度学习从阳台上射击鸽子 ”中进行了描述。 但是,我建议您先阅读有关数据收集的文章。 因此,请保持更新,与此同时, 第2部分 :我在厨房焊接电缆。 对于这个项目,我确实需要很多电缆。
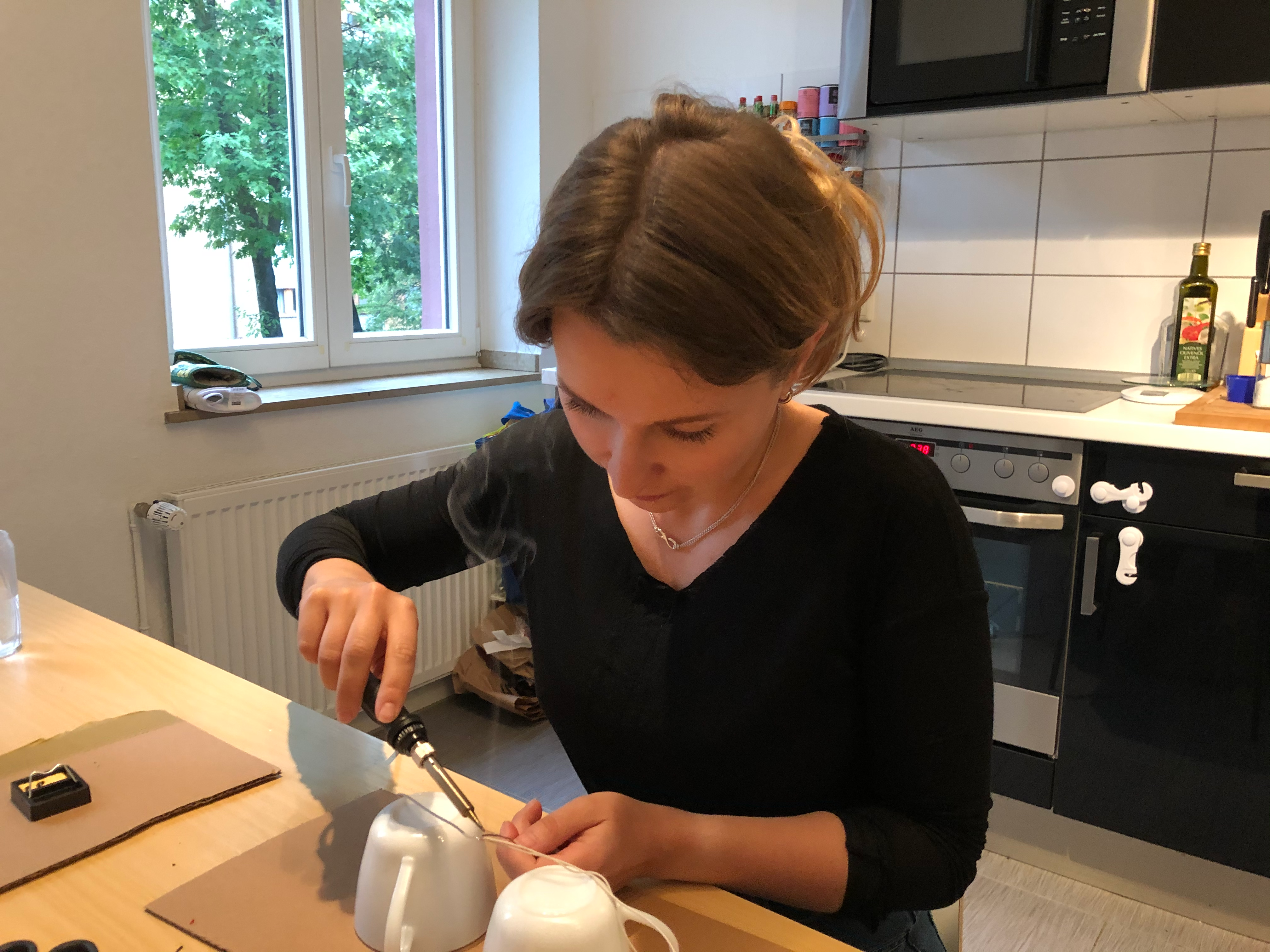
If you got any questions about this project, please feel free to reach me via LinkedIn.
如果您对此项目有任何疑问,请随时通过LinkedIn与我联系。
翻译自: https://towardsdatascience.com/how-artificial-intelligence-helped-me-to-win-the-war-against-the-pigeons-9458293983a1
鸽子 迷信
本文来自互联网用户投稿,该文观点仅代表作者本人,不代表本站立场。本站仅提供信息存储空间服务,不拥有所有权,不承担相关法律责任。如若转载,请注明出处:http://www.mzph.cn/news/392024.shtml
如若内容造成侵权/违法违规/事实不符,请联系多彩编程网进行投诉反馈email:809451989@qq.com,一经查实,立即删除!