一 引言
近10年里以深度学习为代表的机器学习技术在图像处理,语音识别,自然语言处理等领域里取得了非常多的突破,其背后的核心算法是深度学习为代表的AI基础模型。
一般来讲,我们进行AI项目研发时,遵循三个步骤。
第一步,我们需要针对目标任务选定一个合适的模型架构,然后训练出满足精度的模型,这就是模型设计;
第二步,我们需要基于第一步训练好的模型,在不显著降低其精度的前提下,对模型的冗余参数进行精简,对高精度计算进行低精度近似,这就是模型压缩;
第三步,将模型运用于实际的生产环境,即面向用户的产品,这就是模型部署。
深度学习模型必须要部署到实际的生产环境中才能产生真正的应用价值。在各类落地场景中,有的是服务端应用,它需要模型有更高的精度、更复杂的功能;有的是嵌入式平台应用,诸如手机等各类移动端设备与车载设备,它需要模型具有体积小、低延迟特性。因此我们在进行模型设计与部署的时候,需要根据应用场景选择不同的模型和工具。当前模型优化和部署的工具非常多,常见的包括TensorRT、NCNN等;当前的硬件计算平台也非常多,包括CPU、GPU,NPU、FPGA等。
现在市面上有各种算力的嵌入式设备,强劲一点的如NVIDIA Jetson,弱一点的如树莓派,本次我们从CSDN社区收到了一块OrangePi Kunpeng Pro(香橙派开发板),正好来试一试这块板子的使用与性能。
下面是这块板子的实拍图与开机界面。
二 熟悉板子
拿到板子后当然首先要熟悉一下基本情况,包括软硬件相关信息。
第一步:使用/etc/os-release命令查看系统信息。
该板子的操作系统是openeuler 22.03(LTS-SP3),前身是华为自主研发的服务器操作系统EulerOS,其名字来自于1752年数学家欧拉所发现的欧拉公式。后来华为将其捐赠给开放原子开源基金会(OpenAtom Foundation)孵化及运营,项目地址为https://gitee.com/openeuler。
openEuler本质上也是一个Linux系统,每两年推出一个LTS版本,每半年发布一次创新版,支持鲲鹏及其他多种处理器,许多系统底层命令与一般Linux系统无异,一般的文件与目录操作等命令,即ls,cd,cp等均不变,软件安装则与centos一样使用yum或者dnf管理。
第二步:修改一些相关配置
一个全新的Linux系统,总有一些东西需要先改一改,比如默认的软件源。直接打开/etc/yum.repos.d/openEuler.repo,将其内容替换为以下内容。
[OS]name=OSbaseurl=https://mirrors.aliyun.com/openeuler/openEuler-22.03-LTS-SP2/OS/$basearch/enabled=1gpgcheck=1gpgkey=https://mirrors.aliyun.com/openeuler/openEuler-22.03-LTS-SP2/OS/$basearch/RPM-GPG-KEY-openEuler[everything]name=everythingbaseurl=https://mirrors.aliyun.com/openeuler/openEuler-22.03-LTS-SP2/everything/$basearch/enabled=1gpgcheck=1gpgkey=https://mirrors.aliyun.com/openeuler/openEuler-22.03-LTS-SP2/everything/$basearch/RPM-GPG-KEY-openEuler[EPOL]name=EPOLbaseurl=https://mirrors.aliyun.com/openeuler/openEuler-22.03-LTS-SP2/EPOL/main/$basearch/enabled=1gpgcheck=1gpgkey=https://mirrors.aliyun.com/openeuler/openEuler-22.03-LTS-SP2/OS/$basearch/RPM-GPG-KEY-openEuler[debuginfo]name=debuginfobaseurl=https://mirrors.aliyun.com/openeuler/openEuler-22.03-LTS-SP2/debuginfo/$basearch/enabled=1gpgcheck=1gpgkey=https://mirrors.aliyun.com/openeuler/openEuler-22.03-LTS-SP2/debuginfo/$basearch/RPM-GPG-KEY-openEuler[source]name=sourcebaseurl=https://mirrors.aliyun.com/openeuler/openEuler-22.03-LTS-SP2/source/enabled=1gpgcheck=1gpgkey=https://mirrors.aliyun.com/openeuler/openEuler-22.03-LTS-SP2/source/RPM-GPG-KEY-openEuler[update]name=updatebaseurl=https://mirrors.aliyun.com/openeuler/openEuler-22.03-LTS-SP2/update/$basearch/enabled=1gpgcheck=1gpgkey=https://mirrors.aliyun.com/openeuler/openEuler-22.03-LTS-SP2/OS/$basearch/RPM-GPG-KEY-openEuler[update-source]name=update-sourcebaseurl=https://mirrors.aliyun.com/openeuler/openEuler-22.03-LTS-SP2/update/source/enabled=1gpgcheck=1gpgkey=https://mirrors.aliyun.com/openeuler/openEuler-22.03-LTS-SP2/source/RPM-GPG-KEY-openEuler
