先知模型 facebook
Time series prediction is one of the must-know techniques for any data scientist. Questions like predicting the weather, product sales, customer visit in the shopping center, or amount of inventory to maintain, etc - all about time series forecasting, making it a valuable addition to a data scientist’s skillsets.
时间序列预测是任何数据科学家都必须了解的技术之一。 诸如预测天气,产品销售,购物中心的顾客来访或要维护的库存量之类的问题都与时间序列预测有关,这使其成为数据科学家技能的宝贵补充。
In this article, I will introduce how to use Facebook Prophet to predict the crime rate in Chicago. Split into 5 parts:
在本文中,我将介绍如何使用Facebook Prophet预测芝加哥的犯罪率。 分为5部分:
1. Prophet Introduction
1.先知介绍
2. EDA
2. EDA
3. Data processing
3.数据处理
4. Model prediction
4.模型预测
5. Takeaways
5.外卖
Let’s begin the journey.
让我们开始旅程。
1. Prophet Introduction
1.先知介绍
In 2017, Facebook Core Data Science Team open-sourced Prophet. As stated on its Github page, Prophet is:
2017年,Facebook核心数据科学团队开源了Prophet。 如其Github页所述,先知是:
- a procedure for forecasting time series data; 预测时间序列数据的程序;
- based on additive models; 基于加性模型;
- fit non-linear trends with yearly, weekly, and daily seasonality, plus holiday effect. 使非线性趋势与每年,每周和每天的季节性相适应,再加上假期影响。
Prophet uses a decomposable model with three main components, including trend, seasonality, and holidays, as combined below:
先知使用具有三个主要组成部分的可分解模型,包括趋势,季节性和假日,如下所示:

Where:
哪里:
g(t) is the trend function which models non-periodic changes;
g(t)是模拟非周期性变化的趋势函数;
s(t) represents periodic changes (e.g., weekly and yearly seasonality);
s(t)代表周期性变化(例如,每周和每年的季节性变化);
h(t) represents the effects of holidays which occur on potentially irregular schedules;
h(t)表示假期可能在不定期的时间表上发生的影响;
- the error term represents any idiosyncratic changes which are not accommodated by the model. 错误项表示模型不适应的任何特有变化。
So using time as a regressor, Prophet tries to fit linear and non-linear functions of time as components. In effect, Prophet frames the forecasting problem as a curve-fitting exercise, instead of looking at the time-based dependency of each observation, which brings flexibility, fast-fitting, and interpretable parameters.
因此,先知将时间用作回归变量,尝试将时间的线性和非线性函数拟合为分量。 实际上,Prophet将预测问题构造为曲线拟合练习,而不是查看每个观测值基于时间的依赖性,这带来了灵活性,快速拟合和可解释的参数。
Prophet works best with time series that have strong seasonal effects and several seasons of historical data.
先知最适合具有强烈季节性影响和多个季节历史数据的时间序列。
2. EDA
2. EDA
The data used here is the Chicago Crime dataset from Kaggle. It contains a summary of the reported crimes that occurred in the City of Chicago from 2001 to 2017.
这里使用的数据是来自Kaggle的Chicago Crime数据集。 它包含2001年至2017年在芝加哥市发生的所报告犯罪的摘要。
Quickly looking at the data below, you will notice the dataset has 23 columns and 7,941,282 records, including ID, Case Number, Block, Primary Type, Description, etc.
快速查看下面的数据,您会注意到数据集有23列和7,941,282条记录,包括ID,案例编号,块,主要类型,描述等。
First, let’s drop the unused columns. Specifically,
首先,让我们删除未使用的列。 特别,
df.drop([‘Unnamed: 0’, ‘ID’, ‘Case Number’, ‘IUCR’, ‘X Coordinate’, ‘Y Coordinate’,’Updated On’,’Year’, ‘FBI Code’, ‘Beat’,’Ward’,’Community Area’,‘Location’, ‘District’, ‘Latitude’, ‘Longitude’],
axis = 1, inplace=True)
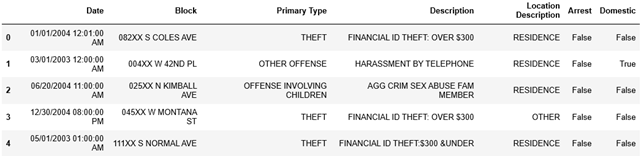
As shown in Fig.1, the column ‘Date’ is in date format. Let’s convert it to a date format Pandas can interpret, and set it as the index. Specifically,
如图1所示, “日期”列为日期格式。 让我们将其转换为熊猫可以解释的日期格式,并将其设置为索引。 特别,
df.Date = pd.to_datetime(df.Date, format = ‘%m/%d/%Y %I:%M:%S %p’)
df.index = pd.DatetimeIndex(df.Date)
df.drop(‘Date’, inplace = True, axis = 1)
Now data is ready for visualization. First, let’s look at the yearly crime distribution. Specifically,
现在,数据已准备好可视化。 首先,让我们看一下每年的犯罪分布。 特别,
plt.plot(df.resample(‘Y’).size())
plt.xlabel(‘Year’)
plt.ylabel(‘Num of crimes’)
Note above df.resample(‘Y’).size() produce the yearly crime count.
