作者:郭震
51. How to get the row number of the nth largest value in a column?
Find the row position of the 5th largest value of column 'a' in df.
# input
df = pd.DataFrame(np.random.randint(1, 30, 30).reshape(10,-1), columns=list('abc'))
df# Solution 1# argsort give the index of the smallest to largest number in an array
# arg_sort[0] is the index of the smallest number in df["a"]
arg_sort = df["a"].argsort()#arg_sort.to_frame()
#arg_sort[0]# now let's sort by arg_sort
#df
df = df.iloc[arg_sort]
df["arg_sort"] = arg_sort
df
n_largest = 5
print("The {} largest values in our DF is at row/index {} and the value is {}".format(n_largest, (df[df["arg_sort"] == (n_largest-1)].index[0]), df[df["arg_sort"] == (n_largest-1)]["a"].iloc[0]))# Shorter solution
n = 5
# select column, argsort, inders (largest to smallest) and select the n largest
df['a'].argsort()[::-1][n]
52. How to find the position of the nth largest value greater than a given value?
In ser, find the position of the 2nd largest value greater than the mean.
# input
ser = pd.Series(np.random.randint(1, 100, 15))# Solution using argsort and boolean filtering of pandas series
# I understood that I wanted the second largest of all values that is greter than the mean
# so I sorted
#ser
sorted_ser = ser[ser.argsort()[::-1]]
#sorted_ser
sorted_ser[sorted_ser > sorted_ser.mean()].index[1]# If you understood that the 2 value you encounter that is bigger than the mean
# This is the correct solution
print('ser: ', ser.tolist(), 'mean: ', round(ser.mean()))
np.argwhere(ser > ser.mean())[1]# Another solution
ser[ser > ser.mean()].index[1]
53. How to get the last n rows of a dataframe with row sum > 100?
Get the last two rows of df whose row sum is greater than 100.
# input
df = pd.DataFrame(np.random.randint(10, 40, 60).reshape(-1, 4))
df1 = df.copy(deep = True)# Solution 1
df["sum"] = df.sum(axis = 1)
dfprint("The index of the rows that are greater than 100 are {}".format((df[df["sum"] > 100].index).to_list()[-2:]))# Solution 2 using numpy
rowsums = df1.apply(np.sum, axis=1)# last two rows with row sum greater than 100
last_two_rows = df1.iloc[np.where(rowsums > 100)[0][-2:], :]
last_two_rows
54. How to find and cap outliers from a series or dataframe column?
Replace all values of ser in the lower 5%ile and greater than 95%ile with respective 5th and 95th %ile value.
# input
ser = pd.Series(np.logspace(-2, 2, 30))
ser1 = ser.copy(deep = True)
ser2 = ser.copy(deep = True)# Solution 1
# get the quantiles values
quantiles = np.quantile(ser, [0.05, 0.95])
ser# filter ser using numpy to know where the values are below or greater than 5% or 95% and replace the values
ser.iloc[np.where(ser < quantiles[0])] = quantiles[0]
ser.iloc[np.where(ser > quantiles[1])] = quantiles[1]# or we can just do
ser1[ser1 < quantiles[0]] = quantiles[0]
ser1[ser1 > quantiles[1]] = quantiles[1]ser1# Solution from the webpage
def cap_outliers(ser, low_perc, high_perc):low, high = ser.quantile([low_perc, high_perc])print(low_perc, '%ile: ', low, '|', high_perc, '%ile: ', high)ser[ser < low] = lowser[ser > high] = highreturn(ser)capped_ser = cap_outliers(ser2, .05, .95)
ser2
capped_ser
55. How to reshape a dataframe to the largest possible square after removing the negative values?
Reshape df to the largest possible square with negative values removed. Drop the smallest values if need be. The order of the positive numbers in the result should remain the same as the original.
# input
df = pd.DataFrame(np.random.randint(-20, 50, 100).reshape(10,-1))# This solution sorts the values.
