趣味数据故事
Meet Julia. She’s a data engineer. Julia is responsible for ensuring that your data warehouses and lakes don’t turn into data swamps, and that, generally speaking, your data pipelines are in good working order.
中号 EETJulia。 她是一名数据工程师。 Julia负责确保您的数据仓库和湖泊不会变成数据沼泽,并且通常来说,您的数据管道运行状况良好。
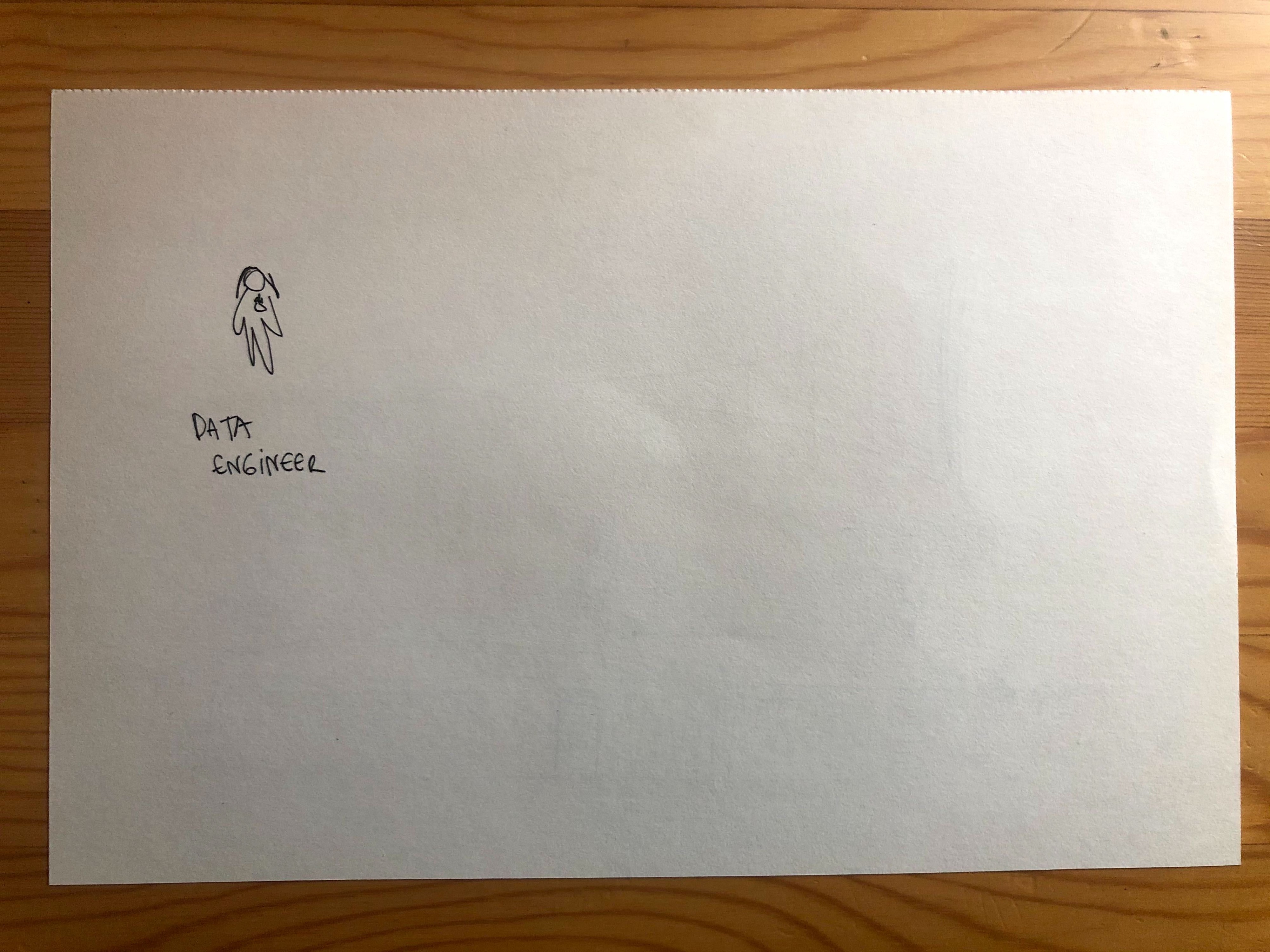
Julia is happy when nothing breaks, but like any good engineer, she knows that this is near-to impossible. So, she just wants to be the first to know when issues do arise so that she can solve them.
