时间序列因果关系
When examining a time series, it is quite common to have an intervention influence that series at a particular point.
在检查时间序列时,在特定时间点对该序列产生干预影响是很常见的。
Some examples of this could be:
例如:
- An advertising campaign that results in a significant change in sales 导致销售发生重大变化的广告活动
- A positive change in traffic policy that results in lower road deaths 交通政策的积极变化,可减少道路死亡人数
- A change in economic policy that affects asset prices 影响资产价格的经济政策变化
The problem with analysing the effects of interventions is that one is then unable to examine how that series would have trended without that intervention.
分析干预措施效果的问题在于,如果没有该干预措施,人们将无法检查该系列趋势。
For instance, suppose that a company implements an advertising campaign with the intent of boosting sales. While sales data can be recorded post-intervention — it is not possible to say what the sales would have been without that intervention.
例如,假设一家公司实施了旨在促进销售的广告活动。 虽然销售数据可以记录后干预-这是不可能说什么销售本来没有这种干预。
我们的例子 (Our Example)
For this example, let us consider the impact of an interest rate change on currency fluctuations.
对于此示例,让我们考虑利率变化对货币波动的影响 。
When a central bank manipulates interest rates — this is hypothesised to have a significant impact on a currency as it affects the perceived value of that currency relative to others in the market.
当中央银行操纵利率时,假设这会对货币产生重大影响,因为它会影响该货币相对于市场上其他货币的感知价值。
However, what if we wish to quantify such an impact? Let’s see how this works with the causalimpact package. This package was originally developed by Google for R, but we will use the Python version (pycasualimpact) in this example.
但是,如果我们希望量化这种影响怎么办? 让我们看看它如何与causalimpact包一起工作。 该软件包最初是由Google for R开发的,但是在此示例中,我们将使用Python版本(pycasualimpact)。
Let’s consider fluctuations in the GBP/USD for the year 2017.
让我们考虑一下2017年英镑/美元的波动。
In November 2017, the Bank of England decided to raise interest rates.
2017年11月,英格兰银行决定提高利率。
We will use causalimpact in an attempt to investigate:
我们将使用因果影响来调查:
- what the forecasted GBP/USD rate would have been in the absence of such an intervention 如果不采取这种干预措施,英镑/美元的汇率将是多少?
- whether such an intervention is significant and has affected the currency level 此类干预是否重大并已影响货币水平
In order to determine whether such an intervention has a significant effect on a time series — we must use a suitable covariate (X). This covariate must not be affected by the intervention.
为了确定此类干预是否会对时间序列产生重大影响,我们必须使用适当的协变量(X)。 此协变量不得受到干预的影响。
For instance, we can see that for the year 2017, the European Central Bank left interest rates unchanged (and have done since):
例如,我们可以看到,欧洲中央银行在2017年维持利率不变(此后一直这样做):
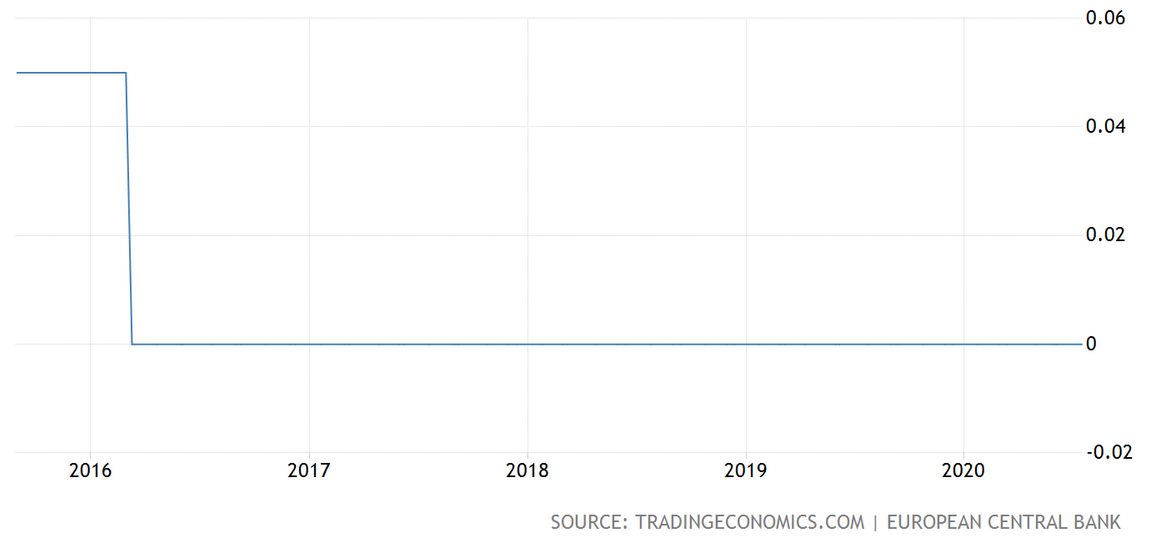
Taking this into account, the EUR/USD will be used as the covariate for this example.