# Not want we want
# my_array = np.array(df.values.reshape(-1, 1))
# my_array = my_array[my_array > 0]
# my_array.shape[0]
# lar_square = int(np.floor(my_array.shape[0]#### 0.5))
# arg_sort = np.argsort(my_array)[::-1]
# my_array[arg_sort][0:lar_square#### 2].reshape(lar_square, lar_square)# Correct solution
my_array = np.array(df.values.reshape(-1, 1)) # convert to numpy
my_array = my_array[my_array > 0] # filter only positive values
lar_square = int(np.floor(my_array.shape[0]#### 0.5)) # find the largest square
arg_sort = np.argsort(my_array)[::-1][0:lar_square#### 2] # eliminate the smallest values that will prevent from converting to a square
my_array = np.take(my_array, sorted(arg_sort)).reshape(lar_square, lar_square) # filter the array and reshape back
my_array# Solution from the webpage
# Step 1: remove negative values from arr
arr = df[df > 0].values.flatten()
arr_qualified = arr[~np.isnan(arr)]# Step 2: find side-length of largest possible square
n = int(np.floor(arr_qualified.shape[0]#### .5))# Step 3: Take top n^2 items without changing positions
top_indexes = np.argsort(arr_qualified)[::-1]
output = np.take(arr_qualified, sorted(top_indexes[:n#### 2])).reshape(n, -1)
print(output)
56. How to swap two rows of a dataframe?
Swap rows 1 and 2 in df.
# input
df = pd.DataFrame(np.arange(25).reshape(5, -1))
df# THIS SWAPS the columns
print("Original DataFrame")
df
temp_col = df[1].copy(deep = True)
df[1], df[2] = df[2], temp_col
print("Swapped Columns DataFrame")
df# # THIS SWAPS the rows
print("Original DataFrame")
df
temp_row = df.iloc[1].copy(deep = True)
df.iloc[1], df.iloc[2] = df.iloc[2], temp_row
print("Swapped Rows DataFrame")
df# Solution from the webpage
def swap_rows(df, i1, i2):a, b = df.iloc[i1, :].copy(), df.iloc[i2, :].copy()df.iloc[i1, :], df.iloc[i2, :] = b, areturn dfprint(swap_rows(df, 1, 2))
57. How to reverse the rows of a dataframe?
Reverse all the rows of dataframe df.
# input
df = pd.DataFrame(np.arange(25).reshape(5, -1))# Solution 1
df
df.iloc[df.index.to_list()[::-1]]# Solutions from the webpage
# Solution 2
df.iloc[::-1, :]# Solution 3
print(df.loc[df.index[::-1], :])
58. How to create one-hot encodings of a categorical variable (dummy variables)?
Get one-hot encodings for column 'a' in the dataframe df and append it as columns.
# input
df = pd.DataFrame(np.arange(25).reshape(5,-1), columns=list('abcde'))'''
Desired Output0 5 10 15 20 b c d e
0 1 0 0 0 0 1 2 3 4
1 0 1 0 0 0 6 7 8 9
2 0 0 1 0 0 11 12 13 14
3 0 0 0 1 0 16 17 18 19
4 0 0 0 0 1 21 22 23 24
'''# Using pd.get_dummies
dummies = pd.get_dummies(df["a"])
df = pd.concat([dummies, df], axis = 1)
df# Solution from the webpage
# in one line
df_onehot = pd.concat([pd.get_dummies(df['a']), df[list('bcde')]], axis=1)
df_onehot
59. Which column contains the highest number of row-wise maximum values?
Obtain the column name with the highest number of row-wise maximum’s in df.