再运行命令更新
sudo dnf clean all
sudo dnf makecache
第三步:查看硬件配置
由于接下来我们要测试AI项目,因此对于板子的硬件能力心里还是要有底。
可以看到是64bit系统,6个CPU,8GB内存,算力暂时不详。
第四步:安装一些必要的库,包括opencv-cv,protobuf等。
sudo dnf install protobuf-compiler protobuf # 文件格式库
sudo dnf install gtk2-devel gtk2-devel-docs # 桌面显示库
sudo dnf install pkg-config # 编译器的辅助工具,可以帮助 GCC 找到所需要的头文件与库文件路径
sudo dnf install mlocate # 文件查找库
sudo dnf install opencv opencv-devel
sudo pip install opencv-python # opencv库
sudo pip install torch # torch库
sudo pip install torchvision # torchvision库
sudo pip install onnxruntime # onnx库
系统已经预装了python3.9,后续使用如果还缺什么就用pip装就是。
三 模型部署
接下来我们使用移动端NCNN框架,以及香橙派,来体验典型的移动端模型部署流程,包括模型的格式转换、模型量化、基于C++的模型推理部署。
框架介绍
本次我们选择的部署框架是NCNN,它是一个在工业界被广为使用的框架,具有非常好的性能。
NCNN是一个纯C++实现的框架,无任何第三方库依赖,不依赖 BLAS/NNPACK 等计算框架,提供了ARM NEON 汇编级良心优化,计算速度极快。NCNN提供了对所有主流操作系统的支持,如图所示;
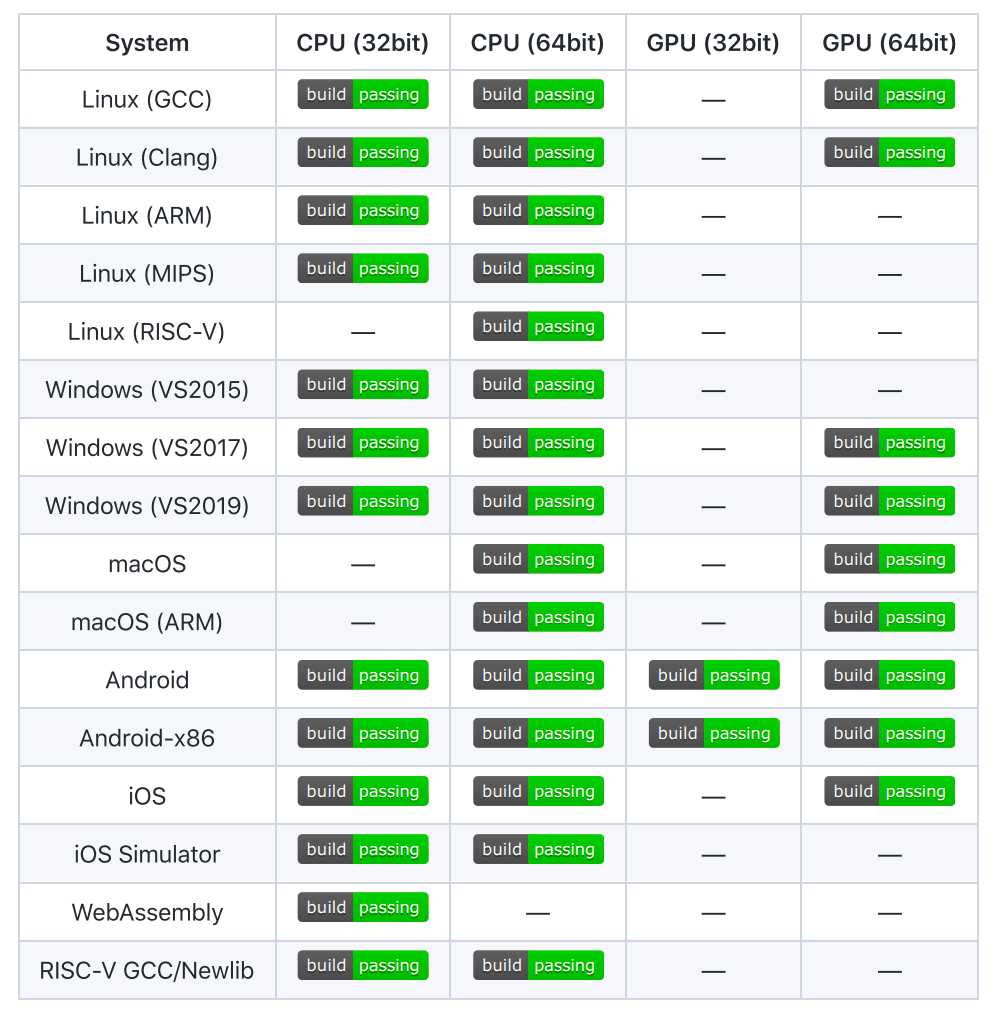
NCNN支持PaddlePaddle/PyTorch/TensorFlow/Caffe/MXNet/DarkNet/OneFlow/ONNX等深度学习框架文件格式,支持CNN、GAN等常用网络模型结构;
NCNN支持Intel架构的CPU与GPU,AMD架构的CPU与GPU,Arm架构的CPU与GPU,高通架构的CPU与GPU,Apple架构的CPU与GPU,其中对高通公司的CPU,ARM公司的CPU以及Apple公司的CPU提供了非常高效率地优化加速;
NCNN支持FP32/FP16/INT8/UINT8等多种运算精度;
NCNN支持C/C++/Python API;
NCNN支持直接内存零拷贝引用加载网络模型,可注册自定义层实现并扩展;
要使用NCNN,首先需要下载源码进行编译安装,相关代码命令如下:
git clone https://github.com/Tencent/ncnn
cd ncnn
mkdir build && cd build
cmake ..