请注意,上面的df.resample('Y')。size()会产生年度犯罪计数。
As indicated in Fig.2, the crime rate starts to drop from 2002 to 2005. But from 2006, the crime rate starts to go up, reaching a peak in 2009 and going down till 2018. This curve may reflect the economic impact on social crime. Before and after the financial crisis, the crime rate goes downs yearly, but the bad economy resulting from the financial crisis causes an increase in crimes.
如图2所示,犯罪率从2002年到2005年开始下降。但是从2006年开始,犯罪率开始上升,2009年达到峰值,然后下降到2018年。该曲线可能反映了经济对社会的影响。犯罪。 金融危机前后,犯罪率逐年下降,但金融危机造成的经济不景气导致犯罪率上升。
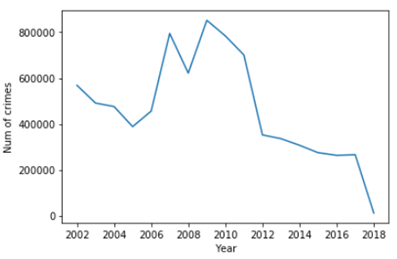
Second, let’s look at the quarterly crime rate distribution. As shown in Fig.3, the crime rate shows a descending trend with periodic ups and downs.
其次,让我们看一下季度犯罪率分布。 如图3所示,犯罪率呈下降趋势,并有周期性的起伏。
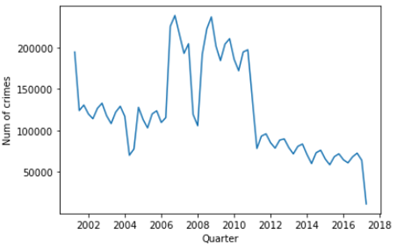
In a similar way, as shown in Fig.4, the monthly crime rate shows the same pattern as the quarterly analysis.
以类似的方式,如图4所示,每月犯罪率显示与季度分析相同的模式。
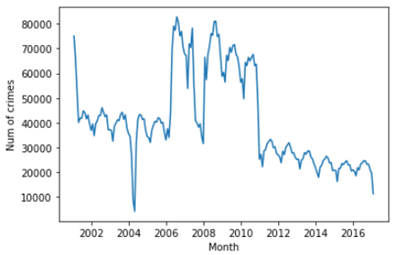
3. Data processing
3.数据处理
The input to Prophet is always a dataframe with two columns: ‘ds’ and ‘y’. The ‘ds’ (datestamp) column should be of a format expected by Pandas, ideally YYYY-MM-DD for a date or YYYY-MM-DD HH:MM:SS for a timestamp. The ‘y’ column must be numeric and represents the measurement we wish to forecast.
先知的输入始终是具有两列的数据框:“ ds”和“ y”。 “ ds”(datestamp)列应采用熊猫期望的格式,理想情况下,日期应为YYYY-MM-DD,时间戳则应为YYYY-MM-DD HH:MM:SS。 “ y”列必须为数字,代表我们希望预测的度量。
Specifically,
特别,
df_m = df.resample(‘M’).size().reset_index()
df_m.columns = [‘Date’, ‘Monthly Crime Count’]
df_m_final = df_m.rename(columns = {‘Date’: ‘ds’, ‘Monthly Crime Count’: ‘y’})
4. Model prediction
4.模型预测
From EDA analysis, we found there is monthly and quarterly seasonality but no yearly seasonality. By default, Prophet fits weekly and yearly seasonality, if the time series is more than two cycles long. Users can add seasonality such as hourly, monthly, and quarterly using ‘add_seasonality’ method.
通过EDA分析,我们发现每个月和每个季度都有季节性,但没有年度季节性。 默认情况下,如果时间序列长于两个周期以上,则先知适合每周和每年的季节性。 用户可以使用“ add_seasonality”方法添加每小时,每月和每季度等季节性信息。
To make a prediction, instantiate a new Prophet object, and call the fit method to train on the data. Specifically,
要进行预测,请实例化一个新的Prophet对象,然后调用fit方法对数据进行训练。 特别,
m = Prophet(interval_width=0.95, yearly_seasonality=False)
m.add_seasonality(name=’monthly’, period=30.5, fourier_order=10)
m.add_seasonality(name=’quarterly’, period=91.5, fourier_order=10)
m.fit(df_m_final)
Note ‘interval_width=0.95’, produces a confidence interval around the forecast. Prophet uses a partial Fourier sum to approximate periodic signal. The number of Fourier order determines how quickly the seasonality can change.
