scikit keras
Welcome all! In the first episode of this series, I investigated the four most known machine learning frameworks and discussed which of these you should learn depending on your needs and goals.
w ^迎阅读所有! 在本系列的第一集中 ,我研究了四种最著名的机器学习框架,并讨论了根据您的需求和目标应该学习的机器学习框架。
Of course, we all know how PyTorch and TensorFlow are overwhelmingly effective and thorough when it comes to building deep learning algorithms from scratch. Similarly, Scikit-learn comes with excellent non-neural solutions and a whole lot of convenient data processing and evaluation functions.
当然,当我们从头开始构建深度学习算法时,我们都知道PyTorch和TensorFlow如何具有压倒性的优势和全面性。 同样, Scikit-learn具有出色的非神经解决方案以及大量便捷的数据处理和评估功能。
Today, I would like to draw your attention to something different: a rising machine learning framework, Libra, that absolutely deserves your attention and has tripled its amount of GitHub stars in only a week (reaching 1.8K)!
今天,我想提请您注意一些不同的东西:新兴的机器学习框架Libra绝对值得您的注意,并且在短短一周内将GitHub star的数量增加了两倍(达到1.8K)!
In this article, I will cover:
在本文中,我将介绍:
The basic concept of the framework
框架的基本概念
The interesting features I spotted
我发现的有趣功能
The expected downsides
预期的缺点
A concrete use example
具体的使用示例
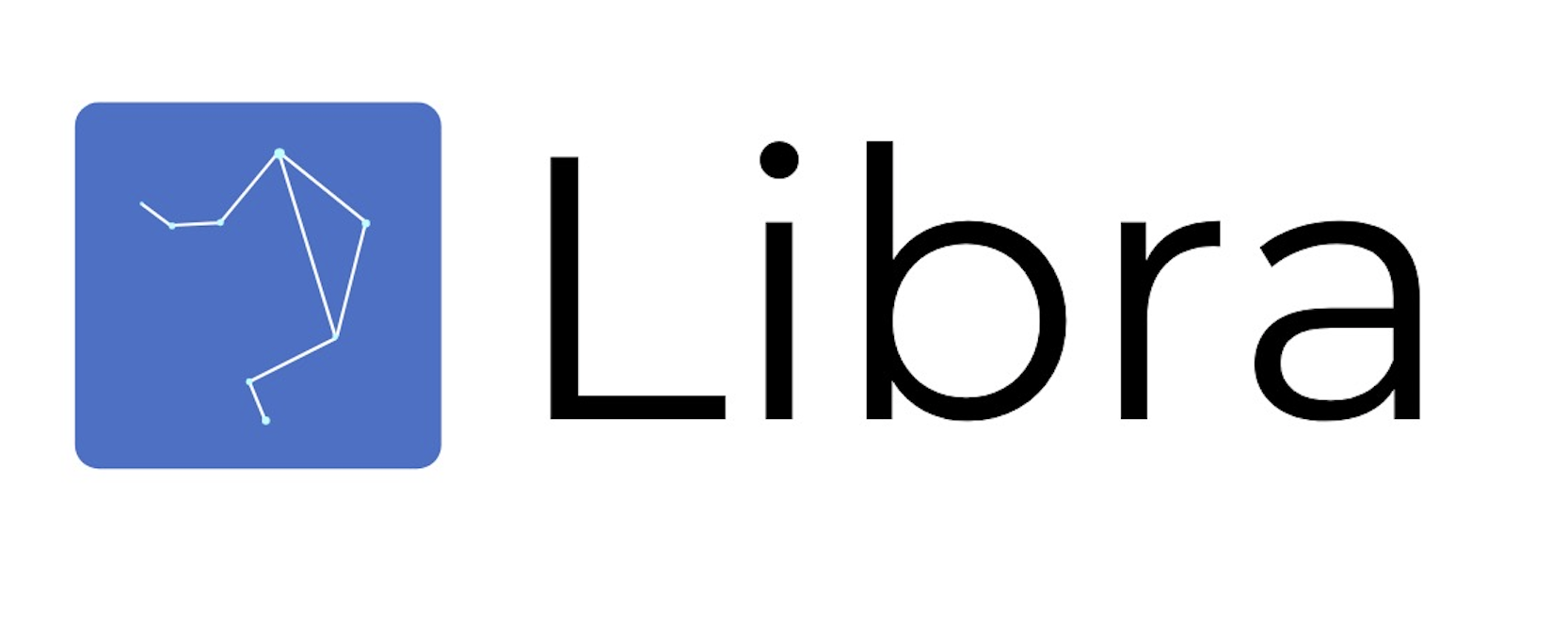
一,基本概念 (I. Base Concept)
Libra is an easy-to-use machine learning framework that will allow you to load data, process it, train models and visualize results with only a few lines of code.
