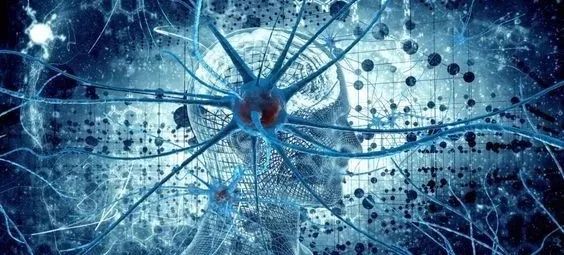
课程概要
本课程来自集智学园图网络论文解读系列活动。
是对论文《Graph WaveNet for Deep Spatial-Temporal Graph Modeling》的解读。
时空图建模 (Spatial-temporal graph modeling)是分析系统中组成部分的空间维相关性和时间维趋势的重要手段。已有算法大多基于已知的固定的图结构信息来获取空间相关性,而邻接矩阵所包含的连接关系并不能反应真实的节点间交互。此外,现有基于 RNN 和 CNN 的时域建模方式不能真正的捕捉其中所存在的长程相关。本文提出了一个新的图神经网络模型 Graph WaveNet 用于时空图建模。其中包括两个组件,一个是自适应依赖矩阵(adaptive dependency matrix),通过节点嵌入进行训练,用来精确建模节点的空间相关性。另一个是堆叠的 1D 带孔卷积(stacked dilated 1D convolution),增加了模型在时域的感受野的大小。通过两个交通流预测数据集的测试,Graph WaveNet 均能达到 state-of-the-art 的效果。课程资料论文题目:Graph WaveNet for Deep Spatial-Temporal Graph Modeling论文地址:https://arxiv.org/abs/1906.00121
课程讲师
王硕
王硕:2014年,毕业于东北大学模式识别与智能系统专业,2016年加入彩云天气,负责雾霾预报算法及系统。论文原文摘要
Spatial-temporal graph modeling is an important task to analyze the spatial relations and temporal trends of components in a system. Existing approaches mostly capture the spatial dependency on a fixed graph structure, assuming that the underlying relation between entities is pre-determined. However, the explicit graph structure (relation) does not necessarily reflect the true dependency and genuine relation may be missing due to the incomplete connections in the data. Furthermore, existing methods are ineffective to capture the temporal trends as the RNNs or CNNs employed in these methods cannot capture long-range temporal sequences. To overcome these limitations, we propose in this paper a novel graph neural network architecture, Graph WaveNet, for spatial-temporal graph modeling. By developing a novel adaptive dependency matrix and learn it through node embedding, our model can precisely capture the hidden spatial dependency in the data. With a stacked dilated 1D convolution component whose receptive field grows exponentially as the number of layers increases, Graph WaveNet is able to handle very long sequences. These two components are integrated seamlessly in a unified framework and the whole framework is learned in an end-to-end manner. Experimental results on two public traffic network datasets, METR-LA and PEMS-BAY, demonstrate the superior performance of our algorithm.
课程大纲
- 介绍了论文的基础信息和论文背景
- 介绍了本篇论文解决的科学问题——时空序列预测
- 分模块介绍了Graph WaveNet算法框架,同时说明每个模块相关的以往研究
- 介绍了对比模型,以及实验结果
- 展开相关的讨论,探讨文章算法的局限性
- 展示相关资源列表
录播学习
学习地址:
https://campus.swarma.org/play/coursedetail?id=11091
推荐学习
集智图网络线上读书会公开招募
图神经网络是深度学习领域的前沿热点议题,尤其是图网络(GraphNetworks)提出以来,深度学习有了实现因果推理的潜力。为了持续追踪相关领域的前沿进展,集智俱乐部联合北师大系统科学学院张江课题组,组织了以图网络为主题的线上读书会,研讨最新论文,孕育研究思路。每一期线上读书会由一位成员主讲,形式为论文分享,时间为每周一21:00-21:40。加入读书会群需报名审核,原则上参与者应有能力独立完成一次线上分享。如果你也正在从事图网络与深度学习方面的研究工作或技术实践,或者对该领域有强烈的学习意愿,欢迎填写报名表,申请加入“集智图网络论文分享小组”!报名请扫下方小程序码,填写报名表。填表之后会有入群方式。《产学结合:自然语言处理及其应用》课程报名
课程中,讲解了自然语言处理的最新学术理论并联合学术界和工业界,追踪最新进展与落地实践。课程由李嫣然、尹相志、小米科技相关技术负责人崔建伟和魏晨、彩云科技NLP算法工程师侯月源,合力打造了 12 节课程,涉及语言模型、机器翻译、情感分析、文本理解、知识图谱、文本生成六大主题。报名方式
点击立即报名https://campus.swarma.org/play/coursedetail?id=11076
集智学园公众号:swarmAI集智学园QQ群:426390994集智学园官网:campus.swarma.org商务合作&投稿转载|swarma@swarma.org
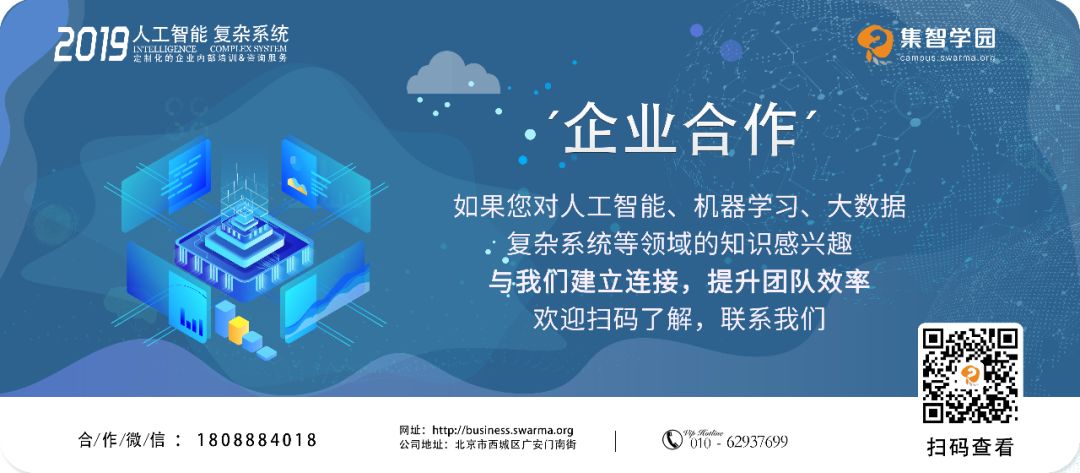