make -j
make install
安装完之后,就可以在build/install目录下看到生成的一系列可执行文件和需要的库文件,它们分别存储于bin子目录和include子目录。如果github访问不了,就换gitee地址。
模型转换与量化
在进行部署之前,需要对模型格式进行转换,相关工具在ncnn根目录/build/install/bin目录下,包括
caffe2ncnn:caffe模型转换工具
darknet2ncnn: mxnet模型转换工具
mxnet2ncnn: mxnet模型转换工具
onnx2ncnn: onnx模型转换工具
ncnn2table, ncnn2int8: 模型量化工具
ncnn2mem:模型加密可执行文件:
ncnnoptimize:模型优化可执行文件
ncnnmerge:模型合并可执行文件
完整的模型量化流程可以分为3步,以ONNX格式为例:
第一步,将ONNX格式的模型转换为NCNN格式的模型,所使用的模型是一个训练好的图像分类模型。
其模型配置如下:
## 简单模型定义
class simpleconv5(nn.Module):def __init__(self,nclass):super(simpleconv5,self).__init__()self.conv1 = nn.Conv2d(3, 32, 3, 2, 1, bias=False)self.bn1 = nn.BatchNorm2d(32)self.conv2 = nn.Conv2d(32, 64, 3, 2, 1, bias=False)self.bn2 = nn.BatchNorm2d(64)self.conv3 = nn.Conv2d(64, 128, 3, 2, 1, bias=False)self.bn3 = nn.BatchNorm2d(128)self.conv4 = nn.Conv2d(128, 256, 3, 2, 1, bias=False)self.bn4 = nn.BatchNorm2d(256)self.conv5 = nn.Conv2d(256, 512, 3, 2, 1, bias=False)self.bn5 = nn.BatchNorm2d(512)self.fc = nn.Linear(512, nclass)def forward(self , x):x = F.relu(self.bn1(self.conv1(x)))x = F.relu(self.bn2(self.conv2(x)))x = F.relu(self.bn3(self.conv3(x)))x = F.relu(self.bn4(self.conv4(x)))x = F.relu(self.bn5(self.conv5(x)))x = nn.AvgPool2d(7)(x)x = x.view(x.size(0), -1) x = self.fc(x)return x
onnx2ncnn simpleconv5.onnx simpleconv5.param simpleconv5.bin
生成的ncnn格式的模型包括两个文件,simpleconv5.param是网络的配置文件,simpleconv5.bin是网络的权重文件。
第二步,生成int8量化所需要的校准表。
ncnn2table models/simpleconv5.param models/simpleconv5.bin images.txt simpleconv5.table mean=[127.5,127.5,127.5] norm=[0.00784,0.00784,0.00784] shape=[48,48,3] pixel=RGB