当一切都没有中断时,茱莉亚很高兴,但是像任何优秀的工程师一样,她知道这几乎是不可能的。 因此,她只想成为第一个知道问题何时发生的人,以便她可以解决问题。
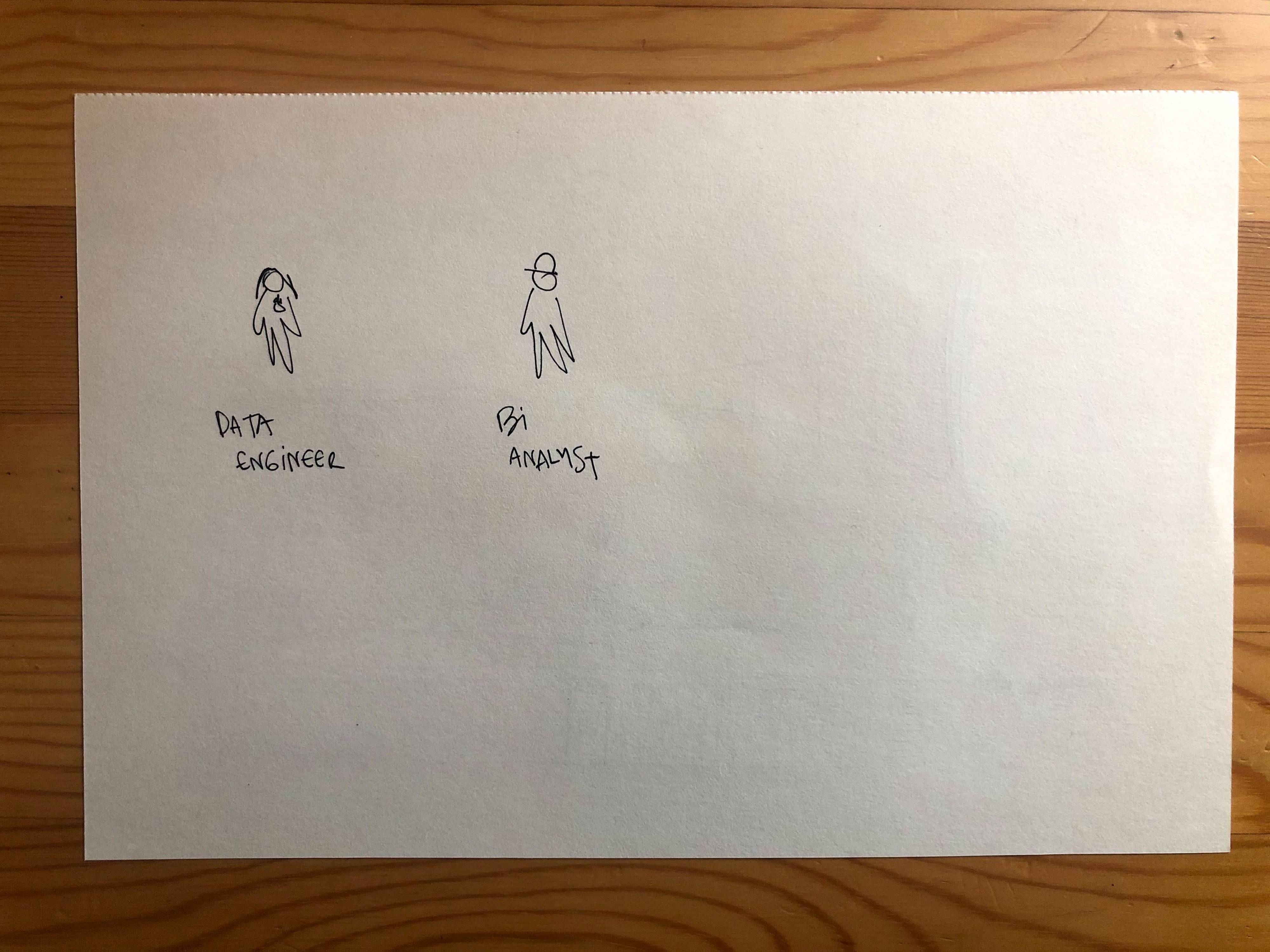
Meet Ted. He’s a data analyst. Ted is known by his company as the “SQL King” because he’s the go-to query wrangler for their Marketing, Customer Support, and Operations teams. He’s an expert in Tableau, and knows all the Excel hacks. Ted is also happy when nothing breaks, and like Julia, knows that this is impossible. However, Ted doesn’t want bad data to ruin his analytics, making his life and the lives of his stakeholders miserable (more on that later).