# input
df = pd.DataFrame(np.random.randint(1,100, 40).reshape(10, -1))# Solution 1
def get_col(df):columns = list(df.columns)df["col_index_with_max"] = ""for i in range(len(df)):row_values = list(df.iloc[i, :-1].values)max_value = np.max(row_values)col_index = row_values.index(max_value)df["col_index_with_max"].iloc[i] = col_indexget_col(df)df
print("The col with maximum amont of maximun per row if {} with a total of {} maximus".format(df.groupby("col_index_with_max").size()[::-1].index[0], \df.groupby("col_index_with_max").size()[::-1].values[0]))# Solution 2
# Another much more elegant solution from the webpage
print('Column with highest row maxes: ', df.apply(np.argmax, axis=1).value_counts().index[0])
60. How to create a new column that contains the row number of nearest column by euclidean distance?
Create a new column such that, each row contains the row number of nearest row-record by euclidean distance.
# input
df = pd.DataFrame(np.random.randint(1,100, 40).reshape(10, -1), columns=list('pqrs'), index=list('abcdefghij'))'''
Desired Outputdf
# p q r s nearest_row dist
# a 57 77 13 62 i 116.0
# b 68 5 92 24 a 114.0
# c 74 40 18 37 i 91.0
# d 80 17 39 60 i 89.0
# e 93 48 85 33 i 92.0
# f 69 55 8 11 g 100.0
# g 39 23 88 53 f 100.0
# h 63 28 25 61 i 88.0
# i 18 4 73 7 a 116.0
# j 79 12 45 34 a 81.0'''#######################################################################################################################################
# Solution 1
# input
df = pd.DataFrame(np.random.randint(1,100, 40).reshape(10, -1), columns=list('pqrs'), index=list('abcdefghij'))# place holders
corr_list = []
index_list = []# temporary var
max_corr = 0
current_index = ""# nested loop to calculate
for i in range(len(df)):for j in range(len(df)):if i == j:passelse:# distancecurr_corr = sum((df.iloc[i] - df.iloc[j])#### 2)#### .5# correlation#curr_corr = df.iloc[i].corr(df.iloc[j])if curr_corr >= max_corr:max_corr = curr_corrcurrent_index = list(df.index)[j]corr_list.append(max_corr)index_list.append(current_index)max_corr = 0current_index = ""df["nearest_row"] = index_list
df["dist"] = corr_list
df
df.drop(["nearest_row", "dist"], axis = 1, inplace = True)######################################################################################################################################## Solution from the webpage
# init outputs
nearest_rows = []
nearest_distance = []# iterate rows.
for i, row in df.iterrows():curr = rowrest = df.drop(i)e_dists = {} # init dict to store euclidean dists for current row.# iterate rest of rows for current rowfor j, contestant in rest.iterrows():# compute euclidean dist and update e_distse_dists.update({j: round(np.linalg.norm(curr.values - contestant.values))})# update nearest row to current row and the distance valuenearest_rows.append(max(e_dists, key=e_dists.get))nearest_distance.append(max(e_dists.values()))df['nearest_row'] = nearest_rows
df['dist'] = nearest_distance
df
61. How to know the maximum possible correlation value of each column against other columns?
For each column get the maximum possible correlation with other columns (only 1 value)
# input
df = pd.DataFrame(np.random.randint(1,100, 80).reshape(8, -1), columns=list('pqrstuvwxy'), index=list('abcdefgh'))# calculate the correlation, returns a matrix
df_corr = np.abs(df.corr())
# sorted -2 because it goes from min to max
# max = 1 because it's correlation againts each other
# so we pick -2
max_corr = df_corr.apply(lambda x: sorted(x)[-2], axis = 0)
max_corr
62. How to create a column containing the minimum by maximum of each row?
Compute the minimum-by-maximum for every row of df.
# input
df = pd.DataFrame(np.random.randint(1,100, 80).reshape(8, -1))
df1 = df.copy(deep = True)
df2 = df.copy(deep = True)# Solution 1
df["min_by_max"] = (df.apply(min, axis = 1)/df.apply(max, axis = 1))
df# Other solution from the webpage
# Solution 2
min_by_max = df1.apply(lambda x: np.min(x)/np.max(x), axis=1)
min_by_max
# Solution 3
min_by_max = np.min(df2, axis=1)/np.max(df2, axis=1)
min_by_max
63. How to create a column that contains the penultimate value in each row?
Create a new column 'penultimate' which has the second largest value of each row of df.
