前言
Indexing(索引)是搭建任何RAG系统的第一步,也是至关重要的一步,良好的索引意味着合理的知识或信息分类,召回环节就会更加精准。在这篇文章中,围绕Indexing(索引)环节,如下图蓝色部分所示,详细介绍一下如何对输入文档构建合理的索引。
在实际应用场景中,文档尺寸可能非常大,因此需要将长篇文档分割成多个文本块,以便更高效地处理和检索信息。
Indexing(索引)环节主要面临三个难题:
-
首先,内容表述不完整,内容块的语义信息容易受分割方式影响,致使在较长的语境中,重要信息被丢失或被掩盖。
-
其次,块相似性搜索不准确,随着数据量增多,检索中的噪声增大,导致频繁与错误数据匹配,使得检索系统脆弱且不可靠。
-
最后,参考轨迹不明晰,检索到的内容块可能来自任何文档,没有引用痕迹,可能出现来自多个不同文档的块,尽管语义相似,但包含的却是完全不同主题的内容。
下面,我们结合源代码,介绍Chunk optimization(块优化)、Multi-representation indexing(多层表达索引)、Specialized embeddings(特殊嵌入)和Hierachical Indexing(多级索引)这四种优化索引的高级方法。
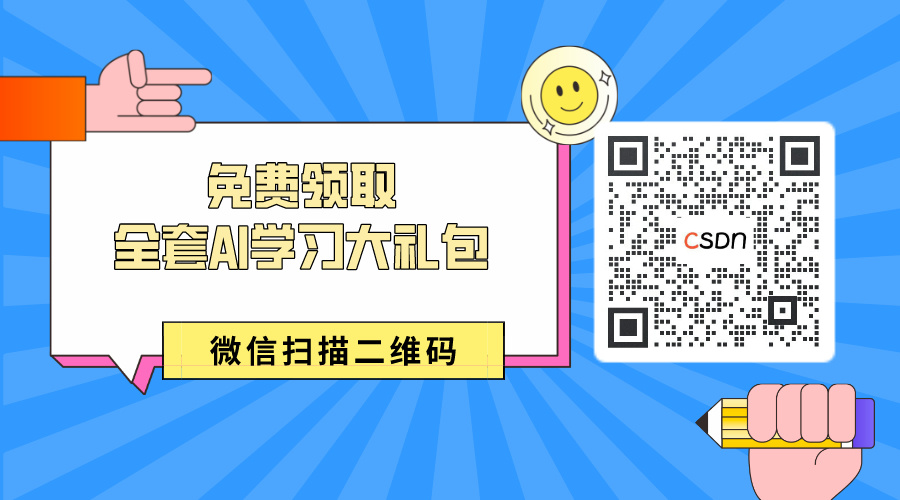
1. Chunk optimization(块优化)
在内容分块的时候,分块大小对索引结果会有很大的影响。较大的块能捕捉更多的上下文,但也会产生更多噪声,需要更长的处理时间和更高的成本;而较小的块噪声更小,但可能无法完整传达必要的上下文。
第一种优化方式:固定大小重叠滑动窗口
该方法根据字符数将文本划分为固定大小的块,实现简单。但是其局限性包括对上下文大小的控制不精确、存在切断单词或句子的风险以及缺乏语义考虑。适用于探索性分析,但不推荐用于需要深度语义理解的任务。
text = "..." # your text
from langchain.text_splitter import CharacterTextSplitter
text_splitter = CharacterTextSplitter(chunk_size = 256,chunk_overlap = 20
)
docs = text_splitter.create_documents([text])
第二种优化方式:递归感知
一种结合固定大小滑动窗口和结构感知分割的混合方法。它试图平衡固定块大小和语言边界,提供精确的上下文控制。实现复杂度较高,存在块大小可变的风险,对于需要粒度和语义完整性的任务有效,但不推荐用于快速任务或结构划分不明确的任务。
text = "..." # your text
from langchain.text_splitter import RecursiveCharacterTextSplitter
text_splitter = RecursiveCharacterTextSplitter(chunk_size = 256,chunk_overlap = 20,separators = ["\n\n", "\n"]
)docs = text_splitter.create_documents([text])
第三种优化方式:结构感知切分
该方法考虑文本的自然结构,根据句子、段落、节或章对其进行划分。尊重语言边界可以保持语义完整性,但结构复杂性的变化会带来挑战。对于需要上下文和语义的任务有效,但不适用于缺乏明确结构划分的文本
text = "..." # your text
docs = text.split(".")