考虑到这一点,本示例将使用EUR / USD作为协变量。
X = eurusd
y = gbpusd
While it is hypothesised that the currency will be affected by fluctuations in the greenback as well as many other macroeconomic conditions — it is assumed that the Bank of England intervention does not impact the EUR/USD.
假设该货币将受到美元波动以及许多其他宏观经济状况的影响-假设英格兰银行的干预不会影响欧元/美元。
分析 (Analysis)
The relevant currency pairs are downloaded from the FRED database using Quandl:
使用Quandl从FRED数据库下载相关货币对:
>>> eurusd = quandl.get("FRED/DEXUSEU", start_date='2017-01-01', end_date='2018-01-01', api_key='enter_api_key')>>> gbpusd = quandl.get("FRED/DEXUSUK", start_date='2017-01-01', end_date='2018-01-01', api_key='enter_api_key')
gbpusd
Here is a plot of the two currency pairs:
这是两个货币对的图表:
英镑/美元 (GBP/USD)
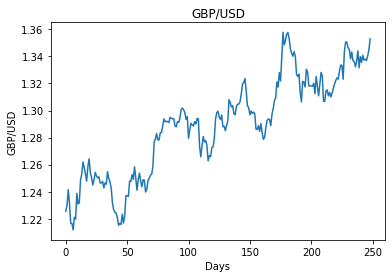
欧元/美元 (EUR/USD)
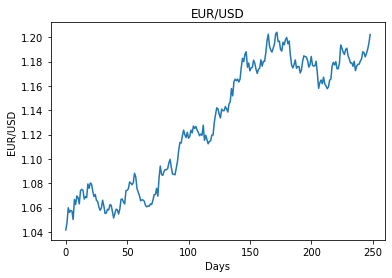
With the rate decision by the Bank of England having been taken on 2nd November 2017, t = 0 to 229 is defined as the pre-period (before intervention) and t = 230 to 248 is defined as the post-period (after intervention).
由于英格兰银行已于2017年11月2日做出利率决定,因此将t = 0到229定义为前期(干预之前),将t = 230到248定义为后期(干预之后) 。
data = pd.DataFrame({'y': y, 'X': X}, columns=['y', 'X'])
pre_period = [0, 229]
post_period = [230, 248]
According to the official GitHub for this package, this model “uses a classical Kalman Filter approach for solving the statespace equations”.
根据该软件包的官方GitHub的说法,该模型“使用经典的Kalman滤波方法来求解状态空间方程”。
The posterior inference can be generated as follows:
可以如下生成后验推断:
>>> ci = CausalImpact(data, pre_period, post_period)
>>> print(ci.summary())
>>> print(ci.summary(output='report'))
>>> ci.plot()
Here is the generated output:
这是生成的输出:
Posterior Inference {Causal Impact}
Average Cumulative
Actual 1.34 25.46
Prediction (s.d.) 1.32 (0.0) 25.17 (0.08)
95% CI [1.32, 1.33] [25.01, 25.33]
Absolute effect (s.d.) 0.02 (0.0) 0.29 (0.08)
95% CI [0.01, 0.02] [0.13, 0.45]
Relative effect (s.d.) 1.15% (0.32%) 1.15% (0.32%)
95% CI [0.52%, 1.77%] [0.52%, 1.77%]
Posterior tail-area probability p: 0.0
Posterior prob. of a causal effect: 100.0%
According to the above findings, the actual GBP/USD rate comes in at 1.34, while the rate without the intervention would have been 1.32.
根据上述发现,英镑/美元的实际汇率为1.34 ,而没有干预的汇率将为1.32 。
Moreover, the model indicates that there is a 100% posterior probability of a causal effect.
此外,该模型表明因果关系的后验概率为100%。
When analysing the more detailed report, the output also states:
在分析更详细的报告时,输出还指出:
The probability of obtaining this effect by chance is very small(Bayesian one-sided tail-area probability p = 0.0).This means the causal effect can be considered statisticallysignificant.
