字符串操作截取后面的字符串
We have to represent every bit of data in numerical values to be processed and analyzed by machine learning and deep learning models. However, strings do not usually come in a nice and clean format and require preprocessing to convert to numerical values. Pandas offers many versatile functions to modify and process string data efficiently.
我们必须以数值表示数据的每一位,以便通过机器学习和深度学习模型进行处理和分析。 但是,字符串通常不会采用简洁的格式,需要进行预处理才能转换为数值。 熊猫提供了许多通用功能,可以有效地修改和处理字符串数据。
In this post, we will discover how Pandas can manipulate strings. I grouped string functions and methods under 5 categories:
在本文中,我们将发现Pandas如何操纵字符串。 我将字符串函数和方法分为5类:
Splitting
分裂
Stripping
剥离
Replacing
更换
Filtering
筛选
Combining
结合
Let’s first create a sample dataframe to work on for examples.
让我们首先创建一个示例数据框以进行示例。
import numpy as np
import pandas as pdsample = {
'col_a':['Houston,TX', 'Dallas,TX', 'Chicago,IL', 'Phoenix,AZ', 'San Diego,CA'],
'col_b':['$64K-$72K', '$62K-$70K', '$69K-$76K', '$62K-$72K', '$71K-$78K' ],
'col_c':['A','B','A','a','c'],
'col_d':[' 1x', ' 1y', '2x ', '1x', '1y ']
}df_sample = pd.DataFrame(sample)
df_sample
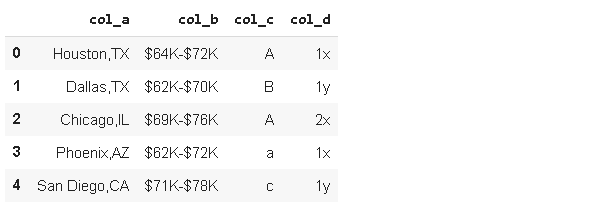
1.分裂 (1. Splitting)
Sometimes strings carry more than one piece of information and we may need to use them separately. For instance, “col_a” contains both city and state. The split function of pandas is a highly flexible function to split strings.
有时字符串包含不止一条信息,我们可能需要单独使用它们。 例如,“ col_a”包含城市和州。 pandas的split函数是用于拆分字符串的高度灵活的函数。
df_sample['col_a'].str.split(',')0 [Houston, TX]
1 [Dallas, TX]
2 [Chicago, IL]
3 [Phoenix, AZ]
4 [San Diego, CA]
Name: col_a, dtype: object
Now each element is converted to a list based on the character used for splitting. We can easily export individual elements from those lists. Let’s create a “state” column.
现在,每个元素都会根据用于拆分的字符转换为列表。 我们可以轻松地从这些列表中导出单个元素。 让我们创建一个“状态”列。
df_sample['state'] = df_sample['col_a'].str.split(',').str[1]df_sample
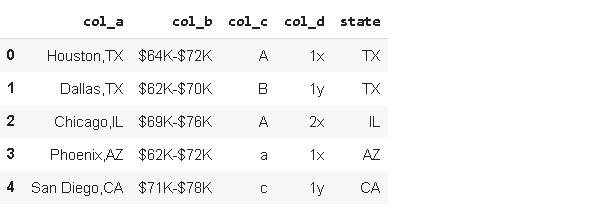
Warning: Subscript ([1]) must be applied with str keyword. Otherwise, we will get the list in the specified row.
警告 :下标([1])必须与str关键字一起应用。 否则,我们将在指定的行中获取列表。
df_sample['col_a'].str.split(',')[1]
['Dallas', 'TX']
The splitting can be done on any character or letter.
可以对任何字符或字母进行拆分。
The split function returns a dataframe if expand parameter is set as True.
如果将expand参数设置为True,则split函数将返回一个数据帧。
df_sample['col_a'].str.split('a', expand=True)
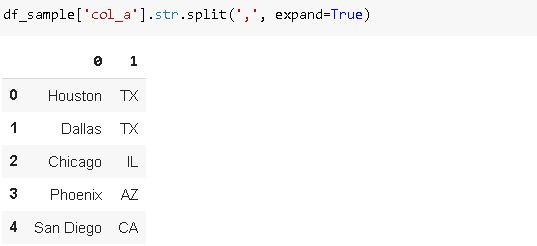
拆分vs rsplit (split vs rsplit)
By default, splitting is done from the left. To do splitting on the right, use rsplit.