第四种优化方式:内容感知切分
此方法侧重于内容类型和结构,尤其是在 Markdown、LaTeX 或 HTML 等结构化文档中。它确保内容类型不会在块内混合,从而保持完整性。挑战包括理解特定语法和不适用于非结构化文档。适用于结构化文档,但不推荐用于非结构化内容。以markdown为例
from langchain.text_splitter import MarkdownTextSplitter
markdown_text = "..."markdown_splitter = MarkdownTextSplitter(chunk_size=100, chunk_overlap=0)
docs = markdown_splitter.create_documents([markdown_text])
第五种块优化方式:基于语义切分
一种基于语义理解的复杂方法,通过检测主题的重大转变将文本划分为块。确保语义一致性,但需要高级 NLP 技术。对于需要语义上下文和主题连续性的任务有效,但不适合高主题重叠或简单的分块任务
text = "..." # your text
from langchain.text_splitter import NLTKTextSplitter
text_splitter = NLTKTextSplitter()
docs = text_splitter.split_text(text)
2. 多层表达索引
多层表达索引是一种构建多级索引的方法,在长上下文环境比较有用。
这种方法通过将原始数据生成 summary后,重新作为embedding再存到summary database中。 检索的时候,首先通过summary database找到最相关的summary,再回溯到原始文档中去。
首先,我们使用 WebBaseLoader 加载两个网页的文档,在这个例子中,我们加载了 Lilian Weng 的两篇博客文章:
from langchain_community.document_loaders import WebBaseLoader
from langchain_text_splitters import RecursiveCharacterTextSplitterloader = WebBaseLoader("https://lilianweng.github.io/posts/2023-06-23-agent/")
docs = loader.load()loader = WebBaseLoader("https://lilianweng.github.io/posts/2024-02-05-human-data-quality/")
docs.extend(loader.load())
模型使用 ChatOpenAI,设置为 gpt-3.5-turbo 版本,利用 chain.batch 批量处理文档,使用 max_concurrency 参数限制并发数。
import uuid
from langchain_core.documents import Document
from langchain_core.output_parsers import StrOutputParser
from langchain_core.prompts import ChatPromptTemplate
from langchain_openai import ChatOpenAIchain = ({"doc": lambda x: x.page_content}| ChatPromptTemplate.from_template("Summarize the following document:\n\n{doc}")| ChatOpenAI(model="gpt-3.5-turbo",max_retries=0)| StrOutputParser()
)summaries = chain.batch(docs, {"max_concurrency": 5})
我们引入了 InMemoryByteStore 和 Chroma 两个模块,分别用于存储原始文档和总结文档。InMemoryByteStore 是一个内存中的存储层,用于存储原始文档,而 Chroma 则是一个文档向量数据库,用于存储文档的向量表示。
from langchain.storage import InMemoryByteStore
from langchain_openai import OpenAIEmbeddings
from langchain_community.vectorstores import Chroma
from langchain.retrievers.multi_vector import MultiVectorRetriever# The vectorstore to use to index the child chunks
vectorstore = Chroma(collection_name="summaries",embedding_function=OpenAIEmbeddings())# The storage layer for the parent documents
store = InMemoryByteStore()
MultiVectorRetriever 类帮助我们在一个统一的接口中管理文档和向量存储,使得检索过程更加高效。
id_key = "doc_id"# The retriever
retriever = MultiVectorRetriever(vectorstore=vectorstore,byte_store=store,id_key=id_key,
)
doc_ids = [str(uuid.uuid4()) for _ in docs]
将总结文档添加到 Chroma 向量数据库中,同时在 InMemoryByteStore 中关联原始文档和 doc_id。
summary_docs = [Document(page_content=s, metadata={id_key: doc_ids[i]})for i, s in enumerate(summaries)
]# Add
retriever.vectorstore.add_documents(summary_docs)
retriever.docstore.mset(list(zip(doc_ids, docs)))
执行检索操作,对于给定的查询 query = “Memory in agents”,我们使用 vectorstore 进行相似性检索,k=1 表示只返回最相关的一个文档。然后使用 retriever 进行检索,n_results=1 表示只返回一个文档结果。
query = "Memory in agents"
sub_docs = vectorstore.similarity_search(query,k=1)
# 打印 sub_docs[0] retrieved_docs = retriever.get_relevant_documents(query,n_results=1)
# 打印 retrieved_docs[0].page_content[0:500]
3. 特殊向量
特殊向量方法常用于多模态数据,比如图片数据,利用特殊的向量去做索引。
ColBERT是一种常用的特殊向量方法,它为段落中的每个标记生成一个受上下文影响的向量,同时也会为查询中的每个标记生成向量。然后,每个文档的得分是每个查询嵌入与任何文档嵌入的最大相似度之和。
可以使用RAGatouille工具来快速实现ColBERT,首先引入RAGatouille。
from ragatouille import RAGPretrainedModel
RAG = RAGPretrainedModel.from_pretrained("colbert-ir/colbertv2.0")
然后我们获取文档数据,这里我们选择了使用wiki页面