# input
df = pd.DataFrame(np.random.randint(1,100, 80).reshape(8, -1))# Using lambda and numpy partition
df["penultimate"] = df.apply(lambda x: np.partition(x, -2)[-2], axis = 1)
df
df.drop("penultimate", inplace = True, axis = 1)# Using lambda and python lists
df["penultimate"] = df.apply(lambda x: sorted(list(x))[-2], axis = 1)
df
df.drop("penultimate", inplace = True, axis = 1)# Solution from the webpage
out = df.apply(lambda x: x.sort_values().unique()[-2], axis=1)
df['penultimate'] = out
df
64. How to normalize all columns in a dataframe?
Normalize all columns of df by subtracting the column mean and divide by standard deviation.
Range all columns of df such that the minimum value in each column is 0 and max is 1.
Don’t use external packages like sklearn####
# input
df = pd.DataFrame(np.random.randint(1,100, 80).reshape(8, -1))
df1 = df.copy(deep = True)# First normalization: mean and std
df = df.apply(lambda x: ((x-np.mean(x))/np.std(x)), axis = 0)
df# min max
df1 = df1.apply(lambda x: ((x.max() - x)/(x.max() - x.min())).round(2))
df1
65. How to compute the correlation of each row with the suceeding row?
Compute the correlation of each row of df with its succeeding row.
# input
df = pd.DataFrame(np.random.randint(1,100, 80).reshape(8, -1))df["corr"] = 0
for i in range(len(df)-1):values1 = df.iloc[i, :-1].astype('float64')values2 = df.iloc[i+1, :-1].astype('float64')corr = values1.corr(values2)df["corr"].iloc[i] = corr
df
df.drop("corr", inplace = True, axis = 1)# Solution from the webpage
# using list comprehension
[df.iloc[i].corr(df.iloc[i+1]).round(2) for i in range(df.shape[0])[:-1]]
66. How to replace both the diagonals of dataframe with 0?
Replace both values in both diagonals of df with 0.
# input
df = pd.DataFrame(np.random.randint(1,100, 100).reshape(10, -1))
df1 = df.copy(deep = True)'''
Desired Output (might change because of randomness)# 0 1 2 3 4 5 6 7 8 9
# 0 0 46 26 44 11 62 18 70 68 0
# 1 87 0 52 50 81 43 83 39 0 59
# 2 47 76 0 77 73 2 2 0 14 26
# 3 64 18 74 0 16 37 0 8 66 39
# 4 10 18 39 98 0 0 32 6 3 29
# 5 29 91 27 86 0 0 28 31 97 10
# 6 37 71 70 0 4 72 0 89 12 97
# 7 65 22 0 75 17 10 43 0 12 77
# 8 47 0 96 55 17 83 61 85 0 86
# 9 0 80 28 45 77 12 67 80 7 0
'''# input
df = pd.DataFrame(np.random.randint(1,100, 100).reshape(10, -1))
df1 = df.copy(deep = True)# Using nested loops
print("Original DF")
df
for i in range(len(df)):for j in range(len(df)):if i == j:df.iloc[i ,j] = 0# Inverse the matrix so that we can replace the other diagonaldf[::-1].iloc[i, j] = 0print("DF from the solution 1")
df# Solution from the webpage
# Solution
for i in range(df1.shape[0]):df1.iat[i, i] = 0df1.iat[df1.shape[0]-i-1, i] = 0print("DF from the solution 2")
df1
67. How to get the particular group of a groupby dataframe by key?
This is a question related to understanding of grouped dataframe. From df_grouped, get the group belonging to 'apple' as a dataframe.
