时间 | 版本 | 修改人 | 描述 |
---|---|---|---|
2024年5月14日10:44:30 | V0.1 | 宋全恒 | 新建文档 |
2024年5月14日16:28:16 | V1.0 | 宋全恒 | 填充了PyTorch对于两种量化方式的内容 |
简介
Pytorch动态量化
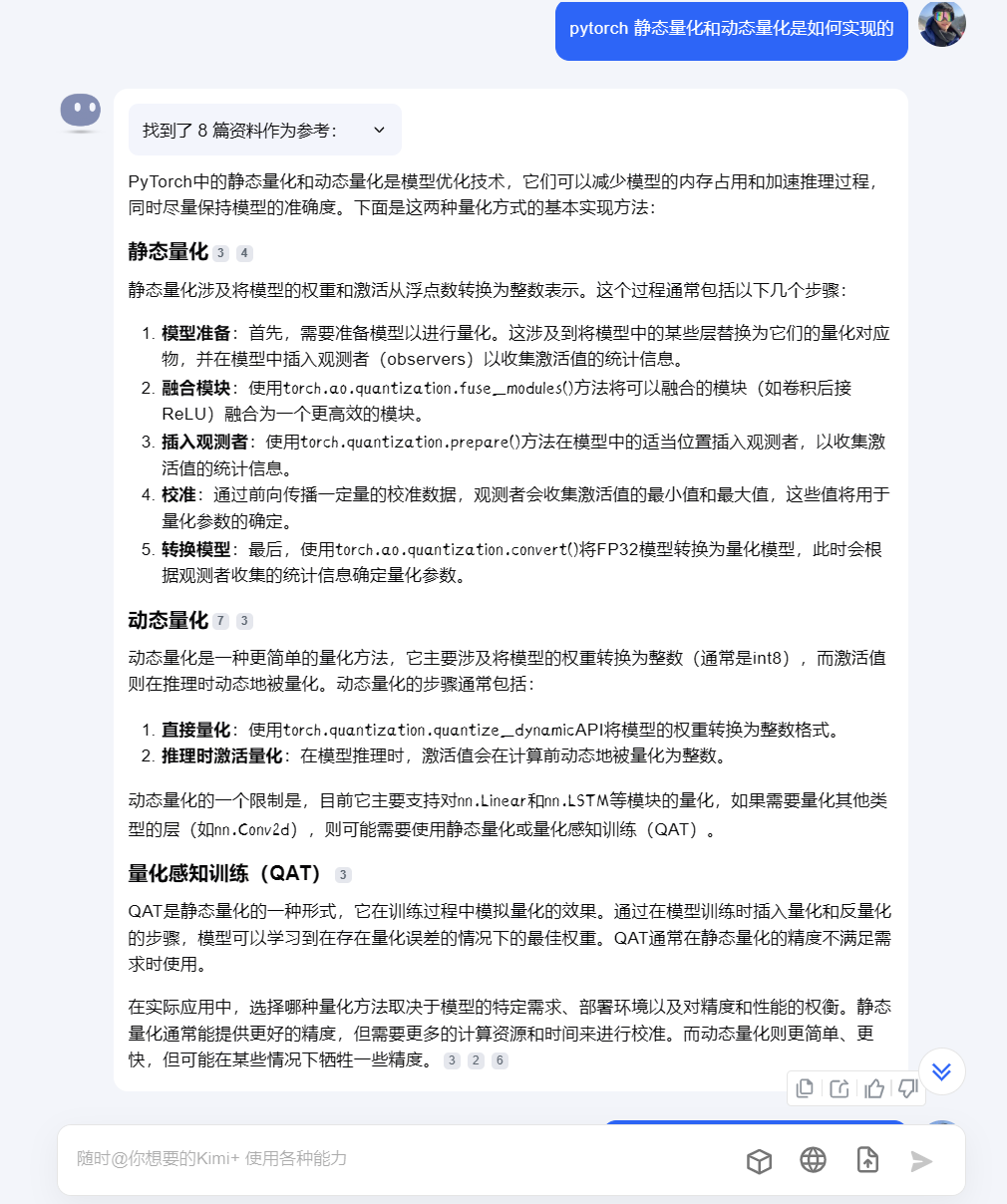
设计神经网络时,可以进行许多权衡。在模型开发和训练期间,您可以改变复发性神经网络中的层数和参数数量,并针对模型大小和/或模型延迟或吞吐量而权衡。
量化为您提供了一种方法,可以在训练完成后使用已知模型在性能和模型准确性之间进行类似的权衡。
量化
动态量化
定义
量化网络意味着将其转换为使用降低精度的整数表示来表示权重和/或激活。从浮点数转换为整数时,基本上是将浮点数乘以某个比例系数,然后将结果四舍五入为整数。
确定scale factor是各种量化方法的差异点。
动态量化的关键思想是,对于激活来说,我们将会根据运行时观察到的数据范围来确定scale factor。
这样可以确保 "调整 "比例因子,从而尽可能多地保留每个观测数据集的信号,而模型参数在模型转化过程中是已知的,他们提前转化并存储成INT8形式。
量化模型中的算术是使用矢量化 INT8 指令完成的。累加通常使用 INT16 或 INT32 完成,以避免溢出。如果下一层被量化或转换为 FP32 进行输出,则此更高精度值将缩小为 INT8。
动态量化相对不需要调整参数,这使得它非常适合作为将 LSTM 模型转换为部署的标准部分添加到生产管道中。
代码实践
# import the modules used here in this recipe
import torch
import torch.quantization
import torch.nn as nn
import copy
import os
import time# define a very, very simple LSTM for demonstration purposes
# in this case, we are wrapping ``nn.LSTM``, one layer, no preprocessing or postprocessing
# inspired by
# `Sequence Models and Long Short-Term Memory Networks tutorial <https://pytorch.org/tutorials/beginner/nlp/sequence_models_tutorial.html`_, by Robert Guthrie
# and `Dynamic Quanitzation tutorial <https://pytorch.org/tutorials/advanced/dynamic_quantization_tutorial.html>`__.
class lstm_for_demonstration(nn.Module):"""Elementary Long Short Term Memory style model which simply wraps ``nn.LSTM``Not to be used for anything other than demonstration."""def __init__(self,in_dim,out_dim,depth):super(lstm_for_demonstration,self).__init__()self.lstm = nn.LSTM(in_dim,out_dim,depth)def forward(self,inputs,hidden):out,hidden = self.lstm(inputs,hidden)return out, hiddentorch.manual_seed(29592) # set the seed for reproducibility#shape parameters
model_dimension=8
sequence_length=20
batch_size=1
lstm_depth=1# random data for input
inputs = torch.randn(sequence_length,batch_size,model_dimension)
# hidden is actually is a tuple of the initial hidden state and the initial cell state
hidden = (torch.randn(lstm_depth,batch_size,model_dimension), torch.randn(lstm_depth,batch_size,model_dimension))# here is our floating point instance