Libra是一个易于使用的机器学习框架,使您仅需几行代码即可加载数据,处理数据,训练模型并可视化结果。
The major advantage of this framework is its user-friendliness and beginner-friendliness. The diagram below shows about how fast you can setup an ML project compared to more common frameworks:
这个框架的主要优点是它的用户友好性和初学者友好性 。 下图显示了与更常见的框架相比,您可以更快地设置ML项目:

This literally means that you can come with no or nearly no technical machine learning knowledge and implement a full project in about 5 minutes.
从字面上看,这意味着您可能没有或几乎没有任何机器学习知识,而仅需5分钟即可实现一个完整的项目。
The library is built for Python (⩾3.6) and revolves around a Client object that will handle your data but also the models you want to build, inference, plotting, etc.
该库是为Python(⩾3.6)构建的,围绕一个Client对象运行,该对象不仅可以处理数据,还可以处理要构建,推断,绘制等的模型。
You can easily install and initialize the library in a project with only lines of code: first, you need to install Libra and all the dependencies in your environment with pip install libra
. Next, simply write from libra import client
in a Python file and you’re all set!
您只需使用几行代码即可轻松地在项目中安装和初始化该库:首先,您需要使用pip install libra
安装Libra以及环境中的所有依赖项。 接下来,只需from libra import client
编写一个Python文件即可,一切就绪!
二。 有趣的功能 (II. Interesting Features)
The first interesting feature I noticed is that some “queries” try to infer what is asked of them: for example, if you call a neural_network_query(), the only required argument is a quick explanation of what you want to do, like “please model the median number of households”.
我注意到的第一个有趣的功能是一些“查询” 尝试推断出他们的要求 :例如,如果您调用Neuro_network_query() ,则唯一需要的参数是对您想做什么的快速说明,例如“请模拟住户中位数”。
The target variable in your data will then be determined through the parsing of your explanation and computing the levenshtein distance on the column names.
然后,将通过解析您的解释并计算列名上的levenshtein区别来确定数据中的目标变量。
Kind of cool, right?
有点酷吧?
Moreover, you can simply load your .csv data file and it will automatically be handled in a Pandas DataFrame and preprocessed for you during the query, which is convenient if you want fast results!
此外,您只需加载.csv数据文件,它将在Pandas DataFrame中自动处理并在查询过程中为您进行预处理,如果您想要快速的结果,这将非常方便!
The fact that Libra relies on other machine learning libraries means that all ML tasks are virtually doable. Here is a list of the currently available queries, and includes Feedforward NN, CNN, SVM, Text generation, Sentiment analysis, etc.
Libra依赖于其他机器学习库这一事实意味着所有ML任务实际上都是可行的。 这是当前可用查询的列表,包括前馈NN,CNN,SVM,文本生成,情感分析等。
When you fine tune a model in with the Libra client, the evaluation results and plots are automatically displayed for you. Here is an example where I tried to predict sentiment scores of tweets from this Kaggle dataset using a simple neural network:
当您使用Libra客户端微调模型时,评估结果和图将自动为您显示。 这是一个示例,我尝试使用简单的神经网络从该Kaggle数据集中预测推文的情感评分:

The project is growing pretty fast and even though the core development has been done, you can put your skills to use and contribute by adding missing bits of code and correcting bugs!
该项目正在Swift发展,即使完成了核心开发,您也可以通过添加缺少的代码并更正错误来利用和贡献自己的技能!
三, 缺点 (III. Downsides)
The major downside you will experience with an ML framework that is so beginner-friendly is process over-simplification: by providing such high-level wrapping techniques, you sort of lose the sense of control over what is happening.
ML框架对初学者非常友好,这将给您带来的主要缺点是过分简化了流程 :通过提供这种高级包装技术,您会失去对正在发生的事情的控制感。
For example, when using the “neural network query”, the number of distinct target variables in your data will determine if a classification or regression task will be performed, and you will not have a choice in the neural net’s architecture details.
例如,当使用“神经网络查询”时,数据中不同目标变量的数量将确定是否要执行分类或回归任务,并且您将无法选择神经网络的体系结构详细信息。
As of today (August 2020), Libra is still being developed by the creators and the community, meaning that a lot of bugs appear here and there, which can be discouraging at first.