import requestsdef get_wikipedia_page(title: str):"""Retrieve the full text content of a Wikipedia page.:param title: str - Title of the Wikipedia page.:return: str - Full text content of the page as raw string."""# Wikipedia API endpointURL = "https://en.wikipedia.org/w/api.php"# Parameters for the API requestparams = {"action": "query","format": "json","titles": title,"prop": "extracts","explaintext": True,}# Custom User-Agent header to comply with Wikipedia's best practicesheaders = {"User-Agent": "RAGatouille_tutorial/0.0.1 (ben@clavie.eu)"}response = requests.get(URL, params=params, headers=headers)data = response.json()# Extracting page contentpage = next(iter(data["query"]["pages"].values()))return page["extract"] if "extract" in page else Nonefull_document = get_wikipedia_page("Hayao_Miyazaki")
最后,完成索引的构建,自动使用ColBERT方法完成索引。
RAG.index(collection=[full_document],index_name="Miyazaki-123",max_document_length=180,split_documents=True,
)
4. 分层索引
分层索引,指的是带层级结构的去索引,比如可以先从关系数据库里索引找出对应的关系,然后再利用索引出的关系再进一步去搜寻basic数据库。 前文介绍的多层表达索引也属于分层索引的一种。
还有一种更有效的分层索引方法叫做Raptor,Recursive Abstractive Processing for Tree-Organized Retrieval,该方法核心思想是将doc构建为一棵树,然后逐层递归的查询,如下图所示:
RAPTOR 根据向量递归地对文本块进行聚类,并生成这些聚类的文本摘要,从而自下而上构建一棵树。聚集在一起的节点是兄弟节点;父节点包含该集群的文本摘要。这种结构使 RAPTOR 能够将代表不同级别文本的上下文块加载到 LLM 的上下文中,以便它能够有效且高效地回答不同层面的问题。
查询有两种方法,基于树遍历(tree traversal)和折叠树(collapsed tree)。遍历是从 RAPTOR 树的根层开始,然后逐层查询;折叠树就是全部平铺,用ANN库查询。
首先,我们使用 LangChain 的 LCEL 文档作为输入数据
import matplotlib.pyplot as plt
import tiktoken
from bs4 import BeautifulSoup as Soup
from langchain_community.document_loaders.recursive_url_loader import RecursiveUrlLoader## Helper Fuction to count the number of Tokensin each text
def num_tokens_from_string(string: str, encoding_name: str) -> int:"""Returns the number of tokens in a text string."""encoding = tiktoken.get_encoding(encoding_name)num_tokens = len(encoding.encode(string))return num_tokens# LCEL docs
url = "https://python.langchain.com/docs/expression_language/"
loader = RecursiveUrlLoader(url=url, max_depth=20, extractor=lambda x: Soup(x, "html.parser").text
)
docs = loader.load()# LCEL w/ PydanticOutputParser (outside the primary LCEL docs)
url = "https://python.langchain.com/docs/modules/model_io/output_parsers/quick_start"
loader = RecursiveUrlLoader(url=url, max_depth=1, extractor=lambda x: Soup(x, "html.parser").text
)
docs_pydantic = loader.load()# LCEL w/ Self Query (outside the primary LCEL docs)
url = "https://python.langchain.com/docs/modules/data_connection/retrievers/self_query/"
loader = RecursiveUrlLoader(url=url, max_depth=1, extractor=lambda x: Soup(x, "html.parser").text
)
docs_sq = loader.load()# Doc texts
docs.extend([*docs_pydantic, *docs_sq])
docs_texts = [d.page_content for d in docs]
对文档进行分块以适合我们的 LLM 上下文窗口。
# Doc texts split
from langchain_text_splitters import RecursiveCharacterTextSplitterchunk_size_tok = 1000
text_splitter = RecursiveCharacterTextSplitter.from_tiktoken_encoder(chunk_size=chunk_size_tok, chunk_overlap=0
)
texts_split = text_splitter.split_text(concatenated_content)
#
print(f"Number of text splits generated: {len(texts_split)}")
生成全局嵌入列表,并将维度减少到2来简化生成的聚类,并可视化
global_embeddings = [embd.embed_query(txt) for txt in texts_split]
print ( len (global_embeddings[ 0 ])import matplotlib.pyplot as plt
from typing import Optional
import numpy as np
import umapdef reduce_cluster_embeddings(embeddings: np.ndarray,dim: int,n_neighbors: Optional[int] = None,metric: str = "cosine",