float_lstm = lstm_for_demonstration(model_dimension, model_dimension,lstm_depth)# this is the call that does the work
quantized_lstm = torch.quantization.quantize_dynamic(float_lstm, {nn.LSTM, nn.Linear}, dtype=torch.qint8
)# show the changes that were made
print('Here is the floating point version of this module:')
print(float_lstm)
print('')
print('and now the quantized version:')
print(quantized_lstm)# 上述代码,已经量化了模型
# replace the FP32 model parameters with INT8 values and some recorded scale factors.# using torch.save to store the model to os, with entitle the model name
def print_size_of_model(model, label=""):torch.save(model.state_dict(), "temp.p")size=os.path.getsize("temp.p")print("model: ",label,' \t','Size (KB):', size/1e3)os.remove('temp.p')return size# compare the sizes, the storage space is less needed
f=print_size_of_model(float_lstm,"fp32")
q=print_size_of_model(quantized_lstm,"int8")
print(f"FP32 model size is {f} times larger than INT8 model size {q}")
print("{0:.2f} times smaller".format(f/q))# quantized model is faster
# 1. Less time spent to moving parameter data in
# 2. Faster INT8 operations# compare the performance something about latency
print("Floating point FP32")float_lstm.forward(inputs, hidden)
print("Quantized INT8")
quantized_lstm.forward(inputs,hidden)# look at Accuracy# run the float model
out1, hidden1 = float_lstm(inputs, hidden)
mag1 = torch.mean(abs(out1)).item()
print('mean absolute value of output tensor values in the FP32 model is {0:.5f} '.format(mag1))# run the quantized model
out2, hidden2 = quantized_lstm(inputs, hidden)
mag2 = torch.mean(abs(out2)).item()
print('mean absolute value of output tensor values in the INT8 model is {0:.5f}'.format(mag2))# compare them
mag3 = torch.mean(abs(out1-out2)).item()
print('mean absolute value of the difference between the output tensors is {0:.5f} or {1:.2f} percent'.format(mag3,mag3/mag1*100))
代码执行结果如下所示:
(vit2) yuzailiang@ubuntu:~/vllm_test$ python lstm.py
Here is the floating point version of this module:
lstm_for_demonstration((lstm): LSTM(8, 8)
)and now the quantized version:
lstm_for_demonstration((lstm): DynamicQuantizedLSTM(8, 8)
)
(vit2) yuzailiang@ubuntu:~/vllm_test$ python lstm.py
Here is the floating point version of this module:
lstm_for_demonstration((lstm): LSTM(8, 8)
)and now the quantized version:
lstm_for_demonstration((lstm): DynamicQuantizedLSTM(8, 8)
)
model: fp32 Size (KB): 4.088
model: int8 Size (KB): 3.0
FP32 model size is 4088 times larger than INT8 model size 3000
1.36 times smaller
通过上述的代码,可以看出,量化方法提升推理速度,减少存储空间。损失的精度也不大。
(beta) Dynamic Quantization on an LSTM Word Language Model — PyTorch Tutorials 2.3.0+cu121 documentation的例子同样也展示了,使用PyTorch进行量化时,非常的方便:
import torch.quantizationquantized_model = torch.quantization.quantize_dynamic(model, {nn.LSTM, nn.Linear}, dtype=torch.qint8
)
print(quantized_model)
(beta) Dynamic Quantization on BERT — PyTorch Tutorials 2.3.0+cu121 documentation官方提供了对于Bert的量化的例子。
静态量化
(beta) Static Quantization with Eager Mode in PyTorch — PyTorch Tutorials 2.3.0+cu121 documentation提供了关于静态量化的官方演示。
定义
训练后静态量化不仅涉及将权重从浮点转换为整数(如动态量化中那样),还涉及执行额外步骤,即首先通过网络喂给批量数据并计算不同激活的结果分布(具体来说,这是通过在记录此数据的不同点插入observer来完成)。然后,使用这些分布来确定在推理时应如何具体量化不同的激活(一种简单的技术是将整个激活范围简单地分为 256 个级别,但我们也支持更复杂的方法)。重要的是,这个额外的步骤允许我们在操作之间传递量化值,而不是在每个操作之间将这些值转换为浮点数,然后再转换回整数,从而显着提高速度。
代码演示
- 定义模型架构,并获取精确性的baseline, 71.9%
- 提供校准数据,观察激活分布,确定scale factor
线图演示了步骤2的具体过程。
num_calibration_batches = 32myModel = load_model(saved_model_dir + float_model_file).to('cpu')
myModel.eval()# Fuse Conv, bn and relu
myModel.fuse_model()# Specify quantization configuration
# Start with simple min/max range estimation and per-tensor quantization of weights
myModel.qconfig = torch.ao.quantization.default_qconfig
print(myModel.qconfig)
torch.ao.quantization.prepare(myModel, inplace=True)# Calibrate first
print('Post Training Quantization Prepare: Inserting Observers')
print('\n Inverted Residual Block:After observer insertion \n\n', myModel.features[1].conv)# Calibrate with the training set
evaluate(myModel, criterion, data_loader, neval_batches=num_calibration_batches)
print('Post Training Quantization: Calibration done')# Convert to quantized model
torch.ao.quantization.convert(myModel, inplace=True)
