I am an avid Youtube user and love watching videos on it in my free time. I decided to do some exploratory data analysis on the youtube videos streamed in the US. I found the dataset on the Kaggle on this link
我是YouTube的狂热用户,喜欢在业余时间观看视频。 我决定对在美国播放的youtube视频进行一些探索性数据分析。 我在此链接的Kaggle上找到了数据集
I downloaded the csv file ‘USvidoes.csv’ and the json file ‘US_category_id.json’ among all the geography-wise datasets available. I have used Jupyter notebook for the purpose of this analysis.
我在所有可用的地理区域数据集中下载了csv文件“ USvidoes.csv”和json文件“ US_category_id.json”。 我已使用Jupyter笔记本进行此分析。
让我们开始吧! (Let's get started!)
Loading the necessary libraries
加载必要的库
import pandas as pdimport numpy as npimport seaborn as snsimport matplotlib.pyplot as pltimport osfrom subprocess import check_outputfrom wordcloud import WordCloud, STOPWORDSimport stringimport re import nltkfrom nltk.corpus import stopwordsfrom nltk import pos_tagfrom nltk.stem.wordnet import WordNetLemmatizer from nltk.tokenize import word_tokenizefrom nltk.tokenize import TweetTokenizer
I created a dataframe by the name ‘df_you’ which will be used throughout the course of the analysis.
我创建了一个名为“ df_you”的数据框,该数据框将在整个分析过程中使用。
df_you = pd.read_csv(r"...\Projects\US Youtube - Python\USvideos.csv")
The foremost step is to understand the length, breadth and the bandwidth of the data.
最重要的步骤是了解数据的长度,宽度和带宽。
print(df_you.shape)
print(df_you.nunique())

There seems to be around 40949 observations and 16 variables in the dataset. The next step would be to clean the data if necessary. I checked if there are any null values which need to be removed or manipulated.
数据集中似乎有大约40949个观测值和16个变量。 下一步将是在必要时清除数据。 我检查了是否有任何需要删除或处理的空值。
df_you.info()

We see that there are total 16 columns with no null values in any of them. Good for us :) Let us now get a sense of data by viewing the top few rows.
我们看到总共有16列,其中任何一列都没有空值。 对我们有用:)现在让我们通过查看前几行来获得数据感。
df_you.head(n=5)
Now comes the exciting part of visualizations! To visualize the data in the variables such as ‘likes’, ‘dislikes’, ‘views’ and ‘comment count’, I first normalize the data using log distribution. Normalization of the data is essential to ensure that these variables are scaled appropriately without letting one dominant variable skew the final result.
现在是可视化令人兴奋的部分! 为了可视化“喜欢”,“喜欢”,“观看”和“评论计数”等变量中的数据,我首先使用对数分布对数据进行规范化。 数据的规范化对于确保适当缩放这些变量而不会让一个主要变量偏向最终结果至关重要。
df_you['likes_log'] = np.log(df_you['likes']+1)
df_you['views_log'] = np.log(df_you['views'] +1)
df_you['dislikes_log'] = np.log(df_you['dislikes'] +1)
df_you['comment_count_log'] = np.log(df_you['comment_count']+1)
Let us now plot these!
现在让我们绘制这些!
plt.figure(figsize = (12,6))
plt.subplot(221)
g1 = sns.distplot(df_you['likes_log'], color = 'green')
g1.set_title("LIKES LOG DISTRIBUTION", fontsize = 16)
plt.subplot(222)
g2 = sns.distplot(df_you['views_log'])
g2.set_title("VIEWS LOG DISTRIBUTION", fontsize = 16)
plt.subplot(223)
g3 = sns.distplot(df_you['dislikes_log'], color = 'r')
g3.set_title("DISLIKES LOG DISTRIBUTION", fontsize=16)
plt.subplot(224)
g4 = sns.distplot(df_you['comment_count_log'])
g4.set_title("COMMENT COUNT LOG DISTRIBUTION", fontsize=16)
plt.subplots_adjust(wspace = 0.2, hspace = 0.4, top = 0.9)
plt.show()
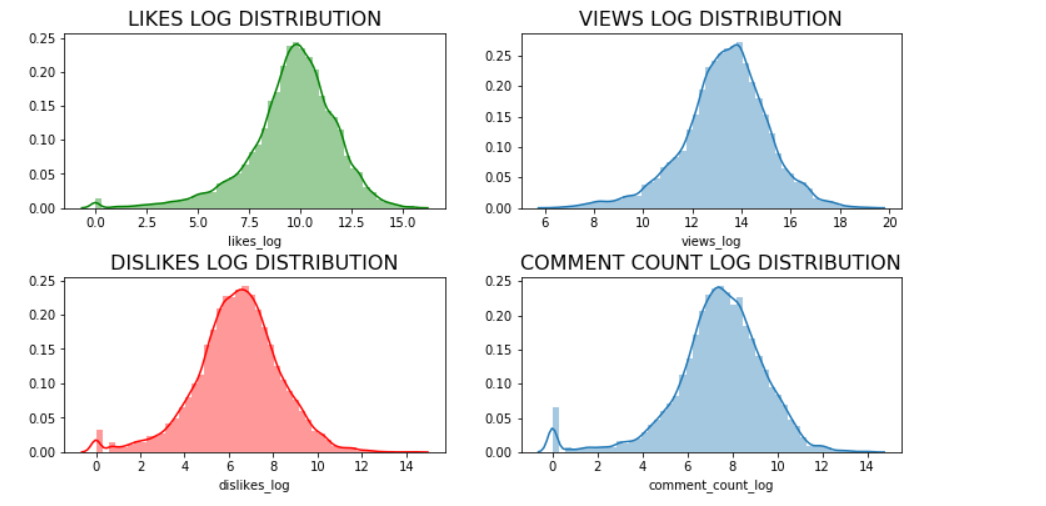
Let us now find out the unique category ids present in our dataset to assign appropriate category names in our dataframe.
现在让我们找出数据集中存在的唯一类别ID,以便在数据框中分配适当的类别名称。
np.unique(df_you["category_id"])