) -> np.ndarray:if n_neighbors is None:n_neighbors = int((len(embeddings) - 1) ** 0.5)return umap.UMAP(n_neighbors=n_neighbors, n_components=dim, metric=metric).fit_transform(embeddings)dim = 2
global_embeddings_reduced = reduce_cluster_embeddings(global_embeddings, dim)
print(global_embeddings_reduced[0])
然后,为每个Raptor步骤定义辅助函数,并构建树。这一段代码是整个Raptor中最复杂的一段,其主要做了以下事情:
-
global_cluster_embeddings使用UAMP算法对所有的Embeddings进行全局降维,local_cluster_embeddings则使用UAMP算法进行局部降维。
-
get_optimal_clusters函数使用高斯混合模型的贝叶斯信息准则 (BIC) 确定最佳聚类数。
-
GMM_cluster函数使用基于概率阈值的高斯混合模型 (GMM) 进行聚类嵌入,返回包含聚类标签和确定的聚类数量的元组。
-
Perform_clustering函数则对嵌入执行聚类,首先全局降低其维数,然后使用高斯混合模型进行聚类,最后在每个全局聚类内执行局部聚类。
-
Embed_cluster_texts函数则用于嵌入文本列表并对其进行聚类,返回包含文本、其嵌入和聚类标签的 DataFrame。
-
Embed_cluster_summarize_texts函数首先为文本生成嵌入,根据相似性对它们进行聚类,扩展聚类分配以便于处理,然后汇总每个聚类内的内容。
-
recursive_embed_cluster_summarize函数递归地嵌入、聚类和汇总文本,直至指定级别或直到唯一聚类的数量变为 1,并在每个级别存储结果。
RANDOM_SEED = 224 # Fixed seed for reproducibilitydef global_cluster_embeddings(embeddings: np.ndarray,dim: int,n_neighbors: Optional[int] = None,metric: str = "cosine",
) -> np.ndarray:"""Perform global dimensionality reduction on the embeddings using UMAP.Parameters:- embeddings: The input embeddings as a numpy array.- dim: The target dimensionality for the reduced space.- n_neighbors: Optional; the number of neighbors to consider for each point.If not provided, it defaults to the square root of the number of embeddings.- metric: The distance metric to use for UMAP.Returns:- A numpy array of the embeddings reduced to the specified dimensionality."""if n_neighbors is None:n_neighbors = int((len(embeddings) - 1) ** 0.5)return umap.UMAP(n_neighbors=n_neighbors, n_components=dim, metric=metric).fit_transform(embeddings)def local_cluster_embeddings(embeddings: np.ndarray, dim: int, num_neighbors: int = 10, metric: str = "cosine"
) -> np.ndarray:"""Perform local dimensionality reduction on the embeddings using UMAP, typically after global clustering.Parameters:- embeddings: The input embeddings as a numpy array.- dim: The target dimensionality for the reduced space.- num_neighbors: The number of neighbors to consider for each point.- metric: The distance metric to use for UMAP.Returns:- A numpy array of the embeddings reduced to the specified dimensionality."""return umap.UMAP(n_neighbors=num_neighbors, n_components=dim, metric=metric).fit_transform(embeddings)def get_optimal_clusters(embeddings: np.ndarray, max_clusters: int = 50, random_state: int = RANDOM_SEED
) -> int:"""Determine the optimal number of clusters using the Bayesian Information Criterion (BIC) with a Gaussian Mixture Model.Parameters:- embeddings: The input embeddings as a numpy array.- max_clusters: The maximum number of clusters to consider.- random_state: Seed for reproducibility.Returns:- An integer representing the optimal number of clusters found."""max_clusters = min(max_clusters, len(embeddings))n_clusters = np.arange(1, max_clusters)bics = []for n in n_clusters:gm = GaussianMixture(n_components=n, random_state=random_state)gm.fit(embeddings)bics.append(gm.bic(embeddings))return n_clusters[np.argmin(bics)]def GMM_cluster(embeddings: np.ndarray, threshold: float, random_state: int = 0):"""Cluster embeddings using a Gaussian Mixture Model (GMM) based on a probability threshold.Parameters:- embeddings: The input embeddings as a numpy array.- threshold: The probability threshold for assigning an embedding to a cluster.- random_state: Seed for reproducibility.Returns:- A tuple containing the cluster labels and the number of clusters determined."""n_clusters = get_optimal_clusters(embeddings)gm = GaussianMixture(n_components=n_clusters, random_state=random_state)gm.fit(embeddings)probs = gm.predict_proba(embeddings)labels = [np.where(prob > threshold)[0] for prob in probs]return labels, n_clustersdef perform_clustering(embeddings: np.ndarray,dim: int,threshold: float,