# input
df = pd.DataFrame({'col1': ['apple', 'banana', 'orange'] * 3,'col2': np.random.rand(9),'col3': np.random.randint(0, 15, 9)})df_grouped = df.groupby(['col1'])# Solution 1
pd.DataFrame(df_grouped)
df_grouped.groups["apple"]
df_grouped.get_group("apple")# Solution 2
for i, dff in df_grouped:if i == 'apple':print(dff)
68. How to get the n’th largest value of a column when grouped by another column?
In df, find the second largest value of 'rating' for 'banana'
# input
df = pd.DataFrame({'fruit': ['apple', 'banana', 'orange'] * 3,'rating': np.random.rand(9),'price': np.random.randint(0, 15, 9)})# Solution 1
grouped_by = df["rating"].groupby(df["fruit"])
grouped_by.get_group("banana")
list(grouped_by.get_group("banana"))[1]# Solution from the webpage
df_grpd = df['rating'].groupby(df.fruit)
df_grpd.get_group('banana')
df_grpd.get_group('banana').sort_values().iloc[-2]
69. How to compute grouped mean on pandas dataframe and keep the grouped column as another column (not index)?
In df, Compute the mean price of every fruit, while keeping the fruit as another column instead of an index.
# input
df = pd.DataFrame({'fruit': ['apple', 'banana', 'orange'] * 3,'rating': np.random.rand(9),'price': np.random.randint(0, 15, 9)})
df# Using pandas pivot table
df_grouped = pd.pivot_table(df[["fruit", "price"]], index = ["fruit"], aggfunc = np.mean ).reset_index()
df_grouped# using groupby
out = df.groupby('fruit', as_index=False)['price'].mean()
out
70. How to join two dataframes by 2 columns so they have only the common rows?
Join dataframes df1 and df2 by ‘fruit-pazham’ and ‘weight-kilo’.
# input
df1 = pd.DataFrame({'fruit': ['apple', 'banana', 'orange'] * 3,'weight': ['high', 'medium', 'low'] * 3,'price': np.random.randint(0, 15, 9)})df2 = pd.DataFrame({'pazham': ['apple', 'orange', 'pine'] * 2,'kilo': ['high', 'low'] * 3,'price': np.random.randint(0, 15, 6)})
df1
df2# Solution 1
# using pandas merge
merge_df = pd.merge(df1, df2, left_on=["fruit", "weight"], right_on=["pazham", "kilo"])
merge_df# Solution from the webpage
pd.merge(df1, df2, how='inner', left_on=['fruit', 'weight'], right_on=['pazham', 'kilo'], suffixes=['_left', '_right'])
71. How to remove rows from a dataframe that are present in another dataframe?
From df1, remove the rows that are present in df2. All three columns must be the same.
# input
df1 = pd.DataFrame({'fruit': ['apple', 'banana', 'orange'] * 3,'weight': ['high', 'medium', 'low'] * 3,'price': np.random.randint(0, 10, 9)})df2 = pd.DataFrame({'pazham': ['apple', 'orange', 'pine'] * 2,'kilo': ['high', 'low'] * 3,'price': np.random.randint(0, 10, 6)})df1
df2# We might use pandas merge
#df1.merge(df2, how = "inner", left_on = ["fruit", "weight", "price"], right_on = ["pazham", "kilo", "price"])df1["concat"] = df1["fruit"].astype(str) + df1["weight"].astype(str) + df1["price"].astype(str)
#df1df2["concat"] = df2["pazham"].astype(str) + df2["kilo"].astype(str) + df2["price"].astype(str)
#df2df1 = df1[~df1["concat"].isin(df2["concat"])]
df1.drop("concat", inplace = True, axis = 1)
df1# Solution from the webpage, IMHO it's incorrect
#df1[~df1.isin(df2).all(1)]
72. How to get the positions where values of two columns match?
Find the index where col fruit1 and fruit2 match
# input
df = pd.DataFrame({'fruit1': np.random.choice(['apple', 'orange', 'banana'], 10),'fruit2': np.random.choice(['apple', 'orange', 'banana'], 10)})
df# Solution
np.where(df.fruit1 == df.fruit2)
73. How to create lags and leads of a column in a dataframe?
Create two new columns in df, one of which is a lag1 (shift column a down by 1 row) of column ‘a’ and the other is a lead1 (shift column b up by 1 row).