认识特德。 他是一名数据分析师。 Ted被他的公司称为“ SQL King”,因为他是其市场营销,客户支持和运营团队的首选查询管理员。 他是Tableau的专家,并且了解所有Excel技巧。 当一切都没有中断时,Ted也很高兴,并且像Julia一样,知道这是不可能的。 但是,Ted不想让不良数据破坏他的分析,从而使他的生活和利益相关者的生活变得痛苦不堪(稍后再详述)。
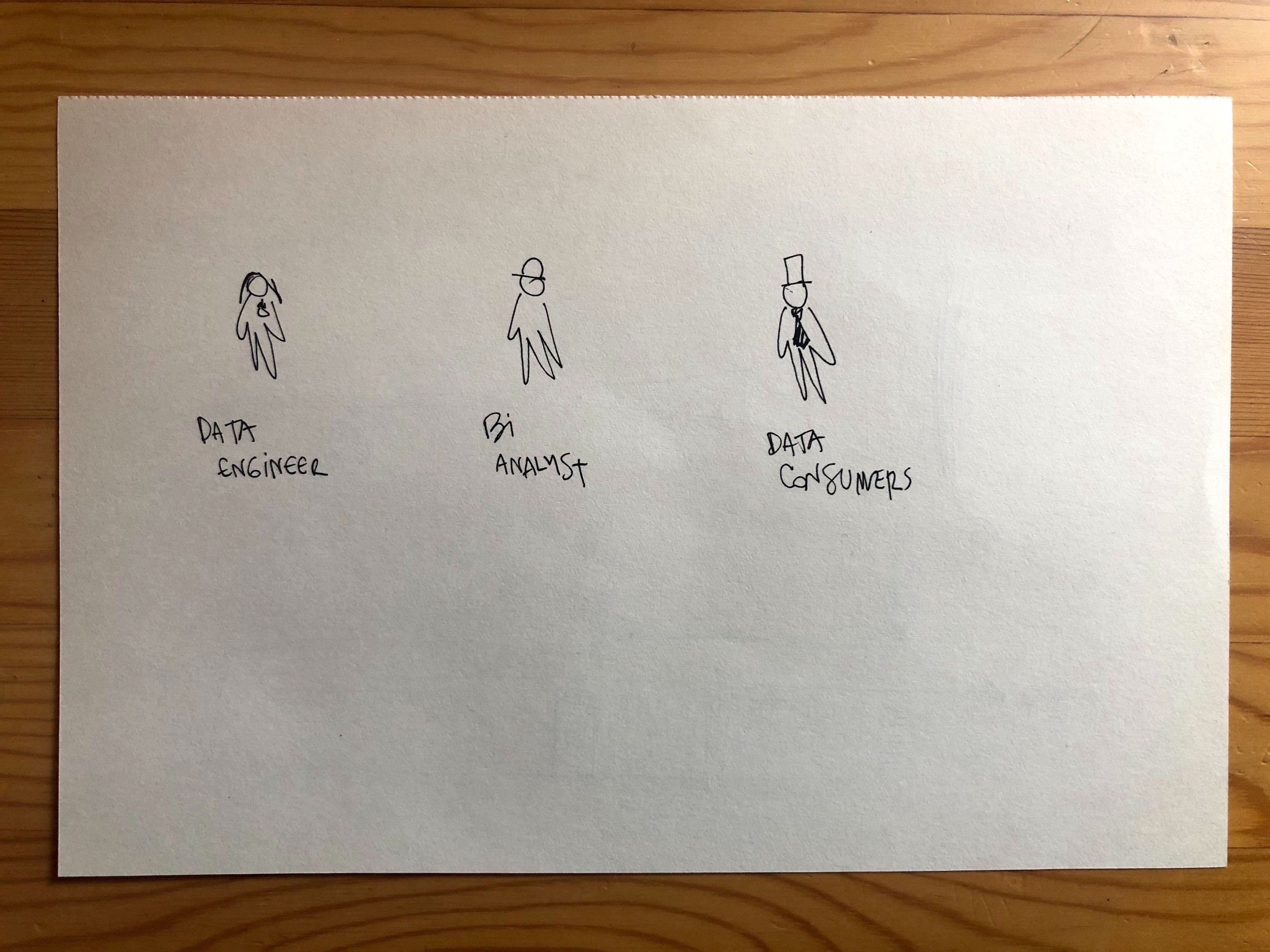
Meet Alex. Alex is a data consumer. She might be a data scientist, a product manager, a VP of Marketing, or even your CEO. Alex uses data to make smarter decisions, whether that’s what the title of her new product should be or which pair of lucky socks she should wear to tomorrow’s board meeting.