) -> List[np.ndarray]:"""Perform clustering on the embeddings by first reducing their dimensionality globally, then clusteringusing a Gaussian Mixture Model, and finally performing local clustering within each global cluster.Parameters:- embeddings: The input embeddings as a numpy array.- dim: The target dimensionality for UMAP reduction.- threshold: The probability threshold for assigning an embedding to a cluster in GMM.Returns:- A list of numpy arrays, where each array contains the cluster IDs for each embedding."""if len(embeddings) <= dim + 1:# Avoid clustering when there's insufficient datareturn [np.array([0]) for _ in range(len(embeddings))]# Global dimensionality reductionreduced_embeddings_global = global_cluster_embeddings(embeddings, dim)# Global clusteringglobal_clusters, n_global_clusters = GMM_cluster(reduced_embeddings_global, threshold)all_local_clusters = [np.array([]) for _ in range(len(embeddings))]total_clusters = 0# Iterate through each global cluster to perform local clusteringfor i in range(n_global_clusters):# Extract embeddings belonging to the current global clusterglobal_cluster_embeddings_ = embeddings[np.array([i in gc for gc in global_clusters])]if len(global_cluster_embeddings_) == 0:continueif len(global_cluster_embeddings_) <= dim + 1:# Handle small clusters with direct assignmentlocal_clusters = [np.array([0]) for _ in global_cluster_embeddings_]n_local_clusters = 1else:# Local dimensionality reduction and clusteringreduced_embeddings_local = local_cluster_embeddings(global_cluster_embeddings_, dim)local_clusters, n_local_clusters = GMM_cluster(reduced_embeddings_local, threshold)# Assign local cluster IDs, adjusting for total clusters already processedfor j in range(n_local_clusters):local_cluster_embeddings_ = global_cluster_embeddings_[np.array([j in lc for lc in local_clusters])]indices = np.where((embeddings == local_cluster_embeddings_[:, None]).all(-1))[1]for idx in indices:all_local_clusters[idx] = np.append(all_local_clusters[idx], j + total_clusters)total_clusters += n_local_clustersreturn all_local_clusters### --- Our code below --- ###def embed(texts):"""Generate embeddings for a list of text documents.This function assumes the existence of an `embd` object with a method `embed_documents`that takes a list of texts and returns their embeddings.Parameters:- texts: List[str], a list of text documents to be embedded.Returns:- numpy.ndarray: An array of embeddings for the given text documents."""text_embeddings = embd.embed_documents(texts)text_embeddings_np = np.array(text_embeddings)return text_embeddings_npdef embed_cluster_texts(texts):"""Embeds a list of texts and clusters them, returning a DataFrame with texts, their embeddings, and cluster labels.This function combines embedding generation and clustering into a single step. It assumes the existenceof a previously defined `perform_clustering` function that performs clustering on the embeddings.Parameters:- texts: List[str], a list of text documents to be processed.Returns:- pandas.DataFrame: A DataFrame containing the original texts, their embeddings, and the assigned cluster labels."""text_embeddings_np = embed(texts) # Generate embeddingscluster_labels = perform_clustering(text_embeddings_np, 10, 0.1) # Perform clustering on the embeddingsdf = pd.DataFrame() # Initialize a DataFrame to store the resultsdf["text"] = texts # Store original textsdf["embd"] = list(text_embeddings_np) # Store embeddings as a list in the DataFramedf["cluster"] = cluster_labels # Store cluster labelsreturn dfdef fmt_txt(df: pd.DataFrame) -> str:"""Formats the text documents in a DataFrame into a single string.Parameters:- df: DataFrame containing the 'text' column with text documents to format.Returns:- A single string where all text documents are joined by a specific delimiter."""unique_txt = df["text"].tolist()return "--- --- \n --- --- ".join(unique_txt)def embed_cluster_summarize_texts(texts: List[str], level: int
) -> Tuple[pd.DataFrame, pd.DataFrame]:"""Embeds, clusters, and summarizes a list of texts. This function first generates embeddings for the texts,clusters them based on similarity, expands the cluster assignments for easier processing, and then summarizesthe content within each cluster.Parameters:- texts: A list of text documents to be processed.- level: An integer parameter that could define the depth or detail of processing.Returns:- Tuple containing two DataFrames:1. The first DataFrame (`df_clusters`) includes the original texts, their embeddings, and cluster assignments.2. The second DataFrame (`df_summary`) contains summaries for each cluster, the specified level of detail,and the cluster identifiers."""# Embed and cluster the texts, resulting in a DataFrame with 'text', 'embd', and 'cluster' columnsdf_clusters = embed_cluster_texts(texts)# Prepare to expand the DataFrame for easier manipulation of clustersexpanded_list = []# Expand DataFrame entries to document-cluster pairings for straightforward processingfor index, row in df_clusters.iterrows():for cluster in row["cluster"]:expanded_list.append({"text": row["text"], "embd": row["embd"], "cluster": cluster})# Create a new DataFrame from the expanded listexpanded_df = pd.DataFrame(expanded_list)# Retrieve unique cluster identifiers for processingall_clusters = expanded_df["cluster"].unique()print(f"--Generated {len(all_clusters)} clusters--")# Summarizationtemplate = """Here is a sub-set of LangChain Expression Langauge doc.LangChain Expression Langauge provides a way to compose chain in LangChain.Give a detailed summary of the documentation provided.Documentation:{context}"""prompt = ChatPromptTemplate.from_template(template)chain = prompt | model | StrOutputParser()# Format text within each cluster for summarizationsummaries = []for i in all_clusters:df_cluster = expanded_df[expanded_df["cluster"] == i]formatted_txt = fmt_txt(df_cluster)summaries.append(chain.invoke({"context": formatted_txt}))# Create a DataFrame to store summaries with their corresponding cluster and leveldf_summary = pd.DataFrame({"summaries": summaries,"level": [level] * len(summaries),"cluster": list(all_clusters),})return df_clusters, df_summarydef recursive_embed_cluster_summarize(texts: List[str], level: int = 1, n_levels: int = 3