# input
df = pd.DataFrame(np.random.randint(1, 100, 20).reshape(-1, 4), columns = list('abcd'))
df'''
Desired Outputa b c d a_lag1 b_lead1
0 66 34 76 47 NaN 86.0
1 20 86 10 81 66.0 73.0
2 75 73 51 28 20.0 1.0
3 1 1 9 83 75.0 47.0
4 30 47 67 4 1.0 NaN
'''df["lag1"] = df["a"].shift(1)
df["lead1"] = df["b"].shift(-1)
df
74. How to get the frequency of unique values in the entire dataframe?
Get the frequency of unique values in the entire dataframe df.
# input
df = pd.DataFrame(np.random.randint(1, 10, 20).reshape(-1, 4), columns = list('abcd'))# Solution
pd.value_counts(df.values.ravel())
75. How to split a text column into two separate columns?
Split the string column in df to form a dataframe with 3 columns as shown.
# input
df = pd.DataFrame(["STD, City State",
"33, Kolkata West Bengal",
"44, Chennai Tamil Nadu",
"40, Hyderabad Telengana",
"80, Bangalore Karnataka"], columns=['row'])df'''
Desired Output0 STD City State
1 33 Kolkata West Bengal
2 44 Chennai Tamil Nadu
3 40 Hyderabad Telengana
4 80 Bangalore Karnataka
'''# we do " ".join(x.split()) to replace multiple spaces to 1 space
# we do split(None, 2, ) to split a string on the second space ()this way we have West Bengal together
df["re"] = df["row"].apply(lambda x: " ".join(x.split()).split(None, 2, ))new_header = df["re"][0]
values = df["re"][1:]# our values is a series of lists, we have to do some list comprehension no extract the values
d = {new_header[0]:[int(values.iloc[i][0].replace(",", "")) for i in range(len(values))], \new_header[1]:[values.iloc[i][1].replace(",", "") for i in range(len(values))], \new_header[2]:[values.iloc[i][2].replace(",", "") for i in range(len(values))]}# create a pandas DF from a dict
new_df = pd.DataFrame(d)
new_df
感谢你的支持,原创不易,希望转发,点击,以及收藏,也可以点击阅读原文更多AI知识分享,同时也可以关注知识星球:郭震AI学习星球
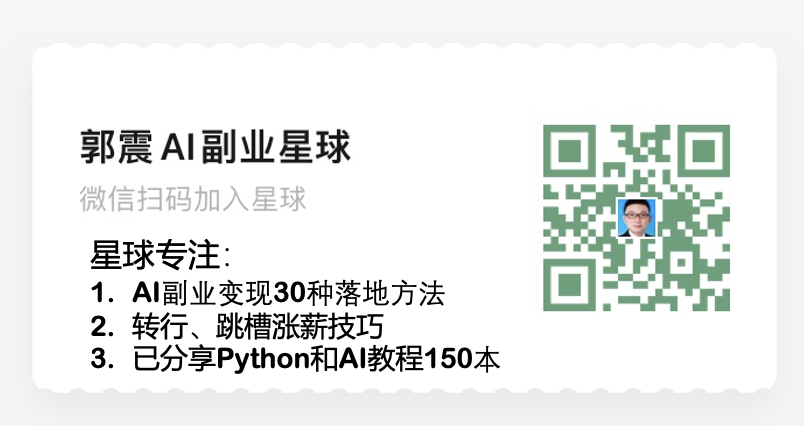
长按上图二维码查看「郭震AI学习星球」
更多Python、数据分析、爬虫、前后端开发、人工智能等教程参考.
以上全文,欢迎继续点击阅读原文学习,AI资讯,[请点击这里] https://ai-jupyter.com/