注意'interval_width = 0.95' ,在预测周围产生一个置信区间。 先知使用部分傅立叶和来近似周期信号。 傅立叶阶数确定季节性可以多快地改变。
Predictions are made on a dataframe with a column ‘ds’ containing the dates for which a prediction is to be made. For instance, to predict the following 24 months, try below:
在具有“ ds”列的数据帧上进行预测,该列包含要进行预测的日期。 例如,要预测接下来的24个月,请尝试以下操作:
future = m.make_future_dataframe(periods = 24, freq = ‘M’)
pred = m.predict(future)
As shown in Fig.5, the predicted value ‘yhat’ is assigned to each date with a lower and upper limit.
如图5所示,将预测值“ yhat”分配给具有上限和下限的每个日期。
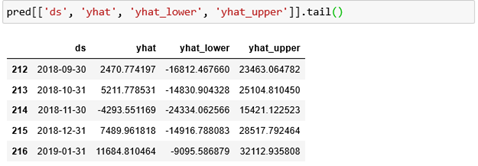
As shown in Fig.6, the black dots are the historical data, and the deep blue line is model predictions. The light blue shadow is a 95% confidence interval around the predictions. The blue line shows a good match with the pattern in Fig.3, indicating a good prediction on historical data. Great!
如图6所示,黑点是历史数据,深蓝线是模型预测。 淡蓝色阴影是围绕预测的95%置信区间。 蓝线表示与图3中的图案非常匹配,表示对历史数据的良好预测。 大!
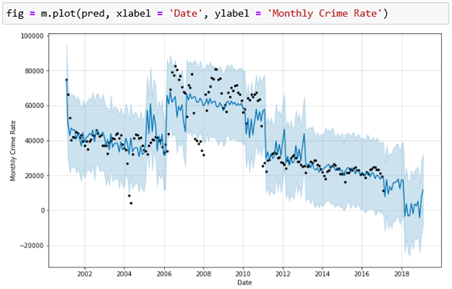
Finally, Fig.7 shows the un-periodic trend, and monthly and quarterly seasonality components of the crime rate pattern.
最后,图7显示了犯罪率模式的非周期性趋势以及每月和每季度的季节性组成。
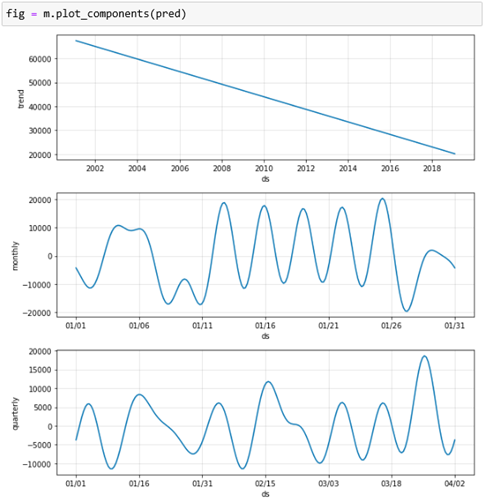
5. Takeaways
5.外卖
We introduced how to make the best use of Facebook Prophet. Specifically,
我们介绍了如何充分利用Facebook Prophet。 特别,
- to use EDA to explore the historical data patterns, helping to create the best suitable model 使用EDA探索历史数据模式,帮助创建最合适的模型
- to use data processing to prepare the data for modeling 使用数据处理为建模准备数据
- to use Prophet to fit the historical data and forecast future crime rate 使用先知来拟合历史数据并预测未来犯罪率
Great! Huge congratulations for making it to the end. If you need the source code, feel free to visit my Github page.
大! 巨大的祝贺,使它走到了尽头。 如果您需要源代码,请随时访问我的Github页面。
1. Facebook Prophet official document
1. Facebook Prophet官方文件
2. Prophet paper: Sean J. Taylor, Benjamin Letham (2018) Forecasting at scale. The American Statistician 72(1):37–45 (https://peerj.com/preprints/3190.pdf).
2.先知论文:肖恩·泰勒(Sean J. Taylor),本杰明·莱瑟姆(Benjamin Letham)(2018)大规模预测。 美国统计师72(1):37-45( https://peerj.com/preprints/3190.pdf )。
翻译自: https://towardsdatascience.com/crime-rate-prediction-using-facebook-prophet-5348e21273d
先知模型 facebook
本文来自互联网用户投稿,该文观点仅代表作者本人,不代表本站立场。本站仅提供信息存储空间服务,不拥有所有权,不承担相关法律责任。如若转载,请注明出处:http://www.mzph.cn/news/389440.shtml
如若内容造成侵权/违法违规/事实不符,请联系多彩编程网进行投诉反馈email:809451989@qq.com,一经查实,立即删除!