其中ncnn2table工具默认使用基于KL散度的8bit量化算法,它输入模型文件simpleconv5.param和simpleconv5.bin,校准表图片路径images.txt,预处理均值mean与标准化norm值,输入图片尺寸,RGB图片的格式,输出simpleconv5.table,即校准表。pixel=RGB表示输入网络的图片是RGB格式,当我们使用OpenCV进行图片读取后是BGR格式,两者需要进行区分。由于ncnn框架读取的图片数据像素值范围是0到127,而模型训练时采用的预处理操作包括除以255进行归一化,再减去均值向量[0.5,0.5,0.5],除以方差向量[0.5,0.5,0.5],因此这里对应的预处理操作需要将归一化操作合并到减均值操作和除以方差操作中,mean=255×[0.5,0.5,0.5]=[127.5,127.5,127.5], norm=1/255/[0.5,0.5,0.5]=[0.00784,0.00784,0.00784]
第三步,基于校准表进行量化。
量化前模型大小为6.4MB,量化后模型大小为1.6MB,8bit模型大小为float32模型大小的1/4,减少了存储空间。
模型部署测试
接下来我们使用C++接口对模型进行部署测试,并比较量化前后的模型精度是否受到严重影响,测试的核心C++功能函数代码如下:
#include "net.h"#include <algorithm>
#if defined(USE_NCNN_SIMPLEOCV)
#include "simpleocv.h"
#else
#include <opencv2/core/core.hpp>
#include <opencv2/highgui/highgui.hpp>
#include <opencv2/imgproc/imgproc.hpp>
#endif
#include <stdio.h>
#include <vector>
#include <cmath>
//推理函数
static int detect_simpleconv5net(const ncnn::Net &simpleconv5net,const cv::Mat& bgr, std::vector<float>& cls_scores)
{ncnn::Mat in = ncnn::Mat::from_pixels_resize(bgr.data, ncnn::Mat::PIXEL_BGR2RGB, bgr.cols, bgr.rows, 224, 224);//读取图片数据const float mean_vals[3] = {0.5f*255.f, 0.5f*255.f, 0.5f*255.f};const float norm_vals[3] = {1/0.5f/255.f, 1/0.5f/255.f, 1/0.5f/255.f};in.substract_mean_normalize(mean_vals, norm_vals); //预处理ncnn::Extractor ex = simpleconv5net.create_extractor();//创建推理引擎ex.input("input.1", in); //填充数据ncnn::Mat out;float start_time = cv::getTickCount(); //计算模型推理时间ex.extract("59", out); //获得模型推理结果float end_time = cv::getTickCount();fprintf(stderr, "%s = %f %s\n", "inference time = ", (end_time-start_time)/cv::getTickFrequency()*1000, " ms");cls_scores.resize(out.w); //取softmax分类概率结果,指数减去固定值防止溢出处理float maxscore = 0.0;for (int j = 0; j < out.w; j++){if(out[j] >= maxscore) maxscore = out[j]; cls_scores[j] = out[j];}float sum = 0.0;for (int j = 0; j < out.w; j++){cls_scores[j] = std::exp(cls_scores[j]-maxscore);sum += cls_scores[j]; }for (int j = 0; j < out.w; j++){cls_scores[j] = cls_scores[j] / sum;}return 0;
