为什么选择做班级管理系统
Accuracy is a go-to metric because it’s highly interpretable and low-cost to evaluate. For this reason, accuracy — perhaps the most simple of machine learning metrics — is (rightfully) commonplace. However, it’s also true that many people are too comfortable with accuracy.
准确性是首选指标,因为它具有很高的解释性和低成本。 因此,准确性(也许是机器学习指标中最简单的一种)(理所应当)是司空见惯的。 然而,这也是事实,很多人都太舒服的准确性。
Being aware of the limitations of accuracy is essential.
意识到准确性的局限性是至关重要的。
Everyone knows that accuracy is misused on unbalanced datasets: for instance, in a medical condition dataset, where the majority of people do not have condition x (let’s say 95%) and the remainder do have condition x.
每个人都知道在不平衡的数据集上滥用了准确性:例如,在医疗状况数据集中,大多数人没有状况x (比如说95%),其余人确实有状况x 。
Since machine learning models are always looking for the easy way out, and especially if an L2 penalization is used (a proportionately less penalty on lower errors), the model can comfortably get away with 95% accuracy only guessing all inputs do not have condition x.
由于机器学习模型一直在寻找简便的方法,特别是如果使用L2惩罚(对较低的错误按比例减少的惩罚),则仅凭猜测所有输入都没有条件x即可轻松获得95%的精度的模型。
The reply to this common issue is to use some sort of metric that takes into account the unbalanced classes and somehow compensates lack of quantity with a boost of importance, like an F1 score or balanced accuracy.
解决此常见问题的方法是使用某种度量标准,该度量标准考虑了不平衡的类别,并以某种重要的方式补偿了数量不足的情况,例如F1得分或平衡准确性。
Beyond this common critique, however — which doesn’t address other limitations of accuracy — there are some other problems with using accuracy that go beyond just dealing with balanced classes.
但是,除了这种常见的批评之外(没有解决准确性的其他限制),使用准确性还有其他一些问题,这些问题不仅仅涉及平衡类。
Everyone agrees that training/testing and deployment of a model should be kept separate. More specifically, the former should be statistical, and the latter should be decision-based. However, there is nothing statistical about turning the outputs of machine learning models — which are (almost) always probabilistic — into decisions, and evaluating its statistical goodness based on this converted output.
每个人都同意,应分开进行模型的培训/测试和部署。 更具体地说,前者应该是统计的,而后者应该是基于决策的。 但是,没有关于将机器学习模型的输出(几乎总是概率)转化为决策,并基于转换后的输出评估其统计优势的统计信息。
Take a look at the outputs for two machine learning models: should they really be getting the same results? Moreover, even if one tries to remedy accuracy with other decision-based metrics like the commonly prescribed specificity/sensitivity or F1 score, the same problem exists.
看一下两个机器学习模型的输出:它们是否真的会得到相同的结果? 此外,即使人们试图用其他基于决策的指标(如通常规定的特异性/敏感性或F1分数)来纠正准确性,也存在相同的问题。
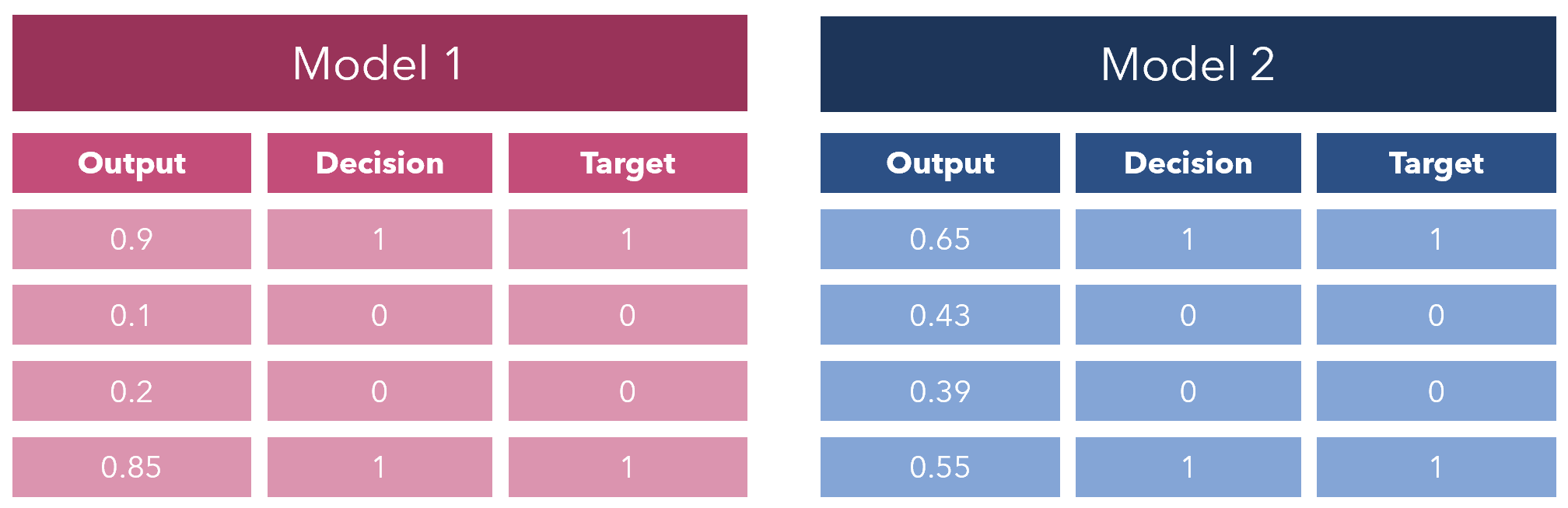
Model 2 is far less confident in its results than Model 1 is, but both receive the same accuracy. Accuracy is not a legitimate scoring rule, and hence it is deceiving in an inherently probabilistic environment.