截至今天(2020年8月),天秤座仍由创作者和社区开发,这意味着到处都会出现许多错误 ,一开始可能会令人沮丧。
Even though Libra is a relatively new machine learning framework, it hasn’t (yet) re-invented the wheel, and relies on popular libraries like Keras, Transformers, Scikit-learn and NLTK. This mainly means that the number of hard dependencies is quite high, and can be an issue if you like to keep a light environment.
尽管Libra是一个相对较新的机器学习框架,但它(尚未)重新发明轮子,而是依赖于Keras,Transformers,Scikit-learn和NLTK等流行的库。 这主要意味着硬依赖性的数量非常高 ,如果您希望保持一个轻量级的环境,则可能会成为一个问题。
IV。 简单项目示例 (IV. Simple Project Example)
To show you how easy and fun it is to use Libra, let’s implement a small project together: based on the beginning of J.R.R Tolkien’s Silmarillion, we will generate new text with only 3 lines of code.
为了向您展示使用Libra是多么容易和有趣,让我们一起实现一个小项目:基于JRR Tolkien的Silmarillion的开头,我们将只用3行代码生成新文本 。
from libra import clienttolkien_client = client('silma_beg.txt')
tolkien_client.generate_text("generate some text please", return_sequences=1)
print(tolkien_client.models['text_generation']['generated_text'])
Libra will load GPT-2 from OpenAI and generate new text for us.
天秤座将从OpenAI加载GPT-2并为我们生成新文本。
In the following Gist, the first line is actually the input file I fed the network with. All the rest was automatically output by the model, and it’s pretty amazing!
在下面的要点中,第一行实际上是我为网络提供的输入文件。 其余的全部由模型自动输出,这真是太神奇了!
There was Eru, the One, who in Arda is called Ilúvatar; and he made first the Ainur, the Holy Ones, that were the offspring of his thought, and they were with him before aught else was made. And he spoke to them, propounding to them themes of music; and they sang before him, and he was glad. But for a long while they sang only each alone, or but few together, while the rest hearkened; for each comprehended only that part of me mind of Ilúvatar from which he came, and in the understanding of their brethren they grew but slowly. Yet ever as they listened they came to deeper understanding, and increased in unison and harmony. And the time came when they saw that, at last, by the love which the people gave her, there had been a way of getting over her by the love of her Creator. And they arose from their hiding place, and sought the land in the wilderness, and there they were put to the slaughter, for they were of the blood of the sons of Ilúvatar, and it was not easy to get over them. For those who had been left by their parents, as it were, had come to know the true nature of what they had done, and to believe that they had done what had been done, and that they had found the love of the Holy Ones; but Ilúvatar said to the other:Thy sons are born of the Father, and thou shalt have their hearts at my feet, and thou shalt have my prayers to thee in the heavens.And the angels said to Ilúvatar:Father, take these people, O Ilúvatar; make them come back.And Ilúvatar said unto them:Thou are worthy, O Ilúvatar, of these sons.And when they were come back to their hiding place, they were put to the slaughter; for they had seen that their father was not in the way of righteousness, but rather from a power of the Holy One, whom they saw the sons of Ilúvatar.And the angels, then, said unto Ilúvatar:The love of God, Ilúvatar, is strong and great; and thou shalt be glad, O Ilúvatar, if thou hast the Holy One with you. For there are few who ever have been so much as children, and
The model probably was pre-trained on the entire book, but the output grammar and generation relevance is nonetheless very good.
该模型可能已在整本书中进行了预训练,但是输出语法和生成相关性仍然非常好。
This is what Libra is about: creating with only a handful of lines of code. The opportunity to load, train, infer, evaluate and plot without prior extended knowledge.
这就是Libra的目的: 只用几行代码创建 。 在没有事先扩展知识的情况下进行加载,训练,推断,评估和绘图的机会。
I hope you have enjoyed this article, thank you very much for reading through and make sure to try out this framework when you get the chance!
我希望您喜欢这篇文章,非常感谢您通读,并确保在有机会的情况下尝试使用此框架!
翻译自: https://towardsdatascience.com/scikit-learn-tensorflow-pytorch-keras-but-what-about-libra-a5102c2d834d
scikit keras
本文来自互联网用户投稿,该文观点仅代表作者本人,不代表本站立场。本站仅提供信息存储空间服务,不拥有所有权,不承担相关法律责任。如若转载,请注明出处:http://www.mzph.cn/news/392016.shtml
如若内容造成侵权/违法违规/事实不符,请联系多彩编程网进行投诉反馈email:809451989@qq.com,一经查实,立即删除!