认识亚历克斯。 Alex是数据消费者。 她可能是数据科学家,产品经理,营销副总裁,甚至是您的CEO。 亚历克斯使用数据做出更明智的决策,无论这是她的新产品的名称,还是她应该在明天的董事会会议上穿的那双幸运袜子。
Alex, or anyone else at the company for that matter, can’t do their job if they can’t trust their data. We call this phenomena data downtime. Data downtime refers to periods of time where your data is inaccurate, missing, or otherwise erroneous, and spares no one, sort of like death and taxes. Unlike death and taxes, however, data downtime can be easily avoided if acted on immediately.
亚历克斯(Alex)或公司中与此有关的任何其他人,如果他们不信任自己的数据,就无法完成他们的工作。 我们称这种现象为数据停机时间。 数据停机时间是指您的数据不准确,丢失或以其他方式错误并且不遗余力的时间段,类似于死亡和税收。 但是,与死亡和税收不同,如果立即采取行动,可以轻松避免数据停机。
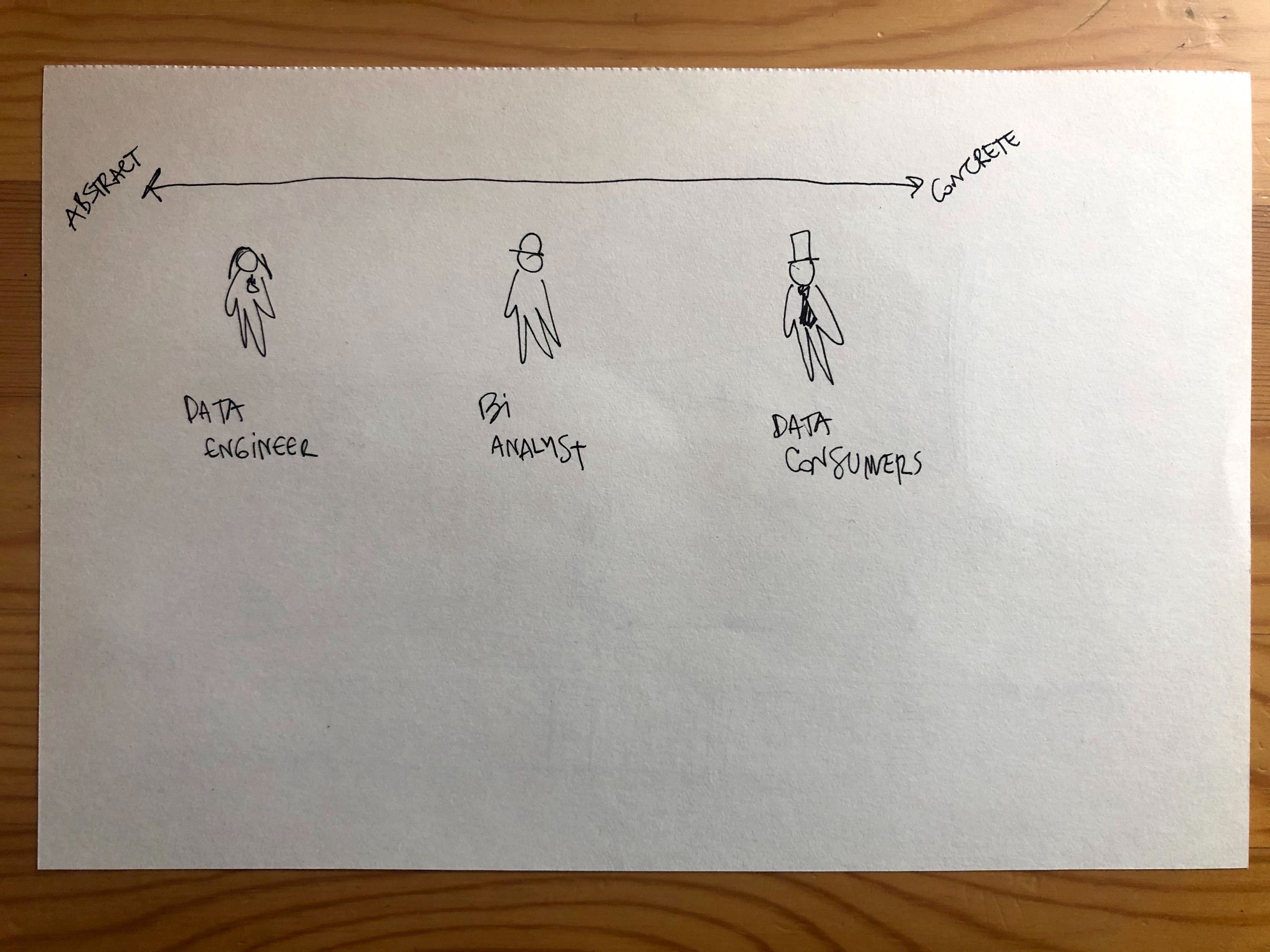
When raw data is consumed by your data pipeline, it’s abstract and meaningless on its own. It doesn’t really matter if there’s data downtime because no one is using it quite yet — other than Julia, to pass it on. The problem is, she doesn’t always know if data is broken.