We see there are 16 unique categories. Let us assign the names to these categories using the information in the json file ‘US_category_id.json’ which we previously downloaded.
我们看到有16个独特的类别。 让我们使用先前下载的json文件“ US_category_id.json”中的信息将名称分配给这些类别。
df_you['category_name'] = np.nan
df_you.loc[(df_you["category_id"]== 1),"category_name"] = 'Film and Animation'
df_you.loc[(df_you["category_id"] == 2), "category_name"] = 'Cars and Vehicles'
df_you.loc[(df_you["category_id"] == 10), "category_name"] = 'Music'
df_you.loc[(df_you["category_id"] == 15), "category_name"] = 'Pet and Animals'
df_you.loc[(df_you["category_id"] == 17), "category_name"] = 'Sports'
df_you.loc[(df_you["category_id"] == 19), "category_name"] = 'Travel and Events'
df_you.loc[(df_you["category_id"] == 20), "category_name"] = 'Gaming'
df_you.loc[(df_you["category_id"] == 22), "category_name"] = 'People and Blogs'
df_you.loc[(df_you["category_id"] == 23), "category_name"] = 'Comedy'
df_you.loc[(df_you["category_id"] == 24), "category_name"] = 'Entertainment'
df_you.loc[(df_you["category_id"] == 25), "category_name"] = 'News and Politics'
df_you.loc[(df_you["category_id"] == 26), "category_name"] = 'How to and Style'
df_you.loc[(df_you["category_id"] == 27), "category_name"] = 'Education'
df_you.loc[(df_you["category_id"] == 28), "category_name"] = 'Science and Technology'
df_you.loc[(df_you["category_id"] == 29), "category_name"] = 'Non-profits and Activism'
df_you.loc[(df_you["category_id"] == 43), "category_name"] = 'Shows'
Let us now plot these to identify the popular video categories!
现在,让我们对它们进行标绘,以识别受欢迎的视频类别!
plt.figure(figsize = (14,10))
g = sns.countplot('category_name', data = df_you, palette="Set1", order = df_you['category_name'].value_counts().index)
g.set_xticklabels(g.get_xticklabels(),rotation=45, ha="right")
g.set_title("Count of the Video Categories", fontsize=15)
g.set_xlabel("", fontsize=12)
g.set_ylabel("Count", fontsize=12)
plt.subplots_adjust(wspace = 0.9, hspace = 0.9, top = 0.9)
plt.show()