}int main(int argc, char** argv)
{if (argc != 5){fprintf(stderr, "Usage: %s%s%s [modelparam modelbin imagepath resultpath]\n", argv[0], argv[1], argv[2], argv[3]);return -1;}const char* modelparam = argv[1];const char* modelbin = argv[2];const char* imagepath = argv[3];const char* resultpath = argv[4];//初始化模型ncnn::Net simpleconv5net;simpleconv5net.opt.use_vulkan_compute = true;simpleconv5net.load_param(modelparam);simpleconv5net.load_model(modelbin);cv::Mat image = cv::imread(imagepath, 1);if (image.empty()){fprintf(stderr, "cv::imread %s failed\n", imagepath);return -1;}//获得topk的分类概率std::vector<float> cls_scores;detect_simpleconv5net(simpleconv5net, image, cls_scores);int topk = 1;int size = cls_scores.size();std::vector<std::pair<float, int> > vec;vec.resize(size);for (int i = 0; i < size; i++){vec[i] = std::make_pair(cls_scores[i], i);}std::partial_sort(vec.begin(), vec.begin() + topk, vec.end(),std::greater<std::pair<float, int> >());for (int i = 0; i < topk; i++){float score = vec[i].first;int index = vec[i].second;fprintf(stderr, "%d = %f\n", index, score);}//绘制结果std::string text;std::string label = "c="+std::to_string(vec[0].second);std::string prob = "prob="+std::to_string(vec[0].first);text.assign(label+" ");text.append(prob);int font_face = cv::FONT_HERSHEY_COMPLEX; double font_scale = 0.75;int thickness = 2;//将文本框居中绘制cv::Mat showimage = image.clone();cv::resize(showimage,showimage,cv::Size(256,256));cv::Point origin; origin.x = showimage.cols / 20;origin.y = showimage.rows / 2;cv::putText(showimage, text, origin, font_face, font_scale, cv::Scalar(0, 255, 255), thickness, 8, 0);cv::namedWindow("image",0);cv::imshow("image",showimage);//cv::waitKey(0);cv::imwrite(resultpath,showimage);return 0;