默认情况下,拆分是从左侧开始的。 要在右侧进行拆分,请使用rsplit 。
Consider the series below:
考虑以下系列:
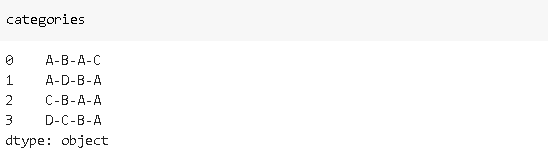
Let’s apply split function and limit the number of splits with n parameter:
让我们应用split函数并使用n参数限制拆分次数:
categories.str.split('-', expand=True, n=2)
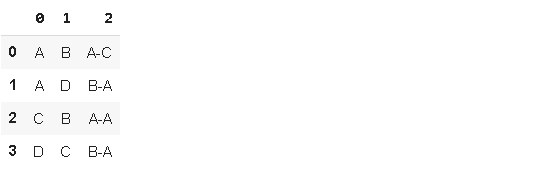
Only 2 splits on the left are performed. If we do the same operation with rsplit:
左侧仅执行2个拆分。 如果我们对rsplit执行相同的操作:
categories.str.rsplit('-', expand=True, n=2)
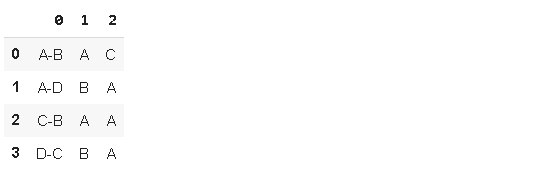
Same operation is done but on the right.
完成相同的操作,但在右侧。
2.剥离 (2. Stripping)
Stripping is like trimming tree branches. We can remove spaces or any other characters at the beginning or end of a string.
剥离就像修剪树枝。 我们可以删除字符串开头或结尾的空格或任何其他字符。
For instance, the strings in “col_b” has $ character at the beginning which can be removed with lstrip:
例如,“ col_b”中的字符串开头有$字符,可以使用lstrip将其删除:
df_sample['col_b'].str.lstrip('$')0 64K-$72K
1 62K-$70K
2 69K-$76K
3 62K-$72K
4 71K-$78K
Name: col_b, dtype: object
Similary, rstrip is used to trim off characters from the end.
类似地, rstrip用于从末尾修剪字符。
Strings may have spaces at the beginning or end. Consider “col_d” in our dataframe.
字符串的开头或结尾可以有空格。 考虑一下我们数据框中的“ col_d”。
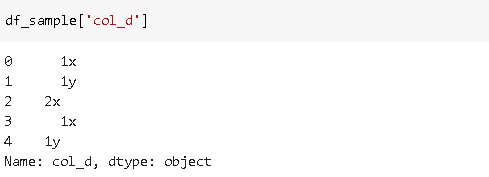
Those leading and trailing spaces can be removed with strip:
那些前导和尾随空格可以用strip除去:
df_sample['col_d'] = df_sample['col_d'].str.strip()
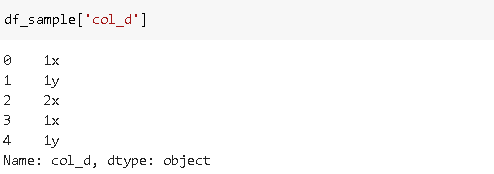
3.更换 (3. Replacing)
Pandas replace function is used to replace values in rows or columns. Similarly, replace as a string operation is used to replace characters in a string.
熊猫替换功能用于替换行或列中的值。 同样,替换为字符串操作用于替换字符串中的字符。
Let’s replace “x” letters in “col_d” with “z”.
让我们用“ z”替换“ col_d”中的“ x”个字母。
df_sample['col_d'] = df_sample['col_d'].str.replace('x', 'z')
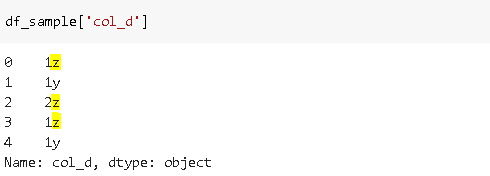
4.筛选 (4. Filtering)
We can filter strings based on the first and last characters. The functions to use are startswith() and endswith().
我们可以根据第一个和最后一个字符来过滤字符串。 要使用的函数是startswith()和endswith() 。
Here is our original dataframe:
这是我们的原始数据框:
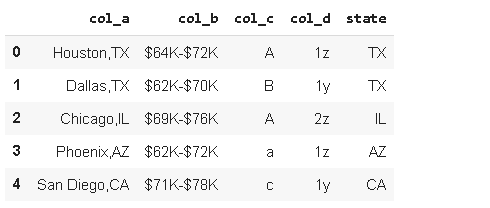
Here is a filtered version that only includes rows in which “col_a” ends with the letter “x”.
这是一个过滤的版本,仅包含“ col_a”以字母“ x”结尾的行。
df_sample[df_sample['col_a'].str.endswith('X')]
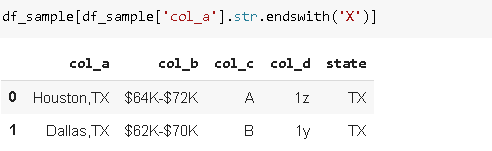
Or, rows in which “col_b” starts with “$6”:
或者,其中“ col_b”以“ $ 6”开头的行:
df_sample[df_sample['col_b'].str.startswith('$6')]
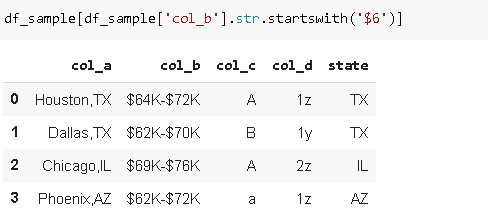
We can also filter strings by extracting certain characters. For instace, we can get the first 2 character of strings in a column or series by str[:2].