# You may see a user warning about needing to calibrate the model. This warning can be safely ignored.
# This warning occurs because not all modules are run in each model runs, so some
# modules may not be calibrated.
print('Post Training Quantization: Convert done')
print('\n Inverted Residual Block: After fusion and quantization, note fused modules: \n\n',myModel.features[1].conv)print("Size of model after quantization")
print_size_of_model(myModel)top1, top5 = evaluate(myModel, criterion, data_loader_test, neval_batches=num_eval_batches)
print('Evaluation accuracy on %d images, %2.2f'%(num_eval_batches * eval_batch_size, top1.avg))
经过量化之后,在eval数据集上的精确性为56.7%,这是因为使用了一个简单的额min/max观察者来确定量化参数。模型体积缩小接近了 4倍。
可以进一步使用如下的建议配置来优化量化过程:
- 基于每个通道量化权重
- 使用直方图观察器收集激活直方图,然后以最佳方式选择量化参数。
per_channel_quantized_model = load_model(saved_model_dir + float_model_file)
per_channel_quantized_model.eval()
per_channel_quantized_model.fuse_model()
# The old 'fbgemm' is still available but 'x86' is the recommended default.
per_channel_quantized_model.qconfig = torch.ao.quantization.get_default_qconfig('x86')
print(per_channel_quantized_model.qconfig)torch.ao.quantization.prepare(per_channel_quantized_model, inplace=True)
evaluate(per_channel_quantized_model,criterion, data_loader, num_calibration_batches)
torch.ao.quantization.convert(per_channel_quantized_model, inplace=True)
top1, top5 = evaluate(per_channel_quantized_model, criterion, data_loader_test, neval_batches=num_eval_batches)
print('Evaluation accuracy on %d images, %2.2f'%(num_eval_batches * eval_batch_size, top1.avg))
torch.jit.save(torch.jit.script(per_channel_quantized_model), saved_model_dir + scripted_quantized_model_file)
仅更改此量化配置方法即可将准确度提高到 67. 3% 以上!尽管如此,这仍比上述 71. 9% 的基线差了 4%。因此,让我们尝试量化感知训练。
总结
本文主要是探讨了PyTorch对于静态量化和动态量化的支持。并且演示了PyTorch对于静态量化和动态量化的支持。两者显著的区别是PTQ静态量化需要一批校准数据,有了校准数据之后,会直接对权重和激活进行量化。而动态量化,是仅仅权重的量化,输入和激活则是在运行时进行量化,实现的方式是通过插入observer来实现的。
在 PyTorch 中,Observer模块收集输入值的统计信息并计算scale和zero_point。
参考
网页 | 描述 |
---|---|
Dynamic Quantization — PyTorch Tutorials 2.3.0+cu121 documentation | 动态量化,提供了代码示例LSTM量化 |
(beta) Dynamic Quantization on an LSTM Word Language Model — PyTorch Tutorials 2.3.0+cu121 documentation | 👍👍高级的动态量tutorial量化涉及将模型的权重和激活值从浮点数转换为整数,这样可以缩小模型大小,加快推理速度,但对准确性的影响很小。提供了一个较为复杂的例子。 |
(beta) Static Quantization with Eager Mode in PyTorch — PyTorch Tutorials 2.3.0+cu121 documentation | 静态量化,需要校准数据,来观察数据分布。 |
详解pytorch动态量化-CSDN博客 | 基于代码阐述了动态量化的执行过程。 Post Training Dynamic Quantization,简称为 Dynamic Quantization,也就是动态量化,或者叫作Weight-only的量化,是提前把模型中某些 op 的参数量化为 INT8,然后在运行的时候动态的把输入量化为 INT8,然后在当前 op 输出的时候再把结果 requantization 回到 float32 类型 。动态量化默认只适用于 Linear 以及 RNN 的变种。 |