当原始数据被数据管道消耗时,它本身就是抽象的且毫无意义。 是否存在数据停机时间并不重要,因为除了Julia之外,没有人正在使用它来传递数据。 问题是,她并不总是知道数据是否损坏。
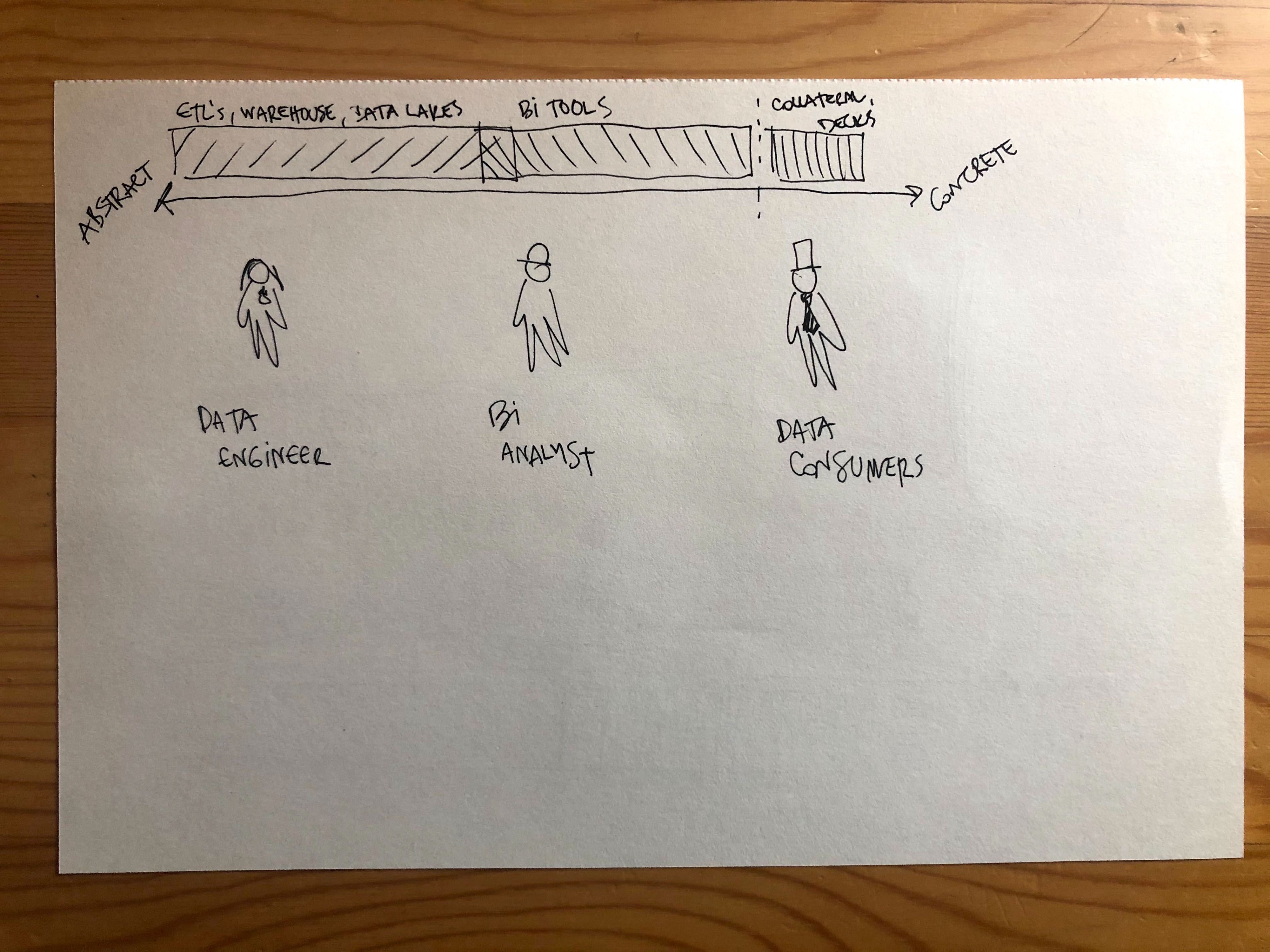
As data moves through the pipeline, it becomes more concrete. Once it reaches the company’s business intelligence tools, Ted can start using it, transforming what was formerly vague and abstract into Excel spreadsheets, Tableau dashboards, and other beautiful vessels of knowledge.
随着数据在管道中移动,它变得更加具体。 一旦它到达公司的商业智能工具,Ted就可以开始使用它,将以前模糊和抽象的内容转换为Excel电子表格,Tableau仪表板和其他精美的知识工具。
Ted can then transform this data (now nearing full maturity) into actionable insights for the rest of his company. Now, Alex can create marketing collateral and PDFs and customer decks with this data, which is polished and concrete and bound to save the world. Or is it?