模型2对结果的信心远不如模型1可靠,但两者的准确性相同。 准确性不是一个合理的评分规则,因此它在固有的概率环境中具有欺骗性。
While it can be used in the final presenting of a model, it leaves an empty void of information pertaining to the confidence of the model; whether it actually knew the class for most of the training samples or if it was only lucky in crossing on the right side of the 0.5 threshold.
虽然可以在模型的最终展示中使用它,但它留下了与模型的置信度有关的信息的空白; 无论是实际上对大多数训练样本都知道这门课,还是只是幸运地越过了0.5个阈值的右侧。
This is also problematic. How can a reliable loss function — the guiding light that shows the model what is right and what is wrong — completely tilt its decision 180 degrees if the output probability shifts 0.01%? If a training sample with label ‘1’ received predictions 0.51 and 0.49 from model 1 and model 2, respectively, is it fair that model 2 is penalized at the full possible value? Thresholds, while necessary for decision-making in a physically deterministic world, are too sensitive and hence inappropriate for training and testing.
这也是有问题的。 如果输出概率偏移0.01%,可靠的损失函数(显示模型正确与错误的指示灯)如何将其决策完全倾斜180度? 如果带有标签“ 1”的训练样本分别从模型1和模型2接收到预测0.51和0.49,那么将模型2惩罚为可能的全部值是否公平? 阈值虽然在物理确定性世界中进行决策是必需的,但过于敏感,因此不适用于培训和测试。
Speaking of thresholds — consider this. You are creating a machine learning model to decide if a patient should receive a very invasive and painful surgery treatment. Where do you decide the threshold to give the recommendation? Instinctively, most likely not at a default 0.5, but at some higher probability: the patient is subjected to this treatment if, and only if, the model is absolutely sure. On the other hand, if the treatment is something less serious like an aspirin, it is less so.
说到阈值,请考虑一下。 您正在创建一个机器学习模型,以决定患者是否应该接受侵入性和痛苦性极高的手术治疗。 您在哪里确定提出建议的门槛? 本能地,最有可能不是默认值0.5,而是更高的概率:当且仅当模型是绝对确定的,患者才接受这种治疗。 另一方面,如果像阿斯匹林这样的不那么严重的治疗方法,那么情况就不那么严重了。
The results of the decision dictate the thresholds for forming it. This idea, hard-coding morality and human feeling into a machine learning model, is difficult to think about. One may be inclined to argue that over time and under the right balanced circumstances, the model will automatically shift its output probability distributions to a 0.5 threshold and manually adding a threshold is tampering with the model’s learning.
决策的结果决定了形成决策的阈值。 将道德和人类感觉硬编码到机器学习模型中的想法很难考虑。 有人可能会争辩说,随着时间的流逝,在正确的平衡情况下,该模型将自动将其输出概率分布更改为0.5阈值,而手动添加阈值会篡改该模型的学习。
The rebuttal would be to not use decision-based scoring functions in the first place, not hard-coding any number, including a 0.5 threshold, at all. This way, the model learns not to cheat and take the easy way out through artificially constructed continuous-to-discrete conversions but to maximize its probability of correct answers.
反对是首先不使用基于决策的评分功能,根本不硬编码任何数字,包括0.5阈值。 这样,该模型就不会通过人工构造的连续到离散转换来欺骗并采取简单的方法,而是最大限度地提高其正确答案的可能性。
Whenever a threshold is introduced in the naturally probabilistic and fluid nature of machine learning algorithms, it causes more problems than it fixes.
每当在机器学习算法的自然概率和流动性中引入阈值时,它都会引起更多的问题,而不是要解决的问题。
Loss functions that treat probability on the continuous scale it is instead of as discrete buckets are the way to go.
损失函数在连续尺度上处理概率,而不是像离散的桶那样走。
What are some better, probability-based, and more informative metrics to use for honestly evaluating a model’s performance?
有什么更好的,基于概率的,更多信息的指标可用于诚实地评估模型的性能?
- Brier score 刺分数
- Log score 日志分数
- Cross-entropy 交叉熵
In the end, accuracy is an important and permanent part of the metrics family. But for those who decide to use it: understand that accuracy’s interpretability and simplicity comes at a heavy cost.
最后,准确性是指标系列的重要且永久的组成部分。 但是对于那些决定使用它的人:请理解准确性的可解释性和简单性要付出沉重的代价。
翻译自: https://medium.com/analytics-vidhya/why-accuracy-is-troublesome-even-with-balanced-classes-590b405f5a06
为什么选择做班级管理系统
本文来自互联网用户投稿,该文观点仅代表作者本人,不代表本站立场。本站仅提供信息存储空间服务,不拥有所有权,不承担相关法律责任。如若转载,请注明出处:http://www.mzph.cn/news/390028.shtml
如若内容造成侵权/违法违规/事实不符,请联系多彩编程网进行投诉反馈email:809451989@qq.com,一经查实,立即删除!