}
NCNN中每一层的数据被保存为自定义的Mat类型数据,它使用from_pixels_resize函数将OpenCV读取的Mat矩阵数据进行转换,由于计算使用了汇编,非常高效。网络定义为一个ncnn::Net类,格式与Caffe中的Net类非常相似,包含了layers和blobs成员变量,其中layers储存了每一层的信息,blobs储存了网络的中间数据。
在进行推理时,首先根据net实例化一个ncnn::Extractor类,extractor中的net会被转为const类。我们可以给extractor的任意一层送入数据,如extractor.input("data", in)就是给输入数据层赋值。通过extractor.extract函数可以取出任意层的数据,在extract方法中,它会调用forward_layer方法递归地遍历网络。
我们从20类中每一类随机选取一张图片来进行测试,比较量化前的模型推理结果和量化后的模型推理结果,下图展示了每一张图片的预测类别及经过Softmax映射后的概率,其中奇数行为量化前的模型推理结果,偶数行为量化后的模型推理结果。
从图中样本的预测结果可以看出,量化前后模型的预测概率是有差异的,但是差异非常小,大多在1%以内,所选测试图片的预测结果都是正确的,说明该模型经过量化后精度没有精度损失。
下图从上到下分别展示了量化前和量化后的模型推理时间,每一张图片的推理时间是通过重复100次推理后计算出来的平均值,这是为了让推理时间的计算更加稳定。可以看出,对于大部分样本,量化前模型的推理时间约为4.4ms左右,量化后模型的推理时间约为3.7ms左右,量化后模型的推理速度提升了16%,验证了模型量化的加速效果。
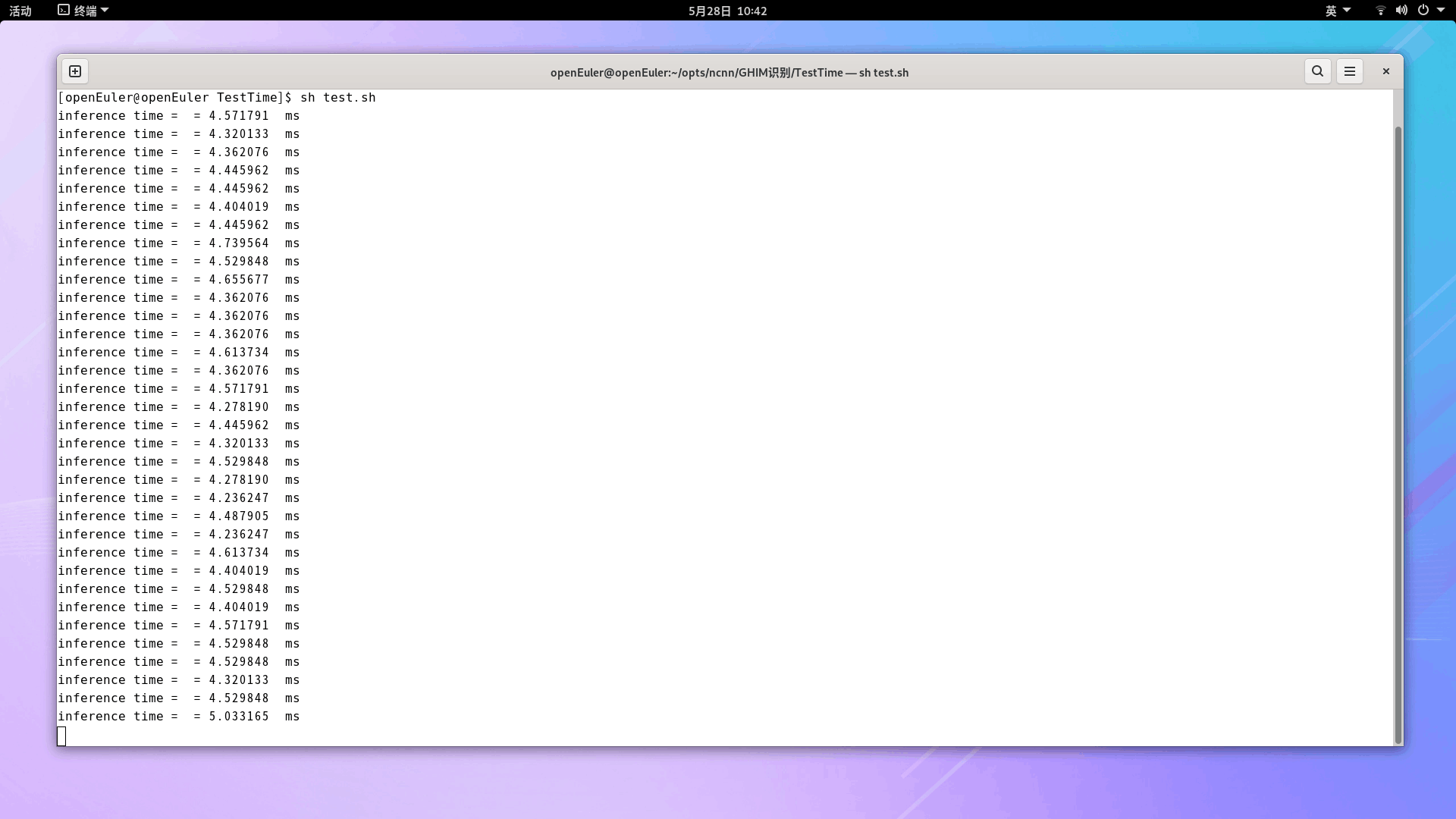
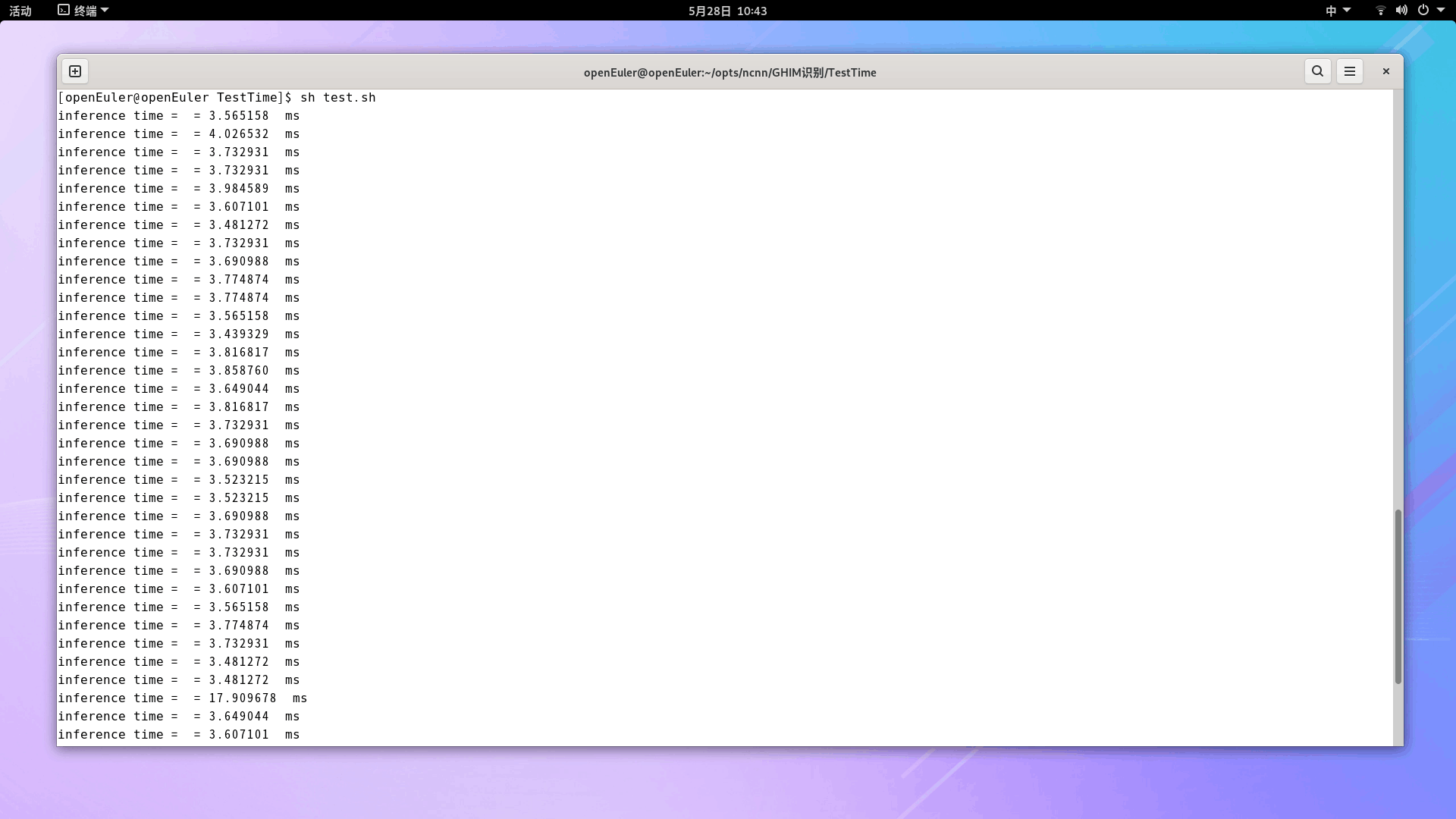
我们也用python对onnx模型以及原生pytorch模型进行了计时,计算了平均推理时间约为25ms,相关代码如下:
#coding:utf8
import torch
import torchvision
from torchsummary import summary
import time
import cv2
import sys
import onnxruntime
import numpy as np
import PIL.Image as Image
import os,glob
from simpleconv5 import simpleconv5
torch.manual_seed(0)
os.environ['CUDA_LAUNCH_BLOCKING'] = '1'# 图像预处理函数
def process_image(img):input_size = [224,224]mean = (0.5,0.5,0.5)std = (0.5,0.5,0.5)img = np.asarray(img.resize((input_size[0],input_size[1]),resample=Image.NEAREST)).astype(np.float32) / 255.0img[:,:,] -= meanimg[:,:,] /= stdimage = img.transpose((2,0,1))[np.newaxis, ...]return imageimgdir = "../GHIM-20"'''
##-----------------------test pytorch------------------------##
# 加载模型
model = simpleconv5(20)
modelpath = '../models/model_best.pth.tar'