然后,Ted可以将这些数据(现在已经接近完全成熟)转换为他的公司其余部分的可行见解。 现在,Alex可以使用这些数据创建营销资料,PDF和客户资料,这些数据经过精心处理和具体化,必将拯救世界。 还是?
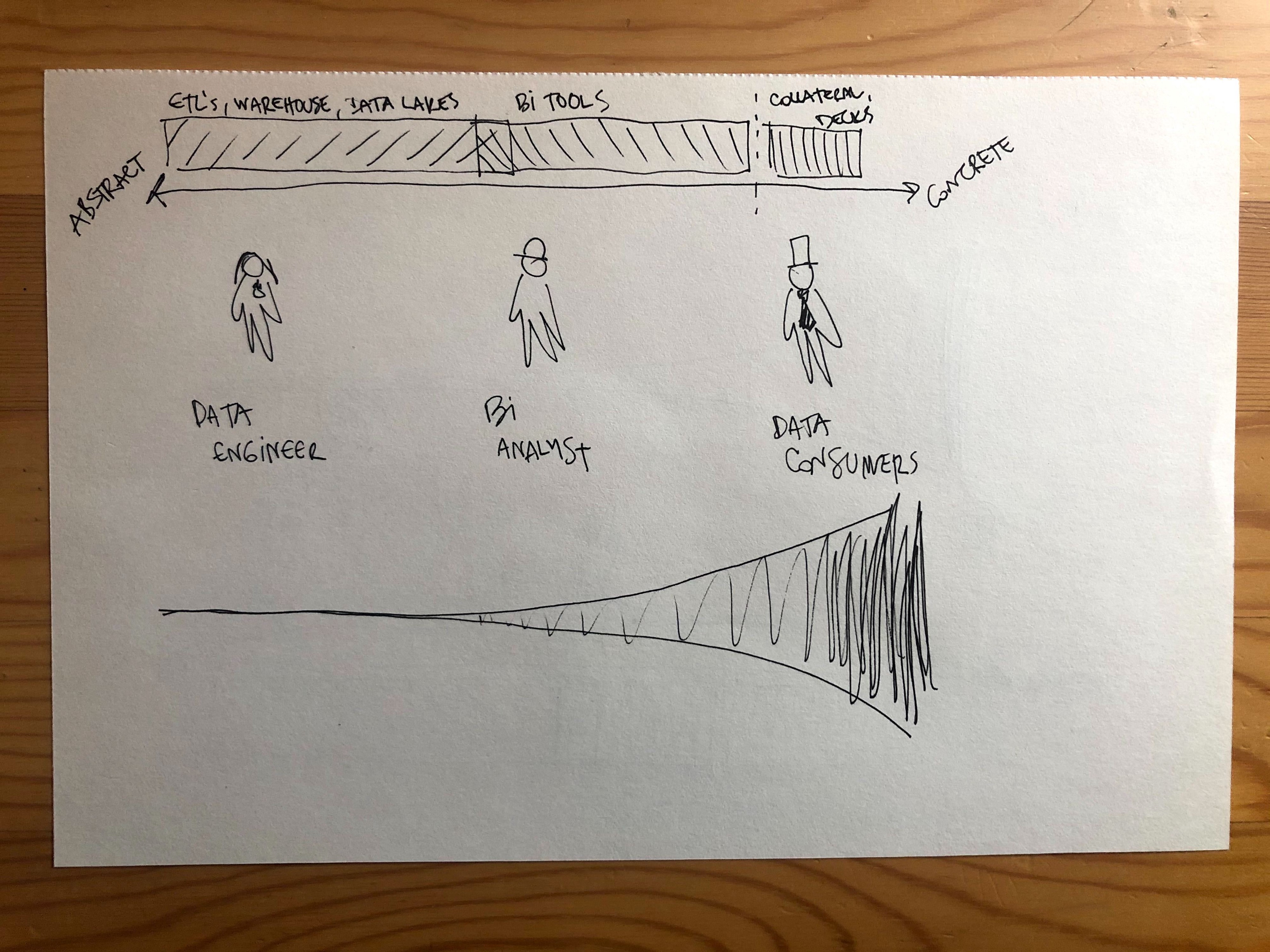
As data errors move down the pipeline, the severity of data downtime increases. There are more and more Teds and Alexs using the data, many of whom have no idea if what they’re looking at is right, wrong, or somewhere in between until it’s too late.
随着数据错误沿流水线向下移动,数据停机的严重性增加。 越来越多的Teds和Alexs使用这些数据,其中许多人不知道自己所看的内容是对,错还是介于两者之间,直到为时已晚。
When is too late, you might ask?
什么时候来不及,您可能会问?
Too late is when Julia is paged at 3 a.m. Monday morning by Ted who was called by Alex, his skip-level manager and the VP of Sales, only a few minutes before about a wonky report he was supposed to present the next morning to their CEO. Too late is when you’ve wasted time, lost revenue, and eroded Alex — and everyone else’s — precious trust.