我们还可以通过提取某些字符来过滤字符串。 对于instace,我们可以通过str [:2]获得列或系列中字符串的前2个字符。
“col_b” represents a value range but numerical values are hidden in a string. Let’s extract them with string subscripts:
“ col_b”表示值范围,但数值隐藏在字符串中。 让我们用字符串下标提取它们:
lower = df_sample['col_b'].str[1:3]
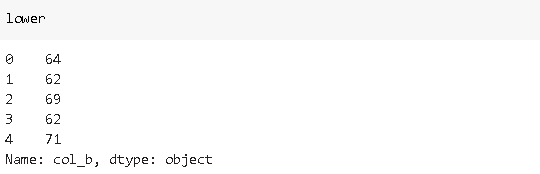
upper = df_sample['col_b'].str[-3:-1]
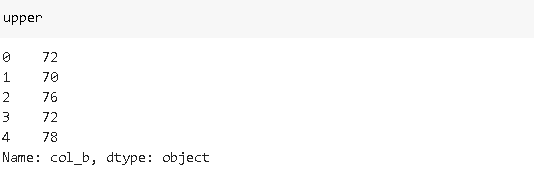
5.结合 (5. Combining)
Cat function can be used to concatenate strings.
Cat函数可用于连接字符串。
We need pass an argument to put between concatenated strings using sep parameter. By default, cat ignores missing values but we can also specify how to handle them using na_rep parameter.
我们需要传递一个参数,以使用sep参数在串联字符串之间放置。 默认情况下,cat会忽略缺失值,但我们也可以使用na_rep参数指定如何处理它们。
Let’s create a new column by concatenating “col_c” and “col_d” with “-” separator.
让我们通过将“ col_c”和“ col_d”与“-”分隔符连接起来创建一个新列。
df_sample['new']=df_sample['col_c'].str.cat(df_sample['col_d'], sep='-')df_sample
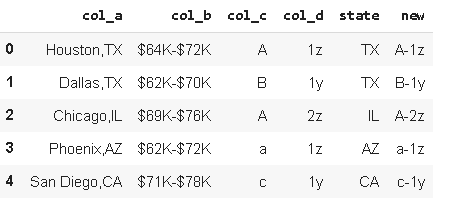
奖励:对象与字符串 (Bonus: Object vs String)
Before pandas 1.0, only “object” datatype was used to store strings which cause some drawbacks because non-string data can also be stored using “object” datatype. Pandas 1.0 introduces a new datatype specific to string data which is StringDtype. As of now, we can still use object or StringDtype to store strings but in the future, we may be required to only use StringDtype.
在pandas 1.0之前,仅使用“对象”数据类型存储字符串,这会带来一些缺点,因为非字符串数据也可以使用“对象”数据类型进行存储。 Pandas 1.0引入了特定于字符串数据的新数据类型StringDtype 。 到目前为止,我们仍然可以使用object或StringDtype来存储字符串,但是在将来,可能需要我们仅使用StringDtype。
One important thing to note here is that object datatype is still the default datatype for strings. To use StringDtype, we need to explicitly state it.
这里要注意的一件事是对象数据类型仍然是字符串的默认数据类型。 要使用StringDtype,我们需要明确声明它。
We can pass “string” or pd.StringDtype() argument to dtype parameter to string datatype.
我们可以将“ string ”或pd.StringDtype()参数传递给dtype参数,以传递给字符串数据类型。
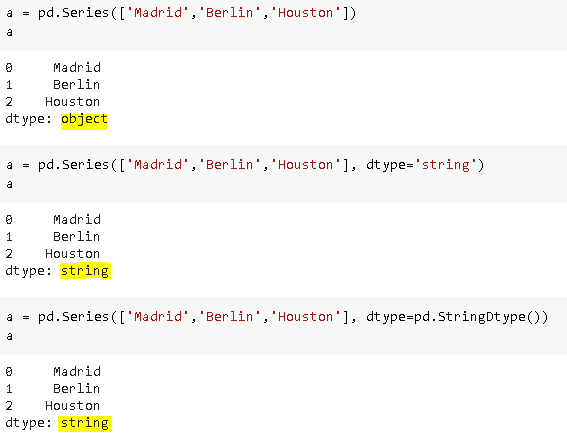
Thank you for reading. Please let me know if you have any feedback.
感谢您的阅读。 如果您有任何反馈意见,请告诉我。
翻译自: https://towardsdatascience.com/5-must-know-pandas-operations-on-strings-4f88ca6b8e25
字符串操作截取后面的字符串
本文来自互联网用户投稿,该文观点仅代表作者本人,不代表本站立场。本站仅提供信息存储空间服务,不拥有所有权,不承担相关法律责任。如若转载,请注明出处:http://www.mzph.cn/news/388207.shtml
如若内容造成侵权/违法违规/事实不符,请联系多彩编程网进行投诉反馈email:809451989@qq.com,一经查实,立即删除!