model.load_state_dict(torch.load(modelpath,map_location='cpu')['state_dict'])
model.eval()
acc = 0.0
nums = 0.0
start_Inference = time.time()
for imgpath in glob.glob(os.path.join(imgdir, "**/*.jpg"),recursive=True):img = Image.open(imgpath).convert('RGB')data_input = process_image(img)input_data = torch.from_numpy(data_input)data_output = model(input_data)output = data_output.squeeze().cpu().detach().numpy()pred1 = output.argmax()label = int(imgpath.split('/')[-2])if label == pred1:acc += 1.0nums += 1.0end_Inference = time.time()
print('Inference use time='+str((end_Inference-start_Inference)*1000/nums)+' ms')
print("acc=",acc/nums)
'''##-----------------------test onnx------------------------##
onnx_path = '../models/simpleconv5.onnx'
result_path = 'onnx_results'
session = onnxruntime.InferenceSession(onnx_path)
inname = [input.name for input in session.get_inputs()]
outname = [output.name for output in session.get_outputs()]
acc = 0.0
nums = 0.0
print("inputs name:",inname,"|| outputs name:",outname)
start = cv2.getTickCount()
for imgpath in glob.glob(os.path.join(imgdir, "**/*.jpg"),recursive=True):img = Image.open(imgpath).convert('RGB')data_input = process_image(img)data_output = session.run(outname, {inname[0]: data_input})output = np.squeeze(data_output[0])pred1 = output.argmax()prob = output[pred1]label = int(imgpath.split('/')[-2])if label == pred1:acc += 1.0nums += 1.0
end = cv2.getTickCount()
print('ONNX Inference Time='+str((end-start)/nums/cv2.getTickFrequency()*1000)+' ms')
print("acc=",acc/nums)
可以看出,C++推理速度相比于python推理速度,有6倍左右的提升。量化后相比于量化前的提升速度并不大,这是因为香橙派本身的硬件足够出色,笔者的MacBook Pro Apple M2上的onnx推理时间也需要8ms左右。
总体来讲,香橙派的性能比我之前的EAIDK-310开发套件性能强多了,同样的模型EAIDK-310量化后的推理时间需要20ms以上,以后做嵌入式项目演示,就用香橙派 Kunpeng Pro了!