太迟了,当周一早上3点,Julia(Julia)被特德(Ted)传呼时,特德(Ted)由他的跳级经理兼销售副总裁亚历克斯(Alex)召集,而几分钟前,他就应该在第二天早上向他们呈报一个奇怪的报告CEO。 浪费时间,失去收入,侵蚀亚历克斯(Alex)和其他所有人的宝贵信任已经为时已晚。
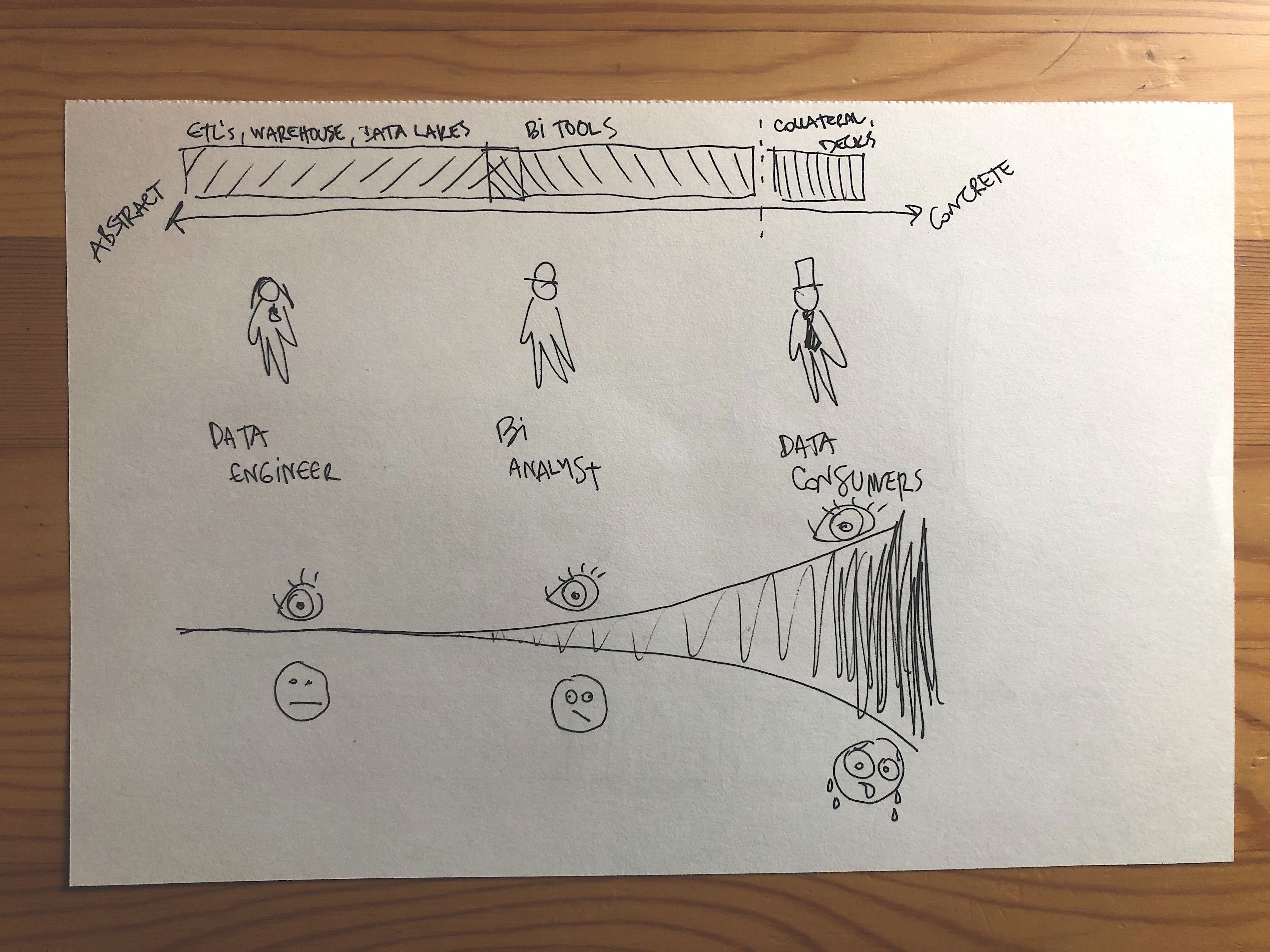
The more concrete and further removed the data gets from Julia’s raw tables, the more severe the impact. We refer to this as the cone of data anxiety.
从Julia的原始表中获取的数据越具体,越深入,影响就越严重。 我们将此称为数据焦虑症 。
Disaster struck and Julia had no idea why, let alone that it had happened. If only she had caught the data downtime immediately — right when it hit — instead of through Alex and her other data consumers (down the cone of anxiety), disaster could have been avoided.
灾难来了,Julia不知道为什么,更不用说发生了。 如果只有她立即(在命中时)捕获了数据停机,而不是通过Alex和她的其他数据消费者(在焦虑中),可以避免灾难。