偶然获得此效应的可能性很小(贝叶斯单侧尾部区域概率p = 0.0)。这意味着因果效应在统计上是有意义的。
From this standpoint, the evidence suggests that the GBP/USD trended higher than it would have done without an interest rate rise. Here is an overview of the actual rate vs predictions:
从这个角度来看,有证据表明,英镑/美元的趋势要高于没有加息的情况。 以下是实际费率与预测的概述:
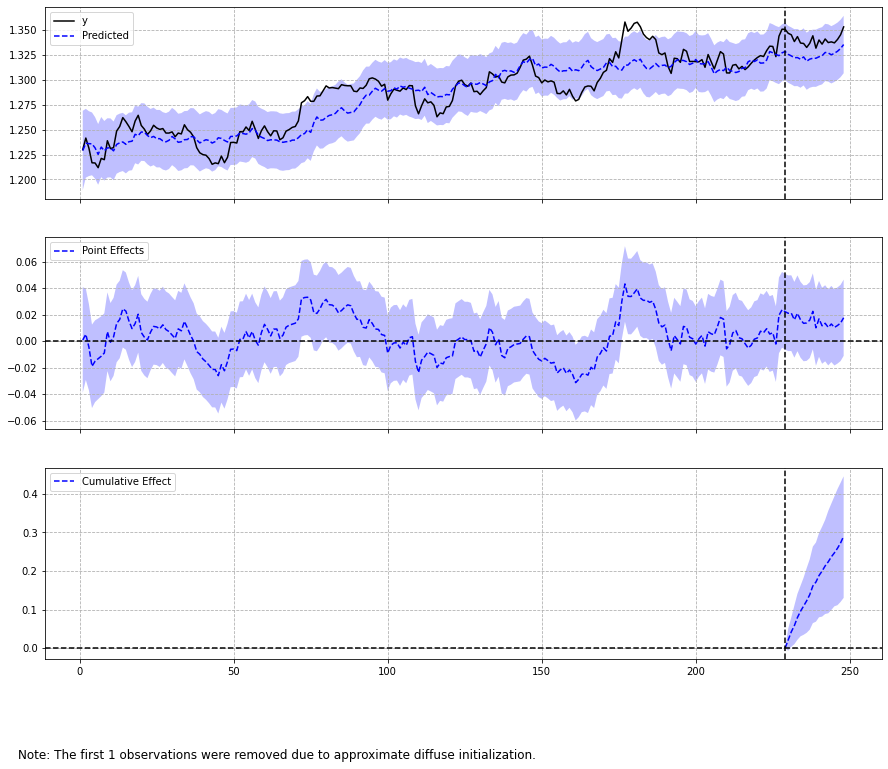
As mentioned, Python uses a different approach than R in generating forecasts without an intervention — the latter relying more on a Bayesian approach that emphasises user prior knowledge. Indeed, one could try both methods to verify if the forecasts being generated coincide.
如前所述,Python使用R的方法与R不同,无需干预即可生成预测-后者更多地依赖于强调用户先验知识的贝叶斯方法。 确实,可以尝试两种方法来验证所生成的预测是否一致。
结论 (Conclusion)
This has been an introductory example to the causalimpact library and how the effects of interventions can be examined across a time series.
这是因果影响库的介绍性示例,以及如何在整个时间序列中检查干预措施的影响。
Many thanks for your time, and any questions or feedback are greatly welcomed.
非常感谢您的宝贵时间,我们欢迎您提出任何问题或反馈。
The Jupyter Notebook for this example is available at the MGCodesandStats repository as referenced below.
MGCodesandStats存储库中提供了此示例的Jupyter Notebook,如下所述。
Disclaimer: This article is written on an “as is” basis and without warranty. It was written with the intention of providing an overview of data science concepts, and should not be interpreted as investment advice, or any other sort of professional advice.
免责声明:本文按“原样”撰写,不作任何担保。 本文档旨在概述数据科学概念,不应将其解释为投资建议或任何其他形式的专业建议。
翻译自: https://towardsdatascience.com/analysing-time-series-interventions-with-causalimpact-currency-fluctuations-e6ab14a30768
时间序列因果关系
本文来自互联网用户投稿,该文观点仅代表作者本人,不代表本站立场。本站仅提供信息存储空间服务,不拥有所有权,不承担相关法律责任。如若转载,请注明出处:http://www.mzph.cn/news/389315.shtml
如若内容造成侵权/违法违规/事实不符,请联系多彩编程网进行投诉反馈email:809451989@qq.com,一经查实,立即删除!