) -> Dict[int, Tuple[pd.DataFrame, pd.DataFrame]]:"""Recursively embeds, clusters, and summarizes texts up to a specified level or untilthe number of unique clusters becomes 1, storing the results at each level.Parameters:- texts: List[str], texts to be processed.- level: int, current recursion level (starts at 1).- n_levels: int, maximum depth of recursion.Returns:- Dict[int, Tuple[pd.DataFrame, pd.DataFrame]], a dictionary where keys are the recursionlevels and values are tuples containing the clusters DataFrame and summaries DataFrame at that level."""results = {} # Dictionary to store results at each level# Perform embedding, clustering, and summarization for the current leveldf_clusters, df_summary = embed_cluster_summarize_texts(texts, level)# Store the results of the current levelresults[level] = (df_clusters, df_summary)# Determine if further recursion is possible and meaningfulunique_clusters = df_summary["cluster"].nunique()if level < n_levels and unique_clusters > 1:# Use summaries as the input texts for the next level of recursionnew_texts = df_summary["summaries"].tolist()next_level_results = recursive_embed_cluster_summarize(new_texts, level + 1, n_levels)# Merge the results from the next level into the current results dictionaryresults.update(next_level_results)return results# Build tree
leaf_texts = docs_texts
results = recursive_embed_cluster_summarize(leaf_texts, level=1, n_levels=3)
接下来,生成最终摘要,有两种方法:
-
树遍历检索:树的遍历从树的根级开始,并根据向量嵌入的余弦相似度检索节点的前 k 个文档。因此,在每一级,它都会从子节点检索前 k 个文档。
-
折叠树检索:折叠树检索是一种更简单的方法。它将所有树折叠成一层,并根据查询向量的余弦相似度检索节点,直到达到阈值数量的标记。
我们将提取数据框文本、聚类文本、最终摘要文本,并将它们组合起来,创建一个包含根文档和摘要的大型文本列表。然后将该文本存储到向量存储中。
# Initialize all_texts with leaf_texts
all_texts = leaf_texts.copy()# Iterate through the results to extract summaries from each level and add them to all_texts
for level in sorted(results.keys()):# Extract summaries from the current level's DataFramesummaries = results[level][1]["summaries"].tolist()# Extend all_texts with the summaries from the current levelall_texts.extend(summaries)#Final Summaries extracted
print(all_texts)
将文本加载到 vectorstore 中,构建索引,并创建查询引擎
# Now, use all_texts to build the vectorstore with Chroma
vectorstore = Chroma.from_texts(texts=all_texts, embedding=embd)
retriever = vectorstore.as_retriever()from langchain import hub
from langchain_core.runnables import RunnablePassthrough
# Prompt
prompt = hub.pull("rlm/rag-prompt")
# Post-processing
def format_docs(docs):return "\n\n".join(doc.page_content for doc in docs)
# Chain
rag_chain = ({"context": retriever | format_docs, "question": RunnablePassthrough()}| prompt| model| StrOutputParser()
)
最后,用一个实际问题进行检验,可以看到实际的回复内容还是比较准确的。
# Question
response =rag_chain.invoke("What is LCEL?")
print(str(response))############# Response ######################################LangChain Expression Language (LCEL) is a declarative way to easily compose chains together in LangChain. It was designed from day 1 to support putting prototypes in production with no code changes, from the simplest "prompt + LLM" chain to complex chains with hundreds of steps. Some reasons why one might want to use LCEL include streaming support (allowing for the best possible time-to-first-token), async support (enabling use in both synchronous and asynchronous APIs), optimized parallel execution (automatically executing parallel steps with the smallest possible latency), retries and fallbacks (a great way to make chains more reliable at scale), access to intermediate results (useful for letting end-users know something is happening or debugging), input and output schemas (providing Pydantic and JSONSchema schemas inferred from chain structure for validation), seamless LangSmith tracing integration (maximum observability and debuggability), and seamless LangServe deployment integration (easy chain deployment).