We see that the top — 5 viewed categories are ‘Entertainment’, ‘Music’, ‘How to and Style’, ‘Comedy’ and ‘People and Blogs’. So if you are thinking of starting your own youtube channel, you better think about these categories first!
我们看到排名前5位的类别是“娱乐”,“音乐”,“操作方法和样式”,“喜剧”和“人与博客”。 因此,如果您想建立自己的YouTube频道,最好先考虑这些类别!
Let us now see how views, likes, dislikes and comments fare across categories using boxplots.
现在,让我们看看使用箱线图,视图,喜欢,不喜欢和评论在不同类别中的表现如何。
plt.figure(figsize = (14,10))
g = sns.boxplot(x = 'category_name', y = 'views_log', data = df_you, palette="winter_r")
g.set_xticklabels(g.get_xticklabels(),rotation=45, ha="right")
g.set_title("Views across different categories", fontsize=15)
g.set_xlabel("", fontsize=12)
g.set_ylabel("Views(log)", fontsize=12)
plt.subplots_adjust(wspace = 0.9, hspace = 0.9, top = 0.9)
plt.show()

plt.figure(figsize = (14,10))
g = sns.boxplot(x = 'category_name', y = 'likes_log', data = df_you, palette="spring_r")
g.set_xticklabels(g.get_xticklabels(),rotation=45, ha="right")
g.set_title("Likes across different categories", fontsize=15)
g.set_xlabel("", fontsize=12)
g.set_ylabel("Likes(log)", fontsize=12)
plt.subplots_adjust(wspace = 0.9, hspace = 0.9, top = 0.9)
plt.show()

plt.figure(figsize = (14,10))
g = sns.boxplot(x = 'category_name', y = 'dislikes_log', data = df_you, palette="summer_r")
g.set_xticklabels(g.get_xticklabels(),rotation=45, ha="right")
g.set_title("Dislikes across different categories", fontsize=15)
g.set_xlabel("", fontsize=12)
g.set_ylabel("Dislikes(log)", fontsize=12)
plt.subplots_adjust(wspace = 0.9, hspace = 0.9, top = 0.9)
plt.show()

plt.figure(figsize = (14,10))
g = sns.boxplot(x = 'category_name', y = 'comment_count_log', data = df_you, palette="plasma")
g.set_xticklabels(g.get_xticklabels(),rotation=45, ha="right")
g.set_title("Comments count across different categories", fontsize=15)
g.set_xlabel("", fontsize=12)
g.set_ylabel("Comment_count(log)", fontsize=12)
plt.subplots_adjust(wspace = 0.9, hspace = 0.9, top = 0.9)
plt.show()

Next I calculated engagement measures such as like rate, dislike rate and comment rate.
接下来,我计算了参与度,例如喜欢率,不喜欢率和评论率。
df_you['like_rate'] = df_you['likes']/df_you['views']
df_you['dislike_rate'] = df_you['dislikes']/df_you['views']
df_you['comment_rate'] = df_you['comment_count']/df_you['views']
Building correlation matrix using a heatmap for engagement measures.
使用热图来建立参与度度量的相关矩阵。
plt.figure(figsize = (10,8))
sns.heatmap(df_you[['like_rate', 'dislike_rate', 'comment_rate']].corr(), annot=True)
plt.show()