Worst of all, she was in the middle of a once-in-a-lifetime dream. Cotton candy clouds, chocolate fountain waterfalls, and no null values. The complete opposite of the reality she was facing at 3 a.m. on Monday morning.
最糟糕的是,她处于千载难逢的梦想之中。 棉花糖云,巧克力喷泉瀑布,并且没有空值。 星期一早上3点,她所面对的现实完全相反。
Sounds familiar? Yeah, I’m with you.
听起来很熟悉? 是的,我和你在一起。
If data downtime is something you’ve experienced, we’d love to hear from you! Reach out to Barr with your own good tales of bad data.
如果您遇到数据宕机的情况,我们将很高兴收到您的来信! 伸出 巴尔坏数据的自己的好故事。
This article was co-written by Barr Moses & Martín Alonso Lago.
本文由 Barr Moses 和 MartínAlonso Lago 共同撰写 。
翻译自: https://towardsdatascience.com/good-tales-of-bad-data-91eccc29cbc5
趣味数据故事
本文来自互联网用户投稿,该文观点仅代表作者本人,不代表本站立场。本站仅提供信息存储空间服务,不拥有所有权,不承担相关法律责任。如若转载,请注明出处:http://www.mzph.cn/news/388129.shtml
如若内容造成侵权/违法违规/事实不符,请联系多彩编程网进行投诉反馈email:809451989@qq.com,一经查实,立即删除!