到这里,优化索引的四种高级方法就介绍完了。
总结
在这篇文章中,风叔详细介绍了优化Indexing(索引)的具体方法,包括Chunk optimization(块优化)、Multi-representation indexing(多层表达索引)、Specialized embeddings(特殊嵌入)和Hierachical Indexing(多级索引)这四种优化方案。
最后的最后
感谢你们的阅读和喜欢,我收藏了很多技术干货,可以共享给喜欢我文章的朋友们,如果你肯花时间沉下心去学习,它们一定能帮到你。
因为这个行业不同于其他行业,知识体系实在是过于庞大,知识更新也非常快。作为一个普通人,无法全部学完,所以我们在提升技术的时候,首先需要明确一个目标,然后制定好完整的计划,同时找到好的学习方法,这样才能更快的提升自己。
这份完整版的大模型 AI 学习资料已经上传CSDN,朋友们如果需要可以微信扫描下方CSDN官方认证二维码免费领取【保证100%免费
】
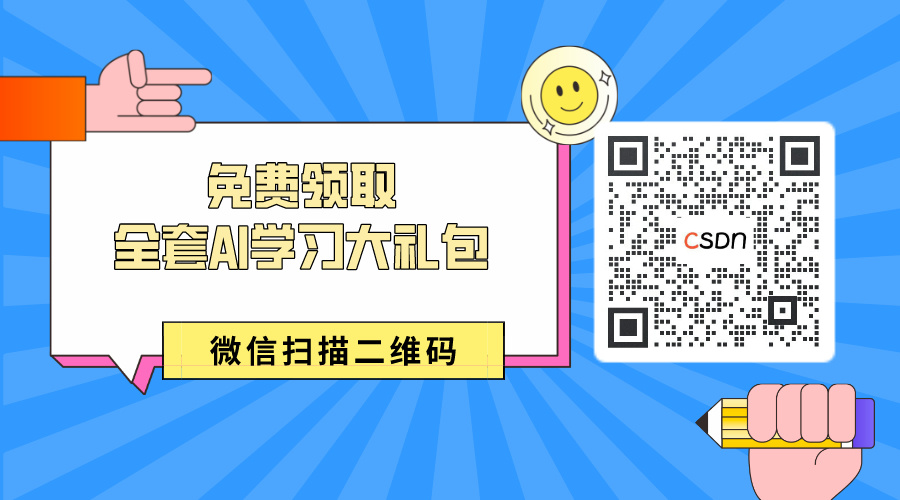
大模型知识脑图
为了成为更好的 AI大模型 开发者,这里为大家提供了总的路线图。它的用处就在于,你可以按照上面的知识点去找对应的学习资源,保证自己学得较为全面。
经典书籍阅读
阅读AI大模型经典书籍可以帮助读者提高技术水平,开拓视野,掌握核心技术,提高解决问题的能力,同时也可以借鉴他人的经验。对于想要深入学习AI大模型开发的读者来说,阅读经典书籍是非常有必要的。
实战案例
光学理论是没用的,要学会跟着一起敲,要动手实操,才能将自己的所学运用到实际当中去,这时候可以搞点实战案例来学习。
面试资料
我们学习AI大模型必然是想找到高薪的工作,下面这些面试题都是总结当前最新、最热、最高频的面试题,并且每道题都有详细的答案,面试前刷完这套面试题资料,小小offer,不在话下
640套AI大模型报告合集
这套包含640份报告的合集,涵盖了AI大模型的理论研究、技术实现、行业应用等多个方面。无论您是科研人员、工程师,还是对AI大模型感兴趣的爱好者,这套报告合集都将为您提供宝贵的信息和启示。
这份完整版的大模型 AI 学习资料已经上传CSDN,朋友们如果需要可以微信扫描下方CSDN官方认证二维码免费领取【保证100%免费
】
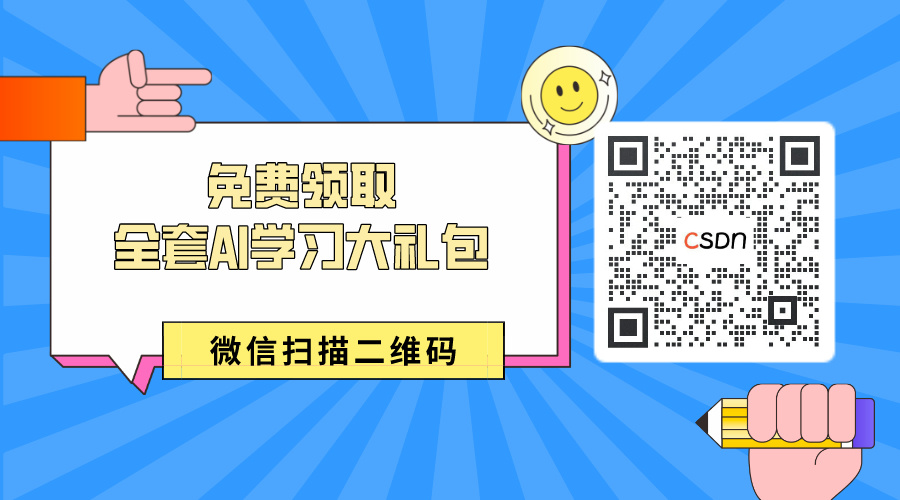