From the above heatmap, it can be seen that if a viewer likes a particular video, there is a 43% chance that he/she will comment on it as opposed to 28% chance of commenting if the viewer dislikes the video. This is a good insight which means if viewers like any videos, they are more likely to comment on them to show their appreciation/feedback.
从上面的热图可以看出,如果观众喜欢某个视频,则有43%的机会对其发表评论,而如果观众不喜欢该视频,则有28%的评论机会。 这是一个很好的见解,这意味着如果观众喜欢任何视频,他们就更有可能对它们发表评论以表示赞赏/反馈。
Next, I try to analyse the word count , unique word count, punctuation count and average length of the words in the ‘Title’ and ‘Tags’ columns
接下来,我尝试在“标题”和“标签”列中分析字数 , 唯一字数,标点符号和字的平均长度
#Word count
df_you['count_word']=df_you['title'].apply(lambda x: len(str(x).split()))
df_you['count_word_tags']=df_you['tags'].apply(lambda x: len(str(x).split()))#Unique word count
df_you['count_unique_word'] = df_you['title'].apply(lambda x: len(set(str(x).split())))
df_you['count_unique_word_tags'] = df_you['tags'].apply(lambda x: len(set(str(x).split())))#Punctutation count
df_you['count_punctuation'] = df_you['title'].apply(lambda x: len([c for c in str(x) if c in string.punctuation]))
df_you['count_punctuation_tags'] = df_you['tags'].apply(lambda x: len([c for c in str(x) if c in string.punctuation]))#Average length of the words
df_you['mean_word_len'] = df_you['title'].apply(lambda x : np.mean([len(x) for x in str(x).split()]))
df_you['mean_word_len_tags'] = df_you['tags'].apply(lambda x: np.mean([len(x) for x in str(x).split()]))
Plotting these…
绘制这些…
plt.figure(figsize = (12,18))
plt.subplot(421)
g1 = sns.distplot(df_you['count_word'],
hist = False, label = 'Text')
g1 = sns.distplot(df_you['count_word_tags'],
hist = False, label = 'Tags')
g1.set_title('Word count distribution', fontsize = 14)
g1.set(xlabel='Word Count')
plt.subplot(422)
g2 = sns.distplot(df_you['count_unique_word'],
hist = False, label = 'Text')
g2 = sns.distplot(df_you['count_unique_word_tags'],
hist = False, label = 'Tags')
g2.set_title('Unique word count distribution', fontsize = 14)
g2.set(xlabel='Unique Word Count')
plt.subplot(423)
g3 = sns.distplot(df_you['count_punctuation'],
hist = False, label = 'Text')
g3 = sns.distplot(df_you['count_punctuation_tags'],
hist = False, label = 'Tags')
g3.set_title('Punctuation count distribution', fontsize =14)
g3.set(xlabel='Punctuation Count')
plt.subplot(424)
g4 = sns.distplot(df_you['mean_word_len'],
hist = False, label = 'Text')
g4 = sns.distplot(df_you['mean_word_len_tags'],
hist = False, label = 'Tags')
g4.set_title('Average word length distribution', fontsize = 14)
g4.set(xlabel = 'Average Word Length')
plt.subplots_adjust(wspace = 0.2, hspace = 0.4, top = 0.9)
plt.legend()
plt.show()

Let us now visualize the word cloud for Title of the videos, Description of the videos and videos Tags. This way we can discover which words are popular in the title, description and tags. Creating a word cloud is a popular way to find out trending words on the blogsphere.
现在让我们可视化视频标题,视频描述和视频标签的词云。 通过这种方式,我们可以发现标题,描述和标签中流行的单词。 创建词云是在Blogsphere上查找流行词的一种流行方法。
Word Cloud for Title of the videos
视频标题的词云
plt.figure(figsize = (20,20))
stopwords = set(STOPWORDS)
wordcloud = WordCloud(
background_color = 'black',
stopwords=stopwords,
max_words = 1000,
max_font_size = 120,
random_state = 42
).generate(str(df_you['title']))#Plotting the word cloud
plt.imshow(wordcloud)
plt.title("WORD CLOUD for Titles", fontsize = 20)
plt.axis('off')
plt.show()
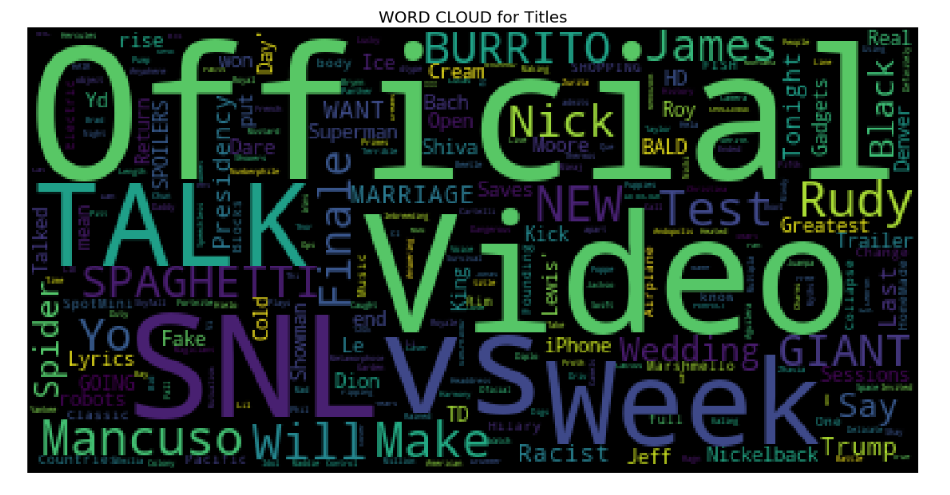
From the above word cloud, it is apparent that most popularly used title words are ‘Official’, ‘Video’, ‘Talk’, ‘SNL’, ‘VS’, and ‘Week’ among others.
从上面的词云中可以看出,最常用的标题词是“ Official”,“ Video”,“ Talk”,“ SNL”,“ VS”和“ Week”等。
2. Word cloud for Title Description
2.标题说明的词云
plt.figure(figsize = (20,20))
stopwords = set(STOPWORDS)
wordcloud = WordCloud(
background_color = 'black',
stopwords = stopwords,
max_words = 1000,
max_font_size = 120,
random_state = 42
).generate(str(df_you['description']))
plt.imshow(wordcloud)
plt.title('WORD CLOUD for Title Description', fontsize = 20)
plt.axis('off')
plt.show()

I found that the most popular words for description of videos are ‘https’, ‘video’, ‘new’, ‘watch’ among others.
我发现最受欢迎的视频描述词是“ https”,“ video”,“ new”,“ watch”等。
3. Word Cloud for Tags
3.标签的词云
plt.figure(figsize = (20,20))
stopwords = set(STOPWORDS)
wordcloud = WordCloud(
background_color = 'black',
stopwords = stopwords,
max_words = 1000,
max_font_size = 120,
random_state = 42
).generate(str(df_you['tags']))
plt.imshow(wordcloud)
plt.title('WORD CLOUD for Tags', fontsize = 20)
plt.axis('off')
plt.show()

Popular tags seem to be ‘SNL’, ‘TED’, ‘new’, ‘Season’, ‘week’, ‘Cream’, ‘youtube’ From the word cloud analysis, it looks like there are a lot of Saturday Night Live fans on the youtube out there!
热门标签似乎是'SNL','TED','new','Season','week','Cream','youtube'。从词云分析来看,似乎有很多Saturday Night Live粉丝在YouTube上!
I used the word cloud library for the very first time and it yielded pretty and useful visuals! If you are interested in knowing more about this library and how to use it then you must absolutely check this out
我第一次使用词云库,它产生了漂亮而有用的视觉效果! 如果您有兴趣了解有关此库以及如何使用它的更多信息,则必须完全检查一下
This analysis is hosted on my Github page here.
此分析托管在我的Github页面上。
Thanks for reading!
谢谢阅读!
翻译自: https://towardsdatascience.com/visualizing-youtube-videos-using-seaborn-and-wordcloud-in-python-b24247f70228
本文来自互联网用户投稿,该文观点仅代表作者本人,不代表本站立场。本站仅提供信息存储空间服务,不拥有所有权,不承担相关法律责任。如若转载,请注明出处:http://www.mzph.cn/news/390930.shtml
如若内容造成侵权/违法违规/事实不符,请联系多彩编程网进行投诉反馈email:809451989@qq.com